- 1Department of Military Medical Psychology, Air Force Medical University, Xi’an, China
- 2Department of Nursing, Air Force Medical University, Xi’an, China
- 3Xiong’an Rongxi Linquan Primary School, Xiong’an New Area, China
- 4Xijing Hospital, Air Force Medical University, Xi’an, China
- 5Tangdu Hospital, Air Force Medical University, Xi’an, China
- 6The First Primary School of Fuliang County, Jingdezhen, China
- 7School of Public Health, Xi’an Medical University, Xi’an, China
- 8Psychology Section, Secondary Sanatorium of Air Force Healthcare Center for Special Services, Hangzhou, China
Background: Internet gaming disorder (IGD) has become a social problem in children. Evidence from previous studies has proven that anxiety is associated with IGD. However, IGD was always assessed as a whole based on total scores, and the fine-grained relationship between anxiety and IGD was hidden.
Objective: The present study aims to investigate the fine-grained relationship between anxiety and IGD in elementary school students during the COVID-19 lockdown, and to identify potential targets for psychological interventions.
Methods: During the lockdown caused by the COVID-19 pandemic, 667 children from a primary school in China were investigated by the Spence Children’s Anxiety Scale-Short Version and Internet Gaming Disorder Scale. R4.1.1 software was used to construct a network model, assess bridge centrality, and test the robustness of the network and conduct a network.
Results: There were 23 cross-community edges (weight ranged from −0.03 to 0.12), and each node of anxiety was connected to different nodes of IGD. The nodes with the top 80th percentile bridge expected influence were A2 “social phobia” (0.20), A3 “panic disorder” (0.21) and IGD5 “escape” (0.22). The robustness of the network was acceptable.
Conclusion: From the perspective of network analysis, the present study explored the correlation pathways between anxiety and IGD in children and identified social phobia and panic disorder as the best targets for intervention to reduce IGD.
1. Introduction
The 2020 national research report on internet access by minors shows that with the rapid development of new internet technologies, internet access by minors is becoming increasingly common. By the end of 2019, 175 million Chinese minors were internet users, accounting for 93.1% of the total number of minors, while the internet penetration rate of elementary school students was also high, at 89.4% (1). There is a trend of younger teenagers accessing the internet (2). Meanwhile, the childhood stage is a critical period for psychological development and personality formation (3). Children are curious about new things, good at imitation (4, 5), undisciplined and easily impulsive (6, 7). Therefore, children are more likely to be addicted to the internet than more mature individuals (8), and one of the main types of internet addiction is internet gaming disorder (IGD) (9). IGD is described as being addicted to online games for a long time and developing a strong sense of dependence and continuous craving behavior (10, 11).
The current diagnosis and definition of IGD mainly relies on the fifth edition of the Diagnostic and Statistical Manual of Mental Disorders (DSM-5) published by the American Psychiatric Association in 2013 (12), which lists nine diagnostic criteria for IGD: addiction to games; increased tolerance; giving up other activities to play games; reckless disregard for the adverse effects; using games as a way to cope and escape negative emotional experiences; social/occupational disruptions because of playing games; trying and failing to control online game time; deliberately hiding online game-related problems; and becoming irritable and temperamental if they cannot access the internet. During the outbreak of COVID-19, school closures, prolonged isolation at home, and reduced offline interactions increases the risk of IGD (13). IGD causes psychological and physical damage to children (14–17), jeopardizes social skills and academic performance (18), and even leads to suicide and delinquency (19–21). In light of these findings, there is an urgent need to explore the mechanisms of IGD in elementary school students to provide targets for early psychological intervention.
Evidence from previous studies has proven that anxiety is associated with IGD (22, 23). Individuals with anxiety disorders are more likely to socialize online, overuse smartphones and be addicted to online games (24–26). Currently, influenced by all kinds of media information and uncertainty about the perceived risk of the epidemic, people are more anxious in the face of the COVID-19 lockdown. Therefore, the relationship between anxiety and IGD during the pandemic has attracted the attention of researchers. Fazeli et al. (13) found that IGD significantly influenced anxiety and depression symptoms in adolescents during the COVID-19 epidemic. Wang et al. (27) found that adolescents with anxiety disorders were more likely to develop IGD in the presence of fear of missing out. Elhai et al. (28) found that COVID-19 anxiety was strongly associated with smartphone use.
However, in previous studies investigating the relationship between anxiety and IGD, IGD was always assessed as a whole based on total scores (29). Indeed, the DSM-5 diagnostic criteria for IGD contain nine heterogeneous symptoms, and each symptom may be sensitive to specific risk factors and represent a unique risk pathway mechanism. The correlation based on the total score hides the fine-grained relationship between the different symptoms of anxiety and IGD (30). In addition, previous correlation studies cannot provide intervention targets at symptom level because there was no measure that quantifies the relative importance of items.
Network analysis is a promising statistical method to solve these problems. As data-driven processing that does not rely on a priori assumptions about the relationships between variables (31), network analysis helps reveal the relationships between psychological variables at the fine-grained level. The network structure consists of nodes representing psychological variables and lines representing statistical relationships between variables (32). Compared with traditional statistical correlation research, network analysis has the following advantages: (a) the ability to clarify fine-grained relationships between variables (33); (b) the potential to avoid spurious correlations due to a large number of variables (34); (c) visualization of association patterns between different variables (35); and (d) the capacity to assess the relative importance of variables by providing a centrality index (36). The term community is used to denote a theory-based set of psychological variables, and index bridge centrality helps to accurately capture the variables that play a key role in bridging communities (37), these bridge variables are often considered as potential targets for psychological intervention. Thus, network analysis may help deepen our understanding of the relationship between anxiety and IGD and provide targets for psychological intervention.
In summary, based on network analysis, the present study investigated the fine-grained relationship between anxiety and IGD in elementary school students during the COVID-19 lockdown. We developed a network model to explore the correlation pathways between the symptoms of anxiety and IGD and assessed bridge centrality to provide a theoretical basis for identifying effective symptom targets for psychological interventions.
2. Materials and methods
2.1. Participants
In April 2022, during the lockdown caused by the COVID-19 pandemic, a cluster sample of children in Baoding City, China, was investigated. Inclusion criteria: (a) children from the elementary grades of primary school; (b) isolated at home for at least 1 week due to the COVID-19 epidemic; (c) clear consciousness and normal perceptual function; (d) volunteered to participate in the study and provided the informed consent. Exclusion criteria: (a) cognitive dysfunction or communication disorder; (b) incomplete questionnaires. The Spence Children’s Anxiety Scale-Short Version (SCAS-S) and Internet Gaming Disorder Scale (IGDS) powered by www.wjx.cn were used to survey the children after explaining the purpose and method of the research to the children and their parents. The parents were required to read the questions and response options of each item to their children, and then the children answered according to their own feelings. A total of 722 questionnaires were distributed, and incomplete questionnaires were deemed invalid. Finally, 667 valid questionnaires were collected for an effective recovery rate of 92.38%. The present research followed the Helsinki Declaration and was approved by the Ethics Committee of Xijing Hospital of Air Force Medical University (Project No. CHiCTR1800019761).
2.2. Measures
2.2.1. SCAS-S
The SCAS-S was revised by Ahlen et al. (38) on the basis of the Spence Children’s Anxiety Scale (39) to evaluate anxiety symptoms. The SCAS-S contains 19 items and consists of 5 dimensions, namely, separation anxiety, social phobia, panic disorder, physical injury fear and generalized anxiety. The SCAS-S is a Likert 4-point scoring scale: 0 = never, 1 = rarely, 2 = sometimes, 4 = always. The higher the score, the more severe the anxiety. The Cronbach’s α of this scale in the present research was 0.80.
2.2.2. IGDS
The IGDS was compiled by Pontes and Griffiths (40) to assess IGD in the previous 6 months. The Chinese version of the IGDS revised by Jiang and Zeng (41) was used in this study. The nine items of the IGDS correspond to the nine diagnostic criteria for online game addiction in DSM-5, namely, preoccupation, tolerance, giving up other activities, continuing despite problems, escape, negative consequences, loss of control, deception, and withdrawal. IGDS is measured on a Likert 5-point scoring scale: 1 = never, 2 = often not, 3 = sometimes, 4 = often, and 5 = always. The higher the score, the stronger the tendency of IGD. The Cronbach’s α of this scale in the present research was 0.89.
2.3. Statistical analysis
Statistics of demographic characteristics and scale scores were calculated by SPSS 22.0 software. Network model construction, bridge centrality analysis, and robustness tests were conducted by R4.1.1 software.
2.3.1. Network model construction
The Gaussian graphical models (GGMs) were fitted to the data (32). In the network model, nodes represented variables of SCAS-S and IGDS and were divided into anxiety community (separation anxiety, social phobia, panic disorder, physical injury fear, and generalized anxiety) and IGD community (preoccupation, tolerance, giving up other activities, continuing despite problems, escape, negative consequences, loss of control, deception, and withdrawal). Edges between nodes represented partial correlations after statistically eliminating interference from all other nodes (34). Edge color indicates the nature of the partial correlation, red edges suggest a negative correlation, blue edges suggest a positive correlation, edge saturation indicates the intensity of the partial correlation, and a more saturated edge suggests a larger partial correlation. The combination of least absolute shrinkage and selection operator (LASSO) (42) regularization and extended Bayesian information criterion (EBIC) (43) was used in network model construction to shrink small edges to zero weight and make the network stable and clear (44). To balance the sensitivity and specificity (45), the EBIC hyperparameter γ was set to 0.5. The Fruchterman-Reingold algorithm was used to build the network layout (46). The anxiety-IGD network model was constructed with the qgraph package (47).
2.3.2. Bridge centrality analysis
In the present study, the bridge expected influence (BEI) was estimated. As a kind of bridge centrality index, the BEI of a node is defined as the sum of the edge weights between the node and all nodes from other communities (37). In a bidirectional network, a higher value of BEI indicates a greater likelihood of affecting or being affected by other communities. The bridge centrality of nodes was evaluated by the networktools package (37).
2.3.3. Network robustness test
To test the network robustness, three operations were conducted. First, we estimated the 95% confidence interval of edge weights to evaluate the accuracy by non-parametric bootstrapping (1,000 bootstrapped samples). A relatively narrow 95% confidence interval ensures adequate accuracy of the edge weights (44). Second, we tested the stability of BEI by case-dropping bootstrapping (1,000 bootstrapped samples). We also calculated the correlation stability coefficient (CS-coefficient) to quantify the stability; a value larger than 0.5 of CS-coefficient indicates ideal stability (44). Finally, we tested the difference in the BEI indices of nodes and the difference in the edge weights of node pairs by bootstrapping (1,000 bootstrapped samples, α = 0.05). The network robustness was tested with the bootnet package (44).
3. Results
3.1. Demographic characteristics and descriptive statistics
The demographic characteristics of the sample are displayed in Table 1. The means and standard deviations of the variables in the anxiety-IGD network are displayed in Table 2.
3.2. Fine-grained relationship between anxiety and IGD
The anxiety-IGD network is displayed in Figure 1A. Logically, there was a maximum of 45 edges across the communities in the network; in the present research, there were 23 cross-community edges (weights ranged from −0.03 to 0.12). Overall, positive edges accounted for the vast majority of cross-communities edges (86.96%). The positive cross-community edges contained A1 “separation anxiety”—IGD3 “giving up other activities” (edge weight = 0.04), A1 “separation anxiety”—IGD5 “escape” (edge weight = 0.02), A1 “separation anxiety”—IGD9 “withdrawal” (edge weight = 0.01), A2 “social phobia”—IGD1 “preoccupation” (edge weight = 0.04), A2 “social phobia”—IGD2 “tolerance” (edge weight = 0.04), A2 “social phobia”—IGD3 “giving up other activities” (edge weight = 0.06), A2 “social phobia”—IGD4 “continuing despite problems” (edge weight = 0.01), A2 “social phobia”—IGD6 “negative consequences” (edge weight = 0.04), A2 “social phobia”—IGD8 “deception” (edge weight = 0.02), A3 “panic disorder”—IGD5 “escape” (edge weight = 0.12), A3 “panic disorder”—IGD6 “negative consequences” (edge weight = 0.06), A3 “panic disorder”—IGD7 “loss of control” (edge weight = 0.02), A3 “panic disorder”—IGD9 “withdrawal” (edge weight = 0.01), A4 “physical injury fear” —IGD5 “escape” (edge weight = 0.01), A5 “generalized anxiety”—IGD1 “preoccupation” (edge weight = 0.003), A5 “generalized anxiety”—IGD4 “continuing despite problems” (edge weight = 0.03), A5 “generalized anxiety”—IGD5 “escape” (edge weight = 0.06), A5 “generalized anxiety”—IGD6 “negative consequences” (edge weight = 0.03), A5 “generalized anxiety”—IGD8 “deception” (edge weight = 0.01) and A5 “generalized anxiety”—IGD9 “withdrawal” (edge weight = 0.04). The negative cross-community edges contain A1 “separation anxiety”—IGD6 “negative consequences” (edge weight = −0.01), A4 “physical injury fear”—IGD6 “negative consequences” (edge weight = −0.03) and A4 “physical injury fear”—IGD8 “deception” (edge weight = −0.02). The correlation matrix of the network model can be found in Supplementary Table 1.
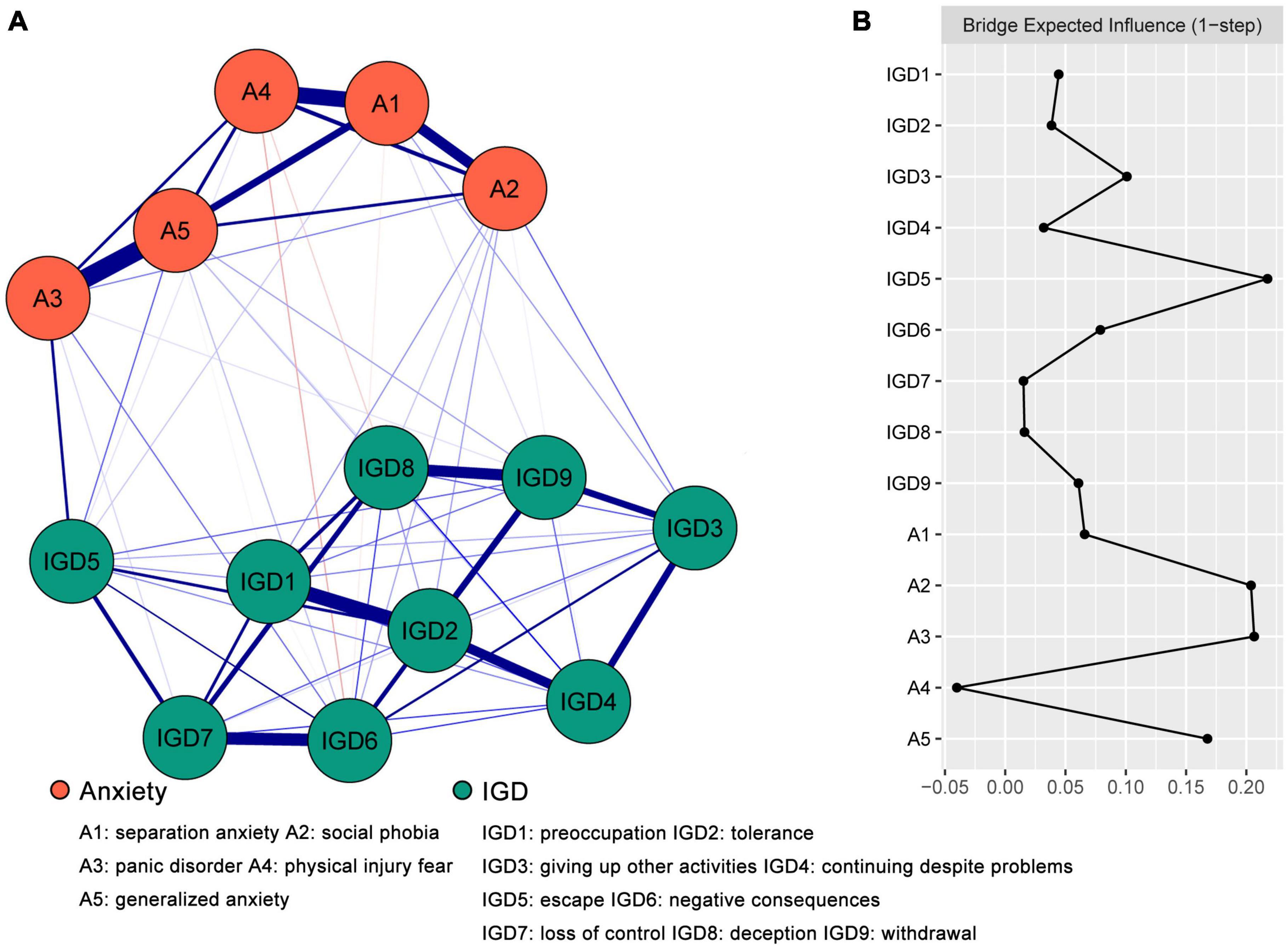
Figure 1. The anxiety-IGD network model and bridge expected influence (BEI). (A) The anxiety-IGD network model. The red edges suggested a negative correlation, while the blue edges suggested a positive correlation, and a more saturated edge suggested a larger partial correlation. (B) The bridge expected influence indices of the nodes in the network (raw score).
The accuracy test of edge weights is displayed in Supplementary Figure 1. The 95% confidence interval of edge weights in the anxiety-IGD network was relatively narrow, indicating that the edge estimation was accurate. The difference test of edge weights is displayed in Supplementary Figure 2.
3.3. Bridges between anxiety and IGD
The BEI is displayed in Figure 1B. The nodes with the top 80th percentile BEI were A2 “social phobia” (0.20), A3 “panic disorder” (0.21) and IGD5 “escape” (0.22). As shown in Supplementary Figure 3, the BEI indices of A2 “social phobia,” A3 “panic disorder” and IGD5 “escape” were statistically larger than those of most other nodes. As described in Supplementary Figure 4, the average correlation of BEI indices of the subsample and the original sample showed a relatively gentle downward trend with the reduction of subsample. The value of the CS-coefficient on the BEI was 0.52, which was larger than 0.5, indicating adequate stability.
4. Discussion
Based on network analysis, the present study revealed the correlation pathways between anxiety and IGD in elementary school children during the COVID-19 lockdown and provided suggestions for IGD interventions through the evaluation of the BEI index. The stability and accuracy of the network model were acceptable, which guaranteed the reliability of our conclusion.
4.1. Correlation pathways between anxiety and IGD
The detailed relationships between variables revealed by network analysis can provide indications of the correlation pathways through which different aspects of anxiety affect IGD (48, 49). These correlation pathways may represent underlying interaction mechanisms of anxiety and IGD. In light of this, we discussed the most powerful pathways bridging various aspects of anxiety and contents in IGD.
Among the four pathways through which “separation anxiety” correlated with IGD, the strongest pathway was “separation anxiety”—“giving up other activities,” while among the six pathways through which “social phobia” correlated with IGD, the strongest pathway was “social phobia”—“giving up other activities.” Separation anxiety is described as the irrational fear or anxiety of being separated from family or close attachment (12, 50). According to Bowlby’s attachment theory, the quality of parental care a child receives in early years has profound effects on future mental health (51). Children who do not receive effective care and attention from parents are incapable of dealing with separation anxiety. Many children have been separated from their relatives and even parents due to the COVID-19 lockdown, and separation anxiety caused by the disruption of close relationships drives children to online games, which may meet their emotional requirements. Social phobia is described as the excessive fear of social occasions and interactions with others and may result in social isolation (52). Baker and Hudson (53) found that children with social phobia had narrower social networks and poorer friendship quality and were more likely to experience social alienation than children with other anxiety symptoms. This may be due to increased sensitivity to social exclusion and social withdrawal in adolescents with symptoms of social phobia leading to deficits in interpersonal skills (54). Therefore, children with social phobia tend to abandon offline social activities and indulge in virtual worlds. The reason is that the internet provides a platform for them to communicate anonymously.
Among the four pathways through which “panic disorder” correlated with IGD, the strongest pathway was “panic disorder”—“escape,” while among the six pathways through which “generalized anxiety” correlated with IGD, the strongest pathway was “generalized anxiety”—“escape.” Panic disorder is characterized by misinterpretations of explosive sensory and emotion, i.e., heart palpitations, dyspnea, dizziness, and near-death experience (55, 56). Inconsistent with panic disorder, generalized anxiety is characterized by persistent, relatively mild symptoms on most days for at least 6 months, such as nervosity, irritability, fatigue, attention and memory problems, and insomnia (12, 57, 58). Both panic disorder and generalized anxiety lead to evident negative emotional experiences, and when there is no appropriate outlet, such as during the COVID-19 lockdown, children often turn to online games to escape these emotional experiences.
Among the three pathways through which “physical injury fear” correlated with IGD, the strongest pathway was “physical injury fear”—“negative consequences.” The symptoms of physical injury fear are similar to those of specific phobia; they all manifest as extreme and persistent fear of a certain object or situation, such as heights, tunnels, darkness, or worms (59). COVID-19 may cause death and sequelae (60), and related reports have led to increased sensitivity to physical injury fear. In addition, the children in the present study were too young to identify the authenticity of information, so they were excessively afraid of dangerous information and were prone to online gaming addiction, which could lead to a series of negative consequences.
4.2. Optimal targets for intervention
Anxiety is comorbid with IGD (61), and the activation of bridge symptoms can increase the risk of transferring from one disorder to another (37). In network analysis, bridge centrality explained the comorbidity and reciprocity of different disorders (62). The current study identified bridge symptoms between anxiety and IGD in children. Bridge symptom identification helps reveal fecund information on comorbidities and provide prioritized clinical targets to prevent co-occurrence (63, 64). The BEI indices of “social phobia” and “panic disorder” were the largest in the anxiety community. From the perspective of the network, the intervention of “social phobia” and “panic disorder” has a greater impact on IGD than other nodes of the anxiety community, so they are potential best targets for interventions. Previous studies have confirmed the relationship between social phobia and IGD (65). In fact, children with social phobia have more personal space and time, which is a crucial risk factor for IGD (66). Given the acuteness and recurrence of panic disorder symptoms (67), individuals may have persistent worries, and online games may provide a distraction. Gaming online is an escape strategy to alleviate anxious emotions, and people may spend excessive amounts of time on online games as a coping mechanism to escape from the reality of their worries and difficulties (24–26). However, online gaming does not actually solve the problem, and wasting too much time on games makes things worse. A vicious cycle may further exacerbate psychological distress in people with IGD. Therefore, there is a need to implement targeted interventions to improve intervention efficiency and save medical resources.
Many intervention methods have been proven effective for anxiety. Cognitive behavioral therapy is the most experienced and effective psychosocial therapy for treating social phobia in adults and children (68); furthermore, the well-known Gestalt therapy is also effective in reducing anxiety in elementary school children (69). Finally, parent–child interaction therapy can prevent IGD by reducing the child’s level of anxiety by establishing a good parent–child relationship (70, 71).
4.3. Limitations
Several limitations should be pointed out in the present study. First, since cross-sectional data were used in our research and the relationships in networks were bidirectional, the determination of causality needs further support from longitudinal research. Second, the sample scope was limited to students in elementary school. Although we aimed to detect and intervene as early as possible, the results may not be suitable for generalization to older children. Finally, there was no control group, and the comparison of networks of students in lockdown and those in school may provide more meaningful findings.
5. Conclusion
In conclusion, the visual network structure provided a delicate description of the correlation pathways between anxiety and IGD; the BEI comparison helped to determine the bridges between anxiety and IGD and recommended social phobia and panic disorder as the potential targets for intervention of IGD. This study represents the first application of network analysis to explore the relationships between anxiety and IGD in children during COVID-19 lockdown and provides a reliable reference for the practice of psychological intervention.
Data availability statement
The raw data supporting the conclusions of this article will be made available by the authors, without undue reservation.
Ethics statement
The studies involving human participants were reviewed and approved by the Ethics Committee of Xijing Hospital of Air Force Medical University (Project No. CHiCTR1800019761). Written informed consent to participate in this study was provided by the participants’ legal guardian/next of kin.
Author contributions
TY, SJW, TX, and BW: concept and design. YY, SMW, RD, and ZP: acquisition of the data. TY, JL, and MY: analysis and interpretation of the data. TY, YH, CH, and LW: drafting of the manuscript. XL and SJW: critical revision of the manuscript. All authors contributed to the article and approved the submitted version.
Funding
This study was funded by Air Force Medical University (18CXZ012, 2019rcfcwsj, and BKJ21J013).
Acknowledgments
We sincerely thank all the students and their parents who contributed to our research.
Conflict of interest
The authors declare that the research was conducted in the absence of any commercial or financial relationships that could be construed as a potential conflict of interest.
Publisher’s note
All claims expressed in this article are solely those of the authors and do not necessarily represent those of their affiliated organizations, or those of the publisher, the editors and the reviewers. Any product that may be evaluated in this article, or claim that may be made by its manufacturer, is not guaranteed or endorsed by the publisher.
Supplementary material
The Supplementary Material for this article can be found online at: https://www.frontiersin.org/articles/10.3389/fpsyt.2023.1144413/full#supplementary-material
Supplementary Figure 1 | Accuracy test of edge weights in the anxiety-IGD network. The red line indicates the sample edge weight values and the gray area indicates the bootstrapped confidence intervals. A1, separation anxiety; A2, social phobia; A3, panic disorder; A4, physical injury fear; A5, generalized anxiety; IGD1, preoccupation; IGD2, tolerance; IGD3, giving up other activities; IGD4, continuing despite problems; IGD5, escape; IGD6, negative consequences; IGD7, loss of control; IGD8, deception; IGD9, withdrawal.
Supplementary Figure 2 | Bootstrapped difference test of edge weights in the anxiety-IGD network. The black box indicates that the edge weights of the two corresponding node pairs have a significant difference, the gray box indicates no significant difference. Blue and red boxes on the diagonal correspond to edge weights with positive and negative correlations, respectively. A1, separation anxiety; A2, social phobia; A3, panic disorder; A4, physical injury fear; A5, generalized anxiety; IGD1, preoccupation; IGD2, tolerance; IGD3, giving up other activities; IGD4, continuing despite problems; IGD5, escape; IGD6, negative consequences; IGD7, loss of control; IGD8, deception; IGD9, withdrawal.
Supplementary Figure 3 | Bootstrapped difference test of bridge expected influences in the anxiety-IGD network. The black box indicates that the bridge expected influences of the two corresponding nodes have a significant difference, the gray box indicated no significant difference. A1, separation anxiety; A2, social phobia; A3, panic disorder; A4, physical injury fear, A5, generalized anxiety, IGD1, preoccupation; IGD2, tolerance; IGD3, giving up other activities; IGD4, continuing despite problems; IGD5, escape; IGD6, negative consequences; IGD7, loss of control; IGD8, deception; IGD9, withdrawal.
Supplementary Figure 4 | Stability of bridge expected influences in the anxiety-IGD network. The red bar represents the average correlation between bridge expected influences in the full sample and subsample with the red area depicting the 2.5th quantile to the 97.5th quantile.
Abbreviations
COVID-19, coronavirus disease 2019; IGD, internet gaming disorder; SCAS-S, Spence Children’s Anxiety Scale-Short Version; IGDS, Internet Gaming Disorder Scale; GGM, Gaussian graphical model; LASSO, least absolute shrinkage and selection operator; EBIC, extended Bayesian information criterion; BEI, bridge expected influence; CS-coefficient, correlation stability coefficient.
References
1. CNNIC,. China internet network information center releases the 46th statistical report on the development status of China’s internet. Natl Libr J. (2021) 19.
2. Wu S, Wu J, Wang H, Li C, Li Y, Nie W, et al. Analysis of the current situation and influencing factors of Chinese elementary school students’ online behavior. Chin J Sch Health. (2020) 41:704–8.
3. Dong Q, Lin C. Key indicators and assessment of psychological development of children and adolescents aged 6-15 years in China. Beijing: Science Press (2011).
4. Kim E, Namkoong K, Ku T, Kim S. The relationship between online game addiction and aggression, self-control and narcissistic personality traits. Eur Psychiatry. (2008) 23:212–8. doi: 10.1016/j.eurpsy.2007.10.010
5. Lai L, Huang S, Zhang C, Tang B, Zhang M, Zhu C, et al. The association of cell phone addiction with interpersonal subjective well-being and school identity among primary and secondary school students. Chin J Sch Health. (2020) 41:139–42.
6. Casey B, Caudle K. The teenage brain: Self control. Curr Dir Psychol Sci. (2013) 22:82–7. doi: 10.1177/0963721413480170
7. Wang Y, Wu L, Wang L, Zhang Y, Du X, Dong G. Impaired decision-making and impulse control in Internet gaming addicts: Evidence from the comparison with recreational Internet game users. Addict Biol. (2016) 22:1610–21. doi: 10.1111/adb.12458
8. Shan X, Ou Y, Ding Y, Yan H, Chen J, Zhao J, et al. Associations between internet addiction and gender, anxiety, coping styles and acceptance in university freshmen in South China. Front Psychiatry. (2021) 12:558080. doi: 10.3389/fpsyt.2021.558080
9. Hwang H, Hong J, Kim S, Han D. The correlation between family relationships and brain activity within the reward circuit in adolescents with internet gaming disorder. Sci Rep. (2020) 10:9951. doi: 10.1038/s41598-020-66535-3
10. Rumpf H, Achab S, Billieux J, Bowden-Jones H, Carragher N, Demetrovics Z, et al. Including gaming disorder in the ICD-11: The need to do so from a clinical and public health perspective. J Behav Addict. (2018) 7:556–61. doi: 10.1556/2006.7.2018.59
11. World Health Organization [WHO]. Public health implications of excessive use of the Internet, computers, smartphones and similar electronic devices: Meeting report. Geneva: World Health Organization (2015).
12. American Psychiatric Association [APA]. Diagnostic and statistical manual of mental disorders. 5th ed. Arlingon, VA: American Psychiatric Publishing (2013).
13. Fazeli S, Zeidi I, Lin C, Namdar P, Griffiths M, Ahorsu D, et al. Depression, anxiety, and stress mediate the associations between internet gaming disorder, insomnia, and quality of life during the COVID-19 outbreak. Addict Behav Rep. (2020) 12:100307. doi: 10.1016/j.abrep.2020.100307
14. Han D, Kim S, Bae S, Renshaw P, Anderson J. Brain connectivity and psychiatric comorbidity in adolescents with Internet gaming disorder. Addict Biol. (2015) 22:802–12. doi: 10.1111/adb.12347
15. Kim J, LaRose R, Peng W. Loneliness as the cause and the effect of problematic Internet use: The relationship between Internet use and psychological well-being. CyberPsychology Behav. (2009) 12:451–5. doi: 10.1089/cpb.2008.0327
16. Lemmens J, Valkenburg P, Peter J. Psychosocial causes and consequences of pathological gaming. Comput Hum Behav. (2010) 27:144–52. doi: 10.1016/j.chb.2010.07.015
17. Liang L, Zhou D, Yuan C, Shao A, Bian Y. Gender differences in the relationship between internet addiction and depression: A cross-lagged study in Chinese adolescents. Comput Hum Behav. (2016) 63:463–70. doi: 10.1016/j.chb.2016.04.043
18. Yao M, Zhong Z. Loneliness, social contacts and Internet addiction: A cross-lagged panel study. Comput Hum Behav. (2014) 30:164–70. doi: 10.1016/j.chb.2013.08.007
19. Rehbein F, Psych G, Kleimann M, Mediasci G, Mößle T. Prevalence and risk factors of video game dependency in adolescence: Results of a German nationwide survey. Cyberpsychol Behav Soc Netw. (2010) 13:269–77. doi: 10.1089/cyber.2009.0227
20. Seo M, Kang H, Yom Y. Internet addiction and interpersonal problems in Korean adolescents. Comput Inform Nurs. (2009) 27:226–33. doi: 10.1097/ncn.0b013e3181a91b3f
21. Toker S, Baturay M. Antecedents and consequences of game addiction. Comput Hum Behav. (2016) 55:668–79. doi: 10.1016/j.chb.2015.10.002
22. Bonnaire C, Baptista D. Internet gaming disorder in male and female young adults: The role of alexithymia, depression, anxiety and gaming type. Psychiatry Res. (2019) 272:521–30. doi: 10.1016/j.psychres.2018.12.158
23. Stavropoulos V, Adams B, Beard C, Dumble E, Trawley S, Gomez R, et al. Associations between attention deficit hyperactivity and internet gaming disorder symptoms: Is there consistency across types of symptoms, gender and countries? Addict Behav Rep. (2019) 9:100158. doi: 10.1016/j.abrep.2018.100158
24. Elhai J, Gallinari E, Rozgonjuk D, Yang H. Depression, anxiety and fear of missing out as correlates of social, non-social and problematic smartphone use. Addict Behav. (2020) 105:106335. doi: 10.1016/j.addbeh.2020.106335
25. Elhai J, McKay D, Yang H, Minaya C, Montag C, Asmundson G. Health anxiety related to problematic smartphone use and gaming disorder severity during COVID-19: Fear of missing out as a mediator. Hum Behav Emerg Technol. (2021) 3:137–46. doi: 10.1002/hbe2.227
26. Wartberg L, Zieglmeier M, Kammerl R. An empirical exploration of longitudinal predictors for problematic internet use and problematic gaming behavior. Psychol Rep. (2021) 124:543–54. doi: 10.1177/0033294120913488
27. Wang Y, Liu B, Zhang L, Zhang P. Anxiety, depression, and stress are associated with internet gaming disorder during COVID-19: Fear of missing out as a mediator. Front Psychiatry. (2022) 13:827519. doi: 10.3389/fpsyt.2022.827519
28. Elhai J, Yang H, McKay D, Asmundson GJG. COVID-19 anxiety symptoms associated with problematic smartphone use severity in Chinese adults. J Affect Disord. (2020) 274:576–82. doi: 10.1016/j.jad.2020.05.080
29. Pereira H, Fehér G, Tibold A, Esgalhado G, Costa V, Monteiro S. The impact of internet addiction and job satisfaction on mental health symptoms among a sample of Portuguese workers. Int J Environ Res Public Health. (2021) 18:6943. doi: 10.3390/ijerph18136943
30. Pourahmadi M. Covariance estimation: The GLM and regularization perspectives. Stat Sci. (2011) 26:369–87. doi: 10.1214/11-sts358
31. Beard C, Millner A, Forgeard M, Fried E, Hsu K, Treadway M, et al. Network analysis of depression and anxiety symptom relationships in a psychiatric sample. Psychol Med. (2016) 46:3359–69. doi: 10.1017/S0033291716002300
32. Epskamp S, Waldorp L, Mõttus R, Borsboom D. The gaussian graphical model in cross-sectional and time-series data. Multivar Behav Res. (2018) 53:453–80.
33. Guineau M, Jones P, Bellet B, McNally RJ. A network analysis of DSM-5 posttraumatic stress disorder symptoms and event centrality. J Trauma Stress. (2021) 34:654–64. doi: 10.1002/jts.22664
34. Epskamp S, Fried EI. A tutorial on regularized partial correlation networks. Psychol Methods. (2018) 23:617–34. doi: 10.1037/met0000167
35. Bringmann L, Eronen M. Don’t blame the model: Reconsidering the network approach to psychopathology. Psychol Rev. (2018) 125:606–15. doi: 10.1037/rev0000108
36. Bringmann L, Elmer T, Epskamp S, Krause R, Schoch D, Wichers M, et al. What do centrality measures measure in psychological networks? J Abnorm Psychol. (2019) 128:892–903. doi: 10.1037/abn0000446
37. Jones P, Ma R, McNally R. Bridge centrality: A network approach to understanding comorbidity. Multivar Behav Res. (2021) 56:353–67. doi: 10.1080/00273171.2019.1614898
38. Ahlen J, Vigerland S, Ghaderi A. Development of the spence children’s anxiety scale-short version (SCAS-S). J Psychopathol Behav Assess. (2018) 40:288–304. doi: 10.1007/s10862-017-9637-3
39. Spence SH. A measure of anxiety symptoms among children. Behav Res Ther. (1998) 36:545–66. doi: 10.1016/s0005-7967(98)00034-5
40. Pontes H, Griffiths M. Measuring DSM-5 internet gaming disorder: Development and validation of a short psychometric scale. Comput Hum Behav. (2015) 45:137–43. doi: 10.1016/j.chb.2014.12.006
41. Jiang S, Zeng H. Validity and reliability of two Chinese versions of Internet addiction subtype scales applied to primary and secondary school students. Chin Ment Health J. (2016) 30:936–8.
42. Tibshirani R. Regression shrinkage and selection via the lasso. J R Stat Soc B. (1996) 58:267–88. doi: 10.1111/j.2517-6161.1996.tb02080.x
43. Chen J, Chen Z. Extended Bayesian information criteria for model selection with large model spaces. Biometrika. (2008) 95:759–71. doi: 10.1093/biomet/asn034
44. Epskamp S, Borsboom D, Fried E. Estimating psychological networks and their accuracy: A tutorial paper. Behav Res Methods. (2018) 50:195–212. doi: 10.3758/s13428-017-0862-1
45. Foygel R, Drton M. Extended Bayesian information criteria for Gaussian graphical models. Adv Neural Inf Process Syst. (2010) 23:604–12.
46. Fruchterman T, Reingold E. Graph drawing by force-directed placement. Softw Pract Exp. (1991) 21:1129–64. doi: 10.1002/spe.4380211102
47. Epskamp S, Cramer A, Waldorp L, Schmittmann V, Borsboom D. qgraph: Network visualizations of relationships in psychometric data. J Stat Softw. (2012) 48:1–18. doi: 10.18637/jss.v048.i04
48. Isvoranu A, van Borkulo C, Boyette L, Wigman J, Vinkers C, Borsboom D, et al. A network approach to psychosis: Pathways between childhood trauma and psychotic symptoms. Schizophr Bull. (2017) 43:187–96. doi: 10.1093/schbul/sbw055
49. Morosan L, Fonseca-Pedrero E, Debbané M. Network analysis of reflective functioning and conduct problems during adolescence. Psychol Violence. (2020) 10:300–11. doi: 10.1037/vio0000258
50. Baldwin D, Gordon R, Abelli M, Pini S. The separation of adult separation anxiety disorder. CNS Spectr. (2016) 21:289–94. doi: 10.1017/s1092852916000080
51. Bowlby J. A secure base: Parent-child attachment and healthy human development. New York: Basic Books (1988).
52. Klinger E, Bouchard S, Légeron P, Roy S, Lauer F, Chemin I, et al. Virtual reality therapy versus cognitive behavior therapy for social phobia: A preliminary controlled study. CyberPsychol Behav. (2005) 8:76–88. doi: 10.1089/cpb.2005.8.76
53. Baker J, Hudson J. Children with social phobia have lower quality friendships than children with other anxiety disorders. Anxiety Stress Coping. (2014) 28:500–13. doi: 10.1080/10615806.2014.978863
54. Dahne J, Banducci A, Kurdziel G, MacPherson L. Early adolescent symptoms of social phobia prospectively predict alcohol use. J Stud Alcohol Drugs. (2014) 75:929–36. doi: 10.15288/jsad.2014.75.929
55. Grant B, Hasin D, Stinson F, Dawson D, Goldstein R, Smith S, et al. The epidemiology of DSM-IV panic disorder and agoraphobia in the United States. J Clin Psychiatry. (2006) 67:363–74. doi: 10.4088/jcp.v67n0305
56. Kwak K, Lee SJ. A comparative study of early maladaptive schemas in obsessive–compulsive disorder and panic disorder. Psychiatry Res. (2015) 230:757–62. doi: 10.1016/j.psychres.2015.11.015
57. Beesdo K, Knappe S, Pine D. Anxiety and anxiety disorders in children and adolescents: Developmental issues and implications for DSM-V. Psychiatr Clin North Am. (2009) 32:483–524. doi: 10.1016/j.psc.2009.06.002
58. Wang R, Wang W, daSilva A, Huckins J, Kelley W, Heatherton T, et al. Tracking depression dynamics in college students using mobile phone and wearable sensing. Proc ACM Interact Mob Wearable Ubiquitous Technol. (2018) 2:1–26. doi: 10.1145/3191775
59. Garcia R. Neurobiology of fear and specific phobias. Learn Mem. (2017) 24:462–71. doi: 10.1101/lm.044115.116
60. Wang F, Kream R, Stefano G. Long-term respiratory and neurological sequelae of COVID-19. Med Sci Monit. (2020) 26:e928996. doi: 10.12659/MSM.928996
61. Ohayon M, Roberts L. Internet gaming disorder and comorbidities among campus-dwelling U.S. university students. Psychiatry Res. (2021) 302:114043. doi: 10.1016/j.psychres.2021.114043
62. Tao Y, Hou W, Niu H, Ma Z, Zhang S, Zhang L, et al. Centrality and bridge symptoms of anxiety, depression, and sleep disturbance among college students during the COVID-19 pandemic-a network analysis. Curr Psychol. (2022): doi: 10.1007/s12144-022-03443-x [Epub ahead of print].
63. Borsboom D, Cramer A. Network analysis: An integrative approach to the structure of psychopathology. Annu Rev Clin Psychol. (2013) 9:91–121. doi: 10.1146/annurev-clinpsy-050212-185608
64. Wang Y, Hu Z, Feng Y, Wilson A, Chen R. Changes in network centrality of psychopathology symptoms between the COVID-19 outbreak and after peak. Mol Psychiatry. (2020) 25:3140–9. doi: 10.1038/s41380-020-00881-6c
65. Wei H, Chen M, Huang P, Bai Y. The association between online gaming, social phobia, and depression: An internet survey. BMC Psychiatry. (2012) 12:92. doi: 10.1186/1471-244X-12-92
66. Severo R, Soares J, Affonso J, Giusti D, de Souza Junior A, de Figueiredo V, et al. Prevalence and risk factors for internet gaming disorder. Braz J Psychiatry. (2020) 42:532–5. doi: 10.1590/1516-4446-2019-0760
67. Lo Y, Chen H, Huang S. Panic disorder correlates with the risk for sexual dysfunction. J Psychiatr Pract. (2020) 26:185–200. doi: 10.1097/pra.0000000000000460
68. Scharfstein L, Beidel D. Behavioral and cognitive-behavioral treatments for youth with social phobia. J Exp Psychopathol. (2011) 2:615–28. doi: 10.5127/jep.014011
69. Farahzadi M, Bahramabadi M, Mohammadifar M. Effectiveness of Gestalt play therapy in decreasing social phobia. Dev Psychol Iran Psychol. (2011) 7:387–95.
70. Gold D. Examination of group format parent-child interaction therapy adapted for anxiety disorders. Degree of Doctor of Philosophy. Hempstead, NY: Hofstra University (2017).
Keywords: anxiety, internet gaming disorder, children, COVID-19, network analysis
Citation: Yang T, He Y, He C, Yang Y, Wu L, Wei B, Dong R, Yang M, Pu Z, Wang S, Li J, Xu T, Liu X and Wu S (2023) The relationship between anxiety and internet gaming disorder in children during COVID-19 lockdown: a network analysis. Front. Psychiatry 14:1144413. doi: 10.3389/fpsyt.2023.1144413
Received: 14 January 2023; Accepted: 11 April 2023;
Published: 17 May 2023.
Edited by:
Lu Yu, Hong Kong Polytechnic University, Hong Kong SAR, ChinaReviewed by:
Toshinori Chiba, Advanced Telecommunications Research Institute International (ATR), JapanBingjie Liu, East China Normal University, China
Amy Zhou, Hong Kong Polytechnic University, Hong Kong SAR, China
Copyright © 2023 Yang, He, He, Yang, Wu, Wei, Dong, Yang, Pu, Wang, Li, Xu, Liu and Wu. This is an open-access article distributed under the terms of the Creative Commons Attribution License (CC BY). The use, distribution or reproduction in other forums is permitted, provided the original author(s) and the copyright owner(s) are credited and that the original publication in this journal is cited, in accordance with accepted academic practice. No use, distribution or reproduction is permitted which does not comply with these terms.
*Correspondence: Shengjun Wu, wushj@fmmu.edu.cn; Xufeng Liu, lxf_fmmu@126.com
†These authors have contributed equally to this work