- 1Department of Psychiatry, Universidad Autonoma de Madrid, Madrid, Spain
- 2Department of Psychiatry, Instituto de Investigación Sanitaria Princesa (IIS Princesa), Madrid, Spain
- 3Center for Biomedical Research in Mental Health (CIBERSAM), Carlos III Health Institute, Madrid, Spain
- 4Department of Legal Medicine, Psychiatry and Pathology, Universidad Complutense de Madrid, Madrid, Spain
- 5School of Health Sciences and Education, Harokopio University, Athens, Greece
- 6First Cardiology Clinic, School of Medicine, University of Athens, Athens, Greece
Background: In recent decades, the relationship between emotional disorders (i.e., depression and anxiety) and alterations in physiological functions (i.e., inflammation or metabolism) have been well supported. However, studies on a symptom-based approach have provided mixed results. Our study aims to gain insight into how subclinical statuses, featured by elevated depressive and/or anxious symptoms, may influence immunometabolic alterations in the concurrent relationship; and the development of metabolic diseases at 10-year follow-up: diabetes, hypertension and hypercholesterolemia.
Methods: Data from 758 Greek adults [394 men (aged 41 ± 10 years) and 364 women (aged 37 ± 12 years)] were used. Four groups were created according to the levels of depressive and anxiety symptoms: (1) control group (CG), (2) depressive group (DG), (3) anxiety group (AG) and (4) depressive and anxiety group (DAG). Multi-indicator multi-causes (MIMIC) modeling was used to estimate metabolic function and inflammatory response scores, on a wide selection of blood biomarkers. Finally, a binary logistic regression was carried out to study the influence of symptoms on the development of the aforementioned metabolic diseases on a 10-year follow-up.
Results: Group membership was not associated with metabolic function score. Conversely, DAG membership was related with higher inflammatory response score (B = 0.20, CI95 = 0.01, 0.40), with respect to the CG (p < 0.05). Both age and sex were significant variables in the calculation of both scores. Regarding disease at 10-year follow-up effect, risk of developing diabetes, hypertension and hypercholesterolemia was associated with age and socioeconomic status. Moreover, DG membership was significant for diabetes risk (OR = 2.08, CI95 = 1.00, 4.22) and DAG for hypercholesterolemia (OR = 1.68, CI95 = 1.16, 2.43).
Limitations: Data on anti-inflammatory drugs and psychopharmacological medication were not collected in this study.
Conclusions: Elevated symptoms of depression and anxiety accounts for inflammatory alterations at concurrent relationship and a higher risk of 10-year follow-up metabolic diseases.
1 Introduction
Emotional disorders (i.e., depression and anxiety) are considered among the top five mental conditions with higher impact, worldwide. In 2015, it is estimated that 4.4% of the world population suffered from a depressive disorder and 3.6% from an anxiety disorder (1). Moreover, comorbidity is quite common between both conditions, observing shared pathophysiological mechanisms and risk factors (2–4). Importantly, emotional disorders may account for large proportion of years of life lived with disability across the lifespan (5, 6).
Emotional disorders may have a critical impact for patients at the individual level, as well as for healthcare provision systems at the socioeconomic level. Further research should be done to improve the understanding of pathophysiological mechanisms to contribute for treatment optimization. In last decades, mounting evidence has stressed the existing relationship between depression and inflammation (7–10). In this line, some depressive endophenotypes (i.e., atypical depression) have been related to evident disturbances in the (pro-)inflammatory response (11–13). Less is known on the relationship between anxiety and inflammation (14). However, existing studies also point to an elevated pro-inflammatory response in patients with an anxiety disorder (10, 15). This inflammation could lead to the occurrence of metabolic diseases comorbid with depression and anxiety, as it seems to have a mediating role in both pathologies, for example, patients with depression are at higher risk of high blood pressure and patients with type 2 were 1.2–2.3 times more likely to have depressive symptoms than the general population (16). The relationship between the two (i.e., emotional disorders and metabolic diseases) seems to be mediated by inflammation. For instance, some evidence stressed a mediating role of NLRP3 inflammatory bodies in hippocampal neuroinflammation and depression-like behavior (16, 17). Thus, the metabolic alteration, would be preceded by an altered inflammatory status.
Unfortunately, studies on comorbid anxiety and depression are scarce. It would be expected to find wider inflammatory alterations in patients with comorbid emotional disorders for several reasons. First, patients with inflammatory diseases have an increased risk of developing both anxiety and depressive disorders (18, 19). Second, both emotional disorders may share some common altered mechanisms. A dysregulation of the hypothalamic pituitary adrenal axis (HPA) is observed in both disorders (20–22), which becomes more evident when they occur together (23).
Dysregulation of the HPA axis, seems to play a role in the immunometabolic alterations observed in patients, such as increased production of cytokines [i.e., Tumor necrosis factor (TNF-α) or interleukin 6 (IL- 6)] (24) and consequent induction of acute phase inflammatory proteins [i.e., and C-reactive protein (CRP) or serum amyloid A (SAA)] (25) and metabolic dysregulation [i.e., stimulation of the release of lipids into the bloodstream, increased triglycerides and decreased cholesterol linked to high-density lipoproteins (HDL-C) or alterations in total cholesterol levels] (26). Moreover, the dysregulation of cortisol secretion may induce alterations in lipid and glucose metabolism that can contribute to metabolic disease development, such as diabetes, hypercholesterolemia or hypertriglyceridemia (27). Evidence is less consistent on the development of cardiovascular conditions and it seems that other mediating pathways (i.e., kynurenine path) may be involved (28).
Although most of the available studies are cross-sectional (8, 9, 14), the longitudinal studies carried out to date seem to relate the presence of emotional disorders with immunometabolic alterations in the short and long term (23, 29–35). Moreover, short-term alterations in the immunometabolic response have also been observed among individuals with subclinical emotional conditions (36–39). Unfortunately, longitudinal studies are scarce on the relationship between subclinical (symptom-based) emotional conditions and metabolic disease development.
The study of subclinical conditions become crucial for prevention to tackle the development of full-blown conditions and mitigate its impact over time. This study aimed to analyze the relationship between subclinical conditions featured by elevated emotional symptoms (i.e., depressive and/or anxious symptoms) and immunometabolic dysregulation at concurrent relationship (diverted levels of immunometabolic markers in plasma) and disease at 10-year follow-up. We hypothesize that participants with elevated levels of both depressive and anxious symptoms would show altered immunometabolic profiles in comparison with people without elevated symptoms. Moreover, it is hypothesized that the presence of comorbid depression-anxiety symptoms would contribute to the development of chronic metabolic diseases (particularly diabetes and hypercholesterolemia) over a 10-year follow-up.
2 Materials and methods
2.1 Study sample
Data from the 10-year follow-up of the ATTICA study (40) were used to satisfy the study aims. The ATTICA study is a population-based cohort focused on examining social, demographic, lifestyle, clinical and biological characteristics of apparently healthy Greek adults living in the greater metropolitan area of Athens (Greece), on cardiovascular disease incidence, and other health-related conditions. In brief, a baseline survey was carried out during 2001–2002 and a sample of 4056 adults was invited to participate (78% from urban area). This survey relied on a random, multi-stage cluster sampling (considering age and sex distribution of the Attica region in 2001). A total of 3042 individuals (18–89 years old, 49% men) agreed to participate (75% response rate). A follow-up survey was conducted during 2011–2012. Most of participants were enrolled the follow-up survey (n = 2583; 85% response rate).
A randomly selected subsample of the ATTICA cohort completed questionnaires on emotional symptoms. Concretely, a sample of 758 adults was used [394 men (aged 41 ± 10 years) and 364 women (aged 37 ± 12 years)]. Further details on how the subsample was reached and randomization algorithm are provided elsewhere (41). The final sample comprised 615 participants (50.98% women; m = 39.20 years, sd = 10.96). Participants were dropped out because: being older than 65 years at baseline (n = 3), blood sample not available (n = 137) or death at follow-up (n = 3). Significant differences were found between the random subsample initially selected and final sample in analysis, only in terms of family composition at baseline [χ2 (2) = 8.99, p < 0.05, Cramer’s V = 0.10], with higher proportion of married individuals in the final sample (62.74%) than the one from the drop-out sample (51.75%); and in terms of depressive symptom levels [t (204.66) = 2.09, p < 0.05, Cohen’s d = 0.2], with lower symptoms in the subsample in analysis (m = 35.12, sd = 7.35) in comparison to the drop-out one (m = 36.62, sd = 7.82).
2.2 Demographic, clinical and lifestyle characteristics
Baseline survey included questions about demographic features (i.e., gender, age, marital status, education level and financial status), anthropometric measures [i.e., height, weight, and body mass index (BMI)], history of medical conditions as well as lifestyle habits (i.e., dietary assessment, alcohol consumption, tobacco use and physical activity). The assessment protocol has already been described elsewhere (42).
Depressive symptoms were assessed using the validated Greek translation of the Zung’s Self-Rating Depression Scale (ZDRS) (43, 44). ZDRS consists of 20 items covering affective, psychological, and somatic symptoms. To perform the assessment, the patient indicates how often they experience a particular symptom (i.e., 1 = some of the time, 2 = some of the time, 3 = a good part of the time, or 4 = most of the time). The ZDRS total score range is 20–80; with higher values indicating more severe depression symptoms.
Anxiety symptoms were assessed using the validated Greek translation of the Spielberger State Anxiety Inventory (STAI), which is a 20-item self-administered questionnaire (45). The 20 items are scored from 1 to 4 in terms of frequency categories with respect to (never, sometimes, many times, always) and the total scores are obtained by adding the values assigned to each response (46). The total score of the 20-item STAI ranges from 20 to 80 with higher score values being indicative of more severe anxiety symptoms (47).
2.3 Biochemical measurements
All participants were summoned on after 12 h of fasting, to carry out the blood test from 8 to 10 a.m. All the blood samples were collected under the same procedure (in a sitting position and were collected from the antecubital vein) and were carried out in the same laboratory that followed the criteria of the World Health Organization Lipid Reference Laboratories.
2.3.1 Metabolic measures
The metabolic indicators were selected according to the National Cholesterol Education Program’s Adult Treatment Panel III (revised) report guidelines for metabolic syndrome (MetS) (48). Triglycerides, high density lipoprotein (HDL)-C and blood sugar levels were quantified to know the metabolic state of participants. These biochemical examinations were measured using chromatographic enzymic method in a Technico Automatic Analyzer RA-1000 (Dade Behring Marburg, Germany). In addition, waist circumference, as well as systolic and diastolic blood pressure were measured to determine the metabolic risk factors.
For assessing the validity of the methods details may found elsewhere (41).
2.3.2 Inflammatory measures
The blood samples for the inflammatory biomarkers were taken under the same procedure, at the same time and place as the samples collected for the analysis of metabolic markers. C-Reactive Protein (CRP) and serum amyloid – A (SAA) were assayed by particle-enhanced immunonephelometry (N Latex, Dade-Behring Marburg GmbH, Marburg, Germany) (49). Interleukin-6 (IL-6) levels were quantified with high sensitivity enzyme linked immunoassay (R&D System Europe Ltd., Abingdon, UK) and tumor necrosis factor (TNF)-α was measured with ELISA method (Quantikine HS/human TNF-α immunoassay kit, R&D Systems, Inc., Minneapolis, USA) (49).
2.4 Follow-up examination
The ATTICA Study’s investigators performed the 10-year follow-up (median follow-up time 8.41) [see in Georgousopoulou et al. (40)]. During follow-up, the presence or absence of diabetes, hypercholesterolemia and hypertension was determined as follows.
Regarding metabolic diseases, some standard criteria were adopted. First, diabetes diagnosis was determined by fasting blood glucose levels greater than 125 mg/dL or the use of antidiabetic medication. Hypercholesterolemia was defined as total serum cholesterol levels greater than 200 mg/dL or the use of lipid-lowering agents. The presence of hypertension was determined by values greater than or equal to 140/90 mmHg or by being under hypertensive medication. Blood pressure was measured with the participant sitting and resting for at least 30 min, the specialist doctor performed three measurements on the right arm, in a 45° position and leaning on the table with the aneroid manometric sphygmomanometer (ELKA, Von Schlieben Co., Munich, Germany). The level of systolic blood pressure was determined by the first perception of sound and the diastolic was determined by phase V when the repetitive sounds disappear completely [for more information on how the samples were collected, see (41)].
2.5 Data analysis
Multi-indicator multi-causes (MIMIC) modeling was used to estimate both a metabolic risk score and an inflammatory response score from the biomarkers. MIMIC modeling constitutes a Structural Equation Modeling (SEM) extension to study nested relationships, simultaneously allowing for identifying underlying (latent) factors that are measured by multiple indicators and controlling for other confounding effects (50). Thus, the metabolic risk score was estimated by means of blood (triglycerides, HDL-C, blood sugar, all of them in loglinear scale), cardiovascular (arterial blood pressure) and anthropometric (waist diameter) indicators. Likewise, the inflammatory response score was estimated by using blood indicators (CRP, SAA, IL-6 and TNF-α). Score estimation was conducted controlling for relevant lifestyle covariates (Mediterranean diet adherence, physical activity, smoking and alcohol use). The diagonally weighted least squares (DWLS) methods were used for model estimation, as some binary (e.g., hypertension diagnosis, smoking, alcohol use) and categorical (physical exercise) were included in our analysis. Standard error estimation was based on bootstrapping methods with 1000 samples, that ensures reliable estimates are derived. Fit indexes used to assess goodness-of-fit of MIMIC models were the χ2 statistic, the root mean square error of approximation index (RMSEA), the comparative fit index (CFI), the Tucker-Lewis index (TLI), and the standardized root mean square residual (SRMR). According to Hu and Bentler (51), adequate model fit is indicated by values of RMSEA < 0.08, CFI ≥ 0.95, TLI ≥ 0.95, and SRMR < 0.08.
For the purposes of our study, sample was categorized into four groups regarding levels of depressive and anxiety symptoms: (1) control group (CG), featured by low levels of depressive and anxiety symptoms; (2) depressive group (DG), comprising individuals with depressive symptom levels overpassing the third quartile of distribution cut-off point and low levels of anxiety symptoms; (3) anxiety group (AG), whose members showed low depressive symptoms but anxiety symptoms overpassing the third quartile of distribution cut-off point; and (4) depressive and anxiety group (DAG), whose members showed elevated levels of both anxiety and depressive symptoms.
Relationship between the metabolic risk and inflammatory scores derived from the MIMIC models (i.e., predicted scores) and study group membership was studied using linear regression. Sex and age were used as covariates. The adjusted R2 was used as an effect size estimate. Beta coefficients and their CI95 was used to explore loading magnitude.
To predict the development of metabolic diseases (i.e., diabetes, hypertension and hypercholesterolemia) over the 10-year follow-up, logistic binary regression was used. Participants with suspected baseline diabetes (glucose level > 126 mg/dL; n = 15), hypertension (systolic blood pressure ≥ 140 or diastolic blood pressure ≥ 90; n = 172) or hypercholesterolaemia (total cholesterol level > 240; n = 57) were removed from analysis for the 10-year outcome. Taking into account the metabolic risk scores at baseline allows to control the levels of markers associated with metabolic alterations, as an additional adjustment following the exclusion of participants with suspected baseline disease for each outcome of interest.
Invariant (sex), and baseline covariates (age, financial status, emotional group and metabolic score) were considered. The baseline inflammatory response score was considered as a weighting factor for the within-subject heteroscedasticity structure, due to the potential influence on the development of both metabolic conditions and emotional disorders.
The Akaike information criterion (AIC) was used to compare the fit of an unconstrained model (model without covariates), a model with sociodemographic covariates (age and financial status) and a model with all the covariates (full model: age, financial status, emotional group and metabolic score). A better fit was proven by a lower AIC of the full model in comparison to the unconstrained one. In addition, the area under the receiver operating curve (AUC) was used as a classification accuracy estimate. AUC > 0.70 indicates adequate accurate in classification. The odds ratio (OR) estimate was used to explore loading magnitude.
All analyses were performed using the R software × 64 3.0.1 (l cmm, ROCR, psych and lmerTest packages).
3 Results
The descriptive statistics are displayed in Table 1, as well as group comparison according to their scores in depressive and anxious symptom scales: CG (61.32% of sample), DG (13.24), AG (11.52%) and DAG (13.90%) (see Table 1).
Regarding the sociodemographic variables, we observed significant differences between the study groups. There was a higher percentage of men with anxiety (62.07%) compared to those in the DG and DAG groups, in which there was a higher percentage of women (68 and 65.71%, respectively). Significant differences were also observed in the variable of years of schooling (F = 29.17; p < 0.01; η2partial = 0.04) and in marital status (χ2 = 17.04; p < 0.01; Cramer’s V = 0.11). The CG had more years of schooling (m = 13.51; sd = 3.27) than the rest of the participants, being participants from the DAG those with the lowest number of years of schooling (m = 11.77; sd = 3.54).
Regarding the emotional symptoms, between-group differences were evident for both the depression (F = 531.05; p < 0.01; η2partial = 0.41) and the anxiety symptoms (F = 1095.28; p < 0.01; η2partial = 0.59). In terms of lifestyle factors, there were significant differences, both in physical exercise and in current alcohol consumption. The DG participants were those who carried out the least level of physical activity compared to the rest of the groups and those who had the least current alcohol consumption.
Finally, data on immunometabolic biomarkers are displayed in Table 1. Significant differences were obtained between the study groups only in CRP levels. The group that had the highest levels of this inflammatory protein was the AG (m = 2.32; sd = 2.9), followed by DAG (m = 2.28; sd = 3.03), CG (m = 1.74; sd = 2.21) and DG (m = 1.73; sd = 2.57). No significant differences were observed between the groups analyzed for metabolic diseases at follow-up.
3.1 Metabolic and inflammatory profiles
The MIMIC model on the inflammatory score was significant and showed adequate fit indexes (χ2 = 36.54, df = 14, p < 0.01; RMSEA = 0.046, CFI = 0.953, TLI = 0.975, SRMR = 0.021). Parameters from the MIMIC model are included in the Supplementary Table 1, as well as correlations between the calculated inflammation score and the inflammatory biomarkers (Supplementary Figure 1). Higher inflammation scores were indicative of elevated inflammation.
In terms of group comparison on the inflammatory score using linear regression, a significant relationship was found between the inflammatory score and the study groups, considering sex and age as covariates (F5,749 = 37.24, p < 0.001, R2adj = 0.19). Inflammation scores according to the study groups are displayed in the Figure 1. Specifically, the DAG group showed a significantly different loading than the CG group (B = 0.20, t = 2.03, p < 0.05). Sex (being a woman) (B = 0.20, t = 2.96, p < 0.01) and age were also significant (B = 0.04, t = 12.24, p < 0.001). Regression coefficients are displayed in Table 2.
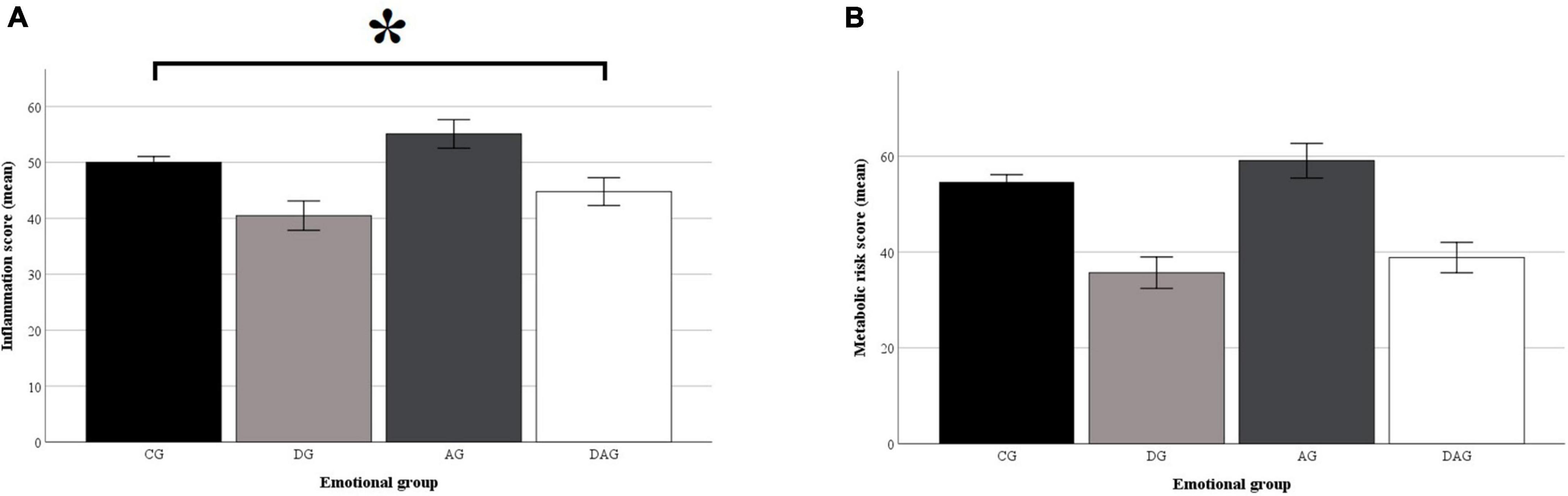
Figure 1. Inflammatory and metabolic risk scores according to the study groups. The inflammatory (A) and metabolic risk (B) scores were calculated using Multiple Indicators Multiple Causes (MIMIC) models. The scores were scaled on a 0–100 scale. For both scores, the higher the score the lower the inflammation/metabolic risk. Both scores were calculated controlling for lifestyle factors (i.e., Mediterranean diet adherence, physical activity and smoking), age and sex at birth. The indicators used for inflammatory score calculation were the C-reactive protein, Interleukin 6, Serum amyloid A, and Tumor necrosis factor α. Indicators used for metabolic risk calculation were waist circumference, blood glucose level, triglycerides, high-density lipoprotein level and hypertension diagnosis. CG, control group; DG, group with elevated depressive symptoms; AG, group with elevated anxiety symptoms; DAG, group with elevated depressive and anxiety symptoms. Whiskers represent the 95% confidence interval of the mean. *p < 0.05.
The MIMIC model to estimate the metabolic risk score also showed adequate fit indexes (χ2 = 61.30, df = 21, p < 0.001; RMSEA = 0.050, CFI = 0.961, TLI = 0.982, SRMR = 0.062). Parameters from the metabolic model are included in the Supplementary Table 2, as well as correlations between the calculated metabolic score and the metabolic biomarkers (Supplementary Figure 1). The higher the metabolic risk score, the higher the risk of metabolic dysregulation.
Regarding the relationship between the metabolic risk profile scores and the study group and covariates, the linear regression (F5,749 = 261.2, p < 0.001, R2adj = 0.63) revealed the significant relationship of the score with sex (being a woman) (being a woman) (B = 19.26, t = 31.12, p < 0.01) and age (B = 0.31, t = 11.11, p < 0.01). No significant relationships were found in terms of study groups (see Table 2 and Figure 1).
3.2 Metabolic diseases 10 years later
The model that showed a better fit for the disease at 10-year follow-up prediction of diabetes was the model that included all covariates (full model) (AIC = 406.05), compared to the model without covariates (AIC = 464.73) and the model that included the sociodemographic variables (AIC = 414.95). This model also showed adequate precision in the classification (AUC = 0.81).
The factors significantly related to the development of diabetes (sample in analysis, n = 600) were age (OR = 1.09, Wald’s Z = 5.01, p < 0.01) the economic status of the second (OR = 0.64, Wald’s Z = −1.99, p < 0.05) third (OR = 0.35, Wald’s Z = 3.25, p < 0.01) and fourth quartile (OR = 0.37, Wald’s Z = −3.34, p < 0.01), with respect to the participants of the first quartile; DG membership (OR = 2.08, Wald’s Z = 2.19, p < 0.05) and the metabolic risk score (OR = 1.05, Wald’s Z = 3.06, p < 0.01) (see Table 3).
The full model to predict hypertension development (sample in analysis, n = 443) showed a better fit to data (AIC = 647.59), in comparison to the unconstrained model (AIC = 684.39) and the model that included sociodemographic variables (AIC = 658.81). The precision of the full model was considered adequate (AUC = 0.70).
The factors significantly related to the development of hypertension over the follow-up were sex (OR = 0.06, Wald’s Z = −2.21, p < 0.05), age (OR = 1.04, Wald’s Z = 4.34, p < 0.01) and metabolic risk score (OR = 1.26, Wald’s Z = 3.34, p < 0.01) (see Table 3).
The model without covariates (AIC = 1675.01) and the one that included the sociodemographic variables (AIC = 1536.10) showed a worse fit than the full model for hypercholesterolemia (sample in analysis, n = 558) (AIC = 1525.33). The full model also showed an adequate precision to predict hypercholesterolemia development (AUC = 0.73).
In Table 3, it can be seen that the variables that were significantly related to the development of hypercholesterolemia in the 10-year follow-up were age (OR = 1.46, Wald’s Z = 2.09, p < 0.05), second (OR = 1.69, Wald’s Z = 2.51, p < 0.05), third (OR = 1.74, Wald’s Z = 2.35, p < 0.05) and fourth financial status quartile membership (OR = 1.06, Wald’s Z = 7.68, p < 0.01); DAG membership (OR = 1.68, Wald’s Z = 2.55, p < 0.01) and metabolic risk score (OR = 1.03, Wald’s Z = 2.90, p < 0.01).
4 Discussion
This study aimed to gain insight into the relationships between subclinical profiles, featured by elevated emotional symptoms (i.e., depression and anxiety symptoms), and immunometabolic alterations. Our study involved a concurrent relationship (i.e., blood inflammatory response and metabolic biomarkers) and disease at 10-year follow-up (i.e., development of chronic metabolic diseases) approach. Individuals with symptoms of anxiety and with symptoms of anxiety and depression had higher CRP levels relative to the control group. However, no differences were observed between the groups (i.e., CG, DG, AG and DAG) in the comparison of the other inflammatory markers (i.e., IL-6, SAA and TNF-α) and metabolic markers (i.e., waist circumference, fasting glucose, triglycerides, HDL cholesterol, SBP and DBP). On the other hand, the DAG group showed higher inflammatory score and was the only group with significant differences with respect to CG. No differences were observed in the metabolic risk profiles between the different groups. At 10-year follow-up, individuals with elevated depressive symptoms had an increased risk of developing diabetes and hypercholesterolemia (in this case when comorbid anxiety systems were present) during the 10-year follow-up.
Our study provided some evidence on the influence of emotional disorders on inflammatory and metabolic function, even from subclinical statuses (i.e., statuses of elevated symptoms, regardless of other criteria to be fulfilled, such as daily interference or functional impairment). The association between inflammation and depression has been supported by studies with clinical samples (15, 25, 52–56). In fact, some emotional symptoms (i.e., anhedonia, hypervigilance, insomnia) may be conceptualized as defensive reactions against (psychological, social) pathogens, leading to increased inflammatory response (57). On the other hand, our study revealed that subclinical profiles of emotional symptoms were not associated with metabolic risk at baseline. Numerous studies have shown significant relationships between metabolic risk and emotional disorders (58, 59). The relationship between emotional disorders and metabolic risk becomes stronger in the older age, due to the effect of the progressive increase of low-grade systemic inflammation with age (60). We speculate that the statuses of full-blown disorders, qualitatively distinct from normal expression variations in both degree and kind, are needed to mobilize alterations in lipid metabolism. In the studies by van Reedt Dortland et al. (61) and Vogelzangs et al. (62), they followed this line because they found no associations with the dichotomous classification by emotional symptoms, only reporting this association in more severe patients.
Different studies support the association between major depressive disorder and the presence of MetS (12, 32, 59, 63, 64). In addition, depression has been determined as a risk factor for the development of metabolic abnormalities such as obesity and adverse patterns of lipoproteins (12). Regarding the relationship between anxiety and metabolic alterations, it has been less studied (61, 62, 65–68), not always finding positive results due to the heterogeneity of the samples and methodologies used (66–68).
Risky lifestyles, as well as the consumption of psychotropic drugs, have some relevance in the association between MetS and emotional disorders because they can alter metabolic patterns (32, 61). In addition, depression and anxiety are stress-related disorders, by which systemic cortisol action occurs along with alterations in glucocorticoid sensitivity and HPA axis action (32, 64). Derived from the deregulation of the HPA axis, the body is favored to accumulate visceral adipose tissue, an active endocrine organ that produces cytokines and inflammatory hormones (32). The greater proinflammatory response activates the release of lipids into the bloodstream, producing a reduction in HDL cholesterol together with an increase in triglycerides (64). This fact, together with the greater production of oxidative stress and dysregulation of the autonomic nervous system derived from the stressed state of the patients, interacts with the glucose homeostasis and insulin resistance, related to the factors that make up MetS (64). All these alterations produced in the organism of people with emotional disorders are those found after the association with MetS.
Patients with depression or anxiety often adopt unhealthy lifestyle habits, such as smoking (69, 70), increased intake of foods high in fat or sugar (71) or sedentary lifestyle (72, 73) that also have a determinant effect on their health status (74). In the sample of our work, we observed significant differences in physical exercise and alcohol intake. On the one hand, the DG group was the one with the highest percentage of individuals who performed little physical activity. Previous studies have shown that patients with depression perform little physical activity, with 88% of them not complying with the recommended guidelines (at least 150 min/week of moderate-intensity aerobic physical activity or 150 min/week of vigorous physical activity) (75, 76). On the other hand, it has been observed that low levels of physical activity are associated with increased risk of depression in the general population (77). In fact, people who exercise regularly show almost 45% less likelihood of having depressive symptoms, being able to be used for the prevention of this disease (78, 79).
Regarding alcohol intake, we observed that the CG subjects were more likely to consume alcohol than the other groups. In contrast, the DG group was the least likely to consume alcohol. Not consuming alcohol was associated with the diagnosis of depression in previous studies (80), and this lower alcohol consumption could be associated with the need for psychopharmacological treatment.
Statuses with comorbid elevated anxiety and depression symptoms may put individuals at higher risk of inflammatory dysregulation due to a greater impact on HPA axis function and subsequent increase of glucocorticoid resistance. In this line, Choi et al. (23) found greater HPA alterations in patients with anxious depression compared to non-anxious depression. The study by Gaspersz et al. (31) revealed an overproduction of cytokines (stimulated by increased lipopolysaccharide response) in patients with comorbid anxiety and depression. In a same vein, Shim et al. (81) observed higher levels of monocytes in patients with major depression and moderate to severe anxiety symptoms compared to the mild-anxiety symptom group. Finally, a reduced number of basophils and elevated fragmented neutrophils have been found in patients with depression who showed higher anxiety symptoms (82). Altogether, these results stress that the statuses featured by higher levels of both anxiety and depression symptoms may boost alterations in the inflammatory response at concurrent relationship.
Regarding the disease at 10-year follow-up effects of subclinical emotional statuses, our results go in line with previous studies on the relationship between the emotional disorders and metabolic disease development (83, 84). More concretely, we found that the status of elevated depression symptoms put individuals at higher risk of diabetes development over the 10-year follow-up. The status of elevated anxiety and depression symptoms was associated with hypercholesterolemia development.
The risk factors for diabetes development were age (i.e., higher age with higher risk), economic status (poorer quartiles), the status of elevated depression symptoms, and the metabolic risk at baseline. Diabetes is a serious health problem, which may contribute to the development of cardiovascular complications, stroke and subsequent early mortality (85). The total prevalence of diabetes increases significantly in relation to age, reaching figures between 10–15% in the population older than 65 years and up to 20% if we consider only those older than 80 years (86).
Mounting evidence supports the elevated comorbidity between diabetes and major depression (87–91). Our study provides further insight into this relationship, supporting a clear relationship between the status of elevated depression symptoms and diabetes. Despite this, the presence of elevated emotional symptoms of depression and anxiety together was not a risk factor. This could be due to the differences found between both groups in performing physical activity. The DAG group performs a greater amount of moderate and intense physical activity, which could be a protective factor for the development of diabetes. Physical exercise is inversely related to different risk factors for the development of diabetes (92, 93). On the one hand, it improves energy balance and reduces adiposity and, in addition to this, it improves insulin sensitivity and glucose homeostasis, which helps improve the metabolic profile of people who do it and reduce the risk of diabetes (92).
On the other hand, the risk factors for the disease at 10-year follow-up development of hypertension were sex, age and metabolic risk. The statuses of elevated emotional symptoms were not associated with hypertension development. Despite some studies have provided some evidence on the relationship between emotional disorders (mainly depression) and hypertension (94–96), other results have shown opposite findings (97–99), being important to consider confounding factors such as lifestyle or metabolic status of the participants (100). Moreover, some cardiovascular mediating paths (i.e., kynurenine path) may be more independent of emotional factors (28).
Finally, the status of elevated anxiety and depression symptoms was proven to be a risk factor of hypercholesterolemia development. Additional risk factors of hypercholesterolemia development were age, economic status (poorer quartiles), and the metabolic risk at baseline.
Depression has been related with altered lipid metabolism (101), even from a first clinical episode (102). Despite the fact that many studies support this fact (35, 103, 104), the results are contradictory with other studies that have found an inverse relationship between cholesterol and depression (105, 106). These discrepancies may be explained by methodological issues (i.e., different sample selection criteria and assessment protocols).
The longitudinal study by van Reedt Dortland et al. (35) stressed that patients with severe anxiety and depression symptoms were at higher risk of presenting dyslipidemia on a 2-year follow-up. Our results extend the conclusions from the study by van Reedt Dortland et al. (35), by including a longer follow-up and individuals with subclinical statuses of elevated symptoms. We speculate that the individuals with statuses of elevated emotional may show an overproduction of HPA agents and higher glucocorticoid resistance. HPA dysregulation may lead to increased levels of circulating free fatty acids, with subsequent low-density lipoprotein secretion and alterations in lipid metabolism (101).
Our study presents some relevant strengths to be mentioned. Compared to previous studies, our study has a large sample of community people. Moreover, our analytical strategy based on robust protocols (e.g., MIMIC models) controlling for relevant covariates, such as, lifestyle factors and health status. Finally, our study focuses on profiles on symptoms, providing new insight into the development of preventive strategies to prevent from full-blown condition development.
Our study presents some shortcomings to be mentioned. The intake of oral hypoglycemic, antihypertensive or lipid-lowering drugs was not taken into account in the baseline evaluation. Data on anti-inflammatory drugs and psychopharmacological medication were also not collected in this study. In this regard, we adopted a symptom-based approach, highly appropriate on a community basis. However, this study should be seen as a wide picture of how subclinical statuses of emotional disorder may be linked with immunometabolic dysregulation and metabolic diseases. On the other hand, it should be noted that only a baseline assessment of the participants’ mental health was carried out. Anxiety and depressive symptoms were not followed up, so the trajectories of the participants’ symptoms could not be known. Longitudinal studies are needed to explore how different trajectories of anxiety and depression symptoms influence the subsequent development of metabolic diseases.
5 Conclusion
Depression and anxiety are two of the most prevalent (1) and disabling (5, 6) mental disorders that carry high socioeconomic costs. Therefore, finding the causes of both disorders to reduce or eliminate symptoms is essential in mental health research. With our study, we have been able to demonstrate that both pathologies have concurrent relationship and disease at 10-year follow-up consequences on the health of individuals. We have determined that subjects with comorbid subclinical symptoms of depression and anxiety have concurrent relationship immune system consequences. In addition, these patients have a higher risk of long-term hypercholesterolemia and patients with depression have a higher risk of diabetes. The results therefore suggest the need to follow these patients and propose early healthy lifestyle interventions that can offset this risk by reducing their metabolic risk and thereby reducing the risk of morbidity.
Data availability statement
The raw data supporting the conclusions of this article will be made available by the authors, without undue reservation.
Ethics statement
The ATTICA study was approved by the Institutional Ethics Committee of Athens Medical School (#017/1.5.2001). The studies were conducted in accordance with the local legislation and institutional requirements. The participants provided their written informed consent to participate in this study. Written informed consent was not obtained from the individual(s) for the publication of any potentially identifiable images or data included in this article because protocols in the current study did not need a specific approval from an Ethics Committee as secondary data analysis was performed.
Author contributions
DP and CP designed the study and wrote the protocol. YS-C and AT-L performed the statistical analyses. YS-C managed the literature searches and wrote the first draft of the manuscript, which was supervised by AT-L, PL-G, and JA-M. EG and CV participated in the recruitment, the collection of information, and the creation of databases. All authors contributed to the interpretation and discussion of the results and have approved the final manuscript.
Funding
This study was supported by the Instituto de Salud Carlos III (grant ref.: PI20/00229) and co-funded by the European Regional Development Fund (ERDF). YS-C work was supported by the FPI pre-doctoral grant (FPI 2016/17) from the Universidad Autonoma de Madrid.
Conflict of interest
The authors declare that the research was conducted in the absence of any commercial or financial relationships that could be construed as a potential conflict of interest.
Publisher’s note
All claims expressed in this article are solely those of the authors and do not necessarily represent those of their affiliated organizations, or those of the publisher, the editors and the reviewers. Any product that may be evaluated in this article, or claim that may be made by its manufacturer, is not guaranteed or endorsed by the publisher.
Supplementary material
The Supplementary Material for this article can be found online at: https://www.frontiersin.org/articles/10.3389/fpsyt.2023.1148643/full#supplementary-material
References
1. World Health Organization. Depression and Other Common Mental Disorders: Global Health Estimates (No. WHO/MSD/MER/2017.2). Geneva: WHO (2017).
2. Al-Asadi AM, Klein B, Meyer D. Multiple comorbidities of 21 psychological disorders and relationships with psychosocial variables: a study of the online assessment and diagnostic system within a web-based population. J Med Internet Res. (2015) 17:e55. doi: 10.2196/jmir.4143
3. de la Torre-Luque A, Essau CA. Symptom network connectivity in adolescents with comorbid major depressive disorder and social phobia. J Affect Disord. (2019) 255:60–8. doi: 10.1016/j.jad.2019.05.015
4. Fusar-Poli P, Solmi M, Brondino N, Davies C, Chae C, Politi P, et al. Transdiagnostic psychiatry: a systematic review. World Psychiatry. (2019) 18:192–207. doi: 10.1002/wps.20631
5. Ferrari AJ, Charlson FJ, Norman RE, Patten SB, Freedman G, Murray CJ, et al. Burden of depressive disorders by country, sex, age, and year: findings from the global burden of disease study 2010. PLoS Med. (2013) 10:e1001547. doi: 10.1371/journal.pmed.1001547
6. GBD 2019 Mental Disorders Collaborators. Global, regional, and national burden of 12 mental disorders in 204 countries and territories, 1990-2019: a systematic analysis for the Global Burden of Disease Study 2019. Lancet Psychiatry. (2022) 9:137–50. doi: 10.1016/S2215-0366(21)00395-3
7. Beurel E, Toups M, Nemeroff CB. The bidirectional relationship of depression and inflammation: double trouble. Neuron. (2020) 107:234–56. doi: 10.1016/j.neuron.2020.06.002
8. Colosanto, et al. (2020) Colasanto M, Madigan S, Korczak DJ. Depression and inflammation among children and adolescents: a meta-analysis. J Affect Disord. (2020) 277, 940–8. doi: 10.1016/j.jad.2020.09.025
9. Osimo EF, Pillinger T, Rodriguez IM, Khandaker GM, Pariante CM, Howes OD. Inflammatory markers in depression: a meta-analysis of mean differences and variability in 5,166 patients and 5,083 controls. Brain Behav Immun. (2020) 87:901–9. doi: 10.1016/j.bbi.2020.02.010
10. Vassou C, Georgousopoulou EN, Chrysohoou CC, Yannakoulia M, Pitsavos C, Cropley M, et al. Irrational beliefs trigger depression and anxiety symptoms, and associated with increased inflammation and oxidative stress markers in the 10-year diabetes mellitus risk: the ATTICA epidemiological study. J Diabetes Metab Disord. (2021) 20:727–39. doi: 10.1007/s40200-021-00805-3
11. Lamers F, Bot M, Jansen R, Chan MK, Cooper JD, Bahn S, et al. Serum proteomic profiles of depressive subtypes. Transl Psychiatry. (2016) 6:e851. doi: 10.1038/tp.2016.115
12. Lamers F, Milaneschi Y, Vinkers CH, Schoevers RA, Giltay EJ, Penninx B. Depression profilers and immuno-metabolic dysregulation: longitudinal results from the NESDA study. Brain Behav Immun. (2020) 88:174–83. doi: 10.1016/j.bbi.2020.04.002
13. Moriarity DP, van Borkulo C, Alloy LB. Inflammatory phenotype of depression symptom structure: a network perspective. Brain Behav Immun. (2021) 93:35–42. doi: 10.1016/j.bbi.2020.12.005
14. Costello H, Gould RL, Abrol E, Howard R. Systematic review and meta-analysis of the association between peripheral inflammatory cytokines and generalised anxiety disorder. BMJ Open. (2019) 9:e027925. doi: 10.1136/bmjopen-2018-027925
15. Renna ME, O’Toole MS, Spaeth PE, Lekander M, Mennin DS. The association between anxiety, traumatic stress, and obsessive–compulsive disorders and chronic inflammation: a systematic review and meta-analysis. Depress Anxiety. (2018) 35:1081–94. doi: 10.1002/da.22790
16. Qiu W, Cai X, Zheng C, Qiu S, Ke H, Huang Y. Update on the relationship between depression and neuroendocrine metabolism. Front Neurosci. (2021) 15:728810. doi: 10.3389/fnins.2021.728810
17. Feng X, Zhao Y, Yang T, Song M, Wang C, Yao Y, et al. Glucocorticoid-driven NLRP3 inflammasome activation in hippocampal microglia mediates chronic stress-induced depressive-like behaviors. Front Mol Neurosci. (2019) 12:210. doi: 10.3389/fnmol.2019.00210
18. Choi K, Chun J, Han K, Park S, Soh H, Kim J, et al. Risk of anxiety and depression in patients with inflammatory bowel disease: a nationwide, population-based study. J Clin Med. (2019) 8:654. doi: 10.3390/jcm8050654
19. Nagavi S, Gorrepati VS, Yadav S, Chintanaboina J, Maher S, Demuth P, et al. Influences and impact of anxiety and depression in the setting of inflammatory bowel disease. Inflamm Bowel Dis. (2018) 24:2303–8. doi: 10.1093/ibd/izy143
20. Fiksdal A, Hanlin L, Kuras Y, Gianferante D, Chen X, Thoma MV, et al. Associations between symptoms of depression and anxiety and cortisol responses to and recovery from acute stress. Psychoneuroendocrinology. (2019) 102:44–52. doi: 10.1016/j.psyneuen.2018.11.035
21. Haroon E, Raison CL, Miller AH. Psychoneuroimmunology meets neuropsychopharmacology: translational implications of the impact of inflammation on behavior. Neuropsychopharmacology. (2012) 37:137–62. doi: 10.1038/npp.2011.205
22. Stetler C, Miller GE. Depression and hypothalamic-pituitary-adrenal activation: a quantitative summary of four decades of research. Psychosom Med. (2011) 73:114–26. doi: 10.1097/PSY.0b013e31820ad12b
23. Choi KW, Kim Y, Jeon HJ. Comorbid anxiety and depression: clinical and conceptual consideration and transdiagnostic treatment. Adv Exp Med Biol. (2020) 1191:219–35. doi: 10.1007/978-981-32-9705-0_14
24. Bierhaus A, Wolf J, Andrassy M, Rohleder N, Humpert PM, Petrov D, et al. A mechanism converting psychosocial stress into mononuclear cell activation. Proc Natl Acad Sci USA. (2003) 100:1920–5. doi: 10.1073/pnas.0438019100
25. Bryleva EY, Keaton SA, Grit J, Madaj Z, Sauro-Nagendra A, Smart L, et al. The acute-phase mediator serum amyloid A is associated with symptoms of depression and fatigue. Acta Psychiatr Scand. (2017) 135:409–18.
26. Esteve E, Ricart W, Fernández-Real JM. Dyslipidemia and inflammation: an evolutionary conserved mechanism. Clin Nut. (2005) 24:16–31. doi: 10.1016/j.clnu.2004.08.004
27. Rosmond R, Björntorp P. The hypothalamic-pituitary-adrenal axis activity as a predictor of cardiovascular disease, type 2 diabetes and stroke. J Intern Med. (2000) 247:188–97. doi: 10.1046/j.1365-2796.2000.00603.x
28. Miller AH, Haroon E, Raison CL, Felger JC. Cytokine targets in the brain: impact on neurotransmitters and neurocircuits. Depress Anxiety. (2013) 30:297–306. doi: 10.1002/da.22084
29. Copeland WE, Shanahan L, Worthman C, Angold A, Costello EJ. Cumulative depression episodes predict later C-reactive protein levels: a prospective analysis. Biol Psychiatry. (2012) 71:15–21. doi: 10.1016/j.biopsych.2011.09.023
30. Copeland WE, Shanahan L, Worthman C, Angold A, Costello EJ. Generalized anxiety and C-reactive protein levels: a prospective, longitudinal analysis. Psychol Med. (2012) 42:2641–50. doi: 10.1017/S0033291712000554
31. Gaspersz R, Lamers F, Wittenberg G, Beekman A, van Hemert AM, Schoevers RA, et al. The role of anxious distress in immune dysregulation in patients with major depressive disorder. Transl Psychiatry. (2017) 7:1268. doi: 10.1038/s41398-017-0016-3
32. Penninx B, Lange S. Metabolic syndrome in psychiatric patients: overview, mechanisms, and implications. Dialogues Clin Neurosci. (2018) 20:63–73. doi: 10.31887/DCNS.2018.20.1/bpenninx
33. Lamers F, Milaneschi Y, Smit JH, Schoevers RA, Wittenberg G, Penninx B. Longitudinal association between depression and inflammatory markers: results from the netherlands study of depression and anxiety. Biol Psychiatry. (2019) 85:829–37. doi: 10.1016/j.biopsych.2018.12.020
34. Liukkonen T, Räsänen P, Jokelainen J, Leinonen M, Järvelin MR, Meyer-Rochow VB, et al. The association between anxiety and C-reactive protein (CRP) levels: results from the Northern Finland 1966 birth cohort study. Eur Psychiatry. (2011) 26:363–9. doi: 10.1016/j.eurpsy.2011.02.001
35. van Reedt Dortland AK, Giltay EJ, van Veen T, Zitman FG, Penninx BW. Longitudinal relationship of depressive and anxiety symptoms with dyslipidemia and abdominal obesity. Psychosom Med. (2013) 75:83–9. doi: 10.1097/PSY.0b013e318274d30f
36. Carroll JE, Low CA, Prather AA, Cohen S, Fury JM, Ross DC, et al. Negative affective responses to a speech task predict changes in interleukin (IL)-6. Brain Behav Immun. (2011) 25:232–8. doi: 10.1016/j.bbi.2010.09.024
37. Christian LM, Franco A, Iams JD, Sheridan J, Glaser R. Depressive symptoms predict exaggerated inflammatory responses to an in vivo immune challenge among pregnant women. Brain Behav Immun. (2010) 24:49–53. doi: 10.1016/j.bbi.2009.05.055
38. Glaser R, Robles TF, Sheridan J, Malarkey WB, Kiecolt-Glaser JK. Mild depressive symptoms are associated with amplified and prolonged inflammatory responses after influenza virus vaccination in older adults. Arch Gen Psychiatry. (2003) 60:1009–14. doi: 10.1001/archpsyc.60.10.1009
39. Moons WG, Shields GS. Anxiety, not anger, induces inflammatory activity: an avoidance/approach model of immune system activation. Emotion. (2015) 15:463–76. doi: 10.1037/emo0000055
40. Georgousopoulou EN, Kouli GM, Panagiotakos DB, Kalogeropoulou A, Zana A, Chrysohoou C, et al. Anti-inflammatory diet and 10-year (2002–2012) cardiovascular disease incidence: the ATTICA study. Int J Cardiol. (2016) 222:473–8. doi: 10.1016/j.ijcard.2016.08.007
41. Panagiotakos DB, Pitsavos C, Chrysohoou C, Skoumas J, Stefanadis C. Status and management of blood lipids in Greek adults and their relation to socio-demographic, lifestyle and dietary factors: the ATTICA Study. Blood lipids distribution in Greece. Atherosclerosis. (2004) 173:353–61. doi: 10.1016/j.atherosclerosis.2003.12.031
42. Pitsavos C, Panagiotakos DB, Chrysohoou C, Stefanadis C. Epidemiology of cardiovascular risk factors in Greece: aims, design and baseline characteristics of the ATTICA study. BMC Public Health. (2003) 3:32. doi: 10.1186/1471-2458-3-32
43. Zung, WW. A self-rating depression scale. Arch Genn Psychiatry. (1965) 12:63–70. doi: 10.1001/archpsyc.1965.01720310065008
44. Fountoulakis KN, Lacovides A, Samolis S, Kleanthous S, Kaprinis SG, St Kaprinis G, et al. Reliability, validity and psychometric properties of the Greek translation of the Zung depression rating scale. BMC Psychiatry. (2001) 1:6. doi: 10.1186/1471-244x-1-6
45. Fountoulakis KN, Papadopoulou M, Kleanthous S, Papadopoulou A, Bizeli V, Nimatoudis I, et al. Reliability and psychometric properties of the Greek translation of the state-trait anxiety inventory form Y: preliminary data. Ann Gen Psychiatry. (2006) 5:2. doi: 10.1186/1744-859X-5-2
46. Spielberger CD, Gorsuch RL, Lushene RE. Manual for the state-trait anxiety inventory (STAI). Palo Alto: Calif Consulting Psychologists Press Inc (1970).
47. Kyrou I, Kollia N, Panagiotakos D, Georgousopoulou E, Chrysohoou C, Tsigos C, et al. Association of depression and anxiety status with 10-year cardiovascular disease incidence among apparently healthy Greek adults: the ATTICA Study. Eur J Prev Cardiol. (2017) 24:145–52. doi: 10.1177/2047487316670918
48. Grundy SM, Brewer HB Jr., Cleeman JI, Smith SC Jr., Lenfant C, National Heart, Lung, and Blood Institute, et al. Definition of metabolic syndrome: report of the National Heart, Lung, and Blood Institute/American Heart Association conference on scientific issues related to definition. Arterioscler Thromb Vasc Biol. (2004) 24:e13–8. doi: 10.1161/01.ATV.0000111245.75752.C6
49. Pitsavos C, Panagiotakos DB, Tzima N, Chrysohoou C, Economou M, Zampelas A, et al. Adherence to the Mediterranean diet is associated with total antioxidant capacity in healthy adults: the ATTICA study. Am J Clin Nutr. (2005) 82:694–9. doi: 10.1093/ajcn.82.3.694
50. Jöreskog KG, Sörbom D. PRELIS 2: User’s Reference Guide. (1996). Chicago: Scientific Software International.
51. Hu LT, Bentler P. Cutoff criteria for fit indexes in covariance structure analysis: conventional criteria versus new alternatives, structural equation modeling. Struct Equ Model. (1999) 6:1–55.
52. Dunn AJ, Swiergiel AH, de Beaurepaire R. Cytokines as mediators of depression: what can we learn from animal studies? Neurosci Biobehav Rev. (2005) 29:891–909. doi: 10.1016/j.neubiorev.2005.03.023
53. Pitsavos C, Panagiotakos DB, Papageorgiou C, Tsetsekou E, Soldatos C, Stefanadis C. Anxiety in relation to inflammation and coagulation markers, among healthy adults: the ATTICA study. Atherosclerosis. (2006) 185:320–6. doi: 10.1016/j.atherosclerosis.2005.06.001
54. Carpenter LL, Gawuga CE, Tyrka AR, Lee JK, Anderson GM, Price LH. Association between plasma IL-6 response to acute stress and early-life adversity in healthy adults. Neuropsychopharmacology. (2010) 35:2617–23. doi: 10.1038/npp.2010.159
55. Lopes RP, Grassi-Oliveira R, de Almeida LR, Stein LM, Luz C, Teixeira AL, et al. Neuroimmunoendocrine interactions in patients with recurrent major depression, increased early life stress and long-standing posttraumatic stress disorder symptoms. Neuroimmunomodulation. (2012) 19:33–42. doi: 10.1159/000327352
56. Smith KJ, Au B, Ollis L, Schmitz N. The association between C-reactive protein, Interleukin-6 and depression among older adults in the community: a systematic review and meta-analysis. Exp Gerontol. (2018) 102:109–32. doi: 10.1016/j.exger.2017.12.005
57. Raison CL, Miller AH. The evolutionary significance of depression in Pathogen Host Defense (PATHOS-D). Mol Psychiatry. (2013) 18:15–37. doi: 10.1038/mp.2012.2
58. Cavicchioli FL, Maes M, Roomruangwong C, Bonifacio KL, Barbosa DS, Anderson G, et al. Associations between severity of anxiety and clinical and biological features of major affective disorders. Psychiatry Res. (2018) 260:17–23. doi: 10.1016/j.psychres.2017.11.024
59. Pan A, Keum N, Okereke OI, Sun Q, Kivimaki M, Rubin RR, et al. Bidirectional association between depression and metabolic syndrome: a systematic review and meta-analysis of epidemiological studies. Diabetes Care. (2012) 35:1171–80. doi: 10.2337/dc11-2055
60. Franceschi C, Capri M, Monti D, Giunta S, Olivieri F, Sevini F, et al. Inflammaging and anti-inflammaging: a systemic perspective on aging and longevity emerged from studies in humans. Mech Ageing Dev. (2007) 128:92–105. doi: 10.1016/j.mad.2006.11.016
61. van Reedt Dortland AK, Giltay EJ, van Veen T, Zitman FG, Penninx BW. Metabolic syndrome abnormalities are associated with severity of anxiety and depression and with tricyclic antidepressant use. Acta Psychiatr Scand. (2010) 122:30–9. doi: 10.1111/j.1600-0447.2010.01565.x
62. Vogelzangs N, Beekman AT, Kritchevsky SB, Newman AB, Pahor M, Yaffe K, et al. Psychosocial risk factors and the metabolic syndrome in elderly persons: findings from the Health, Aging and Body Composition study. J Gerontol A Biol Sci Med Sci. (2007) 62:563–9. doi: 10.1093/gerona/62.5.563
63. Heiskanen TH, Niskanen LK, Hintikka JJ, Koivumaa-Honkanen HT, Honkalampi KM, Haatainen KM, et al. Metabolic syndrome and depression: a cross-sectional analysis. J Clin Psychiatry. (2006) 67:1422–7. doi: 10.4088/jcp.v67n0913
64. Kahl KG, Greggersen W, Schweiger U, Cordes J, Balijepalli C, Lösch C, et al. Prevalence of the metabolic syndrome in unipolar major depression. Eur Arch Psychiatry Clin Neurosci. (2012) 262:313–20. doi: 10.1007/s00406-011-0277-4
65. Carroll D, Phillips AC, Thomas GN, Gale CR, Deary I, Batty GD. Generalized anxiety disorder is associated with metabolic syndrome in the Vietnam experience study. Biol Psychiatry. (2009) 66:91–3. doi: 10.1016/j.biopsych.2009.02.020
66. Herva A, Räsänen P, Miettunen J, Timonen M, Läksy K, Veijola J, et al. Co-occurrence of metabolic syndrome with depression and anxiety in young adults: the Northern Finland 1966 Birth Cohort Study. Psychosom Med. (2006) 68:213–6. doi: 10.1097/01.psy.0000203172.02305.ea
67. Hildrum B, Mykletun A, Midthjell K, Ismail K, Dahl AA. No association of depression and anxiety with the metabolic syndrome: the Norwegian HUNT study. Acta Psychiatr Scand. (2009) 120:14–22. doi: 10.1111/j.1600-0447.2008.01315.x
68. Skilton MR, Moulin P, Terra JL, Bonnet F. Associations between anxiety, depression, and the metabolic syndrome. Biol Psychiatry. (2007) 62:1251–7. doi: 10.1016/j.biopsych.2007.01.012
69. Prochaska JJ. Smoking and mental illness—breaking the link. N Engl J Med. (2011) 365:196–8. doi: 10.1056/NEJMp1105248
70. Weinberger AH, Kashan RS, Shpigel DM, Esan H, Taha F, Lee CJ, et al. Depression and cigarette smoking behaviour: a critical review of population-based studies. Am J Drug Alcohol Abuse. (2017) 43:416–31. doi: 10.3109/00952990.2016.1171327
71. Bernier V, Debarge MH, Hein M, Ammendola S, Mungo A, Loas G. Major depressive disorder, inflammation, and nutrition: a tricky pattern? Nutrients. (2023) 15:3438. doi: 10.3390/nu15153438
72. Stubbs B, Vancampfort D, Hallgren M, Firth J, Veronese N, Solmi M, et al. EPA guidance on physical activity as a treatment for severe mental illness: a meta-review of the evidence and Position Statement from the European Psychiatric Association (EPA), supported by the International Organization of Physical Therapists in Mental Health (IOPTMH). Eur Psychiatry. (2018) 54:124–44.
73. Zhai L, Zhang Y, Zhang D. Sedentary behaviour and the risk of depression: a meta-analysis. Br J Sports Med. (2015) 49:705–9. doi: 10.1136/bjsports-2014-093613
74. Nicholson A, Kuper H, Hemingway H. Depression as an aetiologic and prognostic factor in coronary heart disease: a meta-analysis of 6362 events among 146 538 participants in 54 observational studies. Eur Heart J. (2006) 27:2763–74. doi: 10.1093/eurheartj/ehl338
75. De Moor MH, Beem AL, Stubbe JH, Boomsma DI, De Geus EJ. Regular exercise, anxiety, depression and personality: a population-based study. Prev Med. (2006) 42:273–9. doi: 10.1016/j.ypmed.2005.12.002
77. Mammen G, Faulkner G. Physical activity and the prevention of depression: a systematic review of prospective studies. Am J Prev Med. (2013) 45:649–57. doi: 10.1016/j.amepre.2013.08.001
78. Pareja-Galeano H, Mayero S, Perales M, Garatachea N, Santos-Lozano A, Fiuza-Luces C, et al. Biological rationale for regular physical exercise as an effective intervention for the prevention and treatment of depressive disorders. Curr Pharm Des. (2016) 22:3764–75. doi: 10.2174/1381612822666160322144537
79. Teychenne M, Ball K, Salmon J. Physical activity and likelihood of depression in adults: a review. Prev Med. (2008) 46:397–411. doi: 10.1016/j.ypmed.2008.01.009
80. Sánchez-Carro Y, de la Torre-Luque A, Leal-Leturia I, Salvat-Pujol N, Massaneda C, de Arriba-Arnau A, et al. Importance of immunometabolic markers for the classification of patients with major depressive disorder using machine learning. Prog Neuropsychopharmacol Biol Psychiatry. (2023) 121:110674. doi: 10.1016/j.pnpbp.2022.110674
81. Shim IH, Woo YS, Bahk WM. Associations between immune activation and the current severity of the “with anxious distress” specifier in patients with depressive disorders. Gen Hosp Psychiatry. (2016) 42:27–31. doi: 10.1016/j.genhosppsych.2016.07.003
82. Baek JH, Kim HJ, Fava M, Mischoulon D, Papakostas GI, Nierenberg A, et al. Reduced venous blood basophil count and anxious depression in patients with major depressive disorder. Psychiatry Investig. (2016) 13:321–6. doi: 10.4306/pi.2016.13.3.321
83. Chan KL, Cathomas F, Russo SJ. Central and peripheral inflammation link metabolic syndrome and major depressive disorder. Physiology. (2019) 34:123–33. doi: 10.1152/physiol.00047.2018
84. Lasselin J, Capuron L. Chronic low-grade inflammation in metabolic disorders: relevance for behavioral symptoms. Neuroimmunomodulation. (2014) 21:95–101. doi: 10.1159/000356535
86. Paulweber B, Valensi P, Lindström J, Lalic NM, Greaves CJ, McKee M, et al. A European evidence-based guideline for the prevention of type 2 diabetes. Horm Met Res. (2010) 42, (Suppl. 1):S3–36. doi: 10.1055/s-0029-1240928
87. Graham EA, Deschênes SS, Khalil MN, Danna S, Filion KB, Schmitz N. Measures of depression and risk of type 2 diabetes: a systematic review and meta-analysis. J Affect Disord. (2020) 265:224–32. doi: 10.1016/j.jad.2020.01.053
88. Hasan SS, Clavarino AM, Mamun AA, Kairuz T. Incidence and risk of diabetes mellitus associated with depressive symptoms in adults: evidence from longitudinal studies. Diabetes Metab Syndr. (2014) 8:82–7. doi: 10.1016/j.dsx.2014.04.023
89. Knol MJ, Twisk JW, Beekman AT, Heine RJ, Snoek FJ, Pouwer F. Depression as a risk factor for the onset of type 2 diabetes mellitus. A meta-analysis. Diabetologia. (2006) 49:837–45. doi: 10.1007/s00125-006-0159-x
90. Mezuk B, Eaton WW, Albrecht S, Golden SH. Depression and type 2 diabetes over the lifespan: a meta-analysis. Diabetes Care. (2008) 31:2383–90. doi: 10.2337/dc08-0985
91. Rotella F, Mannucci E. Depression as a risk factor for diabetes: a meta-analysis of longitudinal studies. J Clin Psychiatry. (2013) 74:31–7. doi: 10.4088/JCP.12r07922
92. Aune D, Norat T, Leitzmann M, Tonstad S, Vatten LJ. Physical activity and the risk of type 2 diabetes: a systematic review and dose-response meta-analysis. Eur J Epidemiol. (2015) 30:529–42. doi: 10.1007/s10654-015-0056-z
93. Francesconi C, Lackinger C, Weitgasser R, Haber P, Niebauer J. Körperliche aktivität und training in der prävention und therapie des typ 2 diabetes mellitus [physical activity and exercise training in the prevention and therapy of type 2 diabetes mellitus]. Wie Klin Wochenschr. (2016) 128(Suppl. 2):S141–5. doi: 10.1007/s00508-015-0923-3
94. Deverts DJ, Cohen S, DiLillo VG, Lewis CE, Kiefe C, Whooley M, et al. Depressive symptoms, race, and circulating C-reactive protein: the Coronary Artery Risk Development in Young Adults (CARDIA) study. Psychosom Med. (2010) 72:734–41. doi: 10.1097/PSY.0b013e3181ec4b98
95. Goldstein BI, Fagiolini A, Houck P, Kupfer DJ. Cardiovascular disease and hypertension among adults with bipolar I disorder in the United States. Bipolar Disord. (2009) 11:657–62. doi: 10.1111/j.1399-5618.2009.00735.x
96. Meng L, Chen D, Yang Y, Zheng Y, Hui R. Depression increases the risk of hypertension incidence: a meta-analysis of prospective cohort studies. J Hypertens. (2012) 30:842–51. doi: 10.1097/HJH.0b013e32835080b7
97. Bhat SK, Beilin LJ, Robinson M, Burrows S, Mori TA. Relationships between depression and anxiety symptoms scores and blood pressure in young adults. J Hypertens. (2017) 35:1983–91. doi: 10.1097/HJH.0000000000001410
98. Shinn EH, Poston WS, Kimball KT, St Jeor ST, Foreyt JP. Blood pressure and symptoms of depression and anxiety: a prospective study. Am J Hypertens. (2001) 14(7 Pt. 1):660–4. doi: 10.1016/s0895-7061(01)01304-8
99. Wiehe M, Fuchs SC, Moreira LB, Moraes RS, Pereira GM, Gus M, et al. Absence of association between depression and hypertension: results of a prospectively designed population-based study. J Hum Hypertens. (2006) 20:434–9. doi: 10.1038/sj.jhh.1002017
100. Jackson CA, Pathirana T, Gardiner PA. Depression, anxiety and risk of hypertension in mid-aged women: a prospective longitudinal study. J Hypertens. (2016) 34:1959–66. doi: 10.1097/HJH.0000000000001030
101. Enko D, Brandmayr W, Halwachs-Baumann G, Schnedl WJ, Meinitzer A, Kriegshäuser G. Prospective plasma lipid profiling in individuals with and without depression. Lipids Health Dis. (2018) 17:149. doi: 10.1186/s12944-018-0796-3
102. Wei YG, Cai DB, Liu J, Liu RX, Wang SB, Tang YQ, et al. Cholesterol and triglyceride levels in first-episode patients with major depressive disorder: a meta-analysis of case-control studies. J Affect Disord. (2020) 266:465–72.
103. Moreira FP, Jansen K, Cardoso TA, Mondin TC, Magalhães P, Kapczinski F, et al. Metabolic syndrome in subjects with bipolar disorder and major depressive disorder in a current depressive episode: population-based study: metabolic syndrome in current depressive episode. J Psychiatr Res. (2017) 92:119–23. doi: 10.1016/j.jpsychires.2017.03.025
104. Ledochowski M, Murr C, Sperner-Unterweger B, Neurauter G, Fuchs D. Association between increased serum cholesterol and signs of depressive mood. Clin Chem Lab Med. (2003) 41:821–4. doi: 10.1515/CCLM.2003.124
105. Ong KL, Morris MJ, McClelland RL, Maniam J, Allison MA, Rye KA. Lipids, lipoprotein distribution and depressive symptoms: the multi-ethnic study of atherosclerosis. Transl Psychiatry. (2016) 6:e962. doi: 10.1038/tp.2016.232
Keywords: depression, anxiety, inflammation, metabolic syndrome, diabetes, hypertension, hypercholesterolemia
Citation: Sanchez-Carro Y, de la Torre-Luque A, Vassou C, Lopez-Garcia P, Georgousopoulou E, Pitsavos C, Ayuso-Mateos JL and Panagiotakos D (2023) Effects of elevated emotional symptoms on metabolic disease development: a 10-year follow-up study. Front. Psychiatry 14:1148643. doi: 10.3389/fpsyt.2023.1148643
Received: 20 January 2023; Accepted: 07 November 2023;
Published: 04 December 2023.
Edited by:
Martine Elbejjani, American University of Beirut, LebanonReviewed by:
Pillaveetil Sathyadas Indu, Government Medical College, IndiaMona Nasrallah, American University of Beirut, Lebanon
Copyright © 2023 Sanchez-Carro, de la Torre-Luque, Vassou, Lopez-Garcia, Georgousopoulou, Pitsavos, Ayuso-Mateos and Panagiotakos. This is an open-access article distributed under the terms of the Creative Commons Attribution License (CC BY). The use, distribution or reproduction in other forums is permitted, provided the original author(s) and the copyright owner(s) are credited and that the original publication in this journal is cited, in accordance with accepted academic practice. No use, distribution or reproduction is permitted which does not comply with these terms.
*Correspondence: Alejandro de la Torre-Luque, YWYuZGVsYXRvcnJlQHVjbS5lcw==