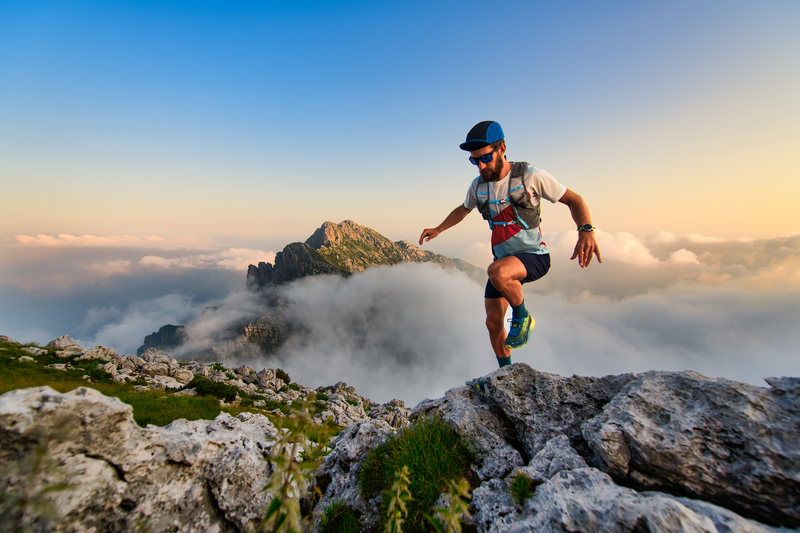
94% of researchers rate our articles as excellent or good
Learn more about the work of our research integrity team to safeguard the quality of each article we publish.
Find out more
ORIGINAL RESEARCH article
Front. Psychiatry , 23 August 2023
Sec. Digital Mental Health
Volume 14 - 2023 | https://doi.org/10.3389/fpsyt.2023.1227261
This article is part of the Research Topic Intelligent Approaches to Smart Mental Health Monitoring and Management: Implementation, Validation and Promotion View all 8 articles
Objective: The number of research into new cognitive assessment tools has increased rapidly in recent years, sparking great interest among professionals. However, there is still little literature revealing the current status and future trends of digital technology use in cognitive assessment. The aim of this study was to summarize the development of digital cognitive assessment tools through the bibliometric method.
Methods: We carried out a comprehensive search in the Web of Science Core Collection to identify relevant papers published in English between January 1, 2003, and April 3, 2023. We used the subjects such as “digital,” “computer,” and “cognitive,” and finally 13,244 related publications were collected. Then we conducted the bibliometric analysis by Bibliometrix” R-package, VOSviewer and CiteSpace software, revealing the prominent countries, authors, institutions, and journals.
Results: 11,045 articles and 2,199 reviews were included in our analyzes. The number of annual publications in this field was rising rapidly. The results showed that the most productive countries, authors and institutions were primarily located in economically developed regions, especially the North American, European, and Australian countries. Research cooperation tended to occur in these areas as well. The application of digital technology in cognitive assessment appealed to growing attention during the outbreak of the COVID-19 epidemic.
Conclusion: Digital technology uses have had a great impact on cognitive assessment and health care. There have been substantial papers published in these areas in recent years. The findings of the study indicate the great potential of digital technology in cognitive assessment.
Around 55 million people worldwide live with dementia, and this number is set to rise to 139 million by 2050 (1). Besides the ever-increasing number of patients, it is worth attention that up to three-quarters of those with dementia worldwide have not received a diagnosis (1). The clinical diagnosis of dementia or mild cognitive impairment is based on a comprehensive assessment framework backed with neurocognitive, neuroradiological, and biochemical evidence (2, 3). As the core clinical criteria for dementia, cognitive screening and monitoring are helpful to formulate clinical treatment plans and determine clinical staging, thus providing an objective basis for clinical diagnosis and treatment (4). Standardized cognitive tests are recommended by some official guidelines to diagnose cognitive disorders and assess their severity (5). A formal neuropsychological assessment always consists of the assessment for various domains of cognitive functions, including memory, language, attention, or executive function.
Traditional cognitive measures, the most common approach in clinical practice, are based on paper-and-pencil assessments. Nowadays, modern health care and medicine are characterized by continuous digital innovation (6). Technological advances with transformative potential prompt the evolvement of cognitive assessment instruments. Digital cognitive assessment technology facilitates repeated and continuous assessments and the collection of clinical data, much more convenient and cost-effective than paper-and-pencil assessments (7). Moreover, the growth of older adult tech-adoption and the outbreak of the COVID-19 pandemic necessitate digital cognitive assessment (8). Digital technology uses in cognitive management are thriving and gaining in popularity. This year, China issued clinical practice guidelines targeting the application of electronic assessment tools and digital auxiliary equipment to the management of cognitive disorders (9). This guideline paves the way for health care about cognition from assisting with diagnosis, recording results, clinical follow-up, and patient education, to referral and consultation. Given the numerous related publications and the growing research interest, it is essential to critically examine and analyze the existing studies to gain a comprehensive understanding of these domains.
Bibliometric analysis is an objective and quantitative method for exploring and analyzing large volumes of scientific data in rigorous ways (10). It evaluates research impact, identifies gaps that require further research, and provides a useful tool for decision-making in academia and industry. In the field of medical healthcare, bibliometric analysis enables researchers, clinicians, and healthcare policymakers to collect information about a specific field and shed light on the emerging areas in that field, while promoting interdisciplinary collaborations (10–13).
Many studies have used bibliometric methods to study progress in the application of digital technologies in medicine or health care (6, 14). There is, however, a paucity of studies providing a holistic snapshot of advances in digital technology on cognitive management, not to mention the cognitive assessment tools, regardless of numerous published literature on this scientific area. The aim of this study was to provide a comprehensive picture of the research related to digital cognitive tests from 2003 to 2023 by bibliometric methods.
The American Academy of Clinical Neuropsychology (AACN) and the National Academy of Neuropsychology (NAN) define digital neuropsychological assessment tools as devices which utilize a computer, digital tablet, handheld device, or other digital interface instead of a human examiner to administer, score, or interpret tests of brain function and related factors relevant to questions of neurologic health and illness (15). Patients perform the cognitive tests in the man–machine interface through a keyboard, voice, mouse, or touch screen, instead of directly interacting with a person. The use of computerized devices increases the accessibility to neuropsychological assessment for patients when professional neuropsychological services are scarce. These potential advantages accelerate the use of computerized testing in research, clinical trials and clinical practice. In addition to technology, society influences the practice of cognitive assessment as well. Although the AACN and the NAN outlined appropriate standards for the development of digital assessment tools development back in 2012 (15), the field had not significantly advanced as expected for the next years (16). The COVID-19 pandemic occasionally imposed the halt of scheduled clinical activities, forcing clinicians to arouse a renewed interest in the use of digital tools as alternative strategies (17). On the other hand, due to the various digital technologies for management rolled out by the government during the pandemic, many older adults had access to digital services. In the first 3 months after the outbreak of the COVID-19, the number of middle-aged and older adult internet users in China increased by 61 million, a number that had not been achieved in the prior 10 years (18). Familiar with digital devices, people are prone to accept the computerized cognitive assessments.
The main cognitive assessment tools on the market are divided into three categories (7) (Figure 1). The first category is the digital version of the existing pen-and-paper conventional tests, like the electronic version of the Montreal cognitive assessment (eMoCA) (19) and the digital Clock Drawing Tests (dCDT) (20, 21). These traditional cognitive measures are programmed for computer administration, and often target specific cognitive domains (15).
Second is new computerized neuropsychological products or new test batteries (22, 23), which are specifically designed for screening, comprehensive assessment or diagnostic purposes (16). These assessment tools, targeting several cognitive domains, include the Geras Solutions Cognitive Test (GSCT) (22), CogState (24), Computerized Cognitive Screen (CoCoSc) (25), Inoue (26) and the Cambridge Neuropsychological Test Automated Battery (CANTAB) (27).
The last is the use of new data streams for cognitive assessment specially designed for computers or other mobile platforms, always embedded with new types of technologies (7). Some games are developed based on the technology of virtual reality (VR) and spatial navigation (8). By watching players’ performance on tasks of various complexity in virtual space during these games, the researchers could measure participants’ cognitive function (28). For example, Sea Hero Quest, a video game designed for aiding the early diagnosis of Alzheimer’s disease, quantifies impairments in navigation performance. Participants were required to navigate a boat to the goal locations in a virtual environment like lakes or rivers. Navigation ability in the real world can be predicted from the results according to performance in this game. More importantly, the validity of this game has been proved in the two cities, London and Paris (29).
Digital cognitive assessment tools have been developed to replace and improve upon traditional cognitive measures, but their development remains in the early stages (30). A range of issues need to be solved. First, high concordance may not be guaranteed after simply converting traditional pen-and-paper tests into the digital versions (15, 16). Once a pen-and-paper test has been digitized, it has become a new one. Researchers must investigate the equivalence of computer tests and paper-and-pencil tests (31). Second, for new tests or batteries, the majority of present studies still focus on their reliability and validity, with small and limited samples (7). Third, rapid obsolescence of particular tests and test norms has to be considered as a result of fast iterations over time in both hardware and software (32). Additionally, various and disparate psychometric analyzes make comparison extra difficult, as well as data transmission, especially across digital device classes. Clinicians always have difficulty interpreting the reported results from different new neuropsychological tests. Therefore, diagnostic errors and poor clinical decisions could potentially arise. Another important consideration is that their application scenarios are narrowly focused on a few specific places, like hospitals, community clinics, psychological counseling institutions, physical examination centers and specialized experience centers (18). The limited application scenarios seriously restrict user accessibility and reduce adherence. These difficulties hinder the widespread application of digital devices for the detection, diagnosis and monitoring of cognitive disorders (16).
The Web of Science Core Collection (WoSCC) was selected as the data source in this study. The WoSCC database is a comprehensive, systematic, and authoritative database with approximately 10,000 prestigious and high-impact academic journals (12). The WoSCC database has now been commonly used for bibliometric analyzes and scientometric visualization (33). The search for potential publications was conducted on April 5, 2023. The search term “cognitive assessment” was searched in the Medical Subject Headings (MeSH: https://www.ncbi.nlm.nih.gov/mesh) of PubMed to obtain related MeSH terms. Other vocabularies in our search were derived from the published literature or based on common knowledge. Then these terms were used to perform advanced searches on WoSCC, with editions set as SCI-EXPANDED and SSCI. The publication time ranged from January 1, 2003 to April 3, 2023. The type of documents was confined to “articles” and “reviews” published in English. The retrieval results were exported in plain text format and tab-delimited file with the content “Fully Recorded and Cited References.” The detailed search strategy is presented in Supplementary Appendix.
Bibliometric analysis was conducted in this study with the aim to provide a holistic view of digital neurocognitive tests. The following characteristics were described: annual publications and their trends, as well as the most prolific countries/regions, institutions, authors and their cooperation networks. To analyze research status and current hotspots, the study also described clustered networks of co-cited references and keywords, and identified the keywords and references with the highest citation bursts. Data were mostly visualized by three tools, namely, “Bibliometrix” R-package (R version 4.1.2; Bibliometrix version 4.1.2) (34, 35), VOSviewer (version 1.6.19) (36), and CiteSpace (version 6.1. R6) (37). All information on the data has been exported into Microsoft Office Excel or R software to analyze. The annual publications and growth trends (Figure 2) were generated by Microsoft Excel 2019 (38). “Bibliometrix,” a package developed in the R language, was used to conduct basic bibliometric analyzes, such as summarizing the number of publications, the most productive countries, sources, affiliations and authors. VOSviewer is a software tool for visualizing and exploring network data in bibliometric analysis (36). In this study, data were imported into VOSviewer for further detailed analyzes like creating a co-authorship network and clustering the authors’ keywords. VOSviewer was also used to identify the top 20 recurring author keywords and references. CiteSpace, produced by Chaomei Chen (37), was used to perform reference cocitation and burst analyzes. In this study, NP stands for the numbers of publications, and TC stands for total citations. Cluster analysis results were evaluated using the Modularity (Q-score) and Silhouette (S-score) coefficients. The Q-score measures network clusters, and a score greater than 0.3 indicates significant clustering. The S-score confirms consistency among data clusters, with coefficients of 0.3, 0.5, or 0.7 indicating a homogeneous, reasonable, or extremely credible network, respectively (39).
Figure 2. Distribution of the annually published documents from 2003 to 2022. y is the annual publications and x is the year rank.
A total of 13,244 records about digital assessment tools of cognition were published from January 2003 to April 2023, including 11,045 articles and 2,199 reviews. We counted the number of annual papers in this field. Figure 2 shows the number and trend of the annual publications. The annual growth rate was 5.51%. As is shown in Figure 2, during the past two decades, the number of articles has increased exponentially, growing from 104 articles in 2003 to 1,761 in 2022. Between 2003 and 2012, the annual paper output for electronic cognitive testing was less than 500, whereas the number of annual publications in 2019 exceeded 1,000 for the first time, showing the rapid growth in the research of electronic measures for testing cognition. Additionally, the fitting curve reflects an exponential relationship between the number of articles and the publication year (excluding 2023) (R2 = 0.996). If this exponential growth continued, there would be roughly 2,152 related papers published in 2023. It appears that digital cognitive assessment is one of the popular hot topics that attract a lot of interest from academics. Other information can be found in Supplementary Appendix.
Over 110 countries made contributions to the research of digital cognitive tests. Table 1 shows the top 10 countries and institutions with the largest contribution. The United States ranked first with a total of 3,953 publications, followed by China (1,132), the United Kingdom (1,129), Australia (830), Germany (764) and Canada (735). Publications from these countries account for more than 75% of the total output. International cooperation between countries is shown in Figure 3. The United States was the most active country. The most frequent cooperation was between the United States and the United Kingdom (frequency = 335), followed by the cooperation between the USA and Canada (frequency = 306), and between the United States and Australia (frequency = 265). As is depicted in the Figure 3, most of the research collaborations occurred among the countries in North America, European, East Asia and Australia.
University of Toronto (450 papers) was the institution that published the most productions, followed by University of Pittsburgh (318 papers), University of Melbourne (315 papers), University of Pennsylvania (312 papers), King’s College London (310 papers) and University of Michigan (300 papers) (Table 1). Of the top 10 most productive institutions, 5 were located in the United States (University of Pittsburgh, University of Pennsylvania, University of Michigan, Harvard Medical School and University of California San Francisco), indicating that institutions from the United States were more actively involved in the study of cognitive assessment.
More than 55,000 authors contributed to the studies on digital testing tools. The contributions of the top 10 authors are shown in Table 2. Ruben C. Gur from University of Pennsylvania contributed the most in this field, with a total of 71 articles (H-index = 25). Gerhard Andersson ranked first in terms of authors’ local impact with an H-index of 29. Figure 4 shows the cooperation between researchers from various institutions. The minimum number of documents per author was set as 8, and 166 authors were included in the analysis. There were 14 clusters, but the links between different clusters were relatively sparse, which revealed the lack of full cooperation or communication between research teams or labs in this area.
The articles on digital cognitive assessment were published across a wide range of 2,666 journals. These jurnals were inclusive multidisciplinary journals, or professional journals classified into psychology, neurology and so on. Additionally, most articles were published in open access journals. Table 3 lists the top 15 journals by the number of articles published. Journals published in the way of open-access tend to publish more articles and obtain a higher number of total citations than non–open access journals (12).
Table 4 displays the 15 most cited articles from the total of 13,244 documents in this study. They were published from 2003 to 2015. The citations of recently published studies were low compared with those of earlier publications. This may lead to the underestimation of the importance of the new publications (40). Of these articles, the top two articles were clinical studies to test the validity of two computerized neurocognitive test batteries, CNS Vital Signs (CNSVS) (41) and CogState (42). Two reviews about assessing or detecting cognitive changes employing computerized testing in the elderly were also highly cited in this field (43, 44), indicating that using digital technology for early detection of changes in cognition in the aging population was the concern of academia. Apart from this, five of these 15 high-cited articles were relevant to sport-related concussion and cognition assessment methods (45–49). Digital neuropsychological testing in the management of sport-related concussions was also a hot topic.
Table 4. The top 15 cited articles related to digital cognitive assessment research from 2003 to 2023.
A total of 23,299 keywords were extracted from the 13,244 articles. Following a previous study (40), keywords with similar meanings were merged and keywords with general meaning were filtered out manually. Only keywords with a minimum of occurrences as 60 were visualized, and at last, 90 keywords met this threshold and were visualized by Vosview (Figures 5A–C). The occurrence frequency of keywords was presented by the size of nodes, while the strength between two words was presented by the distance between two nodes (Figure 5A). The most frequently used keywords were “cognition,” “dementia,” “depression,” “assessment,” “executive function” “Alzheimer’s disease,” and “mild cognitive impairment” and so on (Table 5 and Figure 5A). The color of each circle indicates which cluster it belongs to. Keywords with higher correlations were classified into the same cluster with the same color, which roughly reflected the focus of recent research (40). In this study, selected keywords were roughly divided into five clusters. Cluster 1 is colored in red, with the main keywords focusing on mental health and digital assessment during the COVID-19 pandemic, including terms like “depression,” “anxiety,” “COVID-19,” “digital health” and “e-health.” Cluster 2 in green focuses on different cognition domains, such as “attention,” “executive function,” “memory” and “working memory.” Cluster 3 in dark blue color focuses on cognitive assessment, like “reliability” and “assessment.” Cluster 4 in yellow color focuses on cognitive diseases, including “dementia,” “Alzheimer’s disease” and “mild cognitive impairment.” Cluster 5 in purple color focuses on psychosis with the main keywords “psychosis,” “bipolar disorder” and “schizophrenia.” Cluster 6 in light blue color emphasizes cognitive dysfunction and rehabilitation. Figure 5B displays the overlay visualization of author keywords, in which the terms in blue color appeared earlier and the terms in yellow color appeared recently. Keywords, such as “concussion,” “memory,” “assessment” and “cognition” were the past major topics, while the topics about “mental health,” “depression,” “meta-analysis,” “COVID-19,” “digital health,” and “smartphone” have been popular in recent years. Figure 5C is a density visualization map of included keywords using VOSviewer. The color of a point is closer to yellow when the keyword has a higher degree of attention, and conversely, it is closer to blue. “Cognition,” “dementia,” “depression” have gain the most attention.
Figure 5. Analysis of co-occurring author keywords about digital cognitive assessment. (A) Network visualization of author keywords. The size of the label and the circle of an item is determined by the occurrence frequency of keywords. The color of an item is determined by the cluster to which the item belongs. (B) Overlay visualization of author keywords. The color of an item is determined by the time of appearance. (C) An item density visualization map of author keywords. The color of a point is closer to yellow when there are more keywords with higher weights in its neighborhood, and it is closer to blue when there are fewer keywords with lower weights in its neighborhood. (D) Visualization map of top 20 keywords with the strongest citation bursts.
Burst keyword detection was performed in the Citespace software in order to identify the emerging concepts cited frequently (38). Burst keyword detection identifies abrupt fluctuations in the frequency or occurrence of particular keywords or phrases during a certain period (50). Figure 5D is a visualization map of the top 20 keywords with the strongest citation bursts from 2003 to 2023. Over the past two decades, mental health ranked first with the highest burst strength (42.36), followed by schizophrenia (38.48), systematic review (37.48) and Internet (32.07). Mental health, care and risk factor burst from 2020 to 2023, which may be the current research hotspots. According to the results of the “Trend Topic” analysis in the Bibliometrix, terms including “deep learning,” “natural language processing,” and “machine learning,” were also the “hot words” in this area (Figure 6). In 2016, the research on machine learning was started and lasted until 2021. From 2020, the work on deep learning and natural language processing arose. This means that there has been research on the application of artificial intelligence technology to the measurement of cognitive ability in recent years.
Figure 6. Annual topic trend presented by the Bibliometrix. In 2016, the research on machine learning was started and lasted until 2021. From 2020, the work on deep learning and natural language processing arose.
Reference citation burst detection usually revealed a work of great potential or interest and hit a key part of the complex system in the academic field (38). The 10 most cited references are shown in Table 6, and Figure 7 illustrates the top 25 references with the highest citation burst. The minimum duration of the burst was 2 years, while the blue line represents the observed time interval from 2003 to 2023 and the red line represents the burst duration. Of these articles, the methodological article entitled “Preferred reporting items for systematic reviews and meta-analyzes: the PRISMA statement” written by David Moher has the highest burst strength (57.14). Moreover, the citation burst for several papers is still ongoing, such as Carlbring et al. (51), Cogn Behav Therapy (18.03), Jack et al. (52), Alzheimers Dement (25.23), Koo et al. (7), Innov Aging (15.1) and Livingston et al. (53), Lancet (17.23). These papers covered the academic fields of meta-analysis, cognitive behavior therapy, the diagnosis of Alzheimer’s disease or milf cognitive impairment, dementia prevention and intervention, which suggests that such research topics are likely to remain popular in the future and may become potential frontiers in the research field of cognitive assessment.
Figure 7. Top 25 references with the highest citation bursts. The minimum duration of the burst was 2 years, while the blue line represents the observed time interval from 2003 to 2023 and the red line represents the burst duration.
Co-cited references, simultaneously cited by other 2 publications, represent the scientific relevance of publications (54). Document co-citation analysis, the most representative analysis function of CiteSpace, can evaluate the correlation between documents. By analyzing the co-citations of cited references, the background and knowledge base of digital cognitive assessment can be discovered. Clusters were constructed based on keywords extracted from references using log-likelihood ratio through CiteSpace. After clustering of co-cited references, 1,327 nodes, 3,028 edges, and 238 main clusters were acquired by the log-likelihood ratio algorithm in the CiteSpace software. We presented the 11 clusters (Figure 8A) and their timelines for each cluster label (Figure 8B). The 11 largest clusters were “covid-19 (Cluster #0, size = 146, Silhouette = 0.915),” “concussion (Cluster #1, size = 113, Silhouette = 0.950),” “cognitive training (Cluster #2, size = 97, Silhouette = 0.901),” “mild traumatic brain injury (Cluster #3, size = 88, Silhouette = 0.936),” “depression (Cluster #4, size = 85, Silhouette = 0.959),” “prefrontal cortex (Cluster #5, size = 57, Silhouette = 0.918),” “clinical trial (Cluster #6, size = 56, Silhouette = 0.879),” “spatial working memory (Cluster #7, size = 55, Silhouette = 0.919),” “cognitive behavior therapy (Cluster #8, size = 48, Silhouette = 0.945),” “spinal cord injuries (Cluster #9, size = 38, Silhouette = 0.956),” and “machine learning (Cluster #10, size = 26, Silhouette = 1).” According to the results, the cluster structure was significant and highly reliable, with a total modularity Q of 0.8673 and a weighted mean Silhouette of 0.9345. The position of the nodes in these clusters suggests the pioneering research. For example, clusters #1, #3 and #4 study the clinical application of cognitive asssessment. Clusters #2 and #8 study the therapy. Cluster #0 and #10 are related to popular topics in recent years. The position of the nodes in these clusters suggests the pioneering research focus.
Figure 8. Co-citation references network and correspondent clustering analysis obtained with CiteSpace. (A) Network visualization of the results of the cluster analysis of highly co-cited references in the field of digital cognitive assessment. (B) Timeline diagram of cluster analysis in panel A.
We conducted a bibliometric analysis, providing a thorough overview of international research on digital cognitive assessment from 2003 to 2023. Our study contained more than 13 thousand articles from 55,490 authors published in 2,666 journals and 480,837 references. Interest in this topic has grown rapidly over the preceding two decades. The findings show that a total of 13,244 articles have been published. The trend substantially increased in recent years, and it is estimated that this growth will continue in the next few years. This serves as a reminder that electronic cognitive assessment tools are expected to be widely used in clinical situations. This trend is also consistent with the widespread use of digital devices in other clinical settings (55–57). In terms of literature output, the United States was a highly productive country in this field. China, the United Kingdom, Germany, Canada and Australia have a dominant position in this field as well. Half of the 10 most productive institutions and authors were from the United States, indicating that the United States was the leading country in this field.
The United States is not only the most productive country, but also serves as the hub of global trade. In the area of digital cognitive evaluation, close research collaboration has increased among the top 10 most productive countries. The United States established strong research collaboration with European countries. Moreover, most of the research collaborations occurred among the countries in North America, European, East Asia and Australia. Academic capability, to a large extent, depends on the governmental economic status and its expenditure on healthcare (58). The majority of these countries are economic powers with strong scientific and technological capabilities. They are the global leaders in electronic information science and technology, making it possible for them to conduct research in this field.
Another potential incentive for their cooperation may be the reality of an aging population they had to cope with (59). According to the World Population Prospects 2022 unveiled by the United Nations (60), Europe and Northern America had the largest proportion of the older population, followed by Australia. What’s more, one in every four persons living in Europe and Northern America could be aged 65 or over (60). Elderly people frequently have dementia and other forms of cognitive impairment since becoming older is still the biggest risk factor for dementia (61). In the following decades, dementia sufferers will become more prevalent as the population ages (62). In an aging population, dementia prevention is a public health issue that cannot be disregarded (53). There is a great demand for more accurate, convenient and fast cognitive measurement tools. That may explain why researchers in these countries put so much effort into studies assessing cognitive ability.
At the same time, it should be noted that, apart from China, there are fewer studies from developing countries, and less national collaboration between developing countries and developed countries. Another advantage of digitized cognitive assessment tools is the lower requirement for extra space and professional personnel, allowing remote evaluation, self-assessment or self-management outside of health care (7). This demonstrates how useful electronic instruments might be in rural or underdeveloped areas, particularly where there are few medical resources and a high population density (63). Therefore, the cooperation between developed and developing countries is expected to become closer in the future.
The collaborative relationship between the authors was also a focus of this study. The analysis about co-authorship between researchers is helpful to identify existing partnerships and explore potential collaborators (58). Similar to the findings about regional distribution, several institutions and authors from North America, Western Europe, East Asia and Australia published widely on this topic. The results show that there is a certain degree of cooperative networks among researchers in this field, but most of this cooperation is concentrated within a few groups or teams. Since there are not numerous links between different academic institutions or groups, more chances for researchers from various fields to work together should be offered.
Finding hotspots is a crucial component of bibliometric analysis, which foretells potential study ideas. Future investigations are always guided by the existing hotspots and trends. The essential idea and content of one article are reflected in keywords, which are highly compressed and generalized words. Our findings showed that concussion was a hot topic in the past in this field. These findings are confirmed by the fact that many of the established digital tools were originally designed to assess mild traumatic brain injury or concussion in military and sports psychology (16). But now, the most frequently used keywords focused on terms related to cognitive function and cognitive disorders, including “Alzheimer’s disease” and “mild cognitive impairment.” This demonstrates that digital tools are applied to diverse clinical groups including neurocognitive disorders. Disease diagnosis and severity evaluation are the principal uses of digital cognitive assessment tools nowadays. Apart from this, other frequently used keywords focused on terms related to mood disorders, like depression and anxiety, and appeared with the keyword “COVID-19.” The burst detection analysis about keywords also implied that mental health care was actively discussed in the past few years. This reflects the increased attention that researchers are paying to mental health during the COVID-19 pandemic (64–66). Previous studies indicated that the COVID-19 pandemic had contributed to over 25% increase in the prevalence of anxiety and depression globally (66). Another observational study found a relatively high frequency of cognitive impairment in executive functioning, processing speed and memory encoding among hospitalized patients who had contracted COVID-19 several months before (67). Due to the social isolation brought on by the unprecedented pandemic, traditional face-to-face cognitive assessment has grown to be very challenging. However, this public health emergency has triggered a rapid shift in the way of health care, boosting the global adoption and usage of telehealth or other electronic solutions (68, 69). This offered a critical opportunity for the popularity of electronic cognitive assessment tools (70–72). In the post-pandemic era, digital health approaches will continue to provide services for patients in neurological, psychiatric and mental health care (73).
In the part of analyzing the references, we identified several clusters using the co-occurrence clustering function, with each cluster representing a main theme or topic. Some references with high citations were clustered in the domains of machine learning. Besides, according to the results from the “Bibliometrix” package, “machine learning,” “deep learning” and “natural language processing” were also the main trend topics in the last 4 years. In recent years, numerous complicated medical problems have been solved using machine learning (ML) and other artificial intelligence (AI) techniques (74). ML builds prediction models with high accuracy, and thus enhances the diagnostic performance of many diseases, mainly in cancer, medical imaging and wearable sensors (74). Deep learning, a branch of ML, has quickly become the method of choice for assessing brain imaging, like functional magnetic resonance imaging (fMRI) and electroencephalogram (EEG) (74). At a cognitive and behavioral level, ML systems can extract reliable features originating from the neuropsychological assessment, automatically classify different disease phenotypes, identify development stages, and even predict disease conversion (75, 76). With the emergence of studies on the utilization of ML algorithms to neuropsychological tests and the ever-upgrading algorithms (75, 77), electronic tools that integrate detection, diagnosis, cognitive training and therapy would be a promising direction in clinical practice.
In this study, we provide a comprehensive picture of the basic research information in the field of computerized cognitive assessment. The emergence of digital technologies has opened up a new era for cognitive testing tools. Although some discoveries can be found in our analysis, there are still several inevitable limitations. Similar to the majority of bibliometrics articles, data were solely collected from the English articles or reviews included in WoSCC databases. This is partly due to the limitations of scientometric software (37), because it is extremely difficult to directly merge the data from two different databases, such as Scopus and Embase (40). So some excellent publications might have been missed, like gray articles, meeting abstracts and patent materials.
This bibliometric analysis presented the overall structural framework and identified the key perspectives of the research on digital cognitive assessment. In conclusion, research into this field is accelerating rapidly, receiving growing attention in the past decades. Assisting cognitive assessment in mental health disorders using computer-assisted tools is the current hotspot. Researchers and institutions from America are the top contributors to this topic. America, Canada and other high-income countries or regions represent the main force regarding this domain. Digital evaluation technology is on the ascendant. Our findings will assist researchers and policymakers in grasping the basic research status and formulating the plan for the future.
The original contributions presented in the study are included in the article/Supplementary material, further inquiries can be directed to the corresponding author/s.
LC designed this study, performed the statistical analysis, and drafted the manuscript. WZ and DP revised the manuscript. All authors contributed to the article and approved the submitted version.
This research was funded by the Central Health Research Project (grant number 2020ZD10), and the National Key R&D Program of China (grant number 2022YFC2010103).
The authors thank Xin Li, Yuye Wang and Jiajia Tang for their theoretical guidance on this study.
The authors declare that the research was conducted in the absence of any commercial or financial relationships that could be construed as a potential conflict of interest.
All claims expressed in this article are solely those of the authors and do not necessarily represent those of their affiliated organizations, or those of the publisher, the editors and the reviewers. Any product that may be evaluated in this article, or claim that may be made by its manufacturer, is not guaranteed or endorsed by the publisher.
The Supplementary material for this article can be found online at: https://www.frontiersin.org/articles/10.3389/fpsyt.2023.1227261/full#supplementary-material
1. Gauthier, S, Webster, C, Servaes, S, and Morais, JA. (2022). World Alzheimer report 2022: life after diagnosis: navigating treatment, care and support. Alzheimer’s Disease International. Available at: (https://www.alzint.org/reports-resources/).
2. Mukaetova-Ladinska, EB, De Lillo, C, Arshad, Q, Subramaniam, HE, and Maltby, J. Cognitive assessment of dementia: the need for an inclusive design tool. Curr Alzheimer Res. (2022) 19:265–73. doi: 10.2174/1567205019666220315092008
3. NICE. "National Institute for Health and Care Excellence: Guidelines," in Dementia: Assessment, management and support for people living with Dementia and their Carers. London: National Institute for Health and Care Excellence (NICE) Copyright © NICE 2018 (2018).
4. Arvanitakis, Z, Shah, RC, and Bennett, DA. Diagnosis and Management of Dementia: review. JAMA. (2019) 322:1589–99. doi: 10.1001/jama.2019.4782
5. Petersen, RC, Lopez, O, Armstrong, MJ, Getchius, TSD, Ganguli, M, Gloss, D, et al. Practice guideline update summary: mild cognitive impairment: report of the guideline development, dissemination, and implementation Subcommittee of the American Academy of neurology. Neurology. (2018) 90:126–35. doi: 10.1212/wnl.0000000000004826
6. Yeung, AWK, Kulnik, ST, Parvanov, ED, Fassl, A, Eibensteiner, F, Völkl-Kernstock, S, et al. Research on digital technology use in cardiology: bibliometric analysis. J Med Internet Res. (2022) 24:e36086. doi: 10.2196/36086
7. Koo, BM, and Vizer, LM. Mobile Technology for Cognitive Assessment of older adults: a scoping review. Innov Aging. (2019) 3:igy038. doi: 10.1093/geroni/igy038
8. Öhman, F, Hassenstab, J, Berron, D, Schöll, M, and Papp, KV. Current advances in digital cognitive assessment for preclinical Alzheimer's disease. Alzheimers Dement (Amst). (2021) 13:e12217. doi: 10.1002/dad2.12217
9. Health, C.A.o.G.a.G.C.N.a. Chinese clinical practice guidelines for digital memory clinic. Zhonghua Yi Xue Za Zhi. (2023) 103:1–9. doi: 10.3760/cma.j.cn112137-20221024-02218
10. Donthu, N, Kumar, S, Mukherjee, D, Pandey, N, and Lim, WM. How to conduct a bibliometric analysis: An overview and guidelines. J Bus Res. (2021) 133:285–96. doi: 10.1016/j.jbusres.2021.04.070
11. Ahmadvand, A, Kavanagh, D, Clark, M, Drennan, J, and Nissen, L. Trends and visibility of digital health as a keyword in articles by JMIR publications in the new millennium: bibliographic-bibliometric analysis. J Med Internet Res. (2019) 21:e10477. doi: 10.2196/10477
12. Wu, CC, Huang, CW, Wang, YC, Islam, MM, Kung, WM, Weng, YC, et al. mHealth research for weight loss, physical activity, and sedentary behavior: bibliometric analysis. J Med Internet Res. (2022) 24:e35747. doi: 10.2196/35747
13. Yeung, AWK, Tosevska, A, Klager, E, Eibensteiner, F, Laxar, D, Stoyanov, J, et al. Virtual and augmented reality applications in medicine: analysis of the scientific literature. J Med Internet Res. (2021) 23:e25499. doi: 10.2196/25499
14. Waqas, A, Teoh, SH, Lapão, LV, Messina, LA, and Correia, JC. Harnessing telemedicine for the provision of health care: bibliometric and Scientometric analysis. J Med Internet Res. (2020) 22:e18835. doi: 10.2196/18835
15. Bauer, RM, Iverson, GL, Cernich, AN, Binder, LM, Ruff, RM, and Naugle, RI. Computerized neuropsychological assessment devices: joint position paper of the American Academy of clinical neuropsychology and the National Academy of neuropsychology. Clin Neuropsychol. (2012) 26:177–96. doi: 10.1080/13854046.2012.663001
16. Gates, NJ, and Kochan, NA. Computerized and on-line neuropsychological testing for late-life cognition and neurocognitive disorders: are we there yet? Curr Opin Psychiatry. (2015) 28:165–72. doi: 10.1097/YCO.0000000000000141
17. Salvadori, E, and Pantoni, L. Teleneuropsychology for vascular cognitive impairment: which tools do we have? Cereb Circ Cogn Behav. (2023) 5:100173. doi: 10.1016/j.cccb.2023.100173
18. Chen, H, Hagedorn, A, and An, N. The development of smart eldercare in China. Lancet Reg Health West Pac. (2023) 35:100547. doi: 10.1016/j.lanwpc.2022.100547
19. Berg, JL, Durant, J, Léger, GC, Cummings, JL, Nasreddine, Z, and Miller, JB. Comparing the electronic and standard versions of the Montreal cognitive assessment in an outpatient memory disorders clinic: a validation study. J Alzheimers Dis. (2018) 62:93–7. doi: 10.3233/JAD-170896
20. Chan, JYC, Bat, BKK, Wong, A, Chan, TK, Huo, Z, Yip, BHK, et al. Evaluation of digital drawing tests and paper-and-pencil drawing tests for the screening of mild cognitive impairment and dementia: a systematic review and Meta-analysis of diagnostic studies. Neuropsychol Rev. (2022) 32:566–76. doi: 10.1007/s11065-021-09523-2
21. Yuan, J, Au, R, Karjadi, C, Ang, TF, Devine, S, Auerbach, S, et al. Associations between the digital clock drawing test and brain volume: large community-based prospective cohort (Framingham heart study). J Med Internet Res. (2022) 24:e34513. doi: 10.2196/34513
22. Bloniecki, V, Hagman, G, Ryden, M, and Kivipelto, M. Digital screening for cognitive impairment - a proof of concept study. J Prev Alzheimers Dis. (2021) 8:127–34. doi: 10.14283/jpad.2021.2
23. De Roeck, EE, De Deyn, PP, Dierckx, E, and Engelborghs, S. Brief cognitive screening instruments for early detection of Alzheimer's disease: a systematic review. Alzheimers Res Ther. (2019) 11:21. doi: 10.1186/s13195-019-0474-3
24. Maruff, P, Lim, YY, Darby, D, Ellis, KA, Pietrzak, RH, Snyder, PJ, et al. Clinical utility of the cogstate brief battery in identifying cognitive impairment in mild cognitive impairment and Alzheimer's disease. BMC Psychol. (2013) 1:30. doi: 10.1186/2050-7283-1-30
25. Wong, A, Fong, CH, Mok, VC, Leung, KT, and Tong, RK. Computerized cognitive screen (CoCoSc): a self-administered computerized test for screening for cognitive impairment in community social centers. J Alzheimers Dis. (2017) 59:1299–306. doi: 10.3233/JAD-170196
26. Inoue, M, Jinbo, D, Nakamura, Y, Taniguchi, M, and Urakami, K. Development and evaluation of a computerized test battery for Alzheimer's disease screening in community-based settings. Am J Alzheimers Dis Other Dement. (2009) 24:129–35. doi: 10.1177/1533317508330222
27. Backx, R, Skirrow, C, Dente, P, Barnett, JH, and Cormack, FK. Comparing web-based and lab-based cognitive assessment using the Cambridge neuropsychological test automated battery: a within-subjects counterbalanced study. J Med Internet Res. (2020) 22:e16792. doi: 10.2196/16792
28. Allison, SL, Fagan, AM, Morris, JC, and Head, D. Spatial navigation in preclinical Alzheimer's disease. J Alzheimers Dis. (2016) 52:77–90. doi: 10.3233/JAD-150855
29. Coutrot, A, Schmidt, S, Coutrot, L, Pittman, J, Hong, L, Wiener, JM, et al. Virtual navigation tested on a mobile app is predictive of real-world wayfinding navigation performance. PLoS One. (2019) 14:e0213272. doi: 10.1371/journal.pone.0213272
30. Wilson, S, Milanini, B, Javandel, S, Nyamayaro, P, and Valcour, V. Validity of digital assessments in screening for HIV-related cognitive impairment: a review. Curr HIV/AIDS Rep. (2021) 18:581–92. doi: 10.1007/s11904-021-00585-8
31. Schmand, B. Why are neuropsychologists so reluctant to embrace modern assessment techniques? Clin Neuropsychol. (2019) 33:209–19. doi: 10.1080/13854046.2018.1523468
32. Germine, L, Reinecke, K, and Chaytor, NS. Digital neuropsychology: challenges and opportunities at the intersection of science and software. Clin Neuropsychol. (2019) 33:271–86. doi: 10.1080/13854046.2018.1535662
33. Wang, H, Shi, J, Shi, S, Bo, R, Zhang, X, and Hu, Y. Bibliometric analysis on the Progress of chronic heart failure. Curr Probl Cardiol. (2022) 47:101213. doi: 10.1016/j.cpcardiol.2022.101213
34. Aria, M, and Cuccurullo, C. Bibliometrix: An R-tool for comprehensive science mapping analysis. J Informet. (2017) 11:959–75. doi: 10.1016/j.joi.2017.08.007
35. Darvish, H. Bibliometric analysis using Bibliometrix an R package. J Sci Res. (2020) 8:156–60. doi: 10.5530/jscires.8.3.32
36. van Eck, NJ, and Waltman, L. Software survey: VOSviewer, a computer program for bibliometric mapping. Scientometrics. (2010) 84:523–38. doi: 10.1007/s11192-009-0146-3
37. Chen, C. Cite Space: A Practical Guide for Mapping Scientific Literature. Hauppauge, NY: Nova Science Publishers (2016).
38. Han, X, Zhang, J, Chen, S, Yu, W, Zhou, Y, and Gu, X. Mapping the current trends and hotspots of vascular cognitive impairment from 2000-2021: a bibliometric analysis. CNS Neurosci Ther. (2023) 29:771–82. doi: 10.1111/cns.14026
39. Chen, C. Searching for intellectual turning points: progressive knowledge domain visualization. Proc Natl Acad Sci U S A 101 Suppl. (2004) 1:5303–10. doi: 10.1073/pnas.0307513100
40. Wu, H, Zhou, Y, Wang, Y, Tong, L, Wang, F, Song, S, et al. Current state and future directions of intranasal delivery route for central nervous system disorders: a Scientometric and visualization analysis. Front Pharmacol. (2021) 12:717192. doi: 10.3389/fphar.2021.717192
41. Gualtieri, CT, and Johnson, LG. Reliability and validity of a computerized neurocognitive test battery, CNS vital signs. Arch Clin Neuropsychol. (2006) 21:623–43. doi: 10.1016/j.acn.2006.05.007
42. Maruff, P, Thomas, E, Cysique, L, Brew, B, Collie, A, Snyder, P, et al. Validity of the cog state brief battery: relationship to standardized tests and sensitivity to cognitive impairment in mild traumatic brain injury, schizophrenia, and AIDS dementia complex. Arch Clin Neuropsychol. (2009) 24:165–78. doi: 10.1093/arclin/acp010
43. Wild, K, Howieson, D, Webbe, F, Seelye, A, and Kaye, J. Status of computerized cognitive testing in aging: a systematic review. Alzheimers Dement. (2008) 4:428–37. doi: 10.1016/j.jalz.2008.07.003
44. Zygouris, S, and Tsolaki, M. Computerized cognitive testing for older adults: a review. Am J Alzheimers Dis Other Dement. (2015) 30:13–28. doi: 10.1177/1533317514522852
45. Broglio, SP, Ferrara, MS, Macciocchi, SN, Baumgartner, TA, and Elliott, R. Test-retest reliability of computerized concussion assessment programs. J Athl Train. (2007) 42:509–14.
46. Collie, A, Maruff, P, Makdissi, M, McCrory, P, McStephen, M, and Darby, D. Cog sport: reliability and correlation with conventional cognitive tests used in postconcussion medical evaluations. Clin J Sport Med. (2003) 13:28–32. doi: 10.1097/00042752-200301000-00006
47. Iverson, GL, Lovell, MR, and Collins, MW. Validity of ImPACT for measuring processing speed following sports-related concussion. J Clin Exp Neuropsychol. (2005) 27:683–9. doi: 10.1081/13803390490918435
48. Randolph, C, McCrea, M, and Barr, WB. Is neuropsychological testing useful in the management of sport-related concussion? J Athl Train. (2005) 40:139–52.
49. Van Kampen, DA, Lovell, MR, Pardini, JE, Collins, MW, and Fu, FH. The value added of neurocognitive testing after sports-related concussion. Am J Sports Med. (2006) 34:1630–5. doi: 10.1177/0363546506288677
50. Kleinberg, J. Bursty and hierarchical structure in streams. Data Min Knowl Disc. (2003) 7:373–97. doi: 10.1023/A:1024940629314
51. Carlbring, P, Andersson, G, Cuijpers, P, Riper, H, and Hedman-Lagerlöf, E. Internet-based vs. face-to-face cognitive behavior therapy for psychiatric and somatic disorders: an updated systematic review and meta-analysis. Cogn Behav Ther. (2018) 47:1–18. doi: 10.1080/16506073.2017.1401115
52. Jack, CR Jr, Bennett, DA, Blennow, K, Carrillo, MC, Dunn, B, Haeberlein, SB, et al. NIA-AA research framework: toward a biological definition of Alzheimer's disease. Alzheimers Dement. (2018) 14:535–62. doi: 10.1016/j.jalz.2018.02.018
53. Livingston, G, Huntley, J, Sommerlad, A, Ames, D, Ballard, C, Banerjee, S, et al. Dementia prevention, intervention, and care: 2020 report of the lancet commission. Lancet. (2020) 396:413–46. doi: 10.1016/S0140-6736(20)30367-6
54. Kostoff, RN. Citation analysis of research performer quality. Scientometrics. (2002) 53:49–71. doi: 10.1023/A:1014831920172
55. Fagherazzi, G, and Ravaud, P. Digital diabetes: perspectives for diabetes prevention, management and research. Diabetes Metab. (2019) 45:322–9. doi: 10.1016/j.diabet.2018.08.012
56. Mac Kinnon, GE, and Brittain, EL. Mobile health Technologies in Cardiopulmonary Disease. Chest. (2020) 157:654–64. doi: 10.1016/j.chest.2019.10.015
57. Piau, A, Wild, K, Mattek, N, and Kaye, J. Current state of digital biomarker Technologies for Real-Life, home-based monitoring of cognitive function for mild cognitive impairment to mild Alzheimer disease and implications for clinical care: systematic review. J Med Internet Res. (2019) 21:e12785. doi: 10.2196/12785
58. Sun, HL, Bai, W, Li, XH, Huang, H, Cui, XL, Cheung, T, et al. Schizophrenia and inflammation research: a bibliometric analysis. Front Immunol. (2022) 13:907851. doi: 10.3389/fimmu.2022.907851
59. Chand, M, and Markova, GJTIBR. The European Union's aging population: challenges for human resource management. Thunderbird. Int Bus Rev. (2018) 61:519–29. doi: 10.1002/tie.22023
60. United Nations Department of Economic and Social Affairs (2022). World population prospects 2022: summary of results. Available at: https://population.un.org/wpp/Publications/ (accessed April 10 2023).
61. Hou, Y, Dan, X, Babbar, M, Wei, Y, Hasselbalch, SG, Croteau, DL, et al. Ageing as a risk factor for neurodegenerative disease. Nat Rev Neurol. (2019) 15:565–81. doi: 10.1038/s41582-019-0244-7
62. Grande, G, Qiu, C, and Fratiglioni, L. Prevention of dementia in an ageing world: evidence and biological rationale. Ageing Res Rev. (2020) 64:101045. doi: 10.1016/j.arr.2020.101045
63. Pellé, KG, Rambaud-Althaus, C, D'Acremont, V, Moran, G, Sampath, R, Katz, Z, et al. Electronic clinical decision support algorithms incorporating point-of-care diagnostic tests in low-resource settings: a target product profile. BMJ Glob Health. (2020) 5:e002067. doi: 10.1136/bmjgh-2019-002067
64. Daly, M, and Robinson, E. Depression and anxiety during COVID-19. Lancet. (2022) 399:518. doi: 10.1016/S0140-6736(22)00187-8
65. Zhu, C, Zhang, T, Li, Q, Chen, X, and Wang, K. Depression and anxiety during the COVID-19 pandemic: epidemiology, mechanism, and treatment. Neurosci Bull. (2023) 39:675–84. doi: 10.1007/s12264-022-00970-2
66. COVID-19 Mental Disorders Collaborators. Global prevalence and burden of depressive and anxiety disorders in 204 countries and territories in 2020 due to the COVID-19 pandemic. Lancet. (2021) 398:1700–12. doi: 10.1016/S0140-6736(21)02143-7
67. Becker, JH, Lin, JJ, Doernberg, M, Stone, K, Navis, A, Festa, JR, et al. Assessment of cognitive function in patients after COVID-19 infection. JAMA Netw Open. (2021) 4:e2130645. doi: 10.1001/jamanetworkopen.2021.30645
68. Garfan, S, Alamoodi, AH, Zaidan, BB, Al-Zobbi, M, Hamid, RA, Alwan, JK, et al. Telehealth utilization during the Covid-19 pandemic: a systematic review. Comput Biol Med. (2021) 138:104878. doi: 10.1016/j.compbiomed.2021.104878
69. Stanimirović, D, and Matetić, V. Can the COVID-19 pandemic boost the global adoption and usage of eHealth solutions? J Glob Health. (2020) 10:0203101. doi: 10.7189/jogh.10.0203101
70. Detweiler Guarino, I, Cowan, DR, Fellows, AM, and Buckey, JC. Use of a self-guided computerized cognitive behavioral tool during COVID-19: evaluation study. JMIR Form Res. (2021) 5:e26989. doi: 10.2196/26989
71. Mahoney, A, Li, I, Haskelberg, H, Millard, M, and Newby, JM. The uptake and effectiveness of online cognitive behaviour therapy for symptoms of anxiety and depression during COVID-19. J Affect Disord. (2021) 292:197–203. doi: 10.1016/j.jad.2021.05.116
72. Wahlund, T, Mataix-Cols, D, Olofsdotter Lauri, K, de Schipper, E, Ljótsson, B, Aspvall, K, et al. Brief online cognitive Behavioural intervention for dysfunctional worry related to the COVID-19 pandemic: a randomised controlled trial. Psychother Psychosom. (2021) 90:191–9. doi: 10.1159/000512843
73. Khanna, A, and Jones, GB. Envisioning post-pandemic digital neurological, psychiatric and mental health care. Front Digit Health. (2021) 3:803315. doi: 10.3389/fdgth.2021.803315
74. Shehab, M, Abualigah, L, Shambour, Q, Abu-Hashem, MA, Shambour, MKY, Alsalibi, AI, et al. Machine learning in medical applications: a review of state-of-the-art methods. Comput Biol Med. (2022) 145:105458. doi: 10.1016/j.compbiomed.2022.105458
75. Battista, P, Salvatore, C, Berlingeri, M, Cerasa, A, and Castiglioni, I. Artificial intelligence and neuropsychological measures: the case of Alzheimer's disease. Neurosci Biobehav Rev. (2020) 114:211–28. doi: 10.1016/j.neubiorev.2020.04.026
76. Wang, Y, Gu, X, Hou, W, Zhao, M, Sun, L, and Guo, C. Dual semi-supervised learning for classification of Alzheimer's disease and mild cognitive impairment based on neuropsychological data. Brain Sci. (2023) 13:306. doi: 10.3390/brainsci13020306
Keywords: neuropsychological tests, cognition, computerized assessment, digital cognitive assessment, bibliometric analysis
Citation: Chen L, Zhen W and Peng D (2023) Research on digital tool in cognitive assessment: a bibliometric analysis. Front. Psychiatry. 14:1227261. doi: 10.3389/fpsyt.2023.1227261
Received: 23 May 2023; Accepted: 31 July 2023;
Published: 23 August 2023.
Edited by:
Yang Zhao, Sun Yat-sen University, ChinaReviewed by:
Sorinel Capusneanu, Titu Maiorescu University, RomaniaCopyright © 2023 Chen, Zhen and Peng. This is an open-access article distributed under the terms of the Creative Commons Attribution License (CC BY). The use, distribution or reproduction in other forums is permitted, provided the original author(s) and the copyright owner(s) are credited and that the original publication in this journal is cited, in accordance with accepted academic practice. No use, distribution or reproduction is permitted which does not comply with these terms.
*Correspondence: Dantao Peng, cGVuZ2RhbnRhbzIwMDBAMTYzLmNvbQ==
Disclaimer: All claims expressed in this article are solely those of the authors and do not necessarily represent those of their affiliated organizations, or those of the publisher, the editors and the reviewers. Any product that may be evaluated in this article or claim that may be made by its manufacturer is not guaranteed or endorsed by the publisher.
Research integrity at Frontiers
Learn more about the work of our research integrity team to safeguard the quality of each article we publish.