- 1Institute of Development, Aging and Cancer, Tohoku University, Sendai, Japan
- 2Japan Society for the Promotion of Science, Tokyo, Japan
- 3Center for Information and Neural Networks (CiNet), Osaka, Japan
- 4Graduate School of Arts and Letters, Tohoku University, Sendai, Japan
- 5Faculty of Informatics, Shizuoka Institute of Science and Technology, Fukuroi, Japan
- 6International Research Institute of Disaster Science, Tohoku University, Sendai, Japan
Coronavirus disease 2019 dramatically changed people’s behavior because of the need to adhere to infection prevention and to overcome general adversity resulting from the implementation of infection prevention measures. However, coping behavior has not been fully distinguished from risk perception, and a comprehensive picture of demographic, risk-perception, and psychobehavioral factors that influence the major coping-behavior factors remain to be elucidated. In this study, we recruited 2,885 Japanese participants. Major coping-behavior and risk-perception factors were identified via exploratory factor analysis of 50 candidate items. Then, we conducted a hierarchical multiple regression analysis to investigate factors associated with each coping-behavior factor. We identified four types of coping behavior [CB1 (mask-wearing), CB2 (information-seeking), CB3 (resistance to social stagnation), and CB4 (infection-prevention)] and three risk-perception factors [RP1 (shortages of daily necessities), RP2 (medical concerns), and RP3 (socioeconomic concerns)]. CB1 was positively associated with female sex and etiquette. CB2 was positively related to RP1 and RP3. CB3 was positively related to RP1 and leadership, and negatively associated with etiquette. CB4 was positively associated with female sex, etiquette, and active well-being. This parsimonious model may help to elucidate essential social dynamics and provide a theoretical framework for coping behavior during a pandemic.
1. Introduction
Since the first case of coronavirus disease 2019 (COVID-19) was reported to the WHO, governments have spared no effort to prevent infection and transmission of this virus (1). As a health disaster (2), the response to this novel infectious disease is a public health issue that has had psychological and behavioral effects on individuals. Many studies have reported severe mental-health problems related to COVID-19, such as anxiety, depression, and suicide (3). People wore masks, disinfected their hands, and avoided crowds to prevent infection and transmission of the virus. Simultaneously, they had to manage the social disruption caused by the pandemic and precautions, such as shortages of masks and toilet paper and losses of jobs and important events. In such a stressful environment, it is vital to understand how individuals managed adversity (i.e., both infection and general adversity) to try to maintain their current standard of living.
Recent studies have found positive associations between two types of coping behaviors (infection prevention and general-adversity coping behaviors) and risk perception. For example, self-isolation was found to be positively associated with risk perception of personal safety and health services (4). Information seeking was positively associated with perceived risk at the individual and community levels (5), while behavioral change (e.g., informing others about COVID-19) was positively associated with the perceived risk of infection (6, 7). This effect of risk perception on coping behavior is consistent with findings for other types of hazards, such as hurricanes, tornadoes, earthquakes, and volcanoes (8–13).
Psychobehavioral characteristics have been analyzed in recent studies of COVID-19 coping behavior. Recent COVID-19-related studies have extensively used the Big Five scale to explore the psychological mechanisms underlying coping behavior, as the characteristics in this scale have profound implications for public health (14). Their findings suggest that the Big Five personality traits are significantly associated with infection preventive behaviors (15–24). For example, extraversion, openness, conscientiousness, and agreeableness are positively associated with infection preventive behaviors, whereas neuroticism is negatively associated with such behaviors (15, 21).
However, a consensus regarding these findings is difficult to achieve due to the lack of a common model for the main coping-behavior and risk-perception factors, leading to labels that differ in their definition, level of specificity, or conceptual overlap in different studies. For example, coping behavior has been defined at different levels of specificity, such as infection prevention measures (15) but also as problem-focused and emotion-focused coping behaviors (25). Similar risk perception labels have been used to measure different phenomena. For example, risk perception has been used to represent the degree to which people perceive COVID-19 to be a dangerous disease (26) but also as a proxy for the level of knowledge of the risks associated with COVID-19 (5). Different labels have also been used to measure similar perceptions of risk, such as the likelihood of infection (21) and concerns related to COVID-19 (15). Furthermore, coping behavior and risk perception have not been fully distinguished. Researchers have attempted to identify risk-perception factors from risk-related items rather than from a mixed pool containing coping behaviors (15).
In addition, current studies have not investigated the contribution of survival-oriented psychobehavioral characteristics to coping behaviors, which may provide more nuanced insights into individual differences in the perceptions of and responses to pandemics as a health disaster. We are interested in the Power to Live scale, which was developed in the context of the 2011 Tohoku earthquake and tsunami. This scale assesses eight psychobehavioral characteristics that are advantageous to survival: leadership, problem-solving, altruism, stubbornness, etiquette, emotional regulation, self-transcendence, and active well-being. Compared to the Big Five scale, the Power to Live scale provides a more nuanced understanding of the relationships between psychobehavioral characteristics and coping behaviors, particularly in the context of disasters (12, 27, 28).
We aimed to address two issues in this study. First, we identified major coping-behavior and risk-perception factors. Then, we examined important demographic, risk-perception, and psychobehavioral factors that contributed to coping behavior. We used a battery of questionnaires to investigate coping behaviors and risk perceptions in relation to COVID-19, as well as to obtain demographic information and measure psychobehavioral characteristics. We used the Power to Live scale based on our research interest and the Big Five scale according to previous studies (15–22). First, we conducted an exploratory factor analysis of various coping-behavior and risk-perception items to identify the major factors. Then, we examined the contributions of demographic, risk-perception (as an exploratory factor), and psychobehavioral characteristics to coping-behavior factors. We predicted that we would identify several coping-behavior and risk-perception factors associated with infection and general adversity. In addition to demographic and risk-perception factors, we hypothesized that associations would be found between coping-behavior factors and survival-oriented psychobehavioral characteristics.
2. Materials and methods
2.1. Participants
Data collection was conducted online by Neo Marketing (Tokyo, Japan) from March 19 to 24, 2020, during the early phase of the pandemic in Japan. The first coronavirus death had been reported (February 13); people had experienced the nationwide closure of elementary and junior high schools (March 2) and a national shortage of masks and toilet paper had occurred (March). The survey company emailed invitations to online crowdworkers living in all 47 prefectures of Japan. Participants were divided into six age groups (20s, 30s, 40s, 50s, 60s, and ≥70s) and two sex groups (male and female). Data were collected from 300 respondents in each sex and age group (total of 3,600 participants). All participants were required to have access to the Internet, to be familiar with working online, and to have sufficient time to fill in and submit the online questionnaire. Ultimately, we obtained data from 3,600 respondents (mean age = 49.73 ± 16.75 years). In addition to these 3,600 respondents, 481 participants were previously excluded due to inconsistencies between registered and reported demographic information or identical responses to all questions. We also excluded 715 satisfiers (i.e., people who presumably responded to the questions simply to meet the minimum requirements to finish the session, or people who responded carelessly) whose response time was <4 min (see the Supplemental material for further details regarding this criterion) resulting in a valid dataset of 2,885 individuals (1,524 women, mean age = 52.23 ± 16.52 years). No respondents had COVID-19.
2.2. Measures
The survey was developed in three sections (n = 104 in total), including five aspects of COVID-19-related items, consisting of coping behavior (n = 33), risk perception (n = 17), demographic questions (n = 10), and two psychobehavioral scales (n = 44).
2.2.1. Coping behavior and risk perception
We used 50 items to measure coping behavior and risk perception. These items were taken from previous studies or generated from interviews with people in our network (29, 30). We assessed five aspects of coping behavior and risk perception: self-infection, other-infection, daily shortages of necessities, social and economic impacts, and information access (see Supplemental material).
2.2.2. Demographic information
The demographic questions included 10 items (Table 1): sex, age, place of residence, family structure, have toddlers or not, have children or not, reported local cases of infection, the degree of risk of self-infection becoming severe because of chronic disease or age (two separate items), and the degree of risk of severe disease among their family members because of underlying disease or age.
2.2.3. Psychobehavioral characteristics
We used the 34-item Power to Live scale, which measures eight psychobehavioral characteristics: leadership, problem-solving, altruism, stubbornness, etiquette, emotional regulation, self-transcendence, and active well-being. Previous studies have demonstrated internal consistency and concurrent validity of the scale (31, 32). Participants provided responses using a six-point scale (0: Not at all; 5: Very much). We calculated the mean score for each characteristic.
We also assessed the Big Five personality traits using the Japanese version of the Ten-Item Personality Inventory (TIPI-J). The TIPI-J has good internal consistency and concurrent validity (33, 34). Participants provided responses using a six-point scale (0: Not at all; 5: Very much). Each of the five dimensions (extraversion, agreeableness, conscientiousness, neuroticism, and openness) included a positive and a reverse item. Dimension scores were calculated by subtracting the score for the reverse item from that for the positive item.
3. Analysis
All analyses were performed in R (35) using the tidyverse (36), psych (37), GPArotation (38), EFA.MRFA (39), parameters (40), and effectsize (41) packages.
3.1. Exploratory factor analysis
We performed a factor analysis by pooling all of the coping-behavior and risk-perception items. The aim of the factor analysis was to dissociate coping behavior and risk perception by eliminating items that may convey similar nuances of both. First, we confirmed the appropriateness of the data for exploratory factor analysis by performing the Kaiser–Meyer–Olkin (KMO) test and Bartlett’s test (42). The number of factors was determined based on the minimum average partial procedure (43), the Hull method (44), a parallel analysis (45), and a scree plot. We used the maximum likelihood method with Promax rotation because we assumed that the identified factors were correlated, and this method is well suited to simple structures (46). We excluded items if they met any of the following criteria: commonality <0.3, loading <0.4, or loading >0.4 on more than one factor (cross-loading). After removing an item, we repeated the analysis until all items met the criteria. The sum of squared (SS) loadings indicated the proportion of the variance explained by each factor. Cronbach’s α was calculated for each factor to estimate internal consistency. Factor scores were calculated by averaging the scores of all items for each factor.
3.2. Correlation analysis
We performed a correlation analysis to explore the relationships among the identified coping-behavior factors, risk-perception factors, and psychobehavioral characteristics. Given the large sample size, we used |r| > 0.3 as the effect size threshold (47, 48).
3.3. Hierarchical regression analysis
To further explore the factors contributing to coping behavior and risk perception, we performed hierarchical regression analysis, which provides significant tests for the effects of independent variables on the dependent variable while controlling for the influence of the other independent variables.
We performed hierarchical regression analyses for each of the four coping-behavior factors. With the factor score as the dependent variable, we used 13 background factors in the first block, 3 risk-perception factors in the second block, and 13 psychobehavioral characteristics in the third block as explanatory variables.
We applied similar hierarchical regression models for each of the three risk-perception factors. We entered the 13 background-factor variables in the first block and the 13 psychobehavioral characteristics in the second block as explanatory variables.
Hierarchical regression analysis was performed using the stepwise method, and the variables were selected based on Akaike’s information criterion. For each regression model, we calculated tolerance and the variance inflation factor to detect multicollinearity among predictors. Tolerance values <0.2 and variance inflation factor values >4 are considered problematic (49). Cohen’s f2 was used reflect the overall effect size of each block in the hierarchical regression (47). The term fB/A2 represents the effect size of each predictor (50). Due to the large sample size, we used a small effect size (i.e., Cohen’s f2 = 0.02) as the threshold.
4. Results
The demographic data are summarized in Table 1. The numbers and percentages of participants’ demographic information are given for each item.
4.1. Exploratory factor analysis
The results of the KMO and Bartlett’s tests indicated that the data were suitable for factor analysis (KMO = 0.92; χ2 (1225) = 75783.41, p < 0.001). The minimum average partial, Hull method, and scree plot suggested extraction of eight factors, while the parallel analysis suggested extraction of 11 factors. Therefore, we selected an eight-factor solution; however, the results had a factor containing only two items. Thus, we eliminated one factor and reached a seven-factor solution following the suggestion that a factor should include at least three items (51). Finally, we removed nine items based on the commonality criterion and three items based on the two loading criteria; we thus achieved satisfactory results for the seven-factor solution (Table 2).
Table 2 shows the results of the seven factors. There were four coping-behavior factors: two related to infection, CB1, mask-wearing (representing mask use related to infection prevention behavior); and CB4, infection-prevention (representing general infection prevention measures, such as hand washing); and two related to general adversity, CB2, information-seeking (searching for or checking COVID-19-related information) and CB3, resistance to social stagnation. The CB3 label was based on the fact that all described behaviors serve to prevent social stagnation. This stagnation may be caused by reduced communication, reduced economic activity, and psychological depression. Items 5 and 6 are also behaviors that counteract social disorders, albeit from different viewpoints. There were three risk-perception factors: one related to infection, RP2, medical concerns (indicated concerns about medical resources and becoming infected); and two related to general adversity, RP1, shortages of daily necessities (measured concerns about shortages of daily supplies) and RP3, socioeconomic concerns (represented concerns about society and the economy). The internal consistency coefficients (Cronbach’s α) of all factors were >0.70. They constituted 55% of the total variance.
4.2. Correlation analysis
Among the coping-behavior factors, information-seeking (CB2) was significantly associated with all other coping-behavior factors (CB1, CB3, and CB4) and socioeconomic concerns (RP3). Furthermore, mask-wearing (CB1) was associated with infection-prevention (CB4). Three risk-perception factors were significantly associated with each other; socioeconomic concerns (RP3) were significantly associated with mask-wearing (CB1) and information-seeking (CB2) (Table 3).
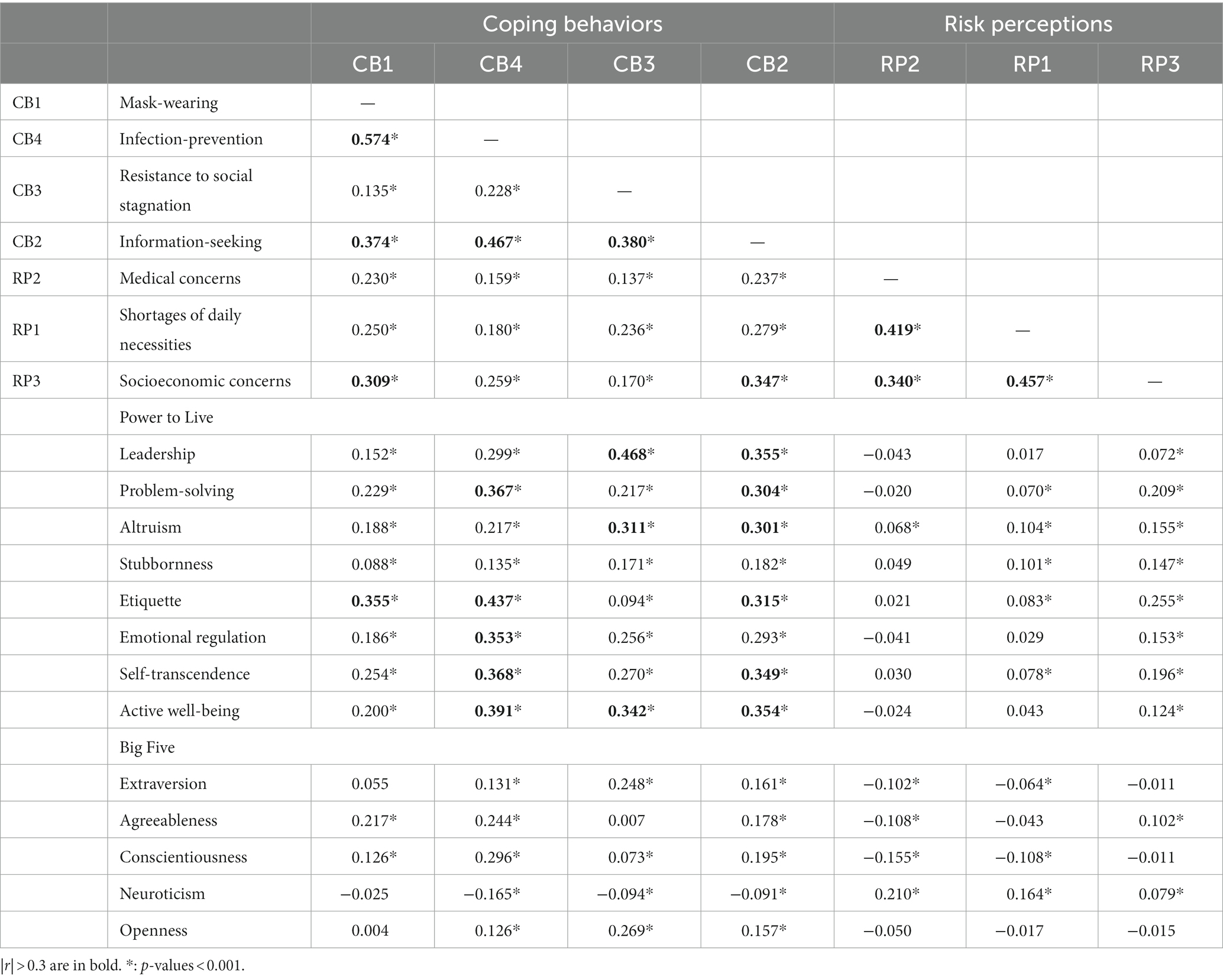
Table 3. Correlation matrix for coping-behavior factors, risk-perception factors, and psychobehavioral characteristics.
All four coping-behavior factors were significantly associated with at least one characteristic in the Power to Live scale, but the risk-perception factors did not demonstrate such associations. Both infection prevention factors (CB1 and CB4) were associated with etiquette; infection-prevention (CB4) was additionally associated with problem-solving, emotional regulation, self-transcendence, and active well-being. Both general-adversity coping behaviors (CB2 and CB3) were associated with leadership, altruism, and active well-being; information-seeking (CB2) was additionally associated with problem-solving, etiquette, and self-transcendence. However, no significant associations were observed between factors and Big Five characteristics (Table 3).
4.3. Hierarchical regression analysis
Tolerance and variance inflation factor analyses indicated no evidence of multicollinearity in any hierarchical regression.
Table 4 summarizes the results of the four coping-behavior factors (see Supplementary Tables S1–S4 online). Among the demographic factors, sex negatively contributed to two infection prevention factors (CB1 and CB4). Among risk-perception factors, shortages of daily necessities (RP1) significantly contributed to two general-adversity coping behaviors (CB2 and CB3), while socioeconomic concerns (RP3) positively contributed to information-seeking (CB2). However, medical concerns (RP2) did not contribute to any of the coping behaviors. Among the psychobehavioral characteristics, etiquette was positively associated with two infection prevention factors (CB1 and CB4), while it was negatively associated with behaviors protecting against social stagnation (CB3). Leadership and active well-being were positively associated with resistance to social stagnation (CB3) and infection-prevention (CB4), respectively.
Table 5 displays a summary of the results for the risk-perception factors (see Supplementary Tables S5–S7 online). Among the demographic factors, age, high-risk age, and having a high-risk family member significantly contributed to medical concerns (RP2), but age demonstrated a negative association. Among psychobehavioral characteristics, etiquette contributed only to socioeconomic concerns (RP3). Table 6 shows the relationships of demographic information, risk-perception factors, and psychobehavioral characteristics with coping-behavior factors.
5. Discussion
The main goal of this study was to identify major coping-behavior factors while exploring the contributions of demographic information, risk-perception factors, and psychobehavioral characteristics to coping-behavior factors. We identified four coping-behavior factors (two related to infection and two related to general adversity) and three risk-perception factors (one related to infection and two related to general adversity). Female sex and etiquette promoted infection prevention behaviors (CB1 and CB4), whereas shortages of daily necessities (RP1) promoted general-adversity coping behaviors (CB2 and CB3). Active well-being promoted infection-prevention (CB4), and socioeconomic concerns (RP3) promoted information-seeking (CB2). Resistance to social stagnation (CB3) was inhibited by etiquette and promoted by leadership. Although some of the correlations between the Big Five scale and coping-behavior factors were consistent with previous studies (15, 21), none of them reached our effect-size threshold (Table 6).
The factors promoting infection prevention behaviors in this study were consistent with existing knowledge. Our findings showed that etiquette and female sex contributed to two infection prevention factors, while medical concerns (RP2) did not. Etiquette was defined as adherence to social norms (32), and infection prevention behaviors may arise from the desire to comply with social norms. Our finding that medical concerns (RP2) lacked an association with infection prevention behaviors while etiquette was associated with infection prevention behaviors was consistent with a previous Japanese study, in which mask-wearing was related to social norms rather than the perceived risk of COVID-19 (52). In addition, our finding that women were more inclined to exhibit infection prevention behaviors was consistent with studies in which women were more willing to self-isolate (53) and more frequently engaged in positive coping behavior than men (54).
There are two potential reasons for the identification of two infection prevention factors in this study (i.e., mask-related and mask-unrelated). The first involves the executability of the two types of infection prevention. The availability of masks may have caused a separation of infection prevention due to the severe shortage and hoarding of masks that occurred during the early stage of the pandemic (55). The second reason involves the emphasis on wearing masks. The Ministry of Health, Labor, and Welfare released 61 documents concerning the latest domestic coronavirus situation in 77 days [1/1/2020–3/18/2020 (immediately before the survey)]; each of the documents included the same message to the public that wearing masks and washing hands are important practices (56). Most indoor and public places required a mask before entering. Thus, people could be divided into two groups: a group that wore masks and followed the mask-wearing recommendation and a group that lacked masks and focused more on general infection prevention.
Shortage of daily necessities (RP1) is a common facilitative factor of general-adversity coping behaviors. Media-dependency theory claims that people become increasingly dependent on social media during severe social disruption (57). Consistent with this theory, our results reveal that individuals tended to use information-seeking strategies (CB2) to be informed, prepared, and responsive to COVID-19 when they knew about shortages of daily necessities. The contribution of shortages of daily necessities (RP1) to resistance to social stagnation (CB3) is congruent with the results of the disaster research described in the Introduction. Shortage of daily necessities, while not totally representing social stagnation, might be seen as an early warning sign of subsequent social stagnation in multiple fields, such as long-lasting impacts on the supply chain (58). Thus, when our participants perceived an existing threat to society (i.e., shortage of daily necessities), they may have responded as if social stagnation was imminent.
However, each of the two general-adversity coping-behavior factors had unique features. Except for shortages of daily necessities (RP1), information-seeking (CB2) was facilitated by socioeconomic concerns (RP3); resistance to social stagnation (CB3) was inhibited by etiquette and enhanced by leadership. The relationship between information-seeking (CB2) and socioeconomic concerns (RP3) is also consistent with the media-dependency theory: perceived social risk enhances information-seeking. The negative contribution of etiquette to resistance to social stagnation (CB3) may have originated from obedience to guidance. For instance, people with high etiquette scores are more likely to maintain social distance and limit their engagement in non-essential activities. On the other hand, people with strong etiquette skills might attempt to maintain their usual routines as before. They may not implement strategies to resist social stagnation because fussiness also violates social norms (59). The essence of leadership refers to the tendency to solve problems through communication, which may explain directly its contribution to responses to imminent social stagnation (CB3). An example item of leadership in the Power to Live scale is: “To resolve problems, I gather everyone involved together to discuss the matter.” People with strong leadership are more likely to take the initiative to reach out to others and solve problems. Previous studies have reported a contribution of leadership to spontaneous evacuation efforts in the context of an imminent tsunami (12), including encouraging other people to evacuate (27) and resolving problems through mutual aid (28).
Our study implies that each coping behavior has distinct facilitatory/inhibitory psychological processes supported by a partial conflict between survival-oriented characteristics. Etiquette facilitated two infection prevention factors (CB1 and CB4) but inhibited the general-adversity coping-behavior factor (i.e., CB3; resistance to social stagnation). This conflict may be the result of a trade-off between infection prevention and general-adversity coping behaviors. For example, maintaining social distance (or self-isolation) is an effective and critical method to stop transmission. However, people may not be able to attend important events or socialize, which makes them feel socially isolated and impairs both physical and mental health.
In summary, our results provide a theoretical framework for sorting out the apparently chaotic social responses to the pandemic into a comprehensive picture by identifying its major factors and investigating the psychobehavioral mechanism underlying each factor. Previous studies have addressed only the psychological factors involved in social responses to the COVID-19 pandemic (4, 5, 15, 21, 52, 60). Our findings imply that coping behaviors can be classified into two categories: infection prevention (CB1: mask-wearing and CB4: infection-prevention) and coping with general adversity (CB2: information-seeking and CB3: resistance to social stagnation). The former behaviors were associated with female sex and etiquette, and the latter behaviors were associated with concerns regarding shortages of daily necessities (RP1). Additionally, infection-prevention (CB4) was facilitated by active well-being. Information-seeking (CB2) was promoted by socioeconomic concern (RP3) and resistance to social stagnation (CB3) was facilitated by leadership and suppressed by etiquette. The opposite associations of etiquette between two infection prevention factors (CB1 and CB4) and resistance to social stagnation (CB3) may underlie trade-offs between these two types of coping behaviors; this perspective may become evident only in this comprehensive framework.
Our framework provides policymakers with a comprehensive picture of a public with different characteristics, the associated coping behaviors exhibited, and their contexts. This may help them to implement policies that maximize social benefits. For instance, to prevent overresponses to adversity, national and local governments can take steps to reduce concerns about shortages of daily necessities, such as by ensuring adequate supplies. It is important to note that increased concern can have unexpected consequences: increased concern about medical issues is unlikely to facilitate infection prevention behaviors, but increased socioeconomic concern may facilitate people’s information seeking and lead to an “infodemic” (61). To optimize the balance between infection prevention and resistance to social stagnation, governments should take age- and culture-specific psychobehavioral characteristics (i.e., leadership, etiquette, and active well-being) into account, or consider educational and intervention approaches to affect such psychobehavioral characteristics. Based on the current theoretical framework, the development of such a set of strategic social approaches to pandemics appears promising.
Our study had several limitations. First, our work may be preliminary with regard to building a comprehensive model; a truly comprehensive model would integrate results for multiple periods characterized by different social responses. It is necessary to consider survey results from other periods. However, we do not consider the current data to be less valuable than such results. Our data reflected the social situation in the early days of the pandemic when the features of COVID-19 were largely unknown, and people’s fears were at their highest. Social turmoil caused by the shortage of masks and toilet paper, for example, was also unique to this period. Second, the comprehensiveness of our results pertains only to individuals without COVID-19 infection, as none of our participants had COVID-19. Third, the sample is limited in its representativeness. Despite efforts to recruit participants from different generations from all over Japan, we acknowledge that the sample may not fully represent the larger population of interest. Finally, this study used self-report measures and included only Japanese participants, which may limit the generalizability of the findings. Moreover, there may have been response biases, such as population and optimism biases, where the participants were all familiar with online surveys and may have overestimated their knowledge of infectious diseases or underestimated the risk of disease. Such biases could have affected the relationships among the variables. Future studies could conduct experiments in other cultures and use other data-collection approaches to enhance the robustness and generalizability of the findings.
6. Conclusion
We proposed a new model comprising four independent coping-behavior factors and three risk-perception factors for COVID-19, which were categorized into infection-related and general adversity-related groups. We have demonstrated that infection prevention and coping with general adversity were associated with different factors. Female sex and etiquette promoted two infection prevention factors, while shortages of daily necessities promoted two general-adversity coping behavior factors. In addition, infection-prevention (CB4) was promoted by active well-being, and information-seeking (CB2) was promoted by socioeconomic concerns; meanwhile, resistance to social stagnation (CB3) was inhibited by etiquette and promoted by leadership. This study provides a theoretical framework for coping behaviors and risk perception during a pandemic and demonstrates their underlying psychobehavioral mechanisms. The contribution of demographic, risk-perception, and psychobehavioral characteristics to coping behavior could help policymakers devise effective strategies for optimizing social responses to pandemics. Future research should continue to refine this model of perceived risk and coping behavior, and including more data from different periods of the epidemic would greatly improve the model. Moreover, future studies can use this model to investigate how mental health and emotional distress affect different types of coping behavior.
Data availability statement
The datasets presented in this study can be found in online repositories. The names of the repository/repositories and accession number(s) can be found at: https://osf.io/t4suw/.
Ethics statement
The studies involving humans were approved by Ethical Committee of the International Research Institute of Disaster Science, Tohoku University (2019-035). The studies were conducted in accordance with the local legislation and institutional requirements. The participants provided their written informed consent to participate in this study.
Author contributions
YD and MS designed the experiment. YD analyzed the data and wrote the initial draft of the manuscript. All authors contributed to the article and approved the submitted version.
Funding
This study was supported by the Subsidy for Interdisciplinary Study and Research Concerning COVID-19 from the Mitsubishi Foundation, KAKENHI (22H04855 and 22KJ0310) from the Japan Society for the Promotion of Science, and JST SPRING (JPMJSP2114).
Acknowledgments
This study was supported by the “Subsidy for Interdisciplinary Study and Research concerning COVID-19” from the Mitsubishi Foundation.
Conflict of interest
The authors declare that the research was conducted in the absence of any commercial or financial relationships that could be construed as a potential conflict of interest.
Publisher’s note
All claims expressed in this article are solely those of the authors and do not necessarily represent those of their affiliated organizations, or those of the publisher, the editors and the reviewers. Any product that may be evaluated in this article, or claim that may be made by its manufacturer, is not guaranteed or endorsed by the publisher.
Supplementary material
The Supplementary material for this article can be found online at: https://www.frontiersin.org/articles/10.3389/fpsyt.2023.1200473/full#supplementary-material
References
1. Anderson, RM, Heesterbeek, H, Klinkenberg, D, and Hollingsworth, TD. How will country-based mitigation measures influence the course of the COVID-19 epidemic? Lancet. (2020) 395:931–4. doi: 10.1016/S0140-6736(20)30567-5
2. Alcántara-Ayala, I, Burton, I, Lavell, A, Mansilla, E, Maskrey, A, Oliver-Smith, A, et al. Editorial: root causes and policy dilemmas of the COVID-19 pandemic global disaster. Int J Disaster Risk Reduct. (2021) 52:101892. doi: 10.1016/j.ijdrr.2020.101892
3. Nomura, S, Kawashima, T, Yoneoka, D, Tanoue, Y, Eguchi, A, Gilmour, S, et al. Trends in suicide in Japan by gender during the COVID-19 pandemic, up to September 2020. Psychiatry Res. (2021) 295:113622. doi: 10.1016/j.psychres.2020.113622
4. Bacon, AM, and Corr, PJ. Coronavirus (COVID-19) in the United Kingdom: a personality-based perspective on concerns and intention to self-isolate. Br J Health Psychol. (2020) 25:839–48. doi: 10.1111/bjhp.12423
5. Capone, V, Caso, D, Donizzetti, AR, and Procentese, F. University student mental well-being during COVID-19 outbreak: what are the relationships between information seeking, perceived risk and personal resources related to the academic context? Sustainability. (2020) 12:7039. doi: 10.3390/su12177039
6. Kuang, J, Ashraf, S, Das, U, and Bicchieri, C. Awareness, risk perception, and stress during the COVID-19 pandemic in communities of Tamil Nadu, India. Int J Environ Res Public Health. (2020) 17:E7177. doi: 10.3390/ijerph17197177
7. Hechler, S, Wendel, C, and Schneider, D. Dealing with the COVID-19 pandemic: how defense strategies relate to empathic reactions during lockdowns. Soc Psychol. (2023) 54:66–77. doi: 10.1027/1864-9335/a000501
8. Dash, N, and Gladwin, H. Evacuation decision making and behavioral responses: individual and household. Nat Hazards Rev. (2007) 8:69–77. doi: 10.1061/(asce)1527-6988(2007)8:3(69)
9. Dooley, D, Catalano, R, Mishra, S, and Serxner, S. Earthquake preparedness: predictors in a community survey. J Appl Soc Psychol. (1992) 22:451–70. doi: 10.1111/j.1559-1816.1992.tb00984.x
10. Lindell, MK, and Perry, RW. The protective action decision model: theoretical modifications and additional evidence. Risk Anal. (2012) 32:616–32. doi: 10.1111/j.1539-6924.2011.01647.x
11. Miceli, R, Sotgiu, I, and Settanni, M. Disaster preparedness and perception of flood risk: a study in an alpine valley in Italy. J Environ Psychol. (2008) 28:164–73. doi: 10.1016/j.jenvp.2007.10.006
12. Sugiura, M, Sato, S, Nouchi, R, Honda, A, Ishibashi, R, Abe, T, et al. Psychological processes and personality factors for an appropriate tsunami evacuation. Geosci Switz. (2019) 9:1–19. doi: 10.3390/geosciences9080326
13. Weinstein, ND, Lyon, JE, Rothman, AJ, and Cuite, CL. Preoccupation and affect as predictors of protective action following natural disaster. Br J Health Psychol. (2000) 5:351–63. doi: 10.1348/135910700168973
14. Lahey, BB. Public health significance of neuroticism. Am Psychol. (2009) 64:241–56. doi: 10.1037/a0015309
15. Aschwanden, D, Strickhouser, JE, Sesker, AA, Lee, JH, Luchetti, M, Stephan, Y, et al. Psychological and behavioural responses to coronavirus disease 2019: the role of personality. Eur J Personal. (2021) 35:51–66. doi: 10.1002/per.2281
16. Bogg, T, and Milad, E. Demographic, personality, and social cognition correlates of coronavirus guideline adherence in a U.S. sample. Health Psychol. (2020) 39:1026–36. doi: 10.1037/hea0000891
17. Carvalho, L d F, Pianowski, G, and Gonçalves, AP. Personality differences and COVID-19: are extroversion and conscientiousness personality traits associated with engagement with containment measures? Trends Psychiatry Psychother. (2020) 42:179–84. doi: 10.1590/2237-6089-2020-0029
18. Liu, S, Lithopoulos, A, Zhang, C-Q, Garcia-Barrera, MA, and Rhodes, RE. Personality and perceived stress during COVID-19 pandemic: testing the mediating role of perceived threat and efficacy. Personal Individ Differ. (2021) 168:110351–1. doi: 10.1016/j.paid.2020.110351
19. Mazza, C, Ricci, E, Marchetti, D, Fontanesi, L, Di Giandomenico, S, Verrocchio, MC, et al. How personality relates to distress in parents during the Covid-19 lockdown: the mediating role of child’s emotional and behavioral difficulties and the moderating effect of living with other people. Int J Environ Res Public Health. (2020) 17:6236. doi: 10.3390/ijerph17176236
20. Prentice, C, Zeidan, S, and Wang, X. Personality, trait EI and coping with COVID 19 measures. Int J Disaster Risk Reduct. (2020) 51:101789. doi: 10.1016/j.ijdrr.2020.101789
21. Qian, K, and Yahara, T. Mentality and behavior in COVID-19 emergency status in Japan: influence of personality, morality and ideology. PLoS One. (2020) 15:e0235883–16. doi: 10.1371/journal.pone.0235883
22. Zajenkowski, M, Jonason, PK, Leniarska, M, and Kozakiewicz, Z. Who complies with the restrictions to reduce the spread of COVID-19?: Personality and perceptions of the COVID-19 situation. Personal Individ Differ. (2020) 166:110199. doi: 10.1016/j.paid.2020.110199
23. MK, AL-O, Alzoubi, IA, Al Nazeh, AA, Alomiri, AK, Maswady, MN, and Lynch, E. COVID-19 and personality: a cross-sectional multicenter study of the relationship between personality factors and COVID-19-related impacts, concerns, and behaviors. Front Psych. (2021) 12:12. doi: 10.3389/fpsyt.2021.608730
24. Liu, T-H, Xia, Y, and Ma, Z. Multifarious linkages between personality traits and psychological distress during and after COVID-19 campus lockdown: a psychological network analysis. Front Psych. (2022) 13:816298. doi: 10.3389/fpsyt.2022.816298
25. Guo, J, Feng, XL, Wang, XH, and van IJzendoorn, MH. Coping with COVID-19: exposure to COVID-19 and negative impact on livelihood predict elevated mental health problems in Chinese adults. Int J Environ Res Public Health. (2020) 17:E3857. doi: 10.3390/ijerph17113857
26. Abdelrahman, M. Personality traits, risk perception, and protective behaviors of Arab residents of Qatar during the COVID-19 pandemic. Int J Ment Health Addict. (2020) 20:237–48. doi: 10.1007/s11469-020-00352-7
27. Sugiura, M, Nouchi, R, Honda, A, Sato, S, Abe, T, and Imamura, F. Survival-oriented personality factors are associated with various types of social support in an emergency disaster situation. PLoS One. (2020) 15:e0228875–5. doi: 10.1371/journal.pone.0228875
28. Sugiura, M, Ishibashi, R, Abe, T, Nouchi, R, Honda, A, Sato, S, et al. Self-help and mutual assistance in the aftermath of a tsunami: how individual factors contribute to resolving difficulties. PLoS One. (2021) 16:e0258325. doi: 10.1371/journal.pone.0258325
29. Duncan, LA, Schaller, M, and Park, JH. Perceived vulnerability to disease: development and validation of a 15-item self-report instrument. Personal Individ Differ. (2009) 47:541–6. doi: 10.1016/j.paid.2009.05.001
30. Wang, IM, Michalak, NM, and Ackerman, JM. Threat of infectious disease, The SAGE handbook of personality and individual differences: Origins of personality and individual differences. SAGE Publications Ltd (2018). 321–345
31. Ishibashi, R, Nouchi, R, Honda, A, Abe, T, and Sugiura, M. A concise psychometric tool to measure personal characteristics for surviving natural disasters: development of a 16-item power to live questionnaire. Geosciences. (2019) 9:366. doi: 10.3390/geosciences9090366
32. Sugiura, M, Sato, S, Nouchi, R, Honda, A, Abe, T, Muramoto, T, et al. Eight personal characteristics associated with the power to live with disasters as indicated by survivors of the 2011 great East Japan earthquake disaster. PLoS One. (2015) 10:1–14. doi: 10.1371/journal.pone.0130349
33. Gosling, SD, Rentfrow, PJ, and Swann, WB. A very brief measure of the big-five personality domains. J Res Personal. (2003) 37:504–28. doi: 10.1016/S0092-6566(03)00046-1
34. Oshio, A, Abe, S, and Cutrone, P. Development, reliability, and validity of the Japanese version of ten item personality inventory (TIPI-J). Jpn J Personal. (2012) 21:40–52. doi: 10.2132/personality.21.40
36. Wickham, H, Averick, M, Bryan, J, Chang, W, McGowan, LD, François, R, et al. Welcome to the {tidyverse}. J Open Source Softw. (2019) 4:1686–6. doi: 10.21105/joss.01686
38. Bernaards, CA, and Jennrich, IR. Gradient projection algorithms and software for arbitrary rotation criteria in factor analysis. Educ Psychol Meas. (2005) 65:676–96. doi: 10.1177/0013164404272507
39. Navarro-Gonzalez, D, and Lorenzo-Seva, U. EFA.MRFA: dimensionality assessment using minimum rank factor analysis. (2021). Available at: https://CRAN.R-project.org/package=EFA.MRFA
40. Lüdecke, D, Ben-Shachar, MS, Patil, I, and Makowski, D. Extracting, computing and exploring the parameters of statistical models using R. J Open Source Softw. (2020) 5:2445. doi: 10.21105/joss.02445
41. Ben-Shachar, MS, Lüdecke, D, and Makowski, D. Effectsize: estimation of effect size indices and standardized parameters. J Open Source Softw. (2020) 5:2815. doi: 10.21105/joss.02815
42. Beavers, A, Lounsbury, J, Richards, J, Huck, S, Skolits, G, and Esquivel, S. Practical considerations for using exploratory factor analysis in educational research. Pract Assess Res Eval. (2013) 18. doi: 10.7275/qv2q-rk76
43. Velicer, WF. Determining the number of components from the matrix of partial correlations. Psychometrika. (1976) 41:321–7. doi: 10.1007/BF02293557
44. Lorenzo-Seva, U, Timmerman, ME, and Kiers, HAL. The Hull method for selecting the number of common factors. Multivar Behav Res. (2011) 46:340–64. doi: 10.1080/00273171.2011.564527
45. Horn, JL. A rationale and test for the number of factors in factor analysis. Psychometrika. (1965) 30:179–85. doi: 10.1007/BF02289447
46. Finch, H. Comparison of the performance of varimax and promax rotations: factor structure recovery for dichotomous items. J Educ Meas. (2006) 43:39–52. doi: 10.1111/j.1745-3984.2006.00003.x
48. Fiske, ST, Gilbert, DT, and Lindzey, G In: ST Fiske, DT Gilbert, and G Lindzey, editors. Handbook of social psychology. 5th ed. Hoboken, NJ: Wiley (2010). 1–578.
49. Hair, JF, Black, WC, Babin, BJ, and Anderson, RE. Multivariate data analysis: a global perspective. 7th ed. New Jersey: Pearson Education Inc. (2010).
50. Selya, A, Rose, J, Dierker, L, Hedeker, D, and Mermelstein, R. A practical guide to calculating Cohen’s f2, a measure of local effect size, from PROC MIXED. Front Psychol. (2012) 3:111. doi: 10.3389/fpsyg.2012.00111
51. Costello, AB, and Osborne, JW. Best practices in exploratory factor analysis: four recommendations for getting the most from your analysis. Pract Assess Res Eval. (2005). 10. doi: 10.7275/jyj1-4868
52. Nakayachi, K, Ozaki, T, Shibata, Y, and Yokoi, R. Why do Japanese people use masks against COVID-19, even though masks are unlikely to offer protection from infection? Front Psychol. (2020) 11:1–5. doi: 10.3389/fpsyg.2020.01918
53. Atchison, CJ, Bowman, L, Vrinten, C, Redd, R, Pristerà, P, Eaton, JW, et al. Perceptions and behavioural responses of the general public during the COVID-19 pandemic: a cross-sectional survey of UK adults. medRxiv. (2020). doi: 10.1101/2020.04.01.20050039
54. Ulloa, RE, Apiquian, R, de la Peña, FR, Díaz, R, Mayer, P, Palacio, JD, et al. Age and sex differences in the impact of the COVID-19 pandemic on mental health and coping mechanisms in Latin American youth. J Psychiatr Res. (2022) 156:372–8. doi: 10.1016/j.jpsychires.2022.10.005
55. Noich, H. Surgical mask shortages as coronavirus fears grow. NHK WORLD (2020), Available at: https://www3.nhk.or.jp/nhkworld/en/news/backstories/865/ (Accessed October 1, 2021)
56. Ministry of Health, Labour and Welfare. Press Release. Curr Situat new coronavirus infect response Minist health labour Welf, Available at: https://www.mhlw.go.jp/stf/houdou/index.html (Accessed March 29, 2022)
57. Lowrey, W. Media dependency during a large-scale social disruption: the case of September 11. Mass Commun Soc. (2004) 7:339–57. doi: 10.1207/s15327825mcs0703_5
58. Paul, SK, and Chowdhury, P. Strategies for managing the impacts of disruptions during COVID-19: an example of toilet paper. Glob J Flex Syst Manag. (2020) 21:283–93. doi: 10.1007/s40171-020-00248-4
59. Scholderer, J, and Veflen, N. Social norms and risk communication. Trends Food Sci Technol. (2019) 84:62–3. doi: 10.1016/j.tifs.2018.08.002
60. Li, D-J, Ko, N-Y, Chang, Y-P, Yen, C-F, and Chen, Y-L. Mediating effects of risk perception on association between social support and coping with COVID-19: an online survey. Int J Environ Res Public Health. (2021) 18:1550. doi: 10.3390/ijerph18041550
Keywords: COVID-19, coping behavior, risk perception, psychobehavioral characteristic, model
Citation: Ding Y, Ishibashi R, Abe T, Honda A and Sugiura M (2023) A multifactorial framework of psychobehavioral determinants of coping behaviors: an online survey at the early stage of the COVID-19 pandemic. Front. Psychiatry. 14:1200473. doi: 10.3389/fpsyt.2023.1200473
Edited by:
Wenjie Duan, East China University of Science and Technology, ChinaReviewed by:
Gaurav Dhiman, Government Bikram College of Commerce Patiala (Punjab), IndiaVince Hooper, Prince Mohammad bin Fahd University, Saudi Arabia
Inmaculada Méndez, University of Murcia, Spain
Copyright © 2023 Ding, Ishibashi, Abe, Honda and Sugiura. This is an open-access article distributed under the terms of the Creative Commons Attribution License (CC BY). The use, distribution or reproduction in other forums is permitted, provided the original author(s) and the copyright owner(s) are credited and that the original publication in this journal is cited, in accordance with accepted academic practice. No use, distribution or reproduction is permitted which does not comply with these terms.
*Correspondence: Yi Ding, ZGluZ3lpOTUwOEBvdXRsb29rLmNvbQ==