- 1Department of Graduate School, Wannan Medical College, Wuhu, An Hui, China
- 2Student Health Center, Wannan Medical College, Wuhu, An Hui, China
- 3Department of Surgical Nursing, School of Nursing, Jinzhou Medical University, Jinzhou, Liaoning, China
- 4Department of Occupational and Environmental Health, Key Laboratory of Occupational Health and Safety for Coal Industry in Hebei Province, School of Public Health, North China University of Science and Technology, Tangshan, Hebei, China
- 5Obstetrics and Gynecology Nursing, School of Nursing, Wannan Medical College, Wuhu, An Hui, China
- 6Department of Emergency and Critical Care Nursing, School of Nursing, Wannan Medical College, Wuhu, An Hui, China
- 7Department of Internal Medicine Nursing, School of Nursing, Wannan Medical College, Wuhu, An Hui, China
- 8Department of Pediatric Nursing, School of Nursing, Wannan Medical College, Wuhu, An Hui, China
- 9Department of Surgical Nursing, School of Nursing, Wannan Medical College, Wuhu, An Hui, China
- 10Rehabilitation Nursing, School of Nursing, Wanna Medical College, Wuhu, An Hui, China
Objective: Depressive symptom is a serious mental illness often accompanied by physical and emotional problems. The prevalence of depressive symptom in older adults has become an increasingly important public health priority. Our study used cardiometabolic indicators to predict depressive symptom in middle-aged and older adults in China.
Methods: The data came from the China Health and Retirement Longitudinal Study 2011 (CHARLS2011), which was a cross-sectional study. The analytic sample included 8,942 participants aged 45 years or above. The study evaluated the relationship between cardiometabolic indicators and depression by measuring 13 indicators, including body mass index (BMI), waist circumference, waist-height ratio (WHtR), conicity index, visceral adiposity index (VAI), Chinese visceral adiposity index (CVAI), lipid accumulation product (LAP), a body shape index (ABSI), body roundness index (BRI), triglyceride glucose index (TyG-index) and its correlation index (TyG-BMI, TyG-waist circumference, TyG-WHtR). Binary logistic regression analysis was used to examine the association between thirteen cardiometabolic indicators and depressive symptom. In addition, the receiver operating characteristic (ROC) curve analysis and area under curve (AUC) were used to evaluate the predictive anthropometric index and to determine the optimum cut-off value.
Results: The study included 8,942 participants, of whom 4,146 (46.37%) and 4,796 (53.63%) were male and female. The prevalence of depressive symptom in mid-aged and older adults in China was 41.12% in males and 55.05% in females. The results revealed that BMI [AUC = 0.440, 95%CI: 0.422–0.457], waist circumference [AUC = 0.443, 95%CI: 0.425–0.460], WHtR [AUC = 0.459, 95%CI: 0.441–0.476], LAP [AUC = 0.455, 95%CI: 0.437–0.472], BRI [AUC = 0.459, 95%CI: 0.441–0.476], CVAI [AUC = 0.449, 95%CI: 0.432–0.467], TyG-BMI [AUC = 0.447, 95%CI: 0.429–0.465], and TyG-waist circumference [AUC =0.452, 95%CI: 0.434–0.470] were weak predictors of depressive symptom (p < 0.05) in males. In females, BMI [AUC = 0.470, 95%CI: 0.453–0.486], LAP [AUC = 0.484, 95%CI: 0.467–0.500], TyG-BMI [AUC = 0.470, 95%CI: 0.454–0.487], and TyG-waist circumference [AUC =0.481, 95%CI: 0.465–0.498] were weak predictors of depressive symptom (p < 0.05). On the other side, VAI, ABSI, conicity index and TyG index could not predict depressive symptom in middle-aged and older adults.
Conclusion: Most cardiometabolic indicators have important value in predicting depressive symptom. Our results can provide measures for the early identification of depressive symptom in middle-aged and older adults in China to reduce the prevalence of depressive symptom and improve health.
Introduction
One of the most common mental illnesses worldwide is depression. Depression is generally defined as a mental condition characterized by low mood, decreased interest and impaired cognitive function. Not only does it affect the brain’s perceptual function, but it can also cause mental and physical difficulties in humans. It is also known as depressive symptom or clinical depression (1). A study in 2023 estimated that 3.8% of the world’s population will suffer from depression, including 5.0% of adults and 5.7% of people over 60 (2). The prevalence of depression is related to national cultural differences. The prevalence of depression in older adults is reported to be 11.5% in 2021 in Korea and 23.7% in 2019 in Thailand (3, 4). The prevalence of geriatric depression symptom in China in 2022 is 38.27% (5). Depression will not only impair human function, but also increase the cost of medical, thus bringing a huge burden to both developed and developing countries (6). Researchers conducted a meta-analysis in 2019 that showed life expectancy with disability per 1,000 people with depression and post-traumatic stress disorder was more than five times the current global average burden of disease (7).
Depressive symptom in older adults is related to many factors. Overall, most surveys have shown that basic sociodemographic factors such as gender, age, marriage, education, and occupation were associated with depressive symptom (8–11). For example, one study found that women have a higher rate of depressive symptom than men, possibly because women have higher levels of inflammatory, neurotrophic and serotonergic markers than men (12). In addition, the influence of depressive symptom on metabolic disorders may be more severe in people with a high prevalence of overweight/obesity (13). Multiple studies have shown that waist circumference, elevated triglyceride (TG) levels and fasting blood sugar, as well as the co-existence of obesity and depression, all increase the risk of cardiovascular diseases (14–16). Kinds of literature have described that waist circumference, body mass index (BMI), waist-height ratio (WHtR), lipid accumulation index (LAP) and other indicators have been widely used in epidemiology to measure obesity or central obesity (17–20).
It is important to note that there is no consensus on the relationship between cardiometabolic indicators and depressive symptom. For example, BMI has been utilized in numerous studies looking for a link between obesity and depressive symptom to forecast increased fat buildup. A study in Germany showed that BMI could be used as a predictor of depression (21). In addition, Ma et al. (22) took BMI and WHtR as continuous variables and used logistic regression and RCS models to explore the obvious nonlinear relationship between obesity and depression. In one meta-analysis, the risk of depression rose 38% in both men and women with increased waist circumference (23). Nevertheless, the main limitations of waist circumference include its sensitivity to body size, fat proportion, and distribution, as well as its correlation with BMI (24). In a United States study, Lei et al. (25) found that VAI was associated with the occurrence of clinical depressive symptom after controlling for confounding factors. Previous studies (26, 27) have shown that these cardiometabolic indicators were associated with depressive symptom, in fact, most studies involved only a single or small number of indicators, and there was no consensus on anthropometric indicators that predict depressive symptom (19, 28). In addition, most studies are based on the Western environment, and the results are different due to ethnic and cultural differences. Therefore, in order to promote the management of depressive symptom in middle-aged and older adults, further studies are needed to determine the relevance of cardiometabolic indicators in predicting depressive symptom in middle-aged and older adults.
As far as we know, there is limited research on this aspect in China. Therefore, this study aimed to investigate the association of cardiometabolic indicators (e.g., BMI, LAP, CVAI) with depressive symptoms among middle-aged and older adults in China. To do so, we used a national study examining the health of China’s rapidly aging society. This study can provide a theoretical basis for pre-onset prevention of depressive symptoms and clinical non-drug treatment, enrich the knowledge and understanding of depression-related factors in mid-aged and older adults, make them realize that reducing depressive symptoms can improve the quality of life, and also provide a basis for future clinical intervention and decision-making.
Methods
Study design and setting
The China Health and Retirement Longitudinal Study (CHARLS) is a nationwide follow-up survey of middle-aged and older adults in China over the age of 45 and their spouses. Every 2 years, the respondents underwent face-to-face Computer Assisted Personal Interviewing (CAPI) were followed up. The baseline survey of CHARLS used a four-stage hierarchical clustering method of random sampling. In the first stage, the regions, rural/urban status, and gross domestic product per capita data were used to stratify each county in China. A total of 150 counties were randomly selected as research objects, representing the socio-economic and geographical types of all counties. In the second stage, 3 PSUs were selected for each county-level unit and scale proportional probability sampling was used. In the third stage, the residents in each building were listed, and 24 households were randomly selected from the respondents aged ≥45 years. Finally, a resident aged ≥45 years was randomly selected as the main respondent and his or her spouse was interviewed at the same time. After completing the questionnaire and measurement, an 8 ml fasting blood sample was taken by a trained caregiver from respondents who had fasted for at least 8 h. A complete blood count (CBC) test is performed within 1–2 h of sampling. Whole blood samples were stored at 4°C for the subsequent determination of HbA1C. The remaining blood samples were separated into plasma and red blood cells and stored at −20°C. All the blood samples have been transported to Beijing and stored at −70°C at the Chinese Center for Disease Control for testing (29). The CHARLS began in 2011 with a cohort of 20,103 participants between the ages of 45 and 101 (Waves1). We excluded individuals who met any of the criteria at baseline (1) depressive symptom data missing, (2) one of the 13 indices missing, (3) age/sex/educational levels/marital status/current smoking/alcohol drinking/exercise/chronic diseases/live place/activities missing. Finally, 8,942 people aged 45 and older were included in our study. Figure 1 shows a flow diagram of the study individuals. All data are openly published as microdata at1 with no direct contact with all participants. Approval for this study was given by the medical ethics committee of Wannan Medical College (approval number 2021–3).
Participants
Study subjects for this investigation were chosen from the CHARLS, Wave 1 (2011). The average age of the 8,942 participants participating in CHARLS was 59.07 years (standard deviation SD = 9.54, range 45–98 years). Males had a mean age of 60.16 years (SD = 9.26; range 45–98 years), while females had a mean age of 58.13 years (SD = 9.69; range 45–91 years).
Baseline characteristics
Covariates
Baseline characteristics including age, sex (1 = male; 2 = female), education (1 = illiterate; 2 = less than elementary school; 3 = high school; 4 = above vocational school), marital status (1 = married; 2 = single), living place (1 = rural; 2 = urban), smoking status (1 = no; 2 = former smoke; 3 = current smoke), drinking status (1 = no; 2 = less than once a month; 3 = more than once a month), taking activities (1 = no; 2 = yes), and having regular exercises (1 = no; 2 = less than exercises; 3 = regular exercises),and the counts of chronic diseases (0 = 0; 1 = 1–2; 2 = 3–14) were collected by self-report. The majority of factors were based on our earlier research investigations (30–35). “Having regular exercises” was defined as exercising at least 3 days a week and over 30 min a day, including moderate to intense physical activity and walking (34).
Depressive symptom
The Center for Epidemiological Research Depression Scale (CES-D) in Chinese has been a good level of validity and reliability and is frequently used to assess depressive symptom in Chinese adults (36–39). The CES-D-10 has 10 items with 4 response options: (1) rarely or never (1 day); (2) sometimes or rarely (1–2 days); (3) occasionally or occasionally but not frequently (3–4 days); and (4) most or always (>1 day; 5–7 days). The values of the four options range from 0 to 3. A lower number denotes a lesser level of depressive symptom, and the total score goes from 0 to 30. A cutoff score ≥ 10 was used to identify respondents with significant depressive symptom (27, 40). The Cronbach alpha coefficient was 0.80 and the construct validity was 0.61 (31).
Measurements
BMI was calculated based on the participants’ measured weight and height. BMI was divided into three categories under the Chinese standard definition: obesity (BMI ≥ 28 kg/m2), overweight (24 ≤ BMI <28 kg/m2), and underweight and normal (BMI < 24 kg/m2) (41). Waist circumference was measured with a flexible measuring tape at a level midway between the lower rib margin (35). The measurement method of conicity index was completed by waist circumference, weight, and height (42). BRI, ABSI, and VAI were calculating the following equations, it was important to note that VAI, unlike other anthropometrics, was divided into men and women (24, 43, 44). WHtR was defined as the waist circumference (m) divided by the height (m) (45). LAP was calculated slightly differently by subtracting a number (males: 65 cm, females: 58 cm) from waist circumference and multiplying by TG (46). CVAI was based on VAI to develop a more appropriate measure for Chinese people (47). The trained investigators measured the participants’ physical characteristics using standardized equipment, including their standing height, weight, and waist circumference [Index: height; Equipment: SecaTM213 Stadiometer Manufacturer/source: China Seca (Hangzhou) Co., Ltd. Index: weight; Equipment: OmronTMHN-286Scale Manufacturer/source: Krill Technology (Yangzhou) Co., Ltd. Index: waist circumference; Equipment: Soft Tape Measure Manufacturer/source: None. Venous blood sample: Standard blood collection materials] (29). The other anthropometric indexes were measured by the following formulas:
(1) BMI =
(2) WHtR =
(3) Males: VAI =
Females: VAI =
(4) ABSI =
(5) BRI =
(6) Males: LAP = [waist circumference (cm)-65] × TG (mmol/l)
Females: LAP = [waist circumference (cm)-58] × TG (mmol/l)
(7) Conicity Index =
(8) Males:
CVAI = −267.93 + 0.68 × age+0.03 × BMI (kg/m2) + 4.00 × waist circumference (cm) + 22.00 × Log10TG (mmol/l) − 16.32 × HDL-C (mmol/l)
Females:
CVAI = −187.32 + 1.71 × age+4.32 × BMI (kg/m2) + 1.12 × waist circumference (cm) + 39.76 × Log10TG (mmol/l) − 11.66 × HDL-C (mmol/l)
(9) TyG index = Ln[(TG (mg/dl) × glucose (mg/dl)/2)]
(10) TyG-BMI = TyG × BMI
(11) TyG-waist circumference = TyG × Waist circumference
(12) TyG-WHtR = TyG × WHtR
Statistical analysis
All the statistical analyses were analyzed using the SPSS software, version 25.0 (IBM SPSS, Armonk, NY, United States). Statistical significance was set at p < 0.05. To compare dichotomous or categorical variables, a chi-squared test was performed. Results of gender-stratified studies were also provided, as the connection between depressive symptom and obesity status differs in sexes. Continuous variables were measured using mean values and SD (continuous data). In order to evaluate the variations in mean distributions by sex, independent samples t-tests were utilized. After adjusting for age, education, marital status, current residence, current smoking, alcohol consumption, activity participation, regular exercise and chronic diseases, 13 indicators were used as independent variables and depressive symptom as dependent variables to evaluate the relationship between cardiometabolic indicators and depressive symptom. We found that there was no difference between these missing data and all data in gender, age and other indicators, so we adopted the direct deletion method for missing data. The unadjusted and adjusted relationships between anthropometrics and depressive symptom were evaluated using binary logistic regression, and odds ratios (ORs) and 95% confidence intervals (95% CI) were calculated after controlling for confounding factors. The ability of these indicators to identify depressive symptom was tested by drawing receiver operating curve (ROC) and calculating area under curve (AUC). The sensitivity, specificity, positive predictive value, negative predictive value, positive likelihood ratio, and negative likelihood ratio can all be calculated using the ROC curve. The cutoff points were chosen using the Youden index (sensitivity + specificity 1) to assess classification accuracy.
Results
Table 1 shows the characteristics of participants with full samples. A total of 8,942 subjects were included in this study, of whom 4,146 (46.37%) were male and 4,796 (53.63%) were female. Most of them were 45–64 years old, there were significant differences between men and women in age, education, marital status, current smoking, alcohol drinking, number of chronic diseases, waist circumference, BMI, WHtR, VAI, ABSI, BRI, LAP, conicity index, CVAI, TyG index, TyG-BMI, TyG-waist circumference and TyG-WHtR (p < 0.05). However, the distribution of current residence, taking activities, and having regular exercises were not statistically significant between the male and female subgroups (p > 0.05). Because of these significant differences between males and females (p < 0.05), we performed the main analyses separately by sex.
Table 2 shows the baseline characteristics of the study participants with and without depressive symptom by sex. According to the research results, the proportion of women suffering from depressive symptom was much higher (55.05%, compared to 41.12% for men). Men with depressive symptom had significant differences in age, education, marital status, current residence, alcohol drinking, taking activities, chronic diseases, waist circumference, BMI, WHtR, BRI, LAP, CVAI, TyG-BMI, TyG-waist circumference and TyG-WHtR (p < 0.05); women had significant differences in age, education, marital status, current residence, smoking, having regular exercises, chronic diseases, BMI, VAI, ABSI, LAP, conicity index, TyG-BMI, and TyG-waist circumference, (p < 0.05). There was no significant difference in the TyG index between subgroups of patients with and without depressive symptom, whether in men or women (p > 0.05).
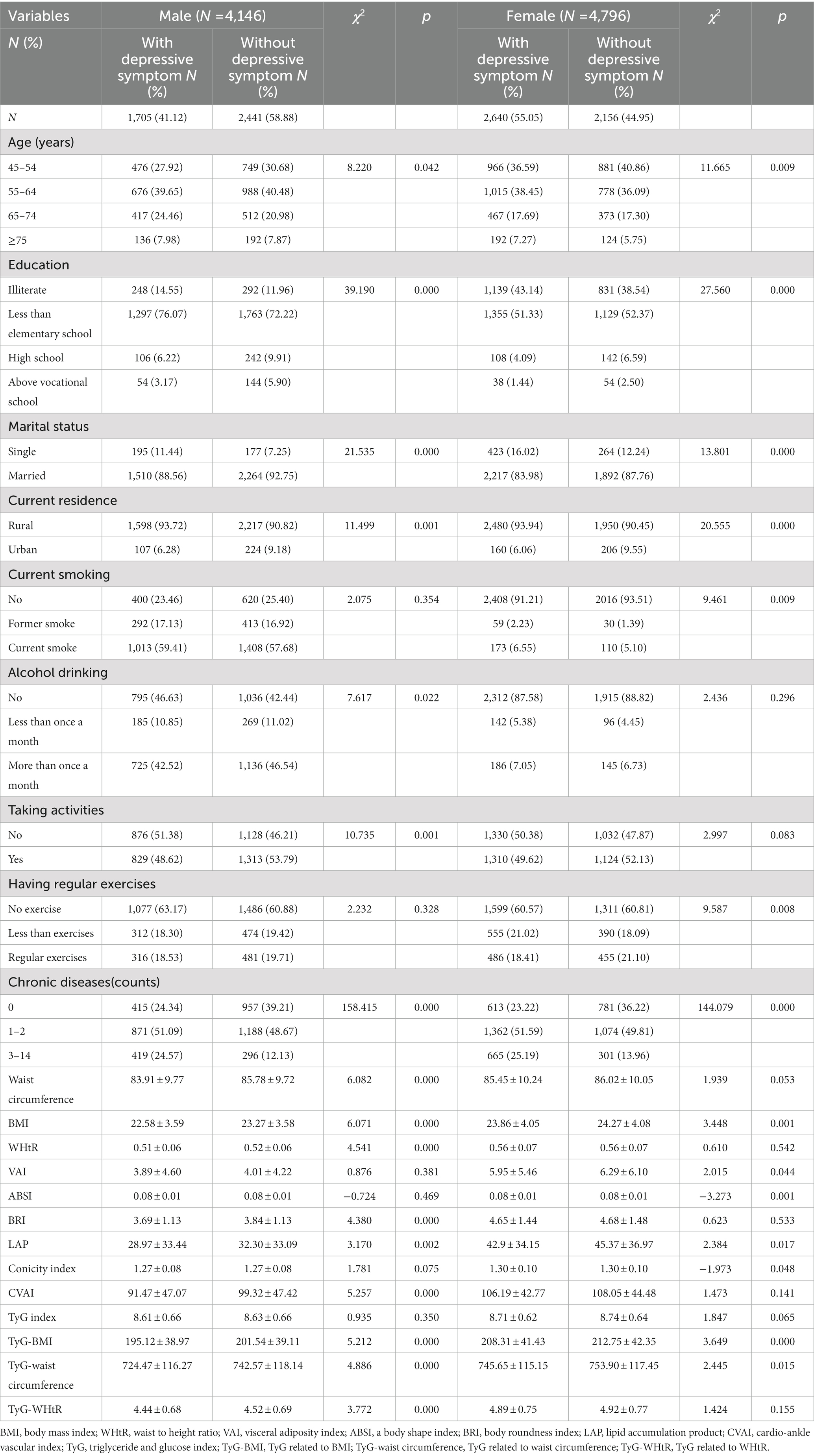
Table 2. Baseline characteristics of the study participants with and without depressive symptom by sex.
Table 3 shows the associations of cardiometabolic indicators with depressive symptom. After controlling for age, education, marital status, current residence, current smoking, alcohol drinking, taking activities, having regular exercises, and chronic diseases, the odds ratio in waist circumference (OR = 0.978, 95%CI: 0.972–0.985), BMI (OR = 0.939, 95%CI: 0.920, 0.957), WHtR (OR = 0.042, 95%CI: 0.013–0.133), BRI (OR = 0.854, 95%CI: 0.805–0.905), LAP (OR = 0.996, 95%CI: 0.994–0.998), conicity index (OR = 0.369, 95%CI: 0.168–0.809), CVAI (OR = 0.996, 95%CI: 0.994–0.997), TyG-BMI (OR = 0.995, 95%CI: 0.993–0.997), TyG-waist circumference (OR = 0.998, 95%CI: 0.998–0.999), and TyG-WHtR (OR = 0.797, 95%CI: 0.724–0.877) in males and waist circumference (OR = 0.990, 95%CI: 0.984–0.995), BMI (OR = 0.968, 95%CI: 0.953, 0.982), WHtR (OR = 0.273, 95%CI: 0.111–0.672), VAI (OR = 0.985, 95%CI: 0.975–0.995), BRI (OR = 0.941, 95%CI: 0.903–0.980), LAP (OR = 0.997, 95%CI: 0.995–0.998), CVAI (OR = 0.997, 95%CI: 0.995–0.998), TyG index (OR = 0.856, 95%CI: 0.779–0.940), TyG-BMI (OR = 0.996, 95%CI: 0.995–0.998), TyG-waist circumference (OR = 0.999, 95%CI: 0.998–0.999), and TyG-WHtR (OR = 0.855, 95%CI: 0.789–0.926) in females were significantly correlated with depressive symptom. In addition, ABSI was not significantly associated with depressive symptom in either men or women.
Table 4 shows the predictive value is evaluated by the ROC curve and AUC. The ROC curves of each indicator in predicting depressive symptom risk in men and women are shown in Figures 2, 3 respectively. In men, the AUCs of waist circumference, BMI, WHtR, BRI, LAP, CVAI, TyG-BMI, TyG-waist circumference, and TyG-WHtR showed weak but significant power in predicting depressive symptom (p < 0.05). It can be observed from the table that WHtR (AUC = 0.459, SE = 0.009, 95% CI = 0.441–0.476, and optimal cut-off = 0.754) and BRI (AUC = 0.459, SE = 0.009, 95% CI = 0.441–0.476, and optimal cut-off =9.371) had similar predictive values. In contrast, the AUC of ABSI did not reach statistical significance (p = 0.518). In women, the AUCs of BMI, LAP, TyG-BMI, and TyG-waist circumference showed weak but significant power in predicting depressive symptom (p < 0.05). Similarly, the predictive values were similar for the BMI (AUC = 0.470, SE = 0.008, 95% CI = 0.453–0.486, and optimal cut-off = 33.997), TyG-BMI (AUC = 0.470, SE = 0.008, 95% CI = 0.454–0.487, and optimal cut-off =113.997). In addition, there was no statistical significance in the AUC of VAI, conicity index and TyG index between men and women (p > 0.05).
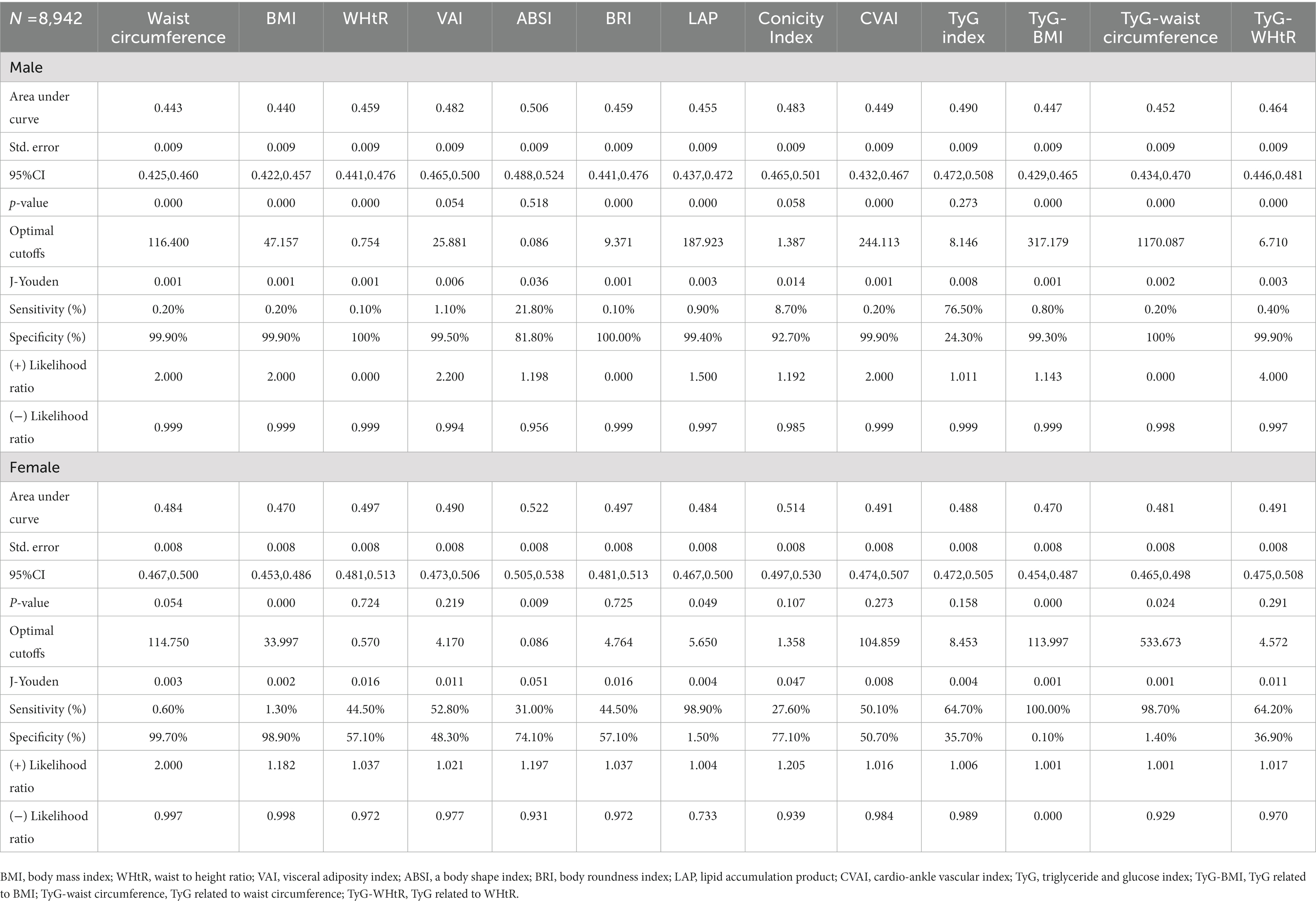
Table 4. Cut-off between area under curve, sensitivity, and specificity for cardiometabolic indicators to detect depressive symptom by sex.
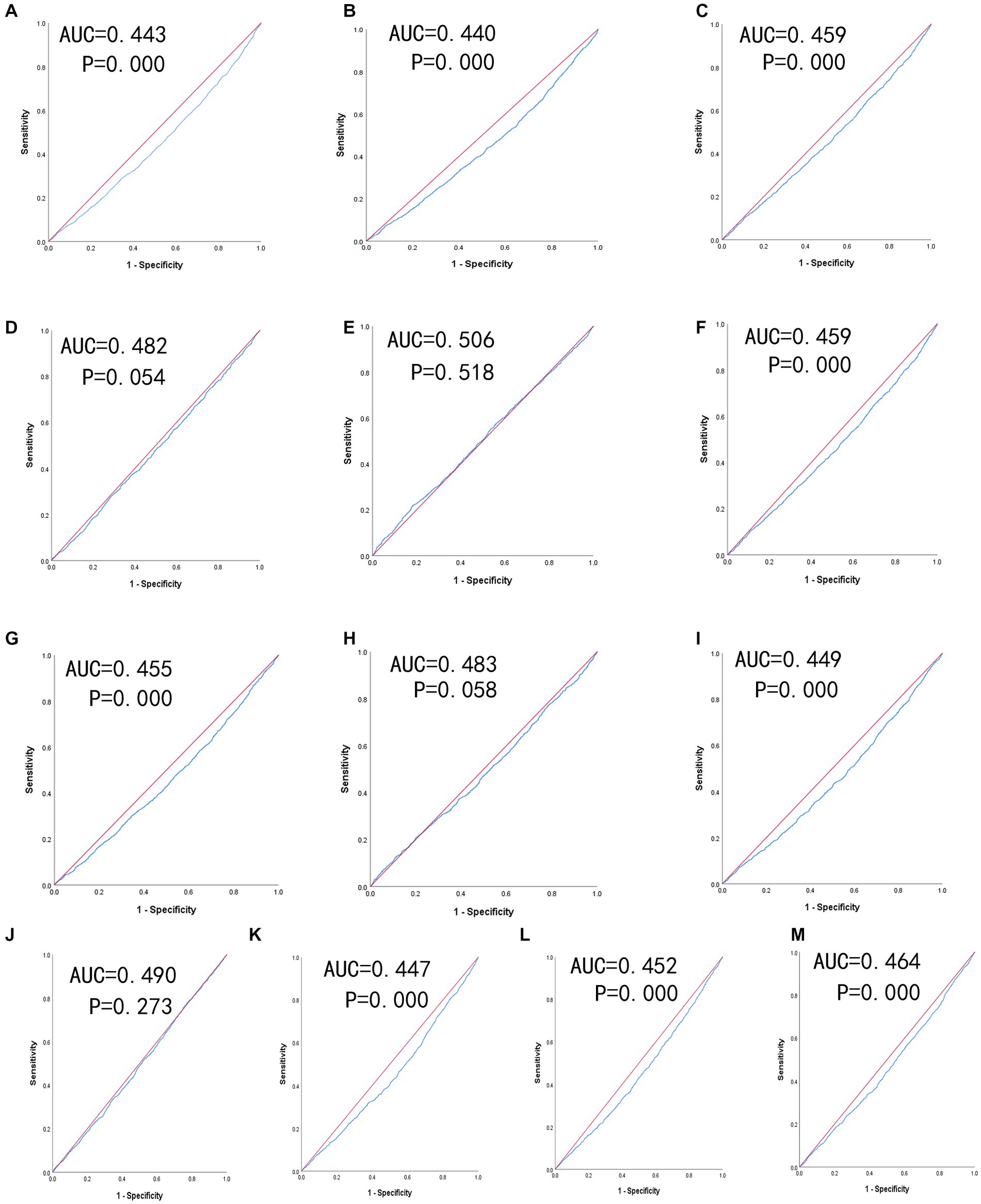
Figure 2. The ROC curves of each indicator in the prediction of depressive symptom risk in males. (A) waist circumference, (B) BMI, (C) WHtR, (D) VAI, (E) ABSI, (F) BRI, (G) LAP, (H) conicity index, (I) CVAI, (J) TyG-index, (K) TyG-BMI, (L) TyG-waist circumference, (M) TyG-WHtR.
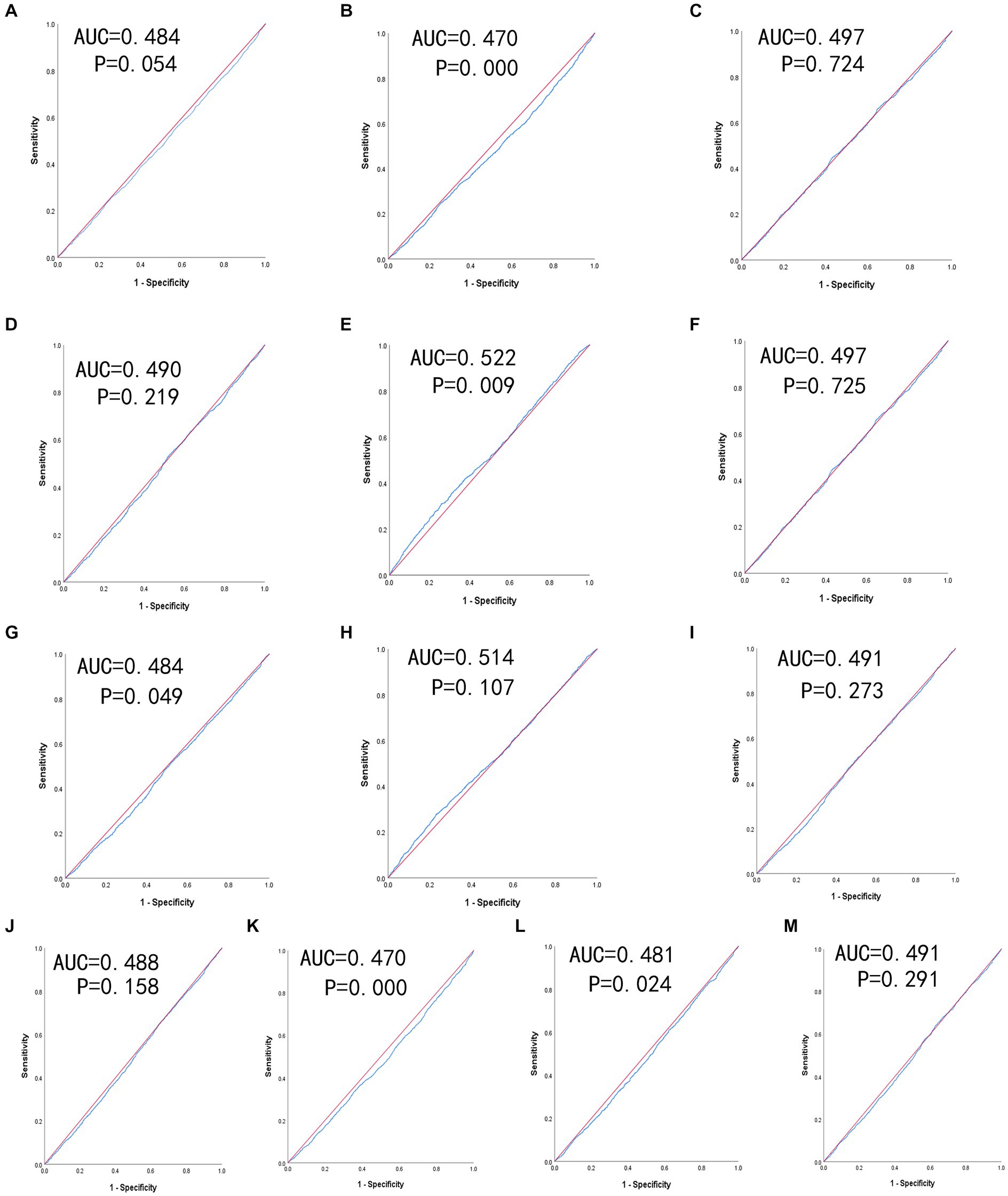
Figure 3. The ROC curves of each indicator in the prediction of depressive symptom risk in females. (A) waist circumference, (B) BMI, (C) WHtR, (D) VAI, (E) ABSI, (F) BRI, (G) LAP, (H) conicity index, (I) CVAI, (J) TyG-index, (K) TyG-BMI, (L) TyG-waist circumference, (M) TyG-WHtR.
Discussion
With the aging problem becoming more and more serious, the influences of depressive symptom on the quality of life and survival of older adults cannot be ignored. Therefore, efficient and simple screening tools are essential to identify patients with depressive symptom. In our study, we found that there was a significant negative correlation between cardiometabolic indicators and depressive symptom, but there were differences in gender (male and female). For example, waist circumference (OR = 0.978, 95%CI: 0.972–0.985), WHtR (OR = 0.042, 95%CI: 0.013–0.133), BRI (OR = 0.854, 95%CI: 0.805–0.905) and CVAI (OR = 0.996, 95%CI: 0.994–0.997) were negatively correlated with depressive symptom in men, but not in women. In addition, BMI, TyG-waist circumference, TyG-BMI and LAP had a certain ability to predict depressive symptom.
Our data showed that BMI-adjusted OR in men and women was inversely associated with depressive symptom, with changes in BMI indicating a higher risk of depressive symptom, the results of this study were similar to several Asian studies (19, 48). In addition, studies have shown that inflammatory cytokines are associated with BMI Obesity and central fat can increase plasma concentrations of inflammatory cytokines leading to low levels of systemic inflammation (49–51), and an increase in inflammatory cytokines can make depressive symptom and anxiety worse (52). So, in our study, something like BMI could reduce depressive symptom by screening patients for depressive symptom, and Vittengl et al. (53) have shown the same idea.
In recent years, the TyG index has emerged as a novel marker for insulin resistance. Many observational studies (54–56) found that diabetes and insulin resistance can exacerbate depressive symptom. In South Korea, a sizable cross-sectional investigation found that depressive symptom risk increased by 4% in young people and 17% in non-diabetics when insulin resistance was raised (57). Multiple studies (58, 59) have found that high levels of Tyg-related indicators perform better in assessing depressive symptom in adults. In our study, TyG-related factors such as TyG-BMI, and TyG-waist circumference can provide a broader basis for cardiometabolic indicators to estimate depressive symptom. Many earlier studies (60–62) shared similar views to our findings, but they involved fewer participants. With the increasing prevalence of chronic diseases in older adults, the correlation between the TyG index and depressive symptom can be further explored in future studies.
Among other measures, LAP was also significant in predicting depressive symptom. LAP was first proposed by Kahn et al. (46). With the increase of abdominal fat accumulation, TG concentration increased, and the association of metabolic insulin resistance was enhanced (63, 64) and LAP was used as a predictor of insulin resistance (65, 66). In this study, LAP was negatively correlated with depressive symptom in males (OR = 0.996, 95%CI: 0.994–0.998), as well as in females (OR = 0.997, 95%CI: 0.995–0.998). Moreover, in ROC analysis, the predicted values were statistically significant (p < 0.05), which could be used as a valuable predictor of depressive symptom.
In addition, the study showed that ABSI was not an ideal predictor compared to BMI and LAP. According to Lotfi et al. (67), there was a substantial positive correlation between ABSI and psychological discomfort in women (OR = 1.40; 95%CI: 1.07, 1.84), but not present in men. On the other hand, they found no significant association between BRI and depressive symptom. These findings were inconsistent with our results. In our study, there was no correlation between ABSI and depressive symptom, which was consistent with Hadi et al. (68). In addition, by observing the ROC curve of cardiometabolic indicators, it is found that the area of the ROC curve of male ABSI had no statistical significance (p > 0.05). In contrast, one study (69) showed superior predictive power and a significant association between BRI and high cardiometabolic risk, and BRI was inversely associated with the incidence of depressive symptom in our study.
Interestingly, there was a significant correlation between waist circumference, WHtR, and depressive symptom in our study. Consistent with our study, Liao et al. (70) found that the dose–response relationship between waist circumference and depressive symptom showed a linear trend. Similarly, among overweight and obese Americans, those with larger waists or bellies were more likely to have severe depressive symptom (71). In prospective research with 6,355 people, WHtR was assessed as the best predictor of cardiovascular risk and mortality (followed by waist circumference and WHR) (72). In Zhi et al.’s (73) study, abdominal obesity was only associated with depression in adult Iranian women. On the contrary, in our study, we found that waist circumference and WHtR had some significance in predicting depressive symptom in men. Waist circumference and WHtR measurements were simple and low-cost, allowing medical professionals to use them as reference factors for future depressive symptom in men.
VAI is the visceral cardiometabolic index, studies (74) showed that VAI reflected the deposition degree of adipose tissues, it used to be common in Caucasians, but VAI had a poor correlation with adipose tissue area in Chinese (75). A cross-sectional study of adults in the United States found that for every unit increase in VAI, the incidence of clinical depression increased by 14% (25). However, in our study, VAI was weakly associated with depressive symptom and could not be used as a predictor of depressive symptom in both men and women. In 2016, Xia et al. (47) developed a clinical indicator, CVAI, to predict the visceral fat region in Chinese adults, which can be used to assess metabolic health in Asians. This was similar to our study, in which CVAI was negatively correlated with depressive symptom. In addition, our data showed that CVAI can be used as a predictor of depressive symptom in men, but not in women.
By contrast, conicity index was not an ideal predictor. Longitudinal studies have confirmed that the severity of depressive symptom correlates with the risk of Alzheimer’s disease, and that the risk of major depressive symptom is higher (76, 77). This was consistent with our findings that conicity index was not associated with depressive symptom. Interestingly, Confortin et al. (78) reported that in addition to conicity index, BMI, waist circumference and WHtR had up to one-third independent association with dementia. Meanwhile, in the older adults (aged 61–90) of the Federal District (Brazil), Brito et al. discovered a correlation between dementia and WHtR but no correlation with conicity index (79).
This study mainly discussed the correlation and prediction between cardiometabolic indicators and depressive symptom, deepened the importance of early detection of depressive symptom based on previous studies, made up for the deficiency of single content of previous studies to a certain extent, and enriched the epidemiological prevention and treatment of depressive symptom. In the field of public health, our findings can provide a reference for clinical work, public health consultation, and identification of high-risk groups for preventive treatment. Therefore, clinicians should monitor depressive symptom in obese populations. Such monitoring can lead to the prevention or early diagnosis of depressive symptom and can subsequently reduce the high disease burden in the population.
Strengths and limitations of the study
Our study has several advantages. In the past, a single index was used to predict the prevalence of depressive symptom, which may cause bias. In this study, we compared the relation of 13 cardiometabolic indicators on depressive symptom, which was helpful for early screening. Secondly, most of the indicators in this study were simple and easy to operate, which can be used in clinical large-scale. In addition, this study included 8,942 respondents aged 45 and above, which ensured the accuracy of this study and has great theoretical and practical significance for the prevention and control of depressive symptom. However, some limitations should be considered. Firstly, the cross-sectional design limits study of the causal relationship between cardiometabolic indicators and depressive symptom. Secondly, although several covariables were considered in our analysis, some residual confounding factors may still influence the results. Thirdly, the data in this study were collected in 2011 and may not reflect the updated relationships between variables; however, the data could contribute to estimating the trend change by adding to the representative evidence and provide a comparison for future intervention and policymaking.
Conclusion
In summary, our findings suggested that most cardiometabolic indicators were associated with depressive symptom. BMI, LAP, TyG-BMI, and TyG-waist circumference predict the correlation of depressive symptom in our study. For example, we can prevent or delay the onset and development of depressive symptom by changing the BMI of middle-aged and older adults. The results of this study may have important implications for the prevention of depressive symptom. Our findings highlight the need to raise awareness of depressive symptom and improve healthcare. Especially in the case of the increasing prevalence of depressive symptom year by year, it can further prevent the cognitive dysfunction of older adults and improve the overall quality of life of the older adults. At the same time, it lays a solid foundation for further research on depressive symptom in the future.
Data availability statement
The original contributions presented in the study are included in the article/supplementary material, further inquiries can be directed to the corresponding author/s.
Ethics statement
The studies involving human participants were reviewed and approved by medical ethics committee of Wannan Medical College (approval number 2021–3). The patients/participants provided their written informed consent to participate in this study.
Author contributions
LZ conceived and designed the research. YW wrote the paper. YW and LZ analyzed the data. YW, XZ, YLi, JG, YM, XY, LZ, HL, LG, JinlL, YLe, XL, LS, LY, TY, CW, DZ, JingL, ML, and YH revised the paper. All authors contributed to the article and approved the submitted version.
Funding
CHARLS was supported by the NSFC (70910107022 and 71130002) and National Institute on Aging (R03-TW008358-01; R01-AG037031-03S1), and World Bank (7159234) and the Support Program for Outstanding Young Talents from the Universities and Colleges of Anhui Province for LZ (gxyqZD2021118).
Acknowledgments
The authors thank the members of the research as well as all participants for their contribution.
Conflict of interest
The authors declare that the research was conducted in the absence of any commercial or financial relationships that could be construed as a potential conflict of interest.
Publisher’s note
All claims expressed in this article are solely those of the authors and do not necessarily represent those of their affiliated organizations, or those of the publisher, the editors and the reviewers. Any product that may be evaluated in this article, or claim that may be made by its manufacturer, is not guaranteed or endorsed by the publisher.
Abbreviations
BMI, body mass index; WHtR, waist-height ratio; VAI, visceral adiposity index; CVAI, cardio-ankle vascular index; LAP, lipid accumulation product; ABSI, a body shape index; BRI, body roundness index; TyG, triglyceride glucose; TyG-WHtR, triglyceride glucose-waist-height ratio; TyG-BMI, triglyceride glucose-body mass index; TyG-waist circumference, triglyceride glucose-waist circumference; CHARLS, China Health and Retirement Longitudinal Study; SD, standard deviation; CES-D, Center for Epidemiological Research Depression Scale; OR, odds ratio; 95% CI, 95% confidence intervals; ROC, receiver operator curve; AUC, area under curve; TG, triglyceride.
Footnotes
References
1. Gou, Y, Wu, N, Xia, J, Liu, Y, Yang, H, Wang, H, et al. Province-and individual-level influential factors of depression: multilevel cross-provinces comparison in China. Front Public Health. (2022) 10:893280. doi: 10.3389/fpubh.2022.893280
2. Cai, H, Jin, Y, Liu, R, Zhang, Q, Su, Z, Ungvari, GS, et al. Global prevalence of depression in older adults: a systematic review and meta-analysis of epidemiological surveys. Asian J Psychiatr. (2023) 80:103417. doi: 10.1016/j.ajp.2022.103417
3. Park, GR, and Seo, BK. Mental health among the Korean older population: how is it related to asset-based welfare? J Appl Gerontol. (2021) 40:142–51. doi: 10.1177/0733464820917295
4. Wongpakaran, N, Wongpakaran, T, Lerttrakarnnon, P, Jiraniramai, S, Sirirak, T, Assanangkornchai, S, et al. Prevalence, clinical and psychosocial variables of depression, anxiety and suicidality in geriatric tertiary care settings. Asian J Psychiatr. (2019) 41:38–44. doi: 10.1016/j.ajp.2018.10.007
5. Li, H, Liu, X, Zheng, Q, Zeng, S, and Luo, X. Gender differences and determinants of late-life depression in China: a cross-sectional study based on CHARLS. J Affect Disord. (2022) 309:178–85. doi: 10.1016/j.jad.2022.04.059
6. Pano, O, Martínez-Lapiscina, EH, Sayón-Orea, C, Martinez-Gonzalez, MA, Martinez, JA, and Sanchez-Villegas, A. Healthy diet, depression and quality of life: a narrative review of biological mechanisms and primary prevention opportunities. World J Psychiatry. (2021) 11:997–1016. doi: 10.5498/wjp.v11.i11.997
7. Charlson, F, van Ommeren, M, Flaxman, A, Cornett, J, Whiteford, H, and Saxena, S. New WHO prevalence estimates of mental disorders in conflict settings: a systematic review and meta-analysis. Lancet. (2019) 394:240–8. doi: 10.1016/S0140-6736(19)30934-1
8. Akhtar-Danesh, N, and Landeen, J. Relation between depression and sociodemographic factors. Int J Ment Heal Syst. (2007) 1:4. doi: 10.1186/1752-4458-1-4
9. Barua, A, Ghosh, MK, Kar, N, and Basilio, MA. Socio-demographic factors of geriatric depression. Indian J Psychol Med. (2010) 32:87–92. doi: 10.4103/0253-7176.78503
10. Kessler, RC, and Bromet, EJ. The epidemiology of depression across cultures. Annu Rev Public Health. (2013) 34:119–38. doi: 10.1146/annurev-publhealth-031912-114409
11. Milanović, SM, Erjavec, K, Poljičanin, T, Vrabec, B, and Brečić, P. Prevalence of depression symptoms and associated socio-demographic factors in primary health care patients. Psychiatr Danub. (2015) 27:31–7.
12. Labaka, A, Goñi-Balentziaga, O, Lebeña, A, and Pérez-Tejada, J. Biological sex differences in depression: a systematic review. Biol Res Nurs. (2018) 20:383–92. doi: 10.1177/1099800418776082
13. Coryell, WH, Butcher, BD, Burns, TL, Dindo, LN, Schlechte, JA, and Calarge, CA. Fat distribution and major depressive disorder in late adolescence. J Clin Psychiatry. (2016) 77:84–9. doi: 10.4088/JCP.14m09169
14. Faulconbridge, LF, Driscoll, CFB, Hopkins, CM, Bailer Benforado, B, Bishop-Gilyard, C, Carvajal, R, et al. Combined treatment for obesity and depression: a pilot study. Obesity. (2018) 26:1144–52. doi: 10.1002/oby.22209
15. Ratliff, JC, Palmese, LB, Reutenauer, EL, Srihari, VH, and Tek, C. Obese schizophrenia spectrum patients have significantly higher 10-year general cardiovascular risk and vascular ages than obese individuals without severe mental illness. Psychosomatics. (2013) 54:67–73. doi: 10.1016/j.psym.2012.03.001
16. Scott, D, and Happell, B. The high prevalence of poor physical health and unhealthy lifestyle behaviours in individuals with severe mental illness. Issues Ment Health Nurs. (2011) 32:589–97. doi: 10.3109/01612840.2011.569846
17. Ahn, N, Baumeister, SE, Amann, U, Rathmann, W, Peters, A, Huth, C, et al. Visceral adiposity index (VAI), lipid accumulation product (LAP), and product of triglycerides and glucose (TyG) to discriminate prediabetes and diabetes. Sci Rep. (2019) 9:9693. doi: 10.1038/s41598-019-46187-8
18. Calderón-García, JF, Roncero-Martín, R, Rico-Martín, S, De Nicolás-Jiménez, JM, López-Espuela, F, Santano-Mogena, E, et al. Effectiveness of body roundness index (BRI) and a body shape index (ABSI) in predicting hypertension: a systematic review and Meta-analysis of observational studies. Int J Environ Res Public Health. (2021) 18:11607. doi: 10.3390/ijerph182111607
19. Ho, RC, Niti, M, Kua, EH, and Ng, TP. Body mass index, waist circumference, waist-hip ratio and depressive symptoms in Chinese elderly: a population-based study. Int J Geriatr Psychiatry. (2008) 23:401–8. doi: 10.1002/gps.1893
20. Shenoy, U, and Jagadamba,. Influence of central obesity assessed by Conicity index on lung age in young adults. J Clin Diagn Res. (2017) 11:Cc09-cc 12. doi: 10.7860/JCDR/2017/23428.9718
21. Herhaus, B, Kersting, A, Brähler, E, and Petrowski, K. Depression, anxiety and health status across different BMI classes: a representative study in Germany. J Affect Disord. (2020) 276:45–52. doi: 10.1016/j.jad.2020.07.020
22. Ma, W, Yan, Z, Wu, W, Li, D, Zheng, S, and Lyu, J. Dose-response Association of Waist-to-Height Ratio Plus BMI and risk of depression: evidence from the NHANES 05-16. Int J Gen Med. (2021) 14:1283–91. doi: 10.2147/IJGM.S304706
23. Jung, SJ, Woo, HT, Cho, S, Park, K, Jeong, S, Lee, YJ, et al. Association between body size, weight change and depression: systematic review and meta-analysis. Br J Psychiatry J Ment Sci. (2017) 211:14–21. doi: 10.1192/bjp.bp.116.186726
24. Krakauer, NY, and Krakauer, JC. A new body shape index predicts mortality hazard independently of body mass index. PLoS One. (2012) 7:e39504. doi: 10.1371/journal.pone.0039504
25. Lei, J, Luo, Y, Xie, Y, and Wang, X. Visceral adiposity index is a measure of the likelihood of developing depression among adults in the United States. Front Psychol. (2022) 13:772556. doi: 10.3389/fpsyg.2022.772556
26. Zavala, GA, Kolovos, S, Chiarotto, A, Bosmans, JE, Campos-Ponce, M, Rosado, JL, et al. Association between obesity and depressive symptoms in Mexican population. Soc Psychiatry Psychiatr Epidemiol. (2018) 53:639–46. doi: 10.1007/s00127-018-1517-y
27. Qian, J, Li, N, and Ren, X. Obesity and depressive symptoms among Chinese people aged 45 and over. Sci Rep. (2017) 7:45637. doi: 10.1038/srep45637
28. Noh, JW, Kwon, YD, Park, J, and Kim, J. Body mass index and depressive symptoms in middle aged and older adults. BMC Public Health. (2015) 15:310. doi: 10.1186/s12889-015-1663-z
29. Zhao, Y, Hu, Y, Smith, JP, Strauss, J, and Yang, G. Cohort profile: the China health and retirement longitudinal study (CHARLS). Int J Epidemiol. (2014) 43:61–8. doi: 10.1093/ije/dys203
30. Zhang, L, Li, JL, Guo, LL, Li, H, Li, D, and Xu, G. The interaction between serum uric acid and triglycerides level on blood pressure in middle-aged and elderly individuals in China: result from a large national cohort study. BMC Cardiovasc Disord. (2020) 20:174. doi: 10.1186/s12872-020-01468-3
31. Zhang, L, Li, JL, Zhang, LL, Guo, LL, Li, H, and Li, D. No association between C-reactive protein and depressive symptoms among the middle-aged and elderly in China: evidence from the China health and retirement longitudinal study. Medicine. (2018) 97:e12352. doi: 10.1097/MD.0000000000012352
32. Zhang, L, Li, JL, Zhang, LL, Guo, LL, Li, H, and Li, D. Body mass index and serum uric acid level: individual and combined effects on blood pressure in middle-aged and older individuals in China. Medicine. (2020) 99:e19418. doi: 10.1097/MD.0000000000019418
33. Zhang, L, Li, JL, Zhang, LL, Guo, LL, Li, H, Yan, W, et al. Relationship between adiposity parameters and cognition: the "fat and jolly" hypothesis in middle-aged and elderly people in China. Medicine. (2019) 98:e14747. doi: 10.1097/MD.0000000000014747
34. Zhang, L, Liu, K, Li, H, Li, D, Chen, Z, Zhang, LL, et al. Relationship between body mass index and depressive symptoms: the "fat and jolly" hypothesis for the middle-aged and elderly in China. BMC Public Health. (2016) 16:1201. doi: 10.1186/s12889-016-3864-5
35. Zhang, L, Yang, L, Wang, C, Yuan, T, Zhang, D, Wei, H, et al. Individual and combined association analysis of famine exposure and serum uric acid with hypertension in the mid-aged and older adult: a population-based cross-sectional study. BMC Cardiovasc Disord. (2021) 21:420. doi: 10.1186/s12872-021-02230-z
36. Liu, L, Chang, Y, Fu, J, Wang, J, and Wang, L. The mediating role of psychological capital on the association between occupational stress and depressive symptoms among Chinese physicians: a cross-sectional study. BMC Public Health. (2012) 12:219. doi: 10.1186/1471-2458-12-219
37. Wang, JN, Sun, W, Chi, TS, Wu, H, and Wang, L. Prevalence and associated factors of depressive symptoms among Chinese doctors: a cross-sectional survey. Int Arch Occup Environ Health. (2010) 83:905–11. doi: 10.1007/s00420-010-0508-4
38. Yu, J, Li, J, Cuijpers, P, Wu, S, and Wu, Z. Prevalence and correlates of depressive symptoms in Chinese older adults: a population-based study. Int J Geriatr Psychiatry. (2012) 27:305–12. doi: 10.1002/gps.2721
39. Zhang, J, and Norvilitis, JM. Measuring Chinese psychological well-being with Western developed instruments. J Pers Assess. (2002) 79:492–511. doi: 10.1207/S15327752JPA7903_06
40. Andresen, EM, Malmgren, JA, Carter, WB, and Patrick, DL. Screening for depression in well older adults: evaluation of a short form of the CES-D (Center for Epidemiologic Studies Depression Scale). Am J Prev Med. (1994) 10:77–84. doi: 10.1016/S0749-3797(18)30622-6
41. Zhou, BF. Effect of body mass index on all-cause mortality and incidence of cardiovascular diseases--report for meta-analysis of prospective studies open optimal cut-off points of body mass index in Chinese adults. Biomed Environ Sci. (2002) 15:245–52.
42. Rato, Q. Conicity index: an anthropometric measure to be evaluated. Revista Portuguesa de cardiologia: orgao oficial da Sociedade Portuguesa de Cardiologia = Portuguese journal of cardiology: an official journal of the Portuguese society of. Cardiology. (2017) 36:365–6. doi: 10.1016/j.repce.2017.04.006
43. Amato, MC, Giordano, C, Galia, M, Criscimanna, A, Vitabile, S, Midiri, M, et al. Visceral adiposity index: a reliable indicator of visceral fat function associated with cardiometabolic risk. Diabetes Care. (2010) 33:920–2. doi: 10.2337/dc09-1825
44. Thomas, DM, Bredlau, C, Bosy-Westphal, A, Mueller, M, Shen, W, Gallagher, D, et al. Relationships between body roundness with body fat and visceral adipose tissue emerging from a new geometrical model. Obesity. (2013) 21:2264–71. doi: 10.1002/oby.20408
45. Zhang, FL, Ren, JX, Zhang, P, Jin, H, Qu, Y, Yu, Y, et al. Strong Association of Waist Circumference (WC), body mass index (BMI), waist-to-height ratio (WHtR), and waist-to-hip ratio (WHR) with diabetes: a population-based cross-sectional study in Jilin Province, China. J Diabetes Res. (2021) 2021:1–9. doi: 10.1155/2021/8812431
46. Kahn, HS. The "lipid accumulation product" performs better than the body mass index for recognizing cardiovascular risk: a population-based comparison. BMC Cardiovasc Disord. (2005) 5:26. doi: 10.1186/1471-2261-5-26
47. Xia, MF, Chen, Y, Lin, HD, Ma, H, Li, XM, Aleteng, Q, et al. A indicator of visceral adipose dysfunction to evaluate metabolic health in adult Chinese. Sci Rep. (2016) 6:38214. doi: 10.1038/srep38214
48. Chang, HH, and Yen, ST. Association between obesity and depression: evidence from a longitudinal sample of the elderly in Taiwan. Aging Ment Health. (2012) 16:173–80. doi: 10.1080/13607863.2011.605053
49. Wirtz, PH, Ehlert, U, Emini, L, and Suter, T. Higher body mass index (BMI) is associated with reduced glucocorticoid inhibition of inflammatory cytokine production following acute psychosocial stress in men. Psychoneuroendocrinology. (2008) 33:1102–10. doi: 10.1016/j.psyneuen.2008.05.002
50. McInnis, CM, Thoma, MV, Gianferante, D, Hanlin, L, Chen, X, Breines, JG, et al. Measures of adiposity predict interleukin-6 responses to repeated psychosocial stress. Brain Behav Immun. (2014) 42:33–40. doi: 10.1016/j.bbi.2014.07.018
51. Park, HS, Park, JY, and Yu, R. Relationship of obesity and visceral adiposity with serum concentrations of CRP, TNF-alpha and IL-6. Diabetes Res Clin Pract. (2005) 69:29–35. doi: 10.1016/j.diabres.2004.11.007
52. Castanon, N, Lasselin, J, and Capuron, L. Neuropsychiatric comorbidity in obesity: role of inflammatory processes. Front Endocrinol. (2014) 5:74. doi: 10.3389/fendo.2014.00074
53. Vittengl, JR. Which body shape dimensions and sizes predict depression? J Affect Disord. (2019) 250:193–8. doi: 10.1016/j.jad.2019.03.032
54. Chatterjee, S, Peters, SA, Woodward, M, Mejia Arango, S, Batty, GD, Beckett, N, et al. Type 2 diabetes as a risk factor for dementia in women compared with men: a pooled analysis of 2.3 million people comprising more than 100, 000 cases of dementia. Diabetes Care. (2016) 39:300–7. doi: 10.2337/dc15-1588
55. Gudala, K, Bansal, D, Schifano, F, and Bhansali, A. Diabetes mellitus and risk of dementia: a meta-analysis of prospective observational studies. J Diabetes Invest. (2013) 4:640–50. doi: 10.1111/jdi.12087
56. Strachan, MW, Reynolds, RM, Frier, BM, Mitchell, RJ, and Price, JF. The relationship between type 2 diabetes and dementia. Br Med Bull. (2008) 88:131–46. doi: 10.1093/bmb/ldn042
57. Lee, JH, Park, SK, Ryoo, JH, Oh, CM, Mansur, RB, Alfonsi, JE, et al. The association between insulin resistance and depression in the Korean general population. J Affect Disord. (2017) 208:553–9. doi: 10.1016/j.jad.2016.10.027
58. Shi, YY, Zheng, R, Cai, JJ, and Qian, SZ. The association between triglyceride glucose index and depression: data from NHANES 2005-2018. BMC Psychiatry. (2021) 21:267. doi: 10.1186/s12888-021-03275-2
59. Wu, H, Li, H, Ding, Y, Jiang, J, Guo, P, Wang, C, et al. Is triglyceride associated with adult depressive symptoms? A big sample cross-sectional study from the rural areas of Central China. J Affect Disord. (2020) 273:8–15. doi: 10.1016/j.jad.2020.03.168
60. Zheng, S, Shi, S, Ren, X, Han, T, Li, Y, Chen, Y, et al. Triglyceride glucose-waist circumference, a novel and effective predictor of diabetes in first-degree relatives of type 2 diabetes patients: cross-sectional and prospective cohort study. J Transl Med. (2016) 14:260. doi: 10.1186/s12967-016-1020-8
61. Zeng, ZY, Liu, SX, Xu, H, Xu, X, Liu, XZ, and Zhao, XX. Association of triglyceride glucose index and its combination of obesity indices with prehypertension in lean individuals: a cross-sectional study of Chinese adults. J Clin Hypertens (Greenwich). (2020) 22:1025–32. doi: 10.1111/jch.13878
62. Ramdas Nayak, VK, Nayak, KR, and Vidyasagar, S. Predictive performance of traditional and novel lipid combined anthropometric indices to identify prediabetes. Diabetes Metab Syndr. (2020) 14:1265–72. doi: 10.1016/j.dsx.2020.06.045
63. Kriketos, AD, Furler, SM, Gan, SK, Poynten, AM, Chisholm, DJ, and Campbell, LV. Multiple indexes of lipid availability are independently related to whole body insulin action in healthy humans. J Clin Endocrinol Metab. (2003) 88:793–8. doi: 10.1210/jc.2002-020848
64. Carr, DB, Utzschneider, KM, Hull, RL, Kodama, K, Retzlaff, BM, Brunzell, JD, et al. Intra-abdominal fat is a major determinant of the National Cholesterol Education Program Adult Treatment Panel III criteria for the metabolic syndrome. Diabetes. (2004) 53:2087–94. doi: 10.2337/diabetes.53.8.2087
65. Anoop, SS, Dasgupta, R, Rebekah, G, Jose, A, Inbakumari, MP, Finney, G, et al. Lipid accumulation product (LAP) as a potential index to predict risk of insulin resistance in young, non-obese Asian Indian males from southern India: observations from hyperinsulinemic-euglycemic clamp studies. BMJ Open Diabetes Res Care. (2021) 9:e002414. doi: 10.1136/bmjdrc-2021-002414
66. Chen, J, Sun, H, Qiu, S, Tao, H, Yu, J, and Sun, Z. Lipid accumulation product combined with urine glucose excretion improves the efficiency of diabetes screening in Chinese adults. Front Endocrinol. (2021) 12:691849. doi: 10.3389/fendo.2021.691849
67. Lotfi, K, Hassanzadeh Keshteli, A, Saneei, P, Afshar, H, Esmaillzadeh, A, and Adibi, P. A body shape index and body roundness index in relation to anxiety, depression, and psychological distress in adults. Front Nutr. (2022) 9:843155. doi: 10.3389/fnut.2022.843155
68. Hadi, S, Momenan, M, Cheraghpour, K, Hafizi, N, Pourjavidi, N, Malekahmadi, M, et al. Abdominal volume index: a predictive measure in relationship between depression/anxiety and obesity. Afr Health Sci. (2020) 20:257–65. doi: 10.4314/ahs.v20i1.31
69. Suliga, E, Ciesla, E, Głuszek-Osuch, M, Rogula, T, Głuszek, S, and Kozieł, D. The usefulness of anthropometric indices to identify the risk of metabolic syndrome. Nutrients. (2019) 11:2598. doi: 10.3390/nu11112598
70. Liao, W, Luo, Z, Hou, Y, Cui, N, Liu, X, Huo, W, et al. Age and gender specific association between obesity and depressive symptoms: a large-scale cross-sectional study. BMC Public Health. (2020) 20:1565. doi: 10.1186/s12889-020-09664-8
71. Zhao, G, Ford, ES, Li, C, Tsai, J, Dhingra, S, and Balluz, LS. Waist circumference, abdominal obesity, and depression among overweight and obese U.S. adults: National Health and nutrition examination survey 2005-2006. BMC Psychiatry. (2011) 11:130. doi: 10.1186/1471-244x-11-130
72. Schneider, HJ, Friedrich, N, Klotsche, J, Pieper, L, Nauck, M, John, U, et al. The predictive value of different measures of obesity for incident cardiovascular events and mortality. J Clin Endocrinol Metab. (2010) 95:1777–85. doi: 10.1210/jc.2009-1584
73. Zhi, T, Wang, Q, Liu, Z, Zhu, Y, Wang, Y, Shi, R, et al. Body mass index, waist circumference and waist-hip ratio are associated with depressive symptoms in older Chinese women: results from the Rugao longevity and ageing study (RuLAS). Aging Ment Health. (2017) 21:518–23. doi: 10.1080/13607863.2015.1124837
74. Numan Ahmad, M, and Halim, HF. Suitability of visceral adiposity index as a marker for CARDIOMETABOLIC risks in JORDANIAN adults. Nutr Hosp. (2015) 32:2701–9. doi: 10.3305/nh.2015.32.6.9543
75. Amato, MC, Giordano, C, Pitrone, M, and Galluzzo, A. Cut-off points of the visceral adiposity index (VAI) identifying a visceral adipose dysfunction associated with cardiometabolic risk in a Caucasian Sicilian population. Lipids Health Dis. (2011) 10:183. doi: 10.1186/1476-511X-10-183
76. Almeida, OP, Hankey, GJ, Yeap, BB, Golledge, J, and Flicker, L. Depression as a modifiable factor to decrease the risk of dementia. Transl Psychiatry. (2017) 7:e1117. doi: 10.1038/tp.2017.90
77. Geerlings, MI, den Heijer, T, Koudstaal, PJ, Hofman, A, and Breteler, MM. History of depression, depressive symptoms, and medial temporal lobe atrophy and the risk of Alzheimer disease. Neurology. (2008) 70:1258–64. doi: 10.1212/01.wnl.0000308937.30473.d1
78. Confortin, SC, Meneghini, V, Ono, LM, Garcia, KC, Schneider, IJC, d'Orsi, E, et al. Anthropometric indicators associated with dementia in the elderly from Florianópolis-SC, Brazil: epi Floripa ageing study. Cienc Saude Colet. (2019) 24:2317–24. doi: 10.1590/1413-81232018246.20492017
Keywords: depressive symptom, cross-sectional study, cardiometabolic indicators, middle-aged and older adults, obesity
Citation: Wang Y, Zhang X, Li Y, Gui J, Mei Y, Yang X, Liu H, Guo L, Li J, Lei Y, Li X, Sun L, Yang L, Yuan T, Wang C, Zhang D, Li J, Liu M, Hua Y and Zhang L (2023) Predicting depressive symptom by cardiometabolic indicators in mid-aged and older adults in China: a population-based cross-sectional study. Front. Psychiatry 14:1153316. doi: 10.3389/fpsyt.2023.1153316
Edited by:
Wei Liang, Shenzhen University, ChinaReviewed by:
Lin Zhou, Hebei Normal University, ChinaJiao Jiao, Hong Kong Baptist University, Hong Kong SAR, China
Copyright © 2023 Wang, Zhang, Li, Gui, Mei, Yang, Liu, Guo, Li, Lei, Li, Sun, Yang, Yuan, Wang, Zhang, Li, Liu, Hua and Zhang. This is an open-access article distributed under the terms of the Creative Commons Attribution License (CC BY). The use, distribution or reproduction in other forums is permitted, provided the original author(s) and the copyright owner(s) are credited and that the original publication in this journal is cited, in accordance with accepted academic practice. No use, distribution or reproduction is permitted which does not comply with these terms.
*Correspondence: Lin Zhang, yaoran2008@163.com