- 1Schizophrenia Division, Centre for Addiction and Mental Health, Toronto, ON, Canada
- 2Institute of Medical Science, University of Toronto, Toronto, ON, Canada
- 3Department of Psychiatry, University of Toronto, Toronto, ON, Canada
- 4Department of Pharmacology and Toxicology, University of Toronto, Toronto, ON, Canada
- 5Pharmacogenetics Research Clinic, Centre for Addiction and Mental Health, Toronto, ON, Canada
- 6Education, Centre for Addiction and Mental Health, Toronto, ON, Canada
- 7Geriatric Mental Health Services, Centre for Addiction and Mental Health, Toronto, ON, Canada
- 8Biostatistics, Centre for Addiction and Mental Health, Toronto, ON, Canada
- 9Department of Computational Medicine and Bioinformatics, University of Michigan Medical School, Ann Arbor, MI, United States
- 10Department of Clinical Pharmacy, University of Michigan College of Pharmacy, Ann Arbor, MI, United States
- 11Department of Psychiatry, University of Michigan Medical School, Ann Arbor, MI, United States
- 12Department of Psychiatry & Behavioral Health, Ohio State University, Columbus, OH, United States
- 13Banting and Best Diabetes Centre, University of Toronto, Toronto, ON, Canada
Psychosis spectrum disorders (PSDs), as well as other severe mental illnesses where psychotic features may be present, like bipolar disorder, are associated with intrinsic metabolic abnormalities. Antipsychotics (APs), the cornerstone of treatment for PSDs, incur additional metabolic adversities including weight gain. Currently, major gaps exist in understanding psychosis illness biomarkers, as well as risk factors and mechanisms for AP-induced weight gain. Metabolomic profiles may identify biomarkers and provide insight into the mechanistic underpinnings of PSDs and antipsychotic-induced weight gain. In this 12-week prospective naturalistic study, we compared serum metabolomic profiles of 25 cases within approximately 1 week of starting an AP to 6 healthy controls at baseline to examine biomarkers of intrinsic metabolic dysfunction in PSDs. In 17 of the case participants with baseline and week 12 samples, we then examined changes in metabolomic profiles over 12 weeks of AP treatment to identify metabolites that may associate with AP-induced weight gain. In the cohort with pre-post data (n = 17), we also compared baseline metabolomes of participants who gained ≥5% baseline body weight to those who gained <5% to identify potential biomarkers of antipsychotic-induced weight gain. Minimally AP-exposed cases were distinguished from controls by six fatty acids when compared at baseline, namely reduced levels of palmitoleic acid, lauric acid, and heneicosylic acid, as well as elevated levels of behenic acid, arachidonic acid, and myristoleic acid (FDR < 0.05). Baseline levels of the fatty acid adrenic acid was increased in 11 individuals who experienced a clinically significant body weight gain (≥5%) following 12 weeks of AP exposure as compared to those who did not (FDR = 0.0408). Fatty acids may represent illness biomarkers of PSDs and early predictors of AP-induced weight gain. The findings may hold important clinical implications for early identification of individuals who could benefit from prevention strategies to reduce future cardiometabolic risk, and may lead to novel, targeted treatments to counteract metabolic dysfunction in PSDs.
1. Introduction
Patients with a psychosis spectrum disorder (PSD) and patients with severe mental illnesses that present with psychotic features, often develop metabolic comorbidities like obesity, type 2 diabetes, and dyslipidemia, leading to a 2-fold increase in cardiovascular mortality rates as compared to the general population (1, 2). While various factors contribute to the metabolic risk in PSDs, antipsychotics (APs), the cornerstone of treatment for many PSDs, worsen elevated baseline risk through metabolic side effects such as weight gain (3–5). Notably, young patients without prior AP exposure (i.e., AP-naïve) are especially susceptible to early AP- induced weight gain (3, 6), although there is individual variability in the propensity to develop AP-induced weight gain that is not fully understood. Overall, there is a need to identify biomarkers and mechanisms of intrinsic and early AP-induced metabolic dysfunction to guide early targeted metabolic treatment strategies in PSD.
In addition to the effects of APs, metabolic dysfunction has been suggested to be intrinsic to the illness of PSDs. For instance, AP-naïve first-episode psychosis patients demonstrate higher prevalence of metabolic syndrome, elevated triglyceride levels, markers of glucose dysregulation, and increased risk for type 2 diabetes as compared to healthy controls, even prior to receiving AP treatment (7–11). The presence of metabolic abnormalities in AP-naïve FEP patients, who are minimally affected by two major confounders, APs and illness duration, is suggestive of an intrinsic metabolic risk that is conferred by the illness of PSDs. However, the mechanisms underlying intrinsic metabolic dysfunction are understudied. Taken together, there is a need to examine AP-naïve patients to identify biomarkers, predictors, and mechanisms of intrinsic and early AP-induced metabolic dysfunction, which would have important implications for guiding early treatment strategies for individuals with PSDs and developing novel, targeted treatments for metabolic dysfunction in PSDs.
Metabolomics represents a novel tool with potential to shed light on metabolic dysfunction in PSD by characterizing global metabolite profiles. For example, metabolomic studies have identified that higher baseline concentrations, and change over time, of the lipid metabolite lysophosphatidylcholine 14:0 were associated with weight gain following olanzapine treatment in first-episode schizophrenia (12). Nonetheless, few studies have examined intrinsic illness markers of metabolic dysregulation and predictors of AP-induced weight gain in patients with minimal-to-no previous exposure.
The objectives of the current pilot study were to: (1) examine whether minimally AP-exposed cases and controls present with different metabolomic profiles that may represent illness biomarkers not confounded by long-term AP use; (2) identify predictors of AP-induced weight gain by comparing baseline metabolomes between minimally AP-exposed cases who do and do not develop clinically significant body weight (≥5%) following 12 weeks of AP exposure; and (3) examine associations between changes in the metabolome and body weight over 12 weeks of AP exposure.
2. Materials and methods
2.1. Participants and study design
Participant data was gathered from three independent observational studies, where recruitment has been completed for two case-only studies [(ClinicalTrials.gov ID: NCT02744313. ClinicalTrials.gov ID is not available for the other study) and is ongoing for the remaining case-control study (ClinicalTrials.gov ID: NCT03414151)]. The study protocols were approved by the Research Ethics Board of the Centre for Addiction and Mental Health (030/2017 and 060/2014) and University of Michigan Institutional Review Board (HUM00132484). All participants provided informed consent using the MacArthur Competence Assessment Tool for Clinical Research (MacCat-CR) (13) or the Evaluation to Sign an Informed Consent Document for Research (14).
Participants (male and female) between the ages of 12–45 were recruited for two arms of the study: cases (N = 25) and controls (N = 6). Inclusion criteria for the cases were as follows: (1) Minimal exposure to AP treatment, as defined as having previous AP exposure for equal to or less than 2 weeks within the past 3 months, and (2) Diagnostic and Statistical Manual of Mental Disorders-5 (DSM-5) or DSM-4 diagnosis of schizophrenia, schizoaffective disorder, schizophreniform disorder, delusional disorder, brief psychotic disorder, psychotic disorder not otherwise specified (NOS) or unspecified schizophrenia spectrum, major depressive disorder with psychotic symptoms, bipolar I disorder or bipolar II disorder with psychotic features. Psychiatric diagnosis was assessed at baseline by conducting either the Mini Neuropsychiatric Interview (MINI) for Psychotic Disorder Studies Version 7.0.2, Structured Clinical Interview for Axis I DSM-5 Disorders (SCID-5), or a medical chart review. Our diagnostic inclusion criteria were broad as our goal was to capture the metabolic effects of antipsychotics that occur early after medication exposure, and have been documented in multiple severe mental illnesses that are treated with APs (15). Furthermore, it can be challenging to recruit patients with psychotic features in early medication exposure studies if diagnostic criteria are too narrow, given that a diagnosis may not be clear early-on in the treatment of psychotic symptoms (16, 17). The approach of including patients based on symptoms and not specific diagnoses is also congruent with the Research domain criteria (RDoC) framework (18, 19).
Controls were included if they had an absence of current or past DSM-5 diagnosis other than a specific phobia or adjustment disorder. For both arms, exclusion criteria included clinically significant medical conditions (e.g., type 1 or 2 diabetes, kidney/liver disease, cancer, pregnancy), use of select groups of medications (e.g., treatment for lipids, glucose, or weight, anti-inflammatory medications, immunosuppressants), and moderate to severe substance use determined either through medical chart review or MINI.
Cases were treated naturalistically with APs by their psychiatrist and followed for 12 weeks. Baseline and endpoint (week 12) assessments were used for metabolomics assays. Controls were assessed at baseline only. Assessments for both groups included anthropometric measures (body weight, height, BMI, and waist circumference), blood pressure, and fasting laboratory measures (glucose, insulin, and lipid panel).
2.2. Metabolomic analysis
Metabolomic analysis of serum samples was conducted at the Michigan Comprehensive Regional Metabolomics Core at the University of Michigan. All samples were drawn in the morning, after an overnight fast, and after serum separation they were frozen on dry ice and stored in −80°F until time of sample transportation (on dry ice) and analysis. Samples did not undergo any freeze/thaw cycles prior to time of assay. Targeted, quantitative metabolomic analysis was performed for acylcarnitines, amino acids, free fatty acids, and bile acids after extraction and separation. In brief, the acylcarnitine assay involved separation by liquid chromatography and then measurement of metabolites using electrospray ionization and triple-quadrupole mass spectrometry, as described in more detail by Chace and colleagues (20). There were similarities in general separation approach and analysis method for the bile acids, as well with respect to separation by liquid chromatography and then analysis with triple-quadrupole mass spectrometry (21). The amino acids were analyzed with the Phenomenex EZfaast kit (Torrance, CA) by Metabolomics Core staff. Finally, for the free fatty acids analysis, after extraction (22), the lipid-rich fractions were analyzed for free fatty acids using Agilent 5,890 gas chromatograph with an Agilent HP 88 column (23).
2.3. Statistical analysis
Demographic and clinical data were compared using Fisher's exact test, t-tests, or ANOVA as appropriate using IBM Statistical Package for the Social Sciences (SPSS) Version 25. All statistical analyses of the metabolomic datasets were conducted using Metaboanalyst 5.0 (24). The quantified metabolomic datasets were transformed and scaled to achieve normal distribution for conducting parametric statistical analyses. T-tests were used to compare mean baseline metabolite concentrations between (1) AP-naïve cases and controls at baseline, and (2) AP-naïve cases who do and do not develop ≥5% body weight gain at 12 weeks. Finally, changes from baseline to endpoint between AP-naïve cases who did (i.e. ≥5%) and did not develop significant body weight gain were compared with a two-way repeated measures ANOVA test. Pearson correlations were calculated between change in weight and change in each metabolite concentrations from week 1 to week 12 for cases. To control for multiple comparisons, a false discovery rate (FDR) was calculated (25). Metabolites presenting with an FDR ≤0.05 were considered statistically significant. Fold-changes comparing metabolite mean concentrations were calculated to determine the direction of difference between groups of comparison for metabolites that met statistical significance criteria.
3. Results
Twenty-five minimally exposed AP cases were enrolled, and 17 cases completed both baseline and 12-week follow up visits, while the remaining 8 cases had baseline visits only. Six healthy controls completed baseline visits. Among cases, the most common diagnosis was unspecified schizophrenia spectrum and other psychotic disorder (N = 13). The mean duration of AP exposure at baseline was 7.9 days. Detailed participant demographics are available in Table 1.
Following 12 weeks of AP exposure, cases experienced increases in body weight (p < 0.001), BMI (p < 0.001), waist circumference (p = 0.015), LDL cholesterol (p = 0.009), and total cholesterol (p = 0.027) (Table 2). Additionally, a subgroup of cases (N = 9) experienced clinically significant increases (≥5%) in body weight. The distribution of low and moderate metabolic risk medications was not significantly different (p = 0.453; data not shown) between the high and low weight gain groups (Table 2). There was also no significant difference between antipsychotic exposure prior to the baseline visit in the high and low weight gain groups (p = 0.330; data not shown).
3.1. Baseline serum metabolome features distinguishing cases from controls
Overall, 20 amino acids, 20 bile acids, 30 fatty acids, and 29 acylcarnitines were identified and quantified. The supplement contains lists of all metabolites identified in the metabolomics assays. Six fatty acids differentiated the two groups with an FDR of <0.05. Behenic acid (22:0), arachidonic acid [20:4 (n-6)], and myristoleic acid [14:1 (n-5)] were higher at baseline in cases, and palmitoleic acid [16:1 (n-7)t], lauric acid (12:0), and heneicosylic acid (21:0) were higher in controls at baseline. The Supplementary material also contains a table with FDR values for the six metabolites. No differences were observed for baseline levels of acylcarnitines, amino acids, nor bile acids between these two groups.
3.2. Metabolome features associated with variable weight gain (<5 vs. ≥5%) due to antipsychotics
Among the identified and quantified metabolites, none met the FDR threshold for significance as being altered from baseline to week 12 when changes were compared between case groups who gained <5 or ≥5% of their baseline body weight, or when examining associations between changes in metabolite concentrations and body weight over 12 weeks. Among metabolites differentiating these groups at baseline, baseline concentrations of the fatty acid adrenic acid [22:4(n-6)] were significantly elevated in cases who had more than 5% body weight gain compared to those who did not (FDR = 0.0408), as shown in Figure 1.
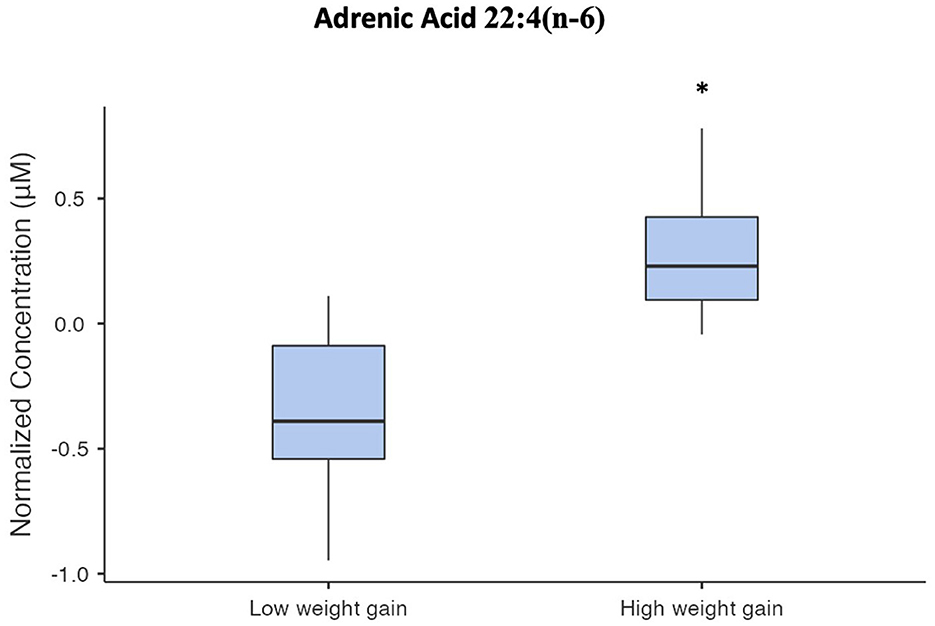
Figure 1. Box and whisker plot of normalized serum adrenic acid concentrations. The asterisk indicates that adrenic acid concentrations in the high-weight gain group were significantly different from the low-weight gain group (FDR = 0.0408).
4. Discussion
Growing evidence supports metabolomic signatures as potential biomarkers for intrinsic and early AP-induced metabolic dysfunction in PSD. In this study, comparisons of metabolomic profiles of minimally AP exposed cases vs. healthy controls at baseline, identified six differential fatty acids. These include reduced palmitoleic acid, lauric acid, and henieocosylic acid levels, and elevated behenic acid, myristoleic acid, and arachidonic acid levels, of which increased arachidonic acid levels are consistent with a previous study (26). Palmitoleic acid and arachidonic acid are particularly interesting due to their roles in inflammation; inflammation has been proposed to underly intrinsic metabolic dysfunction in PSDs (27). Specifically, palmitoleic acid-rich supplementation has been shown to improve dyslipidemia through anti-inflammatory action (28–30). Alternatively, arachidonic acid is an omega-6 polyunsaturated fatty acid (PUFA); PUFAs, which are pro-inflammatory mediators. As such, lower circulating levels of palmitoleic acid and higher circulating levels of arachidonic acid may contribute to inflammation and hence intrinsic metabolic dysfunction in PSDs. Moreover, the conversion of arachidonic acid into inflammatory prostaglandins produces lipid peroxidation products, leading to oxidative stress (31), which in turn has been linked to metabolic dysfunction and PSD (32–35).
However, several association studies have illustrated that elevated circulating palmitoleic acid levels are found in individuals with obesity and metabolic syndrome (36, 37), and associate with higher triglyceride levels and insulin resistance in the general population (38, 39), contrary to the reduced palmitoleic acids observed in our study. Nonetheless, one study demonstrated that in individuals who are at risk for type 2 diabetes, reduced palmitoleic acid levels associate with greater insulin resistance (40). These findings perhaps suggest that the reduction in palmitoleic acid levels found in this study reflects the early, insulin resistant state commonly observed among AP-naïve first-episode psychosis patients (41). Given the small size of this pilot study, an interesting future direction would be to expand these results to a larger patient population to determine if reduced palmitoleic acid and increased arachidonic acid in serum may represent biomarkers of intrinsic metabolic dysfunction in PSD.
With respect to the other fatty acids identified as differentiating the cases and controls at baseline, behenic acid has been associated with anti-inflammatory activity in mice (42), and proinflammatory activity in humans (43). Heneicosylic acid appears to be minimally studied but may be inflammatory when considering it is a saturated fatty acid (44), whereas myristoleic acid and, conversely, the saturated lauric acid have been linked to anti-inflammatory activity (45–47). Figure 2 describes associations between fatty acids identified as significantly different between cases and controls in this pilot study, and whether published literature generally supports their role as inflammatory, or anti-inflammatory.
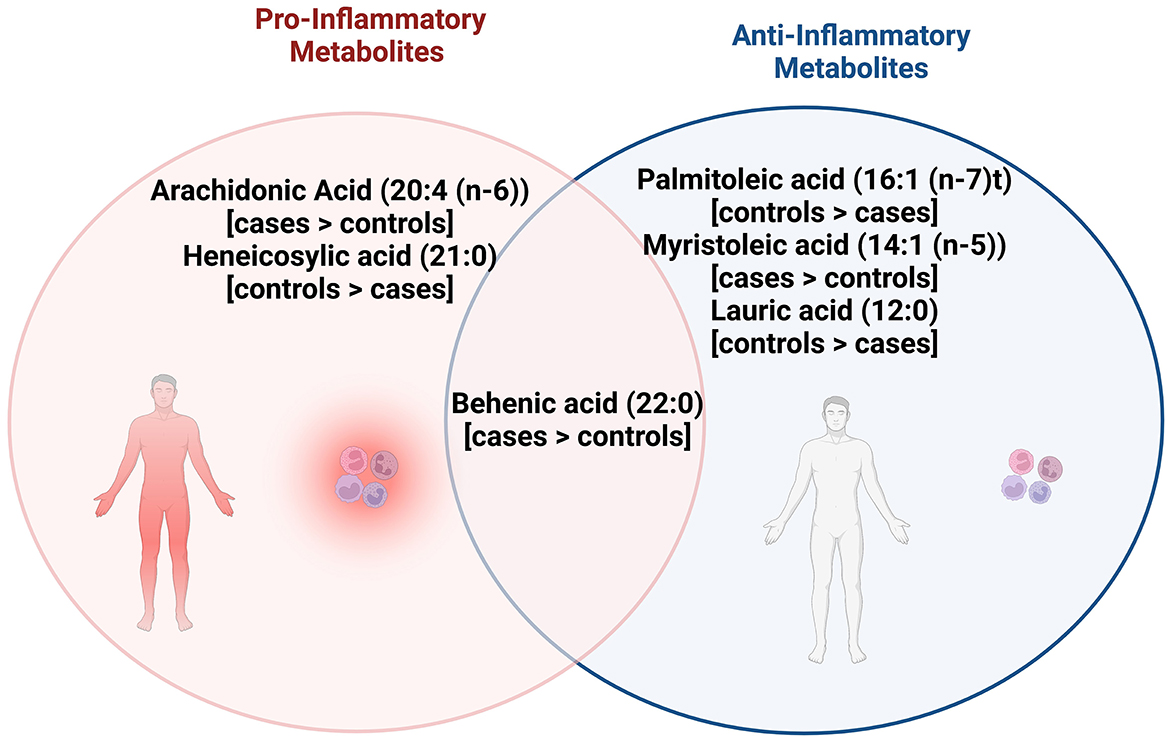
Figure 2. Venn diagram shows the pro and anti-inflammatory fatty acid metabolites that were significantly different between cases and controls, with behenic acid, arachidonic acid, and myristoleic acid observed to be higher in cases while palmitoleic acid, lauric acid, and heneicosylic acid were higher in controls. Behenic acid appears to have both pro and anti-inflammatory actions while arachidonic acid and heneicosylic acid have pro-inflammatory actions. Palmitoleic acid, myristoleic acid, lauric acid appear to have anti-inflammatory actions.
Following 12 weeks of AP exposure, cases had increased in body weight, BMI, waist circumference, total cholesterol, and LDL cholesterol. This increase in weight despite the fact that many of the cases were using antipsychotics with lower weight gain propensity is not necessarily surprising, given that research has suggested all antipsychotics cause weight gain in patients with minimal past exposure, but that the extent of weight gain is different between individual medications (3). Additionally, a subgroup developed clinically significant body weight gain (≥5%) and were distinguished at baseline by elevated levels of the fatty acid, adrenic acid. Studies have shown that specific triacylglycerols predict increases in body weight and BMI following AP treatment in first-episode psychosis patients (12). Our study extends these findings, suggesting elevation of adrenic acid as an additional novel early predictor of AP-induced weight gain. However, we were not able to replicate research from other teams with respect to the extent of changes in lipids; likely this was attributable to different testing platforms and identifiable metabolites, among other differences in study design, including length of medication exposure (48, 49). It is notable that elevations in adrenic acid have been associated with obesity (50), supporting its potential physiological relevance as a predictor of AP-induced weight gain. Adrenic acid is an omega-6 PUFA, which acts as pro-inflammatory mediators. Thus, it may contribute to inflammation, which may underly AP-induced metabolic dysfunction in patients with PSD (27). These findings may have important clinical implications to help identify individuals who are at high risk for AP-induced weight gain and may benefit from early prevention strategies. However, given the preliminary nature of this study, these results must be replicated in larger studies with longer duration of follow up.
Taken together, the prominence of fatty acid dysregulation in this study extends previous findings suggesting that dysregulated lipid metabolism may underlie psychosis illness and AP-induced metabolic dysfunction (12, 26). Our findings corroborate the growing body of evidence supporting individual variabilities in risk for AP-induced metabolic dysfunction and the importance of identifying early biomarkers that predict AP-induced weight gain.
While the physiological relevance of the identified biomarkers reinforces the clinical value of the findings, several limitations should be noted. First, relative to the case group, the control group had a small sample size, resulting in part from COVID-19 pandemic restrictions, where treatment studies with patients were allowed to continue, whereas healthy control studies were halted. The control group also had a larger percentage of patients who identified as Asian, as compared to the case group. These factors may have limited the statistical power in the analyses. Other potential confounders include physical activity levels and diet patterns, which were not considered in the present investigation. Furthermore, the heterogeneity of APs and diagnoses among cases may also have confounded the findings. While there is evidence demonstrating that the impact of antipsychotics on metabolic side effects is potentially disease agnostic (15), there are documented differences among antipsychotics in their ability to cause metabolic side effects (51, 52). This may, in part, explain why there were no detected changes in metabolites over 12 weeks of AP treatment associated with body weight change. Finally, the minimally exposed AP cases in the study had some AP exposure at baseline, which may have hindered examination of illness metabolomics independent of AP exposure. This may also have prevented investigation of the very early metabolic changes induced by APs, especially considering that changes in glucose metabolism can be induced by a single dose of APs (53). Nonetheless, there are ethical challenges to keeping patients AP-naïve for research purposes, which renders it difficult to impose too many exclusion criteria for an already difficult population to recruit. It is also possible that studies in patients with minimal, vs. absent, antipsychotic exposure may be more valuable to translating precision health research into practice given that it is unlikely antipsychotic treatment would be withheld to wait for biomarker results in a patient presenting acutely with psychosis.
5. Conclusion
Collectively, we demonstrate differences in fatty acid metabolites between controls and minimally exposed AP cases with PSD. Additionally, the fatty acid, adrenic acid, may represent an early predictor of AP-induced weight gain. Collectively, these candidate biomarkers provide mechanistic insight into intrinsic and AP-induced metabolic dysfunction in PSDs and represent potential targets for precision health approaches to mitigate metabolic dysfunction in PSDs. Future studies are needed with larger sample sizes and with cases who fully lack AP exposure to rigorously validate these findings before implementation in a clinical setting.
Data availability statement
The original contributions presented in the study are included in the article/Supplementary material, further inquiries can be directed to the corresponding author.
Ethics statement
The studies involving human participants were reviewed and approved by Research Ethics in Review Board of the Centre for Addiction and Mental Health (030/2017 and 060/2014) and the University of Michigan Institutional Review Board (HUM00132484). Written informed consent to participate in this study was provided by the participants' or legal guardian/next of kin.
Author contributions
JL and KW performed the statistical and bioinformatics analyses. JL wrote the first draft of the manuscript. All authors contributed to study design and subsequent drafts.
Funding
Funding for this work was supported in part by grants from the following centers (no funding agencies were involved in study design, collection, analysis and interpretation of data, writing of the manuscript, or decision to submit for publication): the University of Michigan's Regional Comprehensive Metabolomics Resource Core (grant DK097153). KW was supported by the National Institute of Health (NIH), National Center for Advancing Translational Sciences (NCATS), Award Nos. 2UL1TR000433 and KL2TR002241. KS effort was supported in part by an NIH award from the National Institute of General Medical Sciences (NIGMS; R35GM136312). The content is solely the responsibility of the authors and does not necessarily represent the official views of the NIH. JL is supported by the Hilda and William Courtney Clayton Pediatric Research Fund and LG Rao/Industrial Partners Graduate Student Award from the University of Toronto, and Meighen Family Chair in Psychosis Prevention. MH holds the Meighen Family Chair in Psychosis Prevention, the Cardy Schizophrenia Research Chair, a Danish Diabetes Academy Professorship, a Steno Diabetes Center Fellowship, and a U of T Academic Scholar Award, and is funded by operating grants from the Canadian Institutes of Health Research (CIHR), the Banting and Best Diabetes Center, and the PSI Foundation. IT was supported by National Institute of Mental Health (R01MH122491). GR has received research support from the Canadian Institutes of Health Research (CIHR), University of Toronto, and HLS Therapeutics, Inc. ST has received contracted research funding from Boehringer-Ingelheim, not relevant to the content of this report.
Conflict of interest
GR has received research support from the Canadian Institutes of Health Research (CIHR), University of Toronto, and HLS Therapeutics Inc. MH has consulted with Alkermes. KW has worked on an advisory board with Bioxcel Therapeutics.
The remaining authors declare that the research was conducted in the absence of any commercial or financial relationships that could be construed as a potential conflict of interest.
Publisher's note
All claims expressed in this article are solely those of the authors and do not necessarily represent those of their affiliated organizations, or those of the publisher, the editors and the reviewers. Any product that may be evaluated in this article, or claim that may be made by its manufacturer, is not guaranteed or endorsed by the publisher.
Supplementary material
The Supplementary Material for this article can be found online at: https://www.frontiersin.org/articles/10.3389/fpsyt.2023.1169787/full#supplementary-material
References
1. Hennekens CH, Hennekens AR, Hollar D, Casey DE. Schizophrenia and increased risks of cardiovascular disease. Am Heart J. (2005) 150:1115–21. doi: 10.1016/j.ahj.2005.02.007
2. Correll CU, Solmi M, Veronese N, Bortolato B, Rosson S, Santonastaso P, et al. Prevalence, incidence and mortality from cardiovascular disease in patients with pooled and specific severe mental illness: a large-scale meta-analysis of 3,211,768 patients and 113,383,368 controls. World Psychiatry Off J World Psychiatr Assoc WPA. (2017) 16:163–80. doi: 10.1002/wps.20420
3. Correll CU, Manu P, Olshanskiy V, Napolitano B, Kane JM, Malhotra AK. Cardiometabolic risk of second-generation antipsychotic medications during first-time use in children and adolescents. JAMA. (2009) 302:1765–73. doi: 10.1001/jama.2009.1549
4. Smith E, Singh R, Lee J, Colucci L, Graff-Guerrero A, Remington G, et al. Adiposity in schizophrenia: A systematic review and meta-analysis. Acta Psychiatr Scand. (2021) 144:524–36. doi: 10.1111/acps.13365
5. Pillinger T, McCutcheon RA, Vano L, Mizuno Y, Arumuham A, Hindley G, et al. Comparative effects of 18 antipsychotics on metabolic function in patients with schizophrenia, predictors of metabolic dysregulation, and association with psychopathology: a systematic review and network meta-analysis. Lancet Psychiatry. (2020) 7:64–77. doi: 10.1016/S2215-0366(19)30416-X
6. Pérez-Iglesias R, Martínez-García O, Pardo-Garcia G, Amado JA, Garcia-Unzueta MT, Tabares-Seisdedos R, et al. Course of weight gain and metabolic abnormalities in first treated episode of psychosis: the first year is a critical period for development of cardiovascular risk factors. Int J Neuropsychopharmacol. (2014) 17:41–51. doi: 10.1017/S1461145713001053
7. Garrido-Torres N, Rocha-Gonzalez I, Alameda L, Rodriguez-Gangoso A, Vilches A, Canal-Rivero M, et al. Metabolic syndrome in antipsychotic-naïve patients with first-episode psychosis: a systematic review and meta-analysis. Psychol Med. (2021) 51:2307–20. doi: 10.1017/S0033291721002853
8. Greenhalgh AM, Gonzalez-Blanco L, Garcia-Rizo C, Fernandez-Egea E, Miller B, Arroyo MB, et al. Meta-analysis of glucose tolerance, insulin, and insulin resistance in antipsychotic-naïve patients with nonaffective psychosis. Schizophr Res. (2017) 179:57–63. doi: 10.1016/j.schres.2016.09.026
9. Mitchell AJ, Vancampfort D, De Herdt A, Yu W, De Hert M. Is the prevalence of metabolic syndrome and metabolic abnormalities increased in early schizophrenia? A comparative meta-analysis of first episode, untreated and treated patients. Schizophr Bull. (2013) 39:295–305. doi: 10.1093/schbul/sbs082
10. Misiak B, Stańczykiewicz B, Łaczmański Ł, Frydecka D. Lipid profile disturbances in antipsychotic-naive patients with first-episode non-affective psychosis: A systematic review and meta-analysis. Schizophr Res. (2017) 190:18–27. doi: 10.1016/j.schres.2017.03.031
11. Rajkumar AP, Horsdal HT, Wimberley T, Cohen D, Mors O, Børglum AD, et al. Endogenous and antipsychotic-related risks for diabetes mellitus in young people with schizophrenia: a danish population-based cohort study. Am J Psychiatry. (2017) 174:686–94. doi: 10.1176/appi.ajp.2016.16040442
12. Liu JH, Chen N, Guo YH, Guan XN, Wang J, Wang D, et al. Metabolomics-based understanding of the olanzapine-induced weight gain in female first-episode drug-naïve patients with schizophrenia. J Psychiatr Res. (2021) 140:409–15. doi: 10.1016/j.jpsychires.2021.06.001
13. Grisso T, Appelbaum PS, Hill-Fotouhi C. The MacCAT-T: a clinical tool to assess patients' capacities to make treatment decisions. Psychiatr Serv Wash DC. (1997) 48:1415–9. doi: 10.1176/ps.48.11.1415
14. DeRenzo EG, Conley RR, Love R. Assessment of capacity to give consent to research participation: state-of-the-art and beyond. J Health Care Law Policy. (1998) 1:66–87.
15. Barton BB, Segger F, Fischer K, Obermeier M, Musil R. Update on weight-gain caused by antipsychotics: a systematic review and meta-analysis. Expert Opin Drug Saf. (2020) 19:295–314. doi: 10.1080/14740338.2020.1713091
16. Salvatore P, Baldessarini RJ, Tohen M, Khalsa HMK, Sanchez-Toledo JP, Zarate CA, et al. McLean-Harvard International First-Episode Project: two-year stability of ICD-10 diagnoses in 500 first-episode psychotic disorder patients. J Clin Psychiatry. (2011) 72:183–93. doi: 10.4088/JCP.09m05311yel
17. McGorry PD. The influence of illness duration on syndrome clarity and stability in functional psychosis: does the diagnosis emerge and stabilise with time? Aust N Z J Psychiatry. (1994) 28:607–19. doi: 10.1080/00048679409080784
18. Yamada Y, Matsumoto M, Iijima K, Sumiyoshi T. Specificity and continuity of schizophrenia and bipolar disorder: relation to biomarkers. Curr Pharm Des. (2020) 26:191–200. doi: 10.2174/1381612825666191216153508
19. Insel T, Cuthbert B, Garvey M, Heinssen R, Pine DS, Quinn K, et al. Research domain criteria (RDoC): toward a new classification framework for research on mental disorders. Am J Psychiatry. (2010) 167:748–51. doi: 10.1176/appi.ajp.2010.09091379
20. Chace DH, DiPerna JC, Mitchell BL, Sgroi B, Hofman LF, Naylor EW. Electrospray tandem mass spectrometry for analysis of acylcarnitines in dried postmortem blood specimens collected at autopsy from infants with unexplained cause of death. Clin Chem. (2001) 47:1166–82. doi: 10.1093/clinchem/47.7.1166
21. Griffiths WJ, Sjövall J. Bile acids: analysis in biological fluids and tissues. J Lipid Res. (2010) 51:23–41. doi: 10.1194/jlr.R001941
22. Stringer KA, Serkova NJ, Karnovsky A, Guire K, Paine R, Standiford TJ. Metabolic consequences of sepsis-induced acute lung injury revealed by plasma 1H-nuclear magnetic resonance quantitative metabolomics and computational analysis. Am J Physiol Lung Cell Mol Physiol. (2011) 300:L4–11. doi: 10.1152/ajplung.00231.2010
23. Das AK, Hajra AK. Quantification, characterization and fatty acid composition of lysophosphatidic acid in different rat tissues. Lipids. (1989) 24:329–33. doi: 10.1007/BF02535172
24. Pang Z, Chong J, Zhou G, de Lima Morais DA, Chang L, Barrette M, et al. MetaboAnalyst 5.0: Narrowing the gap between raw spectra and functional insights. Nucleic Acids Res. (2021) 49:W388–96. doi: 10.1093/nar/gkab382
25. Benjamini Y, Hochberg Y. Controlling the false discovery rate: a practical and powerful approach to multiple testing. J R Stat Soc Ser B Methodol. (1995) 57:289–300. doi: 10.1111/j.2517-6161.1995.tb02031.x
26. Yang X, Sun L, Zhao A, Hu X, Qing Y, Jiang J, et al. Serum fatty acid patterns in patients with schizophrenia: a targeted metabonomics study. Transl Psychiatry. (2017) 7:e1176. doi: 10.1038/tp.2017.152
27. Prestwood TR, Asgariroozbehani R, Wu S, Agarwal SM, Logan RW, Ballon JS, et al. Roles of inflammation in intrinsic pathophysiology and antipsychotic drug-induced metabolic disturbances of schizophrenia. Behav Brain Res. (2021) 402:113101. doi: 10.1016/j.bbr.2020.113101
28. Griel AE, Cao Y, Bagshaw DD, Cifelli AM, Holub B, Kris-Etherton PM, et al. macadamia nut-rich diet reduces total and LDL-cholesterol in mildly hypercholesterolemic men and women. J Nutr. (2008) 138:761–7. doi: 10.1093/jn/138.4.761
29. Garg ML, Blake RJ, Wills RBH. Macadamia nut consumption lowers plasma total and LDL cholesterol levels in hypercholesterolemic men. J Nutr. (2003) 133:1060–3. doi: 10.1093/jn/133.4.1060
30. Curb JD, Wergowske G, Dobbs JC, Abbott RD, Huang B. Serum lipid effects of a high-monounsaturated fat diet based on macadamia nuts. Arch Intern Med. (2000) 160:1154–8. doi: 10.1001/archinte.160.8.1154
31. Peet M, Laugharne JDE, Horrobin DF, Reynolds GP. Arachidonic acid: A common link in the biology of schizophrenia? Arch Gen Psychiatry. (1994) 51:665–6. doi: 10.1001/archpsyc.1994.03950080077012
32. Noto C, Ota VK, Gadelha A, Noto MN, Barbosa DS, Bonifácio KL, et al. Oxidative stress in drug naïve first episode psychosis and antioxidant effects of risperidone. J Psychiatr Res. (2015) 68:210–6. doi: 10.1016/j.jpsychires.2015.07.003
33. Yubero-Serrano EM, Delgado-Lista J, Peña-Orihuela P, Perez-Martinez P, Fuentes F, Marin C, et al. Oxidative stress is associated with the number of components of metabolic syndrome: LIPGENE study. Exp Mol Med. (2013) 45:e28. doi: 10.1038/emm.2013.53
34. Henriksen EJ, Diamond-Stanic MK, Marchionne EM. Oxidative stress and the etiology of insulin resistance and type 2 diabetes. Free Radic Biol Med. (2011) 51:993–9. doi: 10.1016/j.freeradbiomed.2010.12.005
35. Patel S, Sharma D, Kalia K, Tiwari V. Crosstalk between endoplasmic reticulum stress and oxidative stress in schizophrenia: The dawn of new therapeutic approaches. Neurosci Biobehav Rev. (2017) 83:589–603. doi: 10.1016/j.neubiorev.2017.08.025
36. Okada T, Furuhashi N, Kuromori Y, Miyashita M, Iwata F, Harada K. Plasma palmitoleic acid content and obesity in children. Am J Clin Nutr. (2005) 82:747–50. doi: 10.1093/ajcn/82.4.747
37. Mayneris-Perxachs J, Guerendiain M, Castellote AI, Estruch R, Covas MI, Fitó M, et al. Plasma fatty acid composition, estimated desaturase activities, and their relation with the metabolic syndrome in a population at high risk of cardiovascular disease. Clin Nutr Edinb Scotl. (2014) 33:90–7. doi: 10.1016/j.clnu.2013.03.001
38. Kurotani K, Sato M, Ejima Y, Nanri A, Yi S, Pham NM, et al. High levels of stearic acid, palmitoleic acid, and dihomo-γ-linolenic acid and low levels of linoleic acid in serum cholesterol ester are associated with high insulin resistance. Nutr Res N Y N. (2012) 32:669–675.e3. doi: 10.1016/j.nutres.2012.07.004
39. Paillard F, Catheline D, Duff FL, Bouriel M, Deugnier Y, Pouchard M, et al. Plasma palmitoleic acid, a product of stearoyl-coA desaturase activity, is an independent marker of triglyceridemia and abdominal adiposity. Nutr Metab Cardiovasc Dis NMCD. (2008) 18:436–40. doi: 10.1016/j.numecd.2007.02.017
40. Stefan N, Kantartzis K, Celebi N, Staiger H, Machann J, Schick F, et al. Circulating palmitoleate strongly and independently predicts insulin sensitivity in humans. Diabetes Care. (2010) 33:405–7. doi: 10.2337/dc09-0544
41. Perry BI, McIntosh G, Weich S, Singh S, Rees K. The association between first-episode psychosis and abnormal glycaemic control: systematic review and meta-analysis. Lancet Psychiatry. (2016) 3:1049–58. doi: 10.1016/S2215-0366(16)30262-0
42. da Silva RM, Moreira DKT, Zarricueta ML, Rabelo e Paiva Caria C, Macedo JA, Macedo GA, et al. The postprandial inflammatory response is attenuated by a dietary structured lipid containing behenic acid. J Funct Foods. (2019) 58:350–4. doi: 10.1016/j.jff.2019.05.013
43. Milanski M, Degasperi G, Coope A, Morari J, Denis R, Cintra DE, et al. Saturated fatty acids produce an inflammatory response predominantly through the activation of TLR4 signaling in hypothalamus: implications for the pathogenesis of obesity. J Neurosci Off J Soc Neurosci. (2009) 29:359–70. doi: 10.1523/JNEUROSCI.2760-08.2009
44. Fritsche KL. The science of fatty acids and inflammation123. Adv Nutr. (2015) 6:293S−301S. doi: 10.3945/an.114.006940
45. Khan HU, Aamir K, Jusuf PR, Sethi G, Sisinthy SP, Ghildyal R, et al. Lauric acid ameliorates lipopolysaccharide (LPS)-induced liver inflammation by mediating TLR4/MyD88 pathway in Sprague Dawley (SD) rats. Life Sci. (2021) 265:118750. doi: 10.1016/j.lfs.2020.118750
46. Sergi D, Luscombe-Marsh N, Naumovski N, Abeywardena M, O'Callaghan N. Palmitic acid, but not lauric acid, induces metabolic inflammation, mitochondrial fragmentation, and a drop in mitochondrial membrane potential in human primary myotubes. Front Nutr. (2021) 8:663838. doi: 10.3389/fnut.2021.663838
47. Rocha DM, Bressan J, Hermsdorff HH. The role of dietary fatty acid intake in inflammatory gene expression: a critical review. São Paulo Med J. (2017) 135:157–68. doi: 10.1590/1516-3180.2016.008607072016
48. Suvitaival T, Mantere O, Kieseppä T, Mattila I, Pöhö P, Hyötyläinen T, et al. Serum metabolite profile associates with the development of metabolic co-morbidities in first-episode psychosis. Transl Psychiatry. (2016) 6:e951. doi: 10.1038/tp.2016.222
49. Kaddurah-Daouk R, McEvoy J, Baillie RA, Lee D, Yao JK, Doraiswamy PM, et al. Metabolomic mapping of atypical antipsychotic effects in schizophrenia. Mol Psychiatry. (2007) 12:934–45. doi: 10.1038/sj.mp.4002000
50. Marco-Ramell A, Tulipani S, Palau-Rodriguez M, Gonzalez-Dominguez R, Miñarro A, Jauregui O, et al. Untargeted profiling of concordant/discordant phenotypes of high insulin resistance and obesity to predict the risk of developing diabetes. J Proteome Res. (2018) 17:2307–17. doi: 10.1021/acs.jproteome.7b00855
51. Siskind D, Gallagher E, Winckel K, Hollingworth S, Kisely S, Firth J, et al. Does Switching Antipsychotics Ameliorate Weight Gain in Patients With Severe Mental Illness? A Systematic Review and Meta-analysis. Schizophr Bull. (2021) 47:948–58. doi: 10.1093/schbul/sbaa191
52. Rummel-Kluge C, Komossa K, Schwarz S, Hunger H, Schmid F, Lobos CA, et al. Head-to-head comparisons of metabolic side effects of second generation antipsychotics in the treatment of schizophrenia: a systematic review and meta-analysis. Schizophr Res. (2010) 123:225–33. doi: 10.1016/j.schres.2010.07.012
Keywords: metabolomics, fatty acids, antipsychotics, schizophrenia, weight gain, psychosis
Citation: Lee J, Costa-Dookhan K, Panganiban K, MacKenzie N, Treen QC, Chintoh A, Remington G, Müller DJ, Sockalingam S, Gerretsen P, Sanches M, Karnovsky A, Stringer KA, Ellingrod VL, Tso IF, Taylor SF, Agarwal SM, Hahn MK and Ward KM (2023) Metabolomic signatures associated with weight gain and psychosis spectrum diagnoses: A pilot study. Front. Psychiatry 14:1169787. doi: 10.3389/fpsyt.2023.1169787
Received: 20 February 2023; Accepted: 27 March 2023;
Published: 24 April 2023.
Edited by:
Jaspreet Brar, UPMC Western Psychiatric Hospital, United StatesReviewed by:
Silje Skrede, University of Bergen, NorwayChao Deng, University of Wollongong, Australia
Melanie Foecking, Royal College of Surgeons in Ireland, Ireland
Copyright © 2023 Lee, Costa-Dookhan, Panganiban, MacKenzie, Treen, Chintoh, Remington, Müller, Sockalingam, Gerretsen, Sanches, Karnovsky, Stringer, Ellingrod, Tso, Taylor, Agarwal, Hahn and Ward. This is an open-access article distributed under the terms of the Creative Commons Attribution License (CC BY). The use, distribution or reproduction in other forums is permitted, provided the original author(s) and the copyright owner(s) are credited and that the original publication in this journal is cited, in accordance with accepted academic practice. No use, distribution or reproduction is permitted which does not comply with these terms.
*Correspondence: Kristen M. Ward, a213aWVzZUB1bWljaC5lZHU=
†These authors have contributed equally to this work and share senior authorship