- 1Geisel School of Medicine at Dartmouth, Hanover, NH, United States
- 2Department of Psychiatry, Dartmouth Health, Lebanon, NH, United States
- 3Icahn School of Medicine at Mount Sinai, New York, NY, United States
- 4Northwestern University Feinberg School of Medicine, Chicago, IL, United States
Background: While online reviews from physician rating websites are increasingly utilized by healthcare providers to better understand patient needs, it remains difficult to objectively identify areas for improvement in providing psychiatric care.
Objectives: To quantitatively characterize the sentiment of online written reviews of psychiatrists to determine clinical attributes that can be strengthened to improve psychiatrists’ therapeutic alliance with their patients.
Materials and methods: Sentiment scores of 6,400 written reviews of 400 US-based psychiatrists on a US-based online physician rating website were obtained through a natural-language-processing-based sentiment analysis. Relationships among sentiment scores, average star ratings, and demographics were examined. Linguistic analyses determined words and bigrams that were highly associated with reviews with the most positive and negative sentiment.
Findings: Sentiment scores were significantly correlated with average star ratings of the psychiatrists (R = 0.737, p < 0.001). Psychiatrists who were younger (< 56 years old) and/or practiced in the Northeast had significantly higher average star ratings than those older and/or practicing in the Southwest. Frequency analysis showed that positive reviews most frequently contained “time” (N = 1,138) and “caring” (N = 784) while negative reviews most frequently contained “medication” (N = 495) and “time” (N = 379). Logistic regression analysis revealed that reviews were more likely to be considered positive when they included “great listener” (OR = 16.89) and “comfortable” (OR = 10.72) and more likely to be negative when they included “meds” (OR = 0.55) and “side effect” (OR = 0.59).
Conclusion: Psychiatrists who are younger and located in the Northeast receive more positive reviews; there may be potential for demographic bias among patient reviewers. Patients positively rate psychiatrists who make them feel heard and comfortable but negatively rate encounters centered around medications and their side effects. Our study lends quantitative evidence to support the importance of thorough and empathetic communication of psychiatrists in building a strong therapeutic alliance.
1. Introduction
The therapeutic alliance, a patient’s interpersonal relationship with the patient’s psychiatrist, is a critical component of psychiatric care that significantly impacts outcomes, yet it remains difficult to quantitatively characterize and identify areas for improvement (1–3). Physician rating websites (PRWs) summarize patients’ complex experiences in the form of reviews and ratings and may serve as a window into the strength of the therapeutic alliance psychiatrists form with their patients (4–6). Although PRWs have been quantitatively analyzed in other medical specialties to describe physicians’ characteristics that affect care (7–9), no studies to date have leveraged PRWs to determine clinical attributes of psychiatrists that may affect their therapeutic alliance with their patients.
Sentiment analysis is a machine learning-based technique for rating positive or negative use of language in text that recently led to an increased understanding of clinical contributors to patient satisfaction in surgical fields (10, 11). In the field of psychiatry, previous studies have demonstrated the validity of sentiment analysis among antidepressant users and for online mental health consultation services (12, 13). Specifically, while the general attitude toward online mental health consultation services and the usefulness of online reviews in China have previously been examined, no studies to date have elucidated impactful attributes of psychiatrists that can improve patient experiences during their mental health visits in the United States using PRWs (13). To that end, the present study aimed to utilize sentiment analysis on online written reviews of psychiatrists from PRWs to identify demographic and clinical characteristics strongly associated with reviews with the most positive and negative sentiment.
2. Materials and methods
2.1. Data acquisition
Publicly available star-rating reviews (ratings out of five stars) as well as online written reviews of psychiatrists were gathered from healthgrades.com. This website was selected as it was one of the top websites suggested by an online search engine and without a firewall restricting automated scraping techniques used to collect reviews and demographic data (age, gender, geographic region) pertaining to the psychiatrists. Scraping was accomplished by parsing the HTML code of Healthgrades physician pages. Psychiatrists with fewer than seven reviews (10) were excluded to accurately calculate average star ratings and sentiment scores, as this was determined to be the optimal threshold for a strong association between sentiment scores and star ratings.
2.2. Sentiment analysis and VADER score calculation
In order to quantify the sentiment of each online written review, the Valence Aware Dictionary and sEntiment Reasoner (VADER) sentiment analysis package was employed in Python 3.7. The rule-based VADER package is part of a larger natural language processing library, the Natural Language Toolkit (NLTK) python library. For its comprehensive ability to analyze the sentiment of sentences, VADER has seen increased application in healthcare, particularly in analysis of social media data and reviews of surgeons (10, 14).
In short, the VADER package is able to take in written prose and assign a sentiment score of the sentence. The package performs its calculation mainly by utilizing a word lexicon developed by ten independent human raters, who scored 80% or higher on a standardized college-level reading comprehension test and were trained for online sentiment rating to ensure inter-rater consistency (15). For each word in the dictionary, the human raters attributed scores ranging from −4 (“Extremely Negative”) to +4 (“Extremely Positive”), with 0 reflecting a neutral sentiment. By scanning the inputted sentences for words in its lexicon, VADER generates a summed and normalized score between −1 and +1. In addition, it is able to consider the effects of emphasizing features of a sentence like capitalization and punctuation, as well as the impact of negators (i.e., “not”) and modifiers (i.e., “extremely”) on the sentiment of the lexicon-drawn words. For example, “extremely compassionate” would impact the overall sentiment more positively, and “not compassionate” would contribute more negatively.
2.3. Model validation
We performed a linear regression analysis to confirm the relationship between the average sentiment analysis score for each provider with their average star score, which supported the use of our inclusion and exclusion criteria for subsequent analyses.
2.4. Data analysis
In order to examine how different demographic features may impact average star ratings and sentiment scores of written reviews, we performed Student’s t-tests for age and sex analyses. The ages of the psychiatrists were used to divide them into cohorts of individuals above and below 56 years old, as 56 years split the distribution of psychiatrists the most evenly. Because some psychiatrists did not have their age listed on Healthgrades, these psychiatrists were not included in the age analysis. Physicians were also grouped by region of practice into five categories: Northeast, Southeast, Southwest, Midwest, and West following definitions set by National Geographic.1 One-way ANOVA with subsequent pairwise Tukey tests were conducted to examine the relationship between location and physician ratings and sentiment scores.
Subsequently, a word frequency analysis was conducted to determine the most prevalent words and word pairs (bigrams) used to write the most positive and negative reviews. We independently assessed the best reviews that had a sentiment score of greater than 0.5 as well as the worst reviews with a negative overall sentiment score in order to determine the most common content in each. To further confirm how frequently found clinically relevant words/bigrams impact the overall sentiment of the review, we performed a multivariate logistic regression on these clinically relevant words and bigrams to examine the impact of their association with a positive or negative sentiment score.
3. Results
3.1. Psychiatrist demographics
The application of the inclusion criteria described previously resulted in the initial collection of 8,206 reviews distributed across 2,066 psychiatrists; after removing 1,666 psychiatrists through the exclusion criteria, 400 psychiatrists and 6,400 online reviews were ultimately included for analysis. All demographic information was also gathered from healthgrades.com. The providers’ age, sex identity, and location of practice were scraped along with the associated reviews from the HTML code and have been summarized in Table 1.
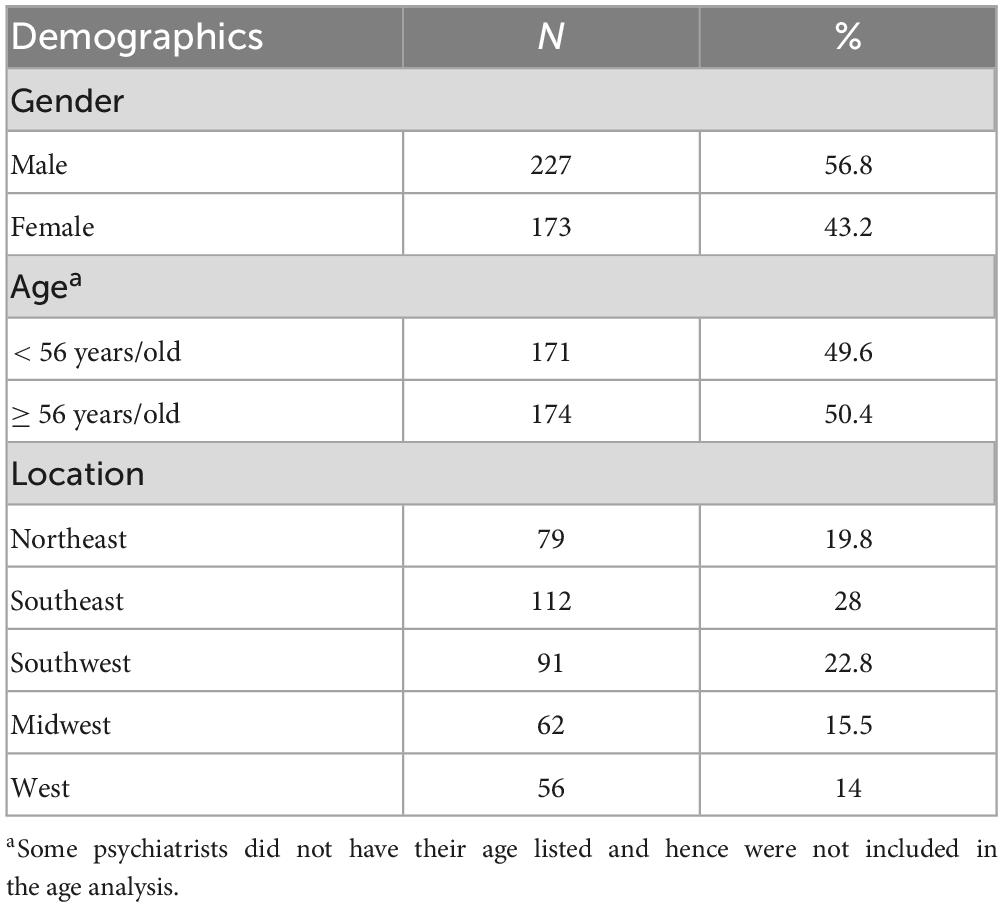
Table 1. Demographic characteristics of the analyzed 400 psychiatrists pulled from healthgrades.com.
3.2. Model validation: linear regression
The linear regression model examining the relationship between average star ratings and average sentiment analysis scores demonstrated a significant correlation (Figure 1, R = 0.737; p < 0.001). The statistically significant correlation validated the utility of sentiment analysis scores generated by VADER for linguistic analyses.
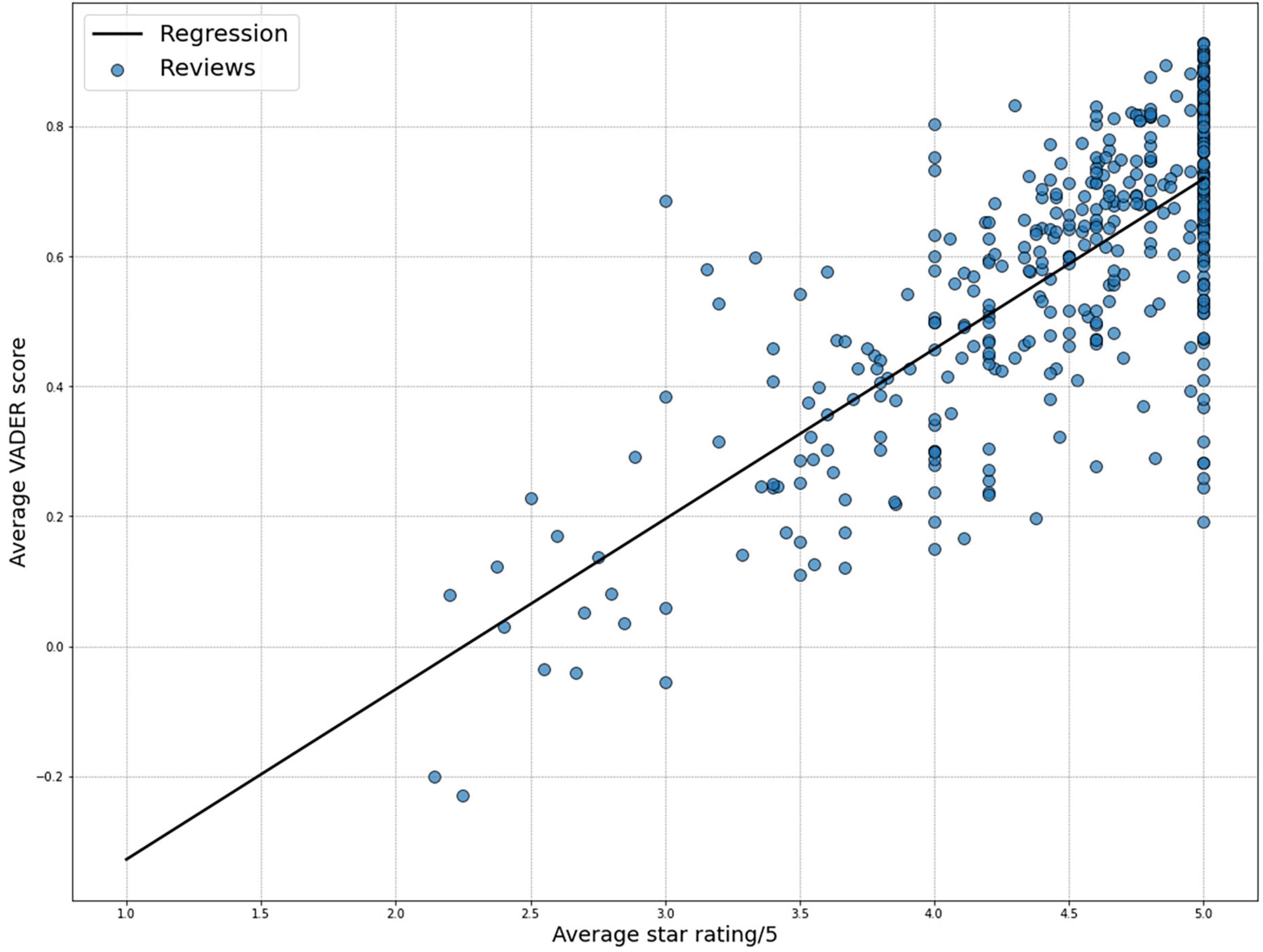
Figure 1. Significant correlation (R = 0.737, p < 0.001) between the psychiatrists’ average star ratings and the average sentiment scores generated using the Valence Aware Dictionary and sEntiment Reasoner (VADER) package.
3.3. Model validation and demographic analyses
There was a significant difference in star rating between older and younger psychiatrists (t = 2.060; p < 0.05), with younger psychiatrists having a larger average star rating (< 56: mean = 4.513) than older psychiatrists (≥ 56: mean = 4.376). No statistically significant difference in sentiment analysis score was found between the two age groups. Gender analysis revealed no statistically significant difference in average star ratings or sentiment analysis scores (p > 0.05) between male and female psychiatrists. Location analysis showed an overall significant difference in star rating (F = 2.479; p = 0.044), but not in sentiment analysis score. Subsequent Tukey’s pairwise comparisons revealed a significant difference in average star rating score between the psychiatrists in the Northeast (mean = 4.588) and the Southwest (mean = 4.320) regions of the United States (p-FWE = 0.035). The results of the analyses have been summarized in Table 2.
3.4. Linguistic analyses
Single-words that were most frequently used in the most positive and negative online written reviews were identified. Only words that were clinically relevant and meaningful were included. For example, words like “great” and “recommend” were frequently used but did not add to the clinical understanding of why the provider was “great,” and were hence omitted. The identified high-frequency words from both positive and negative reviews mainly focused on the length of the visit, the demeanor of providers and staff, and medication. The most positive reviews included “time,” “caring,” “care,” “staff,” “treatment,” “kind,” and “listens.” The most negative reviews included “time,” “depression,” “medication,” “staff,” “meds,” “treatment,” “care,” “medications,” and “anxiety.”
Frequency analysis also revealed the most frequently used clinically relevant bigrams from the most positive and negative reviews. The top reviews focused on the behavioral attributes of the providers: “takes, time,” “easy, talk,” “cares, patients,” “office, staff,” “truly, cares,” “feel, comfortable,” and “time, listen.” The worst reviews included bigrams relevant to medications and time during and prior to the visit: “depression, anxiety,” “side, effects,” “takes, time,” “waiting, room,” “front, desk,” “appointment, time,” and “staff, rude.” These terms and their frequencies have been summarized in Table 3.
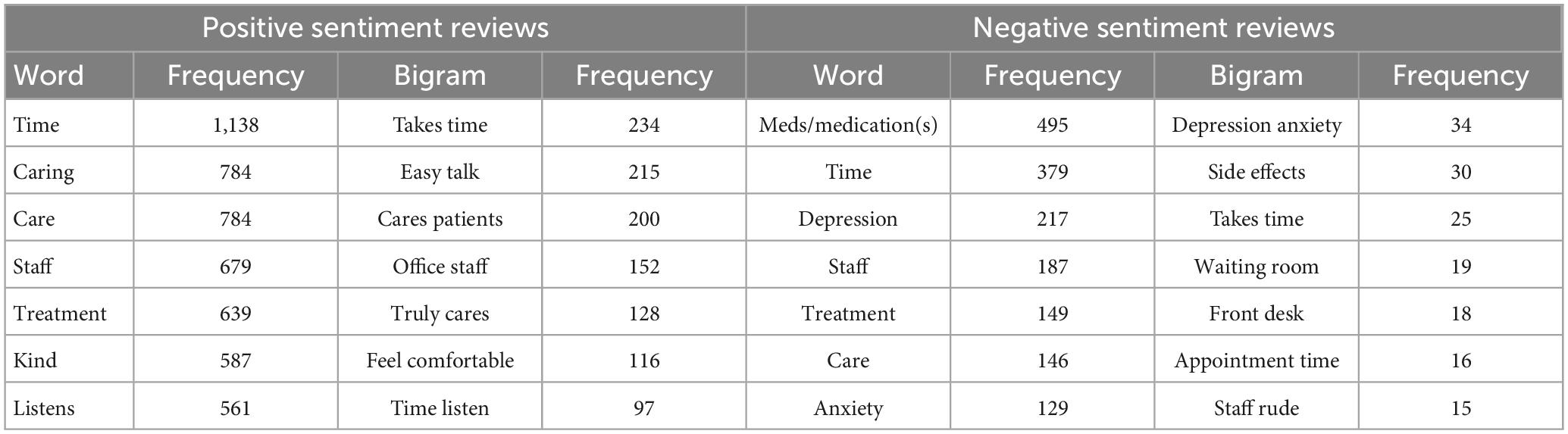
Table 3. Clinically relevant single-word and bigram frequency analysis of reviews with the most positive and negative sentiment.
A multivariate logistic regression was performed to determine how much certain clinically relevant keywords contribute to the review being a positive or a negative review. Words and bigrams strongly associated with positive reviews included “listens,” “great listener,” “takes time,” “kind,” “empathetic,” “comfortable,” “caring,” and “compassionate.” Words and bigrams associated with negative reviews included “meds,” “side effect,” “complication,” and “waiting room.” The odds ratios of these words, which reflect the odds of the terms receiving an overall positive or negative sentiment score, have been summarized in Table 4.
4. Discussion
Psychiatrists have recognized the need to leverage objective feedback to understand what patients expect from their mental health services and deliver the best care (16). While several patient satisfaction scales of psychiatrists have been developed and utilized to understand patient needs (17, 18), PRWs offer a more naturalistic and easily accessible set of reviews that psychiatrists can use to improve their services. The present study examines how patients are reviewing their psychiatrists online in the US by identifying words that are strongly associated with positive and negative reviews, in addition to studying the relationships between demographic factors, star ratings, and sentiment scores. Demographic analyses revealed that younger psychiatrists have significantly higher star ratings than older psychiatrists. Psychiatrists who practice in the Northeast region of the US also received significantly higher star ratings than psychiatrists who practice in the Southwest region. Linguistic analyses showed that positive reviews tend to have a high concentration of single words and bigrams relating to the amount of time taken by the psychiatrists to listen to their patients as well as words pertaining to the care and empathy that the psychiatrists showed for their patients. While negative reviews also emphasized the amount of time that psychiatrists took for their patients, they tended to focus more on medications and their side effects. To our knowledge, this is the first study that explores how patients are reviewing their psychiatrists on PRWs using a sentiment analysis approach.
Our finding that younger psychiatrists received higher star ratings than older psychiatrists is consistent with previous studies on physician reviews in different specialties. Several other studies have shown that younger physicians tend to have higher ratings than older physicians (19, 20). Considering that there was no significant difference in sentiment scores between the two groups, it is possible that older psychiatrists have been providing care for longer and thus had more time to accumulate negative ratings (21). It is also possible that older psychiatrists handle more difficult cases than younger psychiatrists, increasing the likelihood of their obtaining lower star ratings. Because different psychiatric subspecialties have differing patient demographics, more targeted analyses focusing on psychiatrists in specific subspecialties may reveal bias present in such populations and its overall effects on the sentiment of written reviews and star ratings.
Further demographic analyses showed that psychiatrists who practice in the Northeast region of the US received higher star ratings than those in the Southwest region. This finding may be tied to differential proportions of substance use and mental health facilities that only accept certain types of insurance. Previous studies have shown that the proportion of substance use facilities accepting Medicaid is lower in many Southern states than in other areas of the United States (22). Alternatively, other studies have demonstrated that general treatment prevalence in the South and Midwest was lower, relative to the Northeast (23). It is important to note that different policies and ways of practice contribute to the structural forces that ultimately shape longitudinal care and patient satisfaction (24).
Our linguistic analyses demonstrated that the reviews of psychiatrists with the most positive sentiment included words and bigrams pertaining to “time,” “listening,” and “caring.” These findings lend more quantitative and objective support to the existing evidence that highlights how much a psychiatrist’s particular interpersonal skills matter in shaping patient satisfaction (17). For example, the concentration on words related to “listen” and “care” aligns with some of the major categories defined in a recent patient-derived patient satisfaction scale of psychiatrists (17). Indeed, previous studies have shown that star rating is primarily driven by physicians’ bedside manner (25). Along this line of work, our findings emphasize certain behavioral characteristics that may guide psychiatrists in strengthening their therapeutic alliance with their patients.
On the other end of the spectrum, our linguistic analyses showed that words related to “time,” “medications,” and “side effects” significantly contribute to reviews having a higher degree of negative sentiment. It has been shown that most negative reviews online focus on patients’ interaction with psychiatrists and clinic staff (26). Specifically, as “time” seems to be critical in shaping patients’ perception of psychiatrists, if psychiatrists can make their patients feel as though they are taking their time for the patients, patients may view and rate their psychiatrists more favorably. This is much easier to do, of course, if the flow of daily work actually includes adequate time to thoughtfully engage in care (27). Additionally, previous studies have demonstrated that denials of requests for medication were associated with significantly lower patient satisfaction (28). Furthermore, physicians’ communication ability that provides clear medication consumption behavior also tended to have higher returns (5). Consistent with these findings, our results further highlight the significance and impact of medication selection, education, shared decision making, and their adverse effects on how favorably patients perceive their psychiatrists (29).
There are several limitations to our study. First, the 400 psychiatrists whose reviews were analyzed in this study represent a small subsection of the 38,411 actively practicing psychiatrists in the United States (30). Hence, patient reviews of these psychiatrists may not represent the full spectrum of patient perceptions of different forms of psychiatric care, either in the United States or abroad. Nevertheless, the 6,400 patient reviews examined in this sample nevertheless are on par with the sample size found in other sentiment analysis studies of patient reviews in mental healthcare (12). Given today’s accessibility of different publicly available physician rating websites across the globe, future studies may extend this approach by including other physician rating platforms and identifying common attributes of psychiatrists that positively shape patients’ perception of psychiatrists. Second, we did not consider the demographic distribution of patients who submit ratings and scores on PRWs. Patients’ characteristics were unavailable on the publicly accessible website utilized in this study and therefore not controlled. It is likely that younger patients who are more technologically literate compared to their older counterparts leave more online reviews; if the younger patients demonstrate a bias toward younger psychiatrists, the distribution of patients who leave reviews may have differentially affected the ratings of their psychiatrists. Thirdly, it is possible that single reviewers may have submitted multiple reviews for the same provider. Even if the phrases used in the written reviews by the single reviewers are unique, the overall sentiment and the star ratings may have accumulated and emphasized psychiatrists skewed toward one particular preference. Finally, the study did not consider the heterogeneity of psychiatric subspecialties. Though we were concerned with the general trend of the reviews and ratings of psychiatrists on PRWs, further analyses looking at specific subspecialties of psychiatrists may reveal trends and biases within patient-provider interactions specific to that patient population as well as their effects on the overall sentiment and ratings of psychiatrists on PRWs.
In conclusion, the present study conducted a sentiment analysis of reviews of psychiatrists from PRWs to determine demographic biases in the reviews and highlight attributes of psychiatrists that are associated with either positive or negative sentiment. By generating objective sentiment scores of the written reviews and identifying behavioral and clinical characteristics that patients care the most about, psychiatrists can gain greater insight on how to ameliorate their care to reinforce their therapeutic alliance with their patients. Further analyses examining how patients rate their psychiatrists are encouraged to include similar analyses on other PRWs to evaluate whether or not the overall online behavior of patients is consistent with our findings from healthgrades.com.
Data availability statement
The original contributions presented in this study are included in the article/supplementary material, further inquiries can be directed to the corresponding author.
Author contributions
SP contributed to the analysis and interpretation of the results and to the composition of the manuscript. CC contributed to the design of the analysis plan. NB, TS, and WT supported the interpretation and analysis of the data. WT contributed to the study design and edited the manuscript. All authors have critically reviewed the manuscript and approved the final version of the manuscript.
Conflict of interest
The authors declare that the research was conducted in the absence of any commercial or financial relationships that could be construed as a potential conflict of interest.
Publisher’s note
All claims expressed in this article are solely those of the authors and do not necessarily represent those of their affiliated organizations, or those of the publisher, the editors and the reviewers. Any product that may be evaluated in this article, or claim that may be made by its manufacturer, is not guaranteed or endorsed by the publisher.
Footnotes
References
1. Ardito R, Rabellino D. Therapeutic alliance and outcome of psychotherapy: historical excursus, measurements, and prospects for research. Front Psychol. (2011) 2:270. doi: 10.3389/fpsyg.2011.00270
2. Martin D, Garske J, Davis M. Relation of the therapeutic alliance with outcome and other variables: a meta-analytic review. J Consult Clin Psychol. (2000) 68:438–50.
3. Elvins R, Green J. The conceptualization and measurement of therapeutic alliance: an empirical review. Clin Psychol Rev. (2008) 28:1167–87.
4. He L, He C, Wang Y, Hu Z, Zheng K, Chen Y. What do patients care about? Mining fine-grained patient concerns from online physician reviews through computer-assisted multi-level qualitative analysis. AMIA Annu Symp Proc. (2020) 2020:544–53.
5. Bidmon S, Elshiewy O, Terlutter R, Boztug Y. What patients value in physicians: analyzing drivers of patient satisfaction using physician-rating website data. J Med Internet Res. (2020) 22:e13830.
6. Schulz P, Rothenfluh F. Influence of health literacy on effects of patient rating websites: survey study using a hypothetical situation and fictitious doctors. J Med Internet Res. (2020) 22:e14134. doi: 10.2196/14134
7. Ellimoottil C, Hart A, Greco K, Quek M, Farooq A. Online reviews of 500 urologists. J Urol. (2013) 189:2269–73.
8. Frost C, Mesfin A. Online reviews of orthopedic surgeons: an emerging trend. Orthopedics. (2015) 38:e257–62.
9. Trehan S, Daluiski A. Online patient ratings: why they matter and what they mean. J Hand Surg. (2016) 41:316–9. doi: 10.1016/j.jhsa.2015.04.018
10. Tang J, Arvind V, Dominy C, White C, Cho S, Kim J. How are patients reviewing spine surgeons online? A sentiment analysis of physician review website written comments. Glob Spine J. (2022): [Epub ahead of print]. doi: 10.1177/21925682211069933
11. Tang J, Arvind V, White C, Dominy C, Kim J, Cho S, et al. Using sentiment analysis to understand what patients are saying about hand surgeons online. Hand. (2021): [Epub ahead of print]. doi: 10.1177/15589447211060439
12. Compagner C, Lester C, Dorsch M. Sentiment analysis of online reviews for selective serotonin reuptake inhibitors and serotonin–norepinephrine reuptake inhibitors. Pharmacy. (2021) 9:27. doi: 10.3390/pharmacy9010027
13. Liu J, Gao L. Research on the characteristics and usefulness of user reviews of online mental health consultation services: a content analysis. Healthcare. (2021) 9:1111. doi: 10.3390/healthcare9091111
14. Sullivan K, Burden M, Keniston A, Banda J, Hunter L. Characterization of anonymous physician perspectives on COVID-19 using social media data. Pac Symp Biocomput. (2020) 26:95–106.
15. Hutto C, Gilbert E editors. Vader: a parsimonious rule-based model for sentiment analysis of social media text. Proceedings of the international AAAI conference on web and social media. Ann Arbor, MI: AAAI (2014).
16. Bachlani A, Gibson J. Why not patient feedback on psychiatric services? Psychiatrist. (2011) 35:117.
17. Hansen L, Vincent S, Harris S, David E, Surafudheen S, Kingdon D. A patient satisfaction rating scale for psychiatric service users. Psychiatrist. (2010) 34:485–8.
18. Barker D, Shergill S, Higginson I, Orrell M. Patients’ views towards care received from psychiatrists. Br J Psychiatry. (1996) 168:641–6.
19. Calixto N, Chiao W, Durr M, Jiang N. Factors impacting online ratings for otolaryngologists. Ann Otol Rhinol Laryngol. (2018) 127:521–6.
20. Donnally C, McCormick J, Li D, Maguire J, Barker G, Rush A, et al. How do physician demographics, training, social media usage, online presence, and wait times influence online physician review scores for spine surgeons? J Neurosurg Spine. (2018) 30:279–88. doi: 10.3171/2018.8.SPINE18553
21. Basa K, Jabbour N, Rohlfing M, Schmoker S, Lawlor C, Levi J, et al. Online reputations: comparing hospital-and patient-generated ratings in academic otolaryngology. Ann Otol Rhinol Laryngol. (2021) 130:1317–25. doi: 10.1177/00034894211005985
22. Cummings J, Wen H, Ko M, Druss B. Race/ethnicity and geographic access to medicaid substance use disorder treatment facilities in the United States. JAMA Psychiatry. (2014) 71:190–6. doi: 10.1001/jamapsychiatry.2013.3575
23. McDonald D, Jalbert S. Geographic variation and disparity in stimulant treatment of adults and children in the United States in 2008. Psychiatr Serv. (2013) 64:1079–86. doi: 10.1176/appi.ps.004442012
24. Brown A, Ma G, Miranda J, Eng E, Castille D, Brockie T, et al. Structural interventions to reduce and eliminate health disparities. Am J Public Health. (2019) 109:S72–8.
25. Shemirani N, Castrillon J. Negative and positive online patient reviews of physicians—1 vs 5 stars. JAMA Facial Plast Surg. (2017) 19:435–6. doi: 10.1001/jamafacial.2016.2039
26. Newman A. Negative reviews on the internet. In: Ash P, Frierson RL, Friedman SH editors. Malpractice and liability in psychiatry. Berlin: Springer (2022). p. 281–7.
27. Torrey W, Griesemer I, Carpenter-Song E. Beyond “Med Management”. Psychiatr Serv. (2017) 68:618–20.
28. Jerant A, Fenton J, Kravitz R, Tancredi D, Magnan E, Bertakis K, et al. Association of clinician denial of patient requests with patient satisfaction. JAMA Intern Med. (2018) 178:85–91. doi: 10.1001/jamainternmed.2017.6611
29. Torrey W, Drake R. Practicing shared decision making in the outpatient psychiatric care of adults with severe mental illnesses: redesigning care for the future. Commun Ment Health J. (2010) 46:433–40. doi: 10.1007/s10597-009-9265-9
Keywords: physician rating websites, sentiment analysis, psychiatrist, therapeutic alliance, medication side effects
Citation: Park SH, Cheng CP, Buehler NJ, Sanford T and Torrey W (2023) A sentiment analysis on online psychiatrist reviews to identify clinical attributes of psychiatrists that shape the therapeutic alliance. Front. Psychiatry 14:1174154. doi: 10.3389/fpsyt.2023.1174154
Received: 25 February 2023; Accepted: 29 May 2023;
Published: 15 June 2023.
Edited by:
Jimmy Lee, Institute of Mental Health, SingaporeReviewed by:
Wilson Wen Bin Goh, Nanyang Technological University, SingaporeJunhee Lee, Eulji University, Republic of Korea
Chockalingam Viswesvaran, Florida International University, United States
Copyright © 2023 Park, Cheng, Buehler, Sanford and Torrey. This is an open-access article distributed under the terms of the Creative Commons Attribution License (CC BY). The use, distribution or reproduction in other forums is permitted, provided the original author(s) and the copyright owner(s) are credited and that the original publication in this journal is cited, in accordance with accepted academic practice. No use, distribution or reproduction is permitted which does not comply with these terms.
*Correspondence: Soo Hwan Park, Soo.Hwan.Park.MED@Dartmouth.edu
†These authors have contributed equally to this work