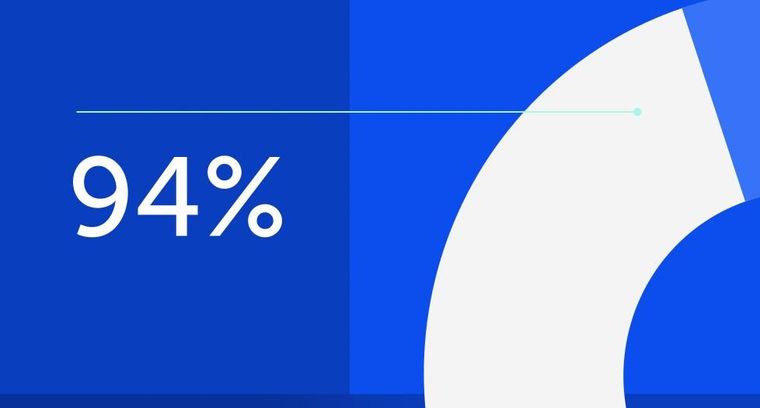
94% of researchers rate our articles as excellent or good
Learn more about the work of our research integrity team to safeguard the quality of each article we publish.
Find out more
ORIGINAL RESEARCH article
Front. Psychiatry, 12 May 2023
Sec. Mood Disorders
Volume 14 - 2023 | https://doi.org/10.3389/fpsyt.2023.1139742
Background: The COVID-19 pandemic has greatly affected treatment-seeking behaviors of psychiatric patients and their guardians. Barriers to access of mental health services may contribute to adverse mental health consequences, not only for psychiatric patients, but also for their guardians. This study explored the prevalence of depression and its association with quality of life among guardians of hospitalized psychiatric patients during the COVID-19 pandemic.
Methods: This multi-center, cross-sectional study was conducted in China. Symptoms of depression and anxiety, fatigue level and quality of life (QOL) of guardians were measured with validated Chinese versions of the Patient Health Questionnaire – 9 (PHQ-9), Generalized Anxiety Disorder Scale – 7 (GAD-7), fatigue numeric rating scale (FNRS), and the first two items of the World Health Organization Quality of Life Questionnaire - brief version (WHOQOL-BREF), respectively. Independent correlates of depression were evaluated using multiple logistic regression analysis. Analysis of covariance (ANCOVA) was used to compare global QOL of depressed versus non-depressed guardians. The network structure of depressive symptoms among guardians was constructed using an extended Bayesian Information Criterion (EBIC) model.
Results: The prevalence of depression among guardians of hospitalized psychiatric patients was 32.4% (95% CI: 29.7–35.2%). GAD-7 total scores (OR = 1.9, 95% CI: 1.8–2.1) and fatigue (OR = 1.2, 95% CI: 1.1–1.4) were positively correlated with depression among guardians. After controlling for significant correlates of depression, depressed guardians had lower QOL than non-depressed peers did [F(1, 1,101) = 29.24, p < 0.001]. “Loss of energy” (item 4 of the PHQ-9), “concentration difficulties” (item 7 of the PHQ-9) and “sad mood” (item 2 of the PHQ-9) were the most central symptoms in the network model of depression for guardians.
Conclusion: About one third of guardians of hospitalized psychiatric patients reported depression during the COVID-19 pandemic. Poorer QOL was related to having depression in this sample. In light of their emergence as key central symptoms, “loss of energy,” “concentration problems,” and “sad mood” are potentially useful targets for mental health services designed to support caregivers of psychiatric patients.
The coronavirus disease 2019 (COVID-19) was first reported in Wuhan, Hubei province of China at the end of 2019 and subsequently emerged in other parts of the world (1, 2). Notwithstanding its negative impact on the health and security of humanity, the COVID-19 pandemic has also had pronounced effects on mental health status and quality of life (QOL) in various populations (3–5).
In times of pandemics, people with mental disorders are more vulnerable to respiratory tract infections (6). Possible correlates of this risk include higher smoking rates, poor personal hygiene and negligence of infection risks due to cognitive impairment as well as crowded living conditions and lack of personal protective equipment (PPE) in psychiatric wards (6–9). As a result of such factors, it is reasonable to hypothesize that hospitalized psychiatric patients are more susceptible to COVID-19. Indeed, this contention was supported early in the pandemic when at least 50 hospitalized psychiatric patients and 30 mental health professionals in a major psychiatric hospital in Wuhan, China were diagnosed with COVID-19 in early 2020 (7, 10). Additionally, a study based on electronic health records in the United States found that patients with psychiatric disorders had a higher risk for COVID-19 infection than those without psychiatric disorders (adjusted OR = 7.64 for depression; adjusted OR = 7.34 for schizophrenia) (11). Two other studies in Spain found that 45% of COVID-19 inpatients had history of psychiatric disorders and 37% of COVID-19 inpatients had medical conditions (12, 13), supporting the view that psychiatric patients are more prone to COVID-19.
In order to minimize infection risk, policies to prevent unnecessary visits and social contacts in hospitals and psychiatric wards were implemented and multiple clinical services were curtailed during early stages of the COVID-19 pandemic (8, 14–16). Psychiatric patients and their guardians have been confronted with numerous barriers in accessing mental health services during the COVID-19 pandemic including difficulties in visiting psychiatrists, reduced access to psychotropic medications and hospital admissions, and problems with evaluating degree of compliance with recommended treatment protocols (8, 16, 17). All of these barriers to optimal psychiatric care could increase risk for depression and reduced quality of life (QOL), not only among patients but also among their guardians. Previous studies have revealed that guardians of adolescents with Type 1 diabetes and isolated COVID-19 patients suffered from higher levels of depression, anxiety and pandemic-related worry compared to adults who did not have family members who were ill during the COVID-19 pandemic (18–20); these findings underscore the importance of considering the mental health status of guardians who must care for psychiatric patients and undertake relevant obligations during the COVID-19 pandemic.
To date, the impact of the COVID-19 pandemic on the mental health status of psychiatric patients has been widely investigated (21–24). In contrast, there has been a paucity of research on the mental health status and QOL of guardians of the hospitalized psychiatric patients. Documenting the prevalence of depression as well as its correlates and association with QOL among guardians of psychiatric patients during the pandemic is important for ensuring close support systems of patients are maintained and distressed caregivers also have access to interventions that reduce their own suffering.
Traditionally, epidemiological research on depression has adopted a latent factor approach (25) in which depression is regarded as an unobservable, latent factor and depressive symptoms are observable manifestations or indicators of depression (26). Key assumptions underlying the latent factor approach are that all symptoms are present or dependent upon one another and equally important in their contributions to overall depression levels (25, 26). However, symptoms such as anhedonia, hopelessness and reduced energy often have robust associations with each other even when diagnostic criteria for MDD are not fulfilled (27, 28). Such data highlight how traditional latent factor approaches cannot elucidate inter-relationships between different depressive symptoms although individual symptoms may play an important role in the onset and maintenance of depression (29, 30). As an alternative to the traditional perspective, a network approach may provide more understanding of how depressive symptoms are interconnected, particular symptoms that are most influential for the syndrome within particular populations (31–33).
Based on the preceding overview, the initial aim of this study was to document the prevalence of depression, its correlates, and its association with QOL among guardians of hospitalized psychiatric patients during the COVID-19 pandemic. In addition, we used network analysis to generate a network model of inter-relations between specific depressive symptoms within this understudied group.
This multi-center, cross-sectional study was conducted between May 24, 2020 and January 18, 2021 in seven tertiary psychiatric hospitals and psychiatric units of general hospitals in China. To avoid COVID-19 infection risk, data were collected using the WeChat-based QuestionnaireStar application as recommended in previous studies (34, 35). Guardians needed to declare their health status using WeChat during the COVID-19 pandemic when they entered participating hospitals. Therefore, all guardians were presumed to be WeChat users. Guardians who visited hospitalized patients during the study period were consecutively invited to participate. Inclusion criteria were as follows: (1) age 18 years or older; (2) ability to read Chinese and understand the purpose and contents of the assessments; (3) status as a guardian (e.g., spouse, child, parent, other kin or friend) of a hospitalized psychiatric patient in participating hospitals; (4) provision of online electronic informed consent. Guardians with a psychiatric history or current psychiatric disorders were excluded from this study since this was a possible confounding factor to estimating depression prevalence for guardians as a population distinct from psychiatric patients. The study protocol was centrally approved by the research ethics committee of Beijing Anding Hospital, Capital Medical University and other participating hospitals.
The data collection form was designed using the QuestionnaireStar application. A Quick Response (QR) code linked to the informed consent and data collection form was scanned by the participants with their smart phones. Those who met eligibility criteria completed the assessment in participating hospitals on a voluntary, anonymous basis.
Socio-demographic data assessed included age, gender, marital status, employment status, education level, urban versus rural residence, presence of chronic physical diseases, perceived financial status, frequency of social media use during the COVID-19 pandemic, and experience of difficulty in visiting mental health services during the pandemic.
Severity of depressive symptoms was assessed using the validated Chinese version of the Patient Health Questionnaire – 9 (PHQ-9). The PHQ-9 consists of nine items, each rated on a frequency scale from 0 (not at all) to 3 (almost every day) (36, 37). Higher PHQ-9 scores represent more severe depression (38). The reliability and validity of the PHQ-9 are satisfactory in Chinese populations (39, 40). Participants were regarded “having clinically relevant depression” (having depression hereafter) if their total PHQ-9 score was ≥5 (38).
Severity of anxiety symptoms was assessed using the Chinese version of the Generalized Anxiety Disorder Scale – 7 (GAD-7). The GAD-7 consists of seven self-report items, each of which is rated on a frequency scale from 0 (not at all) to 3 (almost every day) (41); higher GAD-7 scores reflect more severe anxiety symptoms. The GAD-7 Chinese version has been validated in Chinese populations (42, 43). Level of fatigue was evaluated using a single-item fatigue numeric rating scale (FNRS) (44). FNRS scores range from 0 (no fatigue) to 10 (extreme fatigue).
Global quality of life (QOL) was assessed with the first two items of the World Health Organization Quality of Life Questionnaire - brief version (WHOQOL-BREF) (45, 46). These items queried overall quality of life and general health status from 1 (extremely unsatisfied) to 5 (extremely satisfied) (47). This two-item QOL index has been validated and used widely in Chinese samples (48).
All data analyses were conducted using Statistical Analysis System (SAS) OnDemand for Academics (SAS Institute Inc., Cary, NC, United States) and R version 4.2.1 (49). Sociodemographic and emotional status differences between depressed versus non-depressed guardian subgroups were assessed using independent two-sample t-tests, Wilcoxon rank sum tests, and chi-square tests, as appropriate. Analysis of covariance (ANCOVA) was used to compare global QOL score differences between depressed versus non-depressed guardians after first controlling the impact of other measures on which there were subgroup differences in univariable analyses (i.e., covariates). Independent predictors of depression levels were evaluated using a multiple logistic regression analysis; depression was the dependent variable, and significant univariate correlates of depression subgroup status were predictors in the analysis. Age and sex are generally associated with mental health status and QOL in many populations (50); therefore, they were included as potential predictors in the multiple logistic regression model, even though neither had significant associations with depression in univariate analyses. In addition, independent predictors of depression were explored separately for first-degree relatives (spouse, children, and parents). Two-sided p-values lower than 0.05 were considered to be statistically significant.
To capture the full spectrum of depression severity and increase external validity, the network structure of depressive symptoms was constructed for all guardians of hospitalized psychiatric patients rather than only the depressed guardians, as recommended by previous studies (51, 52). An extended Bayesian Information Criterion (EBIC) model graphical least absolute shrinkage and selection operator (gLASSO) network model was adopted in this study. In the network structure, each individual symptom was a “node,” and connections between symptoms were “edges.” The centrality of each symptom was measured using strength, defined as the sum of the absolute weights of the edges connecting a certain node to all the other nodes. The size of a node represented the strength of a particular symptom. The thickness of each edge represented the strength of the association between two nodes. The color of an edge reflected the direction of the association with green edges indicating positive associations and red edges indicating negative associations between nodes.
Network stability was examined via the correlation stability coefficient (CS-C) using a case-dropping 1,000-time bootstrap method (53, 54). Preferably, a CS-C exceeds 0.5, with a minimum value requirement of 0.25 (55).
To examine the impact of anxiety symptoms and fatigue on the observed network structure of depressive symptoms, the network model of depression was re-estimated after adjusting for anxiety symptoms and fatigue. A flow network was applied to investigate relationships between individual depressive symptoms and QOL. R packages used in this study were networktools version 1.2.3 (56), bootnet version 1.4.3 (55), qgraph version 1.6.5 (57), NetworkComparisonTest version 2.2.1 (58, 59), and mgm version 1.2–10 (60).
In total, 1,163 guardians of hospitalized psychiatric patients were invited to participate in this study; of these, 1,101 (94.7%) agreed to participate, fulfilled the eligibility criteria, and completed the assessment. Table 1 presents demographics and clinical characteristics of final guardian sample. The prevalence of depression among guardians of hospitalized psychiatric patients was 32.4% (95% CI: 29.7–35.2%).
Compared to their non-depressed peers, guardians with depression were more likely to report poorer financial status, difficulty visiting a mental health service during the pandemic, increased fatigue, and elevations in anxiety symptoms. Depressed guardians were also significantly less likely to report that their loved ones showed good compliance with medication during the pandemic and had a lower mean overall QOL level (all p-values<0.05; see Table 1). In contrast, depressed versus non-depressed guardian subgroups did not differ on any demographic measure.
After adjusting for other significant correlates of depression status, guardians with depression continued to have a significantly lower mean QOL level than non-depressed guardians had [F(1, 1,101) = 29.24, p < 0.001].
The multiple logistic regression analysis indicated higher total GAD-7 scores (OR = 1.9, 95% CI: 1.8–2.1) and fatigue scores (OR = 1.2, 95% CI: 1.1–1.4) were the only unique, statistically significant predictors of elevated depression levels within the guardian sample (see Table 2). In a subgroup analysis of first-degree relative guardians, findings were similar to those for the whole sample (see Supplementary Table S1).
Table 2. Independent correlates of depression among guardians of hospitalized psychiatric patients during the COVID-19 pandemic (N = 1,101).
The network structure of depressive symptoms, as estimated with the EBIC glasso model, is shown in Figure 1. PHQ-9 items 4 (DEP-4, loss of energy), 7 (DEP-7, concentration difficulties), and 2 (DEP-2, sad mood) had the highest strengths in the network model of depressive symptoms. Exact centrality strength values are shown in Supplementary Table S2. The CS-C for network model strength was 0.75, indicating that centrality strength values in the network remained stable after dropping 75% of the sample (Figure 2).
Figure 1. Network structure and strength of the depressive symptoms among guardians of hospitalized psychiatric patients (N = 1,101).
Figure 2. Network stability of depressive symptoms among guardians of hospitalized psychiatric patients (N = 1,101).
The re-estimated network structure of depressive symptoms after adjusting for anxiety symptoms and fatigue is shown in Figure 3. Nodes with three highest strengths in the adjusted network (Figure 3) were identical to those in the unadjusted network (Figure 1), suggesting that neither anxiety symptoms nor fatigue had a significant influence on the initial network model. Exact centrality strengths in the adjusted network model are shown in Supplementary Table S3. The flow network of depressive symptoms and QOL indicated PHQ-9 items 6 (DEP-6, guilt feelings), 7 (DEP-7, concentration difficulties) and 3 (DEP-3, sleep problems) were strongly connected with global QOL within the overall guardian sample (Figure 4). The weighted adjacency matrix of the network for global QOL and depressive symptoms was shown in Supplementary Table S4.
Figure 3. Network structure and strength of depressive symptoms among guardians of hospitalized psychiatric patients after adjusting for anxiety symptoms and fatigue (N = 1,101).
Figure 4. Flow network of QOL and depressive symptoms among guardians of hospitalized psychiatric patients (N = 1,101).
Supplementary subgroup network analyses showed that the network features in depressed guardians were similar to those found for the whole sample (Supplementary Figures S1, S2).
To our knowledge, this is the first study to explore the prevalence of depression and its association with QOL among guardians of hospitalized psychiatric patients during the COVID-19 pandemic. The prevalence of depression among guardians was 32.4%. Since no prevalence data from previous studies of guardians of hospitalized psychiatric patients could be identified, it is not entirely clear whether the rate in this sample was elevated relative to related comparison groups. However, previous COVID-19 pandemic era studies (61, 62) on guardians to assisted living residents and guardians to persons with neurocognitive disorders reported rates of depression (38.8% and 36.3% respectively) similar to those of the present study. Given that approximately one third of guardians experienced depression across these three studies, depression among caregivers of vulnerable patient groups appears to be a noteworthy yet overlooked mental health problem during the COVID-19 pandemic.
The relatively high prevalence of depression among guardians in this study could be attributed to several reasons. First, the closure of clinical psychiatric services during the early COVID-19 pandemic phase could have contributed acute patient crises (8, 63), including difficulties in visiting psychiatrists, reduced access to psychotropic medications, and/or barriers in maintaining medication compliance, all of which could exacerbate distress in patients as well as concerned family members including guardians. Second, news reports of increased nosocomial infections of COVID-19 within psychiatric hospitals could have aggravated guardians’ pandemic-related worries (10). Third, cancellations of routine family visits to hospitals during the COVID-19 pandemic increased uncertainty about care for both patients and guardians. Finally, the PHQ-9 cutoff we adopted to identify depressed status may have contributed to this rate and is not necessarily identical to prevalence estimates that might be garnered from structured diagnostic interviews.
With respect to unique predictors of depression among guardians in our sample, higher GAD-7 total scores were positively correlated with depression scores. This finding aligns with previous studies indicating anxiety and depression are frequently comorbid with each other (64, 65). To elaborate, a worldwide survey reported that almost 46% of patients with a lifetime prevalence of major depressive disorder (MDD) also have a lifetime history of anxiety disorder (66). Data from the Sequenced Treatment Alternatives to Relieve Depression (STAR*D) study found 53% of patients with MDD had significant concurrent anxiety symptoms (67). Depression and anxiety are also intertwined with one another over time (68); the presence of one condition may predispose the vulnerable to the other condition (69). Supporting biological foundations of comorbidity, genetic epidemiological studies suggest that depression and anxiety have a shared genetic etiology (70–73).
High levels of fatigue also emerged as a unique correlate of elevated depression scores in our sample. Paralleling comorbidity evidence for anxiety, fatigue is often viewed as comorbid with depression and is highly prevalent in a cluster of depressive symptoms (74–76), particularly within East-Asian samples who may somatize depressive symptoms (77). Neural pathway studies have also found chronic fatigue and depression have shared neurobiological mechanisms (78, 79). In contrast to comorbidity interpretations, associations between depression and fatigue may be attributed to construct overlaps. Specifically, the diagnosis of depression and PHQ-9 include “loss of energy” as a criterion (36, 37) that overlaps with fatigue.
Network analysis indicated “loss of energy” (DEP-4) had the highest centrality strength in the structure of depressive symptoms in our guardian sample. This finding aligns with Hinz et al. (80) who reported “loss of energy” had the highest factor loading of any PHQ-9 item. In tandem, these results underscore the importance of loss of energy vis a vis other symptoms of depression. In community-based settings, “loss of energy” is frequently endorsed when people encounter depressing life events (81, 82). Conversely, in psychiatric samples, the most central symptom is often “sad mood” (83, 84). This discrepancy highlights potential differences in the expression of depression between psychiatric and non-psychiatric samples such as guardians in this study. “Loss of energy” may be more central to experiences of depression among guardians of hospitalized psychiatric patients, in part, due to adopting a less physically active lifestyle during lockdowns (85, 86) and/or increased stress associated with potentially heavier caregiving burdens related to fulfilling the guardian role during a pandemic (87).
“Concentration difficulties” (DEP-7) had the second highest strength centrality in the network of depressive symptoms in our guardian sample. “Sad mood” and “anhedonia” are conventionally accepted as two core symptoms of MDD, in contrast to our finding that “concentration difficulties” emerged as the second most influential depressive symptom in guardians of hospitalized psychiatric patients. This could be explained, in part, by the fact that the PHQ-9 is a screening measure on depressive symptoms based on continuous severity ratings, rather than an MDD diagnosis. Nonetheless, more influential symptoms in the network model of depressive symptoms based on the PHQ-9 assessment align with symptoms of MDD based on DSM criteria as well as research based on samples with similar characteristics. Specifically, our centrality influence findings are consistent with a previous study in which individuals with an external locus of attribution were more vulnerable to concentration problems than those with an internal locus of attribution (88). A comparatively stronger external orientation may help to explain the centrality of “concentration difficulties” (DEP-7) in the network model of depressive symptoms among guardians since extra guardianship and caregiving responsibilities of this group may have increased the likelihood of emphasizing external influences as causes of stress experiences. Moreover, concentration problems may be more prominent when levels of depression severity are low (89); presumably, a majority of guardians in our study sample did not experience severe depression in light of the need for considerable competence in undertaking their role. Our data suggest that “concentration difficulties” could be an important yet easily overlooked indicator in populations that experience stress and undertake guardianship or caregiving responsibilities.
“Sad mood” (DEP-2) had the third highest strength centrality in the network model of depression among guardians in this study. This finding converges with evidence from Cheung et al.’s (90) network structure study of depressive symptoms in a community sample from Hong Kong during the COVID-19 pandemic, Hartung et al.’s (91) network analysis of PHQ-9 items in a sample from the general population in Germany, and Fried et al.’s (92) study of depressive symptomatology in outpatients with MDD. Despite differences in sample characteristics, these studies highlight sad mood as a central symptom of depression even when samples are relatively high functioning.
Finally, after adjusting for significant correlates of depression including anxiety and fatigue, depressed guardians had significantly lower QOL levels than their non-depressed peers did. The negative depression-QOL association appears to be robust given that it has also been observed in other populations including community-dwellers, older persons, and patients with cancer (93–96). From a symptom-level perspective, “guilt feelings” (DEP-6), “concentration difficulties” (DEP-7) and “sleep problems” (DEP-3) had the strongest associations with global QOL in our guardian sample. As such, these symptoms could be useful targets for interventions designed to alleviate depression and improve QOL in this population.
Strengths of this study included its relatively large sample size, multi-center study design, and adoption of both a broad epidemiological perspective and a symptom-level perspective to evaluate depressive symptoms within an understudied population involved in the care of patients with psychiatric disorders. However, the study also had several methodological limitations. First, because a cross-sectional design was used, the time course of depression and changes in the expression of individual depressive symptoms over different phases of the pandemic could not be elucidated. On a related note, pre-versus post-pandemic rates of depression and network models could not be assessed due to the cross-sectional design and initiation of this study only after the COVID-19 pandemic had begun. Third, the network structure of depression was limited to PHQ-9 items so it is possible that the network structure might differ based on a different depression questionnaire or interview-based assessment. Fourth, although WeChat is widely used in China and all guardians were presumed to be WeChat users, recruitment based on consecutive (i.e., non-probability sampling) rather than random sampling, is more prone to selection biases. Finally, it is not clear how well our findings extend to guardian samples in other countries that have experienced high levels of morbidity and mortality from COVID-19 and have adopted different policies for managing the pandemic.
In conclusion, this study found approximately 1/3 of guardians of hospitalized psychiatric patients in China reported depression during the COVID-19 pandemic. Anxiety and fatigue emerged as unique correlates of depression in the sample. “Loss of energy” (DEP-4), “concentration difficulties” (DEP-7), and “sad mood” (DEP-2) were the most influential symptoms in the associated network model. These symptoms could be valuable targets in treatments for depression while strategies to reduce sleep problems and guilt may aid in improving QOL of guardians.
The datasets presented in this article are not readily available because the Research Ethics Committee of Beijing Anding Hospital that approved the study prohibits the authors from making publicly available the research dataset of clinical studies. Requests to access the datasets should be directed to xyutly@gmail.com.
The studies involving human participants were reviewed and approved by Research Ethics Committee of Beijing Anding Hospital, Capital Medical University. The patients/participants provided their electronic written informed consent to participate in this study.
Y-JZ, LZ, YF, SS, and Y-TX: study design. Y-JZ, LZ, YF, SS, ML, Y-YW, J-XL, ZS, TC, and GU: data collection, analysis, and interpretation. Y-JZ and Y-TX: drafting of the manuscript. TJ: critical revision of the manuscript. All authors contributed to the article and approved the submitted version.
This study was supported by Beijing Hospitals Authority Youth Programme (code: 1-1-2-2-xm202301-01), Beijing Municipal Science & Technology Commission (No. Z181100001518005), the National Science and Technology Major Project for investigational new drug (2018ZX09201-014), the Beijing Hospitals Authority Clinical Medicine Development of special funding support (XMLX202128), and the University of Macau (MYRG2019-00066-FHS and MYRG2022-00187-FHS).
The authors are grateful to all participants and clinicians involved in this study.
The authors declare that the research was conducted in the absence of any commercial or financial relationships that could be construed as a potential conflict of interest.
All claims expressed in this article are solely those of the authors and do not necessarily represent those of their affiliated organizations, or those of the publisher, the editors and the reviewers. Any product that may be evaluated in this article, or claim that may be made by its manufacturer, is not guaranteed or endorsed by the publisher.
The Supplementary material for this article can be found online at: https://www.frontiersin.org/articles/10.3389/fpsyt.2023.1139742/full#supplementary-material
1. World Health Organization. Naming the coronavirus disease (COVID-19) and the virus that causes it. Available at: https://wwwwhoint/emergencies/diseases/novel-coronavirus-2019/technical-guidance/naming-the-coronavirus-disease-(covid-2019)-and-the-virus-that-causes-it (Accessed February 11, 2020) (2020).
2. World Health Organization. Novel coronavirus – China. Availabel at: (https://wwwwhoint/csr/don/12-january-2020-novel-coronavirus-china/en/) (2020).
3. Zhao, YJ, Zhang, SF, Li, W, Zhang, L, Guo, T, Cheung, T, et al. Associations between depressive symptoms and quality of life among residents of Wuhan, China during the later stage of the COVID-19 pandemic: a network analysis. J Affect Disord. (2022) 318:456–64. doi: 10.1016/j.jad.2022.08.104
4. Kılınçel, Ş, Kılınçel, O, Muratdağı, G, Aydın, A, and Usta, MB. Factors affecting the anxiety levels of adolescents in home-quarantine during Covid-19 pandemic in Turkey. Asia Pac Psychiatry. (2021) 13:e12406. doi: 10.1111/appy.12406
5. Li, T, Sun, S, Liu, B, Wang, J, Zhang, Y, Gong, C, et al. Prevalence and risk factors for anxiety and depression in patients with Covid-19 in Wuhan. China Psychosom Med. (2021) 83:368–72. doi: 10.1097/psy.0000000000000934
6. Seminog, OO, and Goldacre, MJ. Risk of pneumonia and pneumococcal disease in people with severe mental illness: English record linkage studies. Thorax. (2013) 68:171–6. doi: 10.1136/thoraxjnl-2012-202480
7. Xiang, YT, Zhao, YJ, Liu, ZH, Li, XH, Zhao, N, Cheung, T, et al. The COVID-19 outbreak and psychiatric hospitals in China: managing challenges through mental health service reform. Int J Biol Sci. (2020) 16:1741–4. Epub 2020/04/01. doi: 10.7150/ijbs.45072
8. Li, L. Challenges and priorities in responding to Covid-19 in inpatient psychiatry. Psychiatr Serv. (2020) 71:624–6. doi: 10.1176/appi.ps.202000166
9. Shinn, AK, and Viron, M. Perspectives on the Covid-19 pandemic and individuals with serious mental illness. J Clin Psychiatry. (2020) 81. doi: 10.4088/JCP.20com13412
10. China News Weekly. Hospital-acquired infection in Wuhan mental health center: around 80 medical staff and patients were diagnosed with 2019-Ncov pneumonia (in Chinese). Available at: (https://newssinacomcn/c/2020-02-08/doc-iimxxste9892538shtml) (2020).
11. Wang, Q, Xu, R, and Volkow, ND. Increased risk of COVID-19 infection and mortality in people with mental disorders: analysis from electronic health records in the United States. World Psychiatry. (2021) 20:124–30. doi: 10.1002/wps.20806
12. Iftimie, S, López-Azcona, AF, Lozano-Olmo, MJ, Hernández-Aguilera, A, Sarrà-Moretó, S, Joven, J, et al. Characteristics of hospitalized patients with Sars-Cov-2 infection during successive waves of the Covid-19 pandemic in a reference Hospital in Spain. Sci Rep. (2022) 12:17384. doi: 10.1038/s41598-022-22145-9
13. Diez-Quevedo, C, Iglesias-González, M, Giralt-López, M, Rangil, T, Sanagustin, D, Moreira, M, et al. Mental disorders, psychopharmacological treatments, and mortality in 2150 COVID-19 Spanish inpatients. Acta Psychiatr Scand. (2021) 143:526–34. doi: 10.1111/acps.13304
14. Bojdani, E, Rajagopalan, A, Chen, A, Gearin, P, Olcott, W, Shankar, V, et al. COVID-19 pandemic: impact on psychiatric care in the United States. Psychiatry Res. (2020) 289:113069. doi: 10.1016/j.psychres.2020.113069
15. Li, S, and Zhang, Y. Mental healthcare for psychiatric inpatients during the COVID-19 epidemic. Gen Psychiatr. (2020) 33:e100216. doi: 10.1136/gpsych-2020-100216
16. Xie, Q, Fan, F, Fan, XP, Wang, XJ, Chen, MJ, Zhong, BL, et al. COVID-19 patients managed in psychiatric inpatient settings due to first-episode mental disorders in Wuhan, China: clinical characteristics, treatments, outcomes, and our experiences. Transl Psychiatry. (2020) 10:337. doi: 10.1038/s41398-020-01022-x
17. Cui, LB, Wang, XH, and Wang, HN. Challenges of facing coronavirus disease 2019: psychiatric services for patients with mental disorders. Psychiatry Clin Neurosci. (2020) 74:371–2. doi: 10.1111/pcn.13003
18. Dorman-Ilan, S, Hertz-Palmor, N, Brand-Gothelf, A, Hasson-Ohayon, I, Matalon, N, Gross, R, et al. Anxiety and depression symptoms in COVID-19 isolated patients and in their relatives. Front Psych. (2020) 11:581598. doi: 10.3389/fpsyt.2020.581598
19. Czeisler, M, Rohan, EA, Melillo, S, Matjasko, JL, DePadilla, L, Patel, CG, et al. Mental health among parents of children aged <18 years and unpaid caregivers of adults during the COVID-19 pandemic – United States, December 2020 and February-march 2021. MMWR Morb Mortal Wkly Rep. (2021) 70:879–87. doi: 10.15585/mmwr.mm7024a3
20. Alessi, J, de Oliveira, GB, Feiden, G, Schaan, BD, and Telo, GH. Caring for caregivers: the impact of the COVID-19 pandemic on those responsible for children and adolescents with type 1 diabetes. Sci Rep. (2021) 11:6812. doi: 10.1038/s41598-021-85874-3
21. Reece, L, and Sams, DP. The impact of Covid-19 on adolescent psychiatric inpatient admissions. Clin Child Psychol Psychiatry. (2022) 27:112–21. doi: 10.1177/13591045211030666
22. Hu, Y, Chen, Y, Zheng, Y, You, C, Tan, J, Hu, L, et al. Factors related to mental health of inpatients with COVID-19 in Wuhan, China. Brain Behav Immun. (2020) 89:587–93. doi: 10.1016/j.bbi.2020.07.016
23. Yalçin, M, Sönmez Güngör, E, Ergelen, M, Beşikçi Keleş, D, Yerebakan Tüzer, M, Öcek Baş, T, et al. Characteristics and outcomes of psychiatric inpatients with severe mental illness and COVID-19: experience from a COVID-19-specific acute psychiatric Ward in Istanbul. J Nerv Ment Dis. (2021) 209:884–91. doi: 10.1097/nmd.0000000000001450
24. Yao, H, Chen, JH, and Xu, YF. Patients with mental health disorders in the Covid-19 epidemic. Lancet Psychiatry. (2020) 7:e21. doi: 10.1016/s2215-0366(20)30090-0
26. Schmittmann, VD, Cramer, AO, Waldorp, LJ, Epskamp, S, Kievit, RA, and Borsboom, D. Deconstructing the construct: a network perspective on psychological phenomena. New Ideas Psychol. (2013) 31:43–53. doi: 10.1016/j.newideapsych.2011.02.007
27. Borsboom, D. Psychometric perspectives on diagnostic systems. J Clin Psychol. (2008) 64:1089–108. doi: 10.1002/jclp.20503
28. Santos, H Jr, Fried, EI, Asafu-Adjei, J, and Ruiz, RJ. Network structure of perinatal depressive symptoms in Latinas: relationship to stress and reproductive biomarkers. Res Nurs Health. (2017) 40:218–28. doi: 10.1002/nur.21784
29. Marchetti, I. Hopelessness: a network analysis. Cogn Ther Res. (2018) 43:611–9. doi: 10.1007/s10608-018-9981-y
30. Mullarkey, MC, Marchetti, I, and Beevers, CG. Using network analysis to identify central symptoms of adolescent depression. J Clin Child Adolesc Psychol. (2019) 48:656–68. doi: 10.1080/15374416.2018.1437735
31. Borsboom, D. A network theory of mental disorders. World Psychiatry. (2017) 16:5–13. doi: 10.1002/wps.20375
32. Borsboom, D, and Cramer, AO. Network analysis: An integrative approach to the structure of psychopathology. Annu Rev Clin Psychol. (2013) 9:91–121. doi: 10.1146/annurev-clinpsy-050212-185608
33. Fried, EI, and Nesse, RM. Depression sum-scores don't add up: why analyzing specific depression symptoms is essential. BMC Med. (2015) 13:72. doi: 10.1186/s12916-015-0325-4
34. Liu, J, Zhu, Q, Fan, W, Makamure, J, Zheng, C, and Wang, J. Online mental health survey in a medical College in China during the COVID-19 outbreak. Front Psych. (2020) 11:459. doi: 10.3389/fpsyt.2020.00459
35. Liu, Y, Zhang, Z, and Zhao, H. The influence of the Covid-19 event on deviant workplace behavior taking Tianjin, Beijing and Hebei as an example. Int J Environ Res Public Health. (2020) 18. doi: 10.3390/ijerph18010059
36. Spitzer, RL, Kroenke, K, and Williams, JB. Validation and utility of a self-report version of prime-md: the PHQ primary care study. Primary care evaluation of mental disorders. Patient health questionnaire. JAMA. (1999) 282:1737–44. doi: 10.1001/jama.282.18.1737
37. Kroenke, K, Spitzer, RL, and Williams, JB. The PHQ-9: validity of a brief depression severity measure. J Gen Intern Med. (2001) 16:606–13. doi: 10.1046/j.1525-1497.2001.016009606.x
38. Chen, MM, Sheng, L, and Qu, S. Diagnostic test of screening depressive disorder in general hospital with the patient health questionnaire (in Chinese). J Chinese Mental Health. (2015) 29:241–5. doi: 10.3969/j.issn.1000-6729.2015.04.001
39. Wang, W, Bian, Q, Zhao, Y, Li, X, Wang, W, Du, J, et al. Reliability and validity of the Chinese version of the patient health questionnaire (PHQ-9) in the general population. Gen Hosp Psychiatry. (2014) 36:539–44. doi: 10.1016/j.genhosppsych.2014.05.021
40. Xu, Y, Wu, HS, and Xu, YF. The application of Patient Health Questionnaire 9 in community elderly population: reliability and validity (in Chinese). Shanghai Arch Psychiatry. (2007) 19:257.
41. Spitzer, RL, Kroenke, K, Williams, JB, and Löwe, B. A brief measure for assessing generalized anxiety disorder: the GAD-7. Arch Intern Med. (2006) 166:1092–7. doi: 10.1001/archinte.166.10.1092
42. Zhang, C, Wang, T, Zeng, P, Zhao, M, Zhang, G, Zhai, S, et al. Reliability, validity, and measurement invariance of the general anxiety disorder scale among Chinese medical university students. Front Psych. (2021) 12:648755. doi: 10.3389/fpsyt.2021.648755
43. Zeng, Q-Z, He, Y-L, Liu, H, Miao, J-M, Chen, J-X, Xu, H-N, et al. Reliability and validity of Chinese version of the Generalized Anxiety Disorder 7-item (GAD-7) scale in screening anxiety disorders in outpatients from traditional Chinese internal department (in Chinese). Chin Ment Health J. (2013) 27:163–8.
44. Gladman, D, Nash, P, Goto, H, Birt, JA, Lin, CY, Orbai, AM, et al. Fatigue numeric rating scale validity, discrimination and responder definition in patients with psoriatic arthritis. RMD Open. (2020) 6:e000928. doi: 10.1136/rmdopen-2019-000928
45. Fang, J-Q, Hao, Y-T, and Li, C-X. Reliability and validity for Chinese version of who quality of life scale (in Chinese). Chinese. J Ment Health. (1999) 13
46. The WHOQOL GROUP. Development of the World Health Organization WHOQOL-BREF quality of life assessment. The WHOQOL Group. Psychol Med. (1998) 28:551–8. doi: 10.1017/s0033291798006667
47. Skevington, SM, and Tucker, C. Designing response scales for cross-cultural use in health care: data from the development of the UK WHOQOL. Br J Med Psychol. (1999) 72:51–61. doi: 10.1348/000711299159817
48. Xia, P, Li, N, Hau, KT, Liu, C, and Lu, Y. Quality of life of Chinese Urban Community residents: a psychometric study of the mainland Chinese version of the WHOQOL-BREF. BMC Med Res Methodol. (2012) 12:37. doi: 10.1186/1471-2288-12-37
49. R Core Team. R: A language and environment for statistical computing. Vienna, Austria: R Foundation for Statistical Computing (2020).
50. Zhao, YJ, Xing, X, Tian, T, Wang, Q, Liang, S, Wang, Z, et al. Post Covid-19 mental health symptoms and quality of life among COVID-19 frontline clinicians: a comparative study using propensity score matching approach. Transl Psychiatry. (2022) 12:376. doi: 10.1038/s41398-022-02089-4
51. Bai, W, Zhao, YJ, Cai, H, Sha, S, Zhang, Q, Lei, SM, et al. Network analysis of depression, anxiety, insomnia and quality of life among Macau residents during the COVID-19 pandemic. J Affect Disord. (2022) 311:181–8. doi: 10.1016/j.jad.2022.05.061
52. Di Blasi, M, Gullo, S, Mancinelli, E, Freda, MF, Esposito, G, Gelo, OCG, et al. Psychological distress associated with the COVID-19 lockdown: a two-wave network analysis. J Affect Disord. (2021) 284:18–26. doi: 10.1016/j.jad.2021.02.016
53. Chernick, MR. Bootstrap methods: A guide for practitioners and researchers. 2nd ed. New Jersey: John Wiley & Sons (2008). 619 p.
54. Costenbader, E, and Valente, TW. The stability of centrality measures when networks are sampled. Soc Networks. (2003) 25:283–307. doi: 10.1016/S0378-8733(03)00012-1
55. Epskamp, S, Borsboom, D, and Fried, EI. Estimating psychological networks and their accuracy: a tutorial paper. Behav Res Methods. (2018) 50:195–212. doi: 10.3758/s13428-017-0862-1
56. Jones, P. Networktools: tools for identifying important nodes in networks. R package version 1.2.3. Available at: https://CRANR-projectorg/package=networktools (2020).
57. Epskamp, S, Cramer, AO, Waldorp, LJ, Schmittmann, VD, and Borsboom, D. qgraph: network visualizations of relationships in psychometric data. J Stat Softw. (2012) 48:1–18.
58. van Borkulo, C, Boschloo, L, Kossakowski, J, Tio, P, Schoevers, R, Borsboom, D, et al. Comparing network structures on three aspects: a permutation test. J Stat Softw. (2017). doi: 10.13140/RG.2.2.29455.38569
59. van Borkulo, CD, van Bork, R, Boschloo, L, Kossakowski, JJ, Tio, P, Schoevers, RA, et al. Comparing network structures on three aspects: a permutation test. Psychol Methods. (2022). doi: 10.1037/met0000476
60. Haslbeck, J, and Waldorp, LJ. mgm: estimating time-varying mixed graphical models in high-dimensional data. J Stat Softw. (2020) 93:1–46. doi: 10.18637/jss.v093.i08
61. Lane, NE, Hoben, M, Amuah, JE, Hogan, DB, Baumbusch, J, Gruneir, A, et al. Prevalence and correlates of anxiety and depression in caregivers to assisted living residents during Covid-19: a cross-sectional study. BMC Geriatr. (2022) 22:662. doi: 10.1186/s12877-022-03294-y
62. Li, Q, Zhang, H, Zhang, M, Li, T, Ma, W, An, C, et al. Prevalence and risk factors of anxiety, depression, and sleep problems among caregivers of people living with neurocognitive disorders during the COVID-19 pandemic. Front Psych. (2020) 11:590343. doi: 10.3389/fpsyt.2020.590343
63. Souza, ALR, Guimarães, RA, de Araújo, VD, de Assis, RM, de Almeida Cavalcante Oliveira, LM, Souza, MR, et al. Factors associated with the burden of family caregivers of patients with mental disorders: a cross-sectional study. BMC Psychiatry. (2017) 17:353. doi: 10.1186/s12888-017-1501-1
64. Haug, TT, Mykletun, A, and Dahl, AA. The association between anxiety, depression, and somatic symptoms in a large population: the hunt-ii study. Psychosom Med. (2004) 66:845–51. doi: 10.1097/01.psy.0000145823.85658.0c
65. Ligthart, L, Gerrits, MM, Boomsma, DI, and Penninx, BW. Anxiety and depression are associated with migraine and pain in general: an investigation of the interrelationships. J Pain. (2013) 14:363–70. doi: 10.1016/j.jpain.2012.12.006
66. Kessler, RC, Sampson, NA, Berglund, P, Gruber, MJ, Al-Hamzawi, A, Andrade, L, et al. Anxious and non-anxious major depressive disorder in the World Health Organization world mental health surveys. Epidemiol Psychiatr Sci. (2015) 24:210–26. doi: 10.1017/s2045796015000189
67. Fava, M, Alpert, JE, Carmin, CN, Wisniewski, SR, Trivedi, MH, Biggs, MM, et al. Clinical correlates and symptom patterns of anxious depression among patients with major depressive disorder in Star*D. Psychol Med. (2004) 34:1299–308. doi: 10.1017/s0033291704002612
68. Jansson-Fröjmark, M, and Lindblom, K. A bidirectional relationship between anxiety and depression, and insomnia? A prospective study in the general population. J Psychosom Res. (2008) 64:443–9. doi: 10.1016/j.jpsychores.2007.10.016
69. Frances, A, Manning, D, Marin, D, Kocsis, J, McKinney, K, Hall, W, et al. Relationship of anxiety and depression. Psychopharmacology. (1992) 106:S82–6. doi: 10.1007/BF02246243
70. Hettema, JM. What is the genetic relationship between anxiety and depression? Am J Med Genet C Semin Med Genet. (2008) 148c:140–6. doi: 10.1002/ajmg.c.30171
71. Sullivan, PF, Neale, MC, and Kendler, KS. Genetic epidemiology of major depression: review and meta-analysis. Am J Psychiatry. (2000) 157:1552–62. doi: 10.1176/appi.ajp.157.10.1552
72. Hettema, JM, Neale, MC, and Kendler, KS. A review and meta-analysis of the genetic epidemiology of anxiety disorders. Am J Psychiatry. (2001) 158:1568–78. doi: 10.1176/appi.ajp.158.10.1568
73. Middeldorp, CM, Cath, DC, Van Dyck, R, and Boomsma, DI. The co-morbidity of anxiety and depression in the perspective of genetic epidemiology. A review of twin and family studies. Psychol Med. (2005) 35:611–24. doi: 10.1017/s003329170400412x
74. Corfield, EC, Martin, NG, and Nyholt, DR. Co-occurrence and symptomatology of fatigue and depression. Compr Psychiatry. (2016) 71:1–10. doi: 10.1016/j.comppsych.2016.08.004
75. Pae, C-U, Lim, H-K, Han, C, Patkar, AA, Steffens, DC, Masand, PS, et al. Fatigue as a Core symptom in major depressive disorder: overview and the role of bupropion. Expert Rev Neurother. (2007) 7:1251–63. doi: 10.1586/14737175.7.10.1251
76. Reyes-Gibby, CC, Aday, LA, Anderson, KO, Mendoza, TR, and Cleeland, CS. Pain, depression, and fatigue in community-dwelling adults with and without a history of cancer. J Pain Symptom Manage. (2006) 32:118–28. doi: 10.1016/j.jpainsymman.2006.01.008
78. Berman, RM, Narasimhan, M, Miller, HL, Anand, A, Cappiello, A, Oren, DA, et al. Transient depressive relapse induced by catecholamine depletion: potential phenotypic vulnerability marker? Arch Gen Psychiatry. (1999) 56:395–403. doi: 10.1001/archpsyc.56.5.395
79. Chaves-Filho, AJM, Macedo, DS, de Lucena, DF, and Maes, M. Shared microglial mechanisms underpinning depression and chronic fatigue syndrome and their comorbidities. Behav Brain Res. (2019) 372:111975. doi: 10.1016/j.bbr.2019.111975
80. Hinz, A, Mehnert, A, Kocalevent, R-D, Brähler, E, Forkmann, T, Singer, S, et al. Assessment of depression severity with the PHQ-9 in cancer patients and in the general population. BMC Psychiatry. (2016) 16:1–8.
81. Fava, GA, and Tossani, E. Prodromal stage of major depression. Early Interv Psychiatry. (2007) 1:9–18. doi: 10.1111/j.1751-7893.2007.00005.x
82. Robinson, RL, Stephenson, JJ, Dennehy, EB, Grabner, M, Faries, D, Palli, SR, et al. The importance of unresolved fatigue in depression: costs and comorbidities. Psychosomatics. (2015) 56:274–85. doi: 10.1016/j.psym.2014.08.003
83. Beard, C, Millner, AJ, Forgeard, MJ, Fried, EI, Hsu, KJ, Treadway, MT, et al. Network analysis of depression and anxiety symptom relationships in a psychiatric sample. Psychol Med. (2016) 46:3359–69. doi: 10.1017/S0033291716002300
84. van Rooijen, G, Isvoranu, AM, Kruijt, OH, van Borkulo, CD, Meijer, CJ, Wigman, JTW, et al. A state-independent network of depressive, negative and positive symptoms in male patients with schizophrenia spectrum disorders. Schizophr Res. (2018) 193:232–9. doi: 10.1016/j.schres.2017.07.035
85. McIlvenny, S, DeGlume, A, Elewa, M, Fernandez, O, and Dormer, P. Factors associated with fatigue in a family medicine clinic in the United Arab Emirates. Fam Pract. (2000) 17:408–13. doi: 10.1093/fampra/17.5.408
86. Stewart, D, Abbey, S, Meana, M, and Boydell, KM. What makes women tired? A Community Sample J Womens Health. (1998) 7:69–76. doi: 10.1089/jwh.1998.7.69
88. Bekhuis, E, Olde Hartman, TC, Boschloo, L, and Lucassen, PL. A new approach to psychopathology: the example of depression. Br J Gen Pract. (2019) 69:146–7. doi: 10.3399/bjgp19X701717
89. Cole, DA, Cai, L, Martin, NC, Findling, RL, Youngstrom, EA, Garber, J, et al. Structure and measurement of depression in youths: applying item response theory to clinical data. Psychol Assess. (2011) 23:819–33. doi: 10.1037/a0023518
90. Cheung, T, Jin, Y, Lam, S, Su, Z, Hall, BJ, and Xiang, YT. Network analysis of depressive symptoms in Hong Kong residents during the COVID-19 pandemic. Transl Psychiatry. (2021) 11:460. doi: 10.1038/s41398-021-01543-z
91. Hartung, TJ, Fried, EI, Mehnert, A, Hinz, A, and Vehling, S. Frequency and network analysis of depressive symptoms in patients with cancer compared to the general population. J Affect Disord. (2019) 256:295–301. doi: 10.1016/j.jad.2019.06.009
92. Fried, EI, Epskamp, S, Nesse, RM, Tuerlinckx, F, and Borsboom, D. What are 'Good' depression symptoms? Comparing the centrality of DSM and non-DSM symptoms of depression in a network analysis. J Affect Disord. (2016) 189:314–20. doi: 10.1016/j.jad.2015.09.005
93. Lépine, JP, and Briley, M. The increasing burden of depression. Neuropsychiatr Dis Treat. (2011) 7:3–7. doi: 10.2147/ndt.S19617
94. Sivertsen, H, Bjørkløf, GH, Engedal, K, Selbæk, G, and Helvik, AS. Depression and quality of life in older persons: a review. Dement Geriatr Cogn Disord. (2015) 40:311–39. doi: 10.1159/000437299
95. Lou, Z, Li, Y, Yang, Y, Wang, L, and Yang, J. Affects of anxiety and depression on health-related quality of life among patients with benign breast lumps diagnosed via ultrasonography in China. Int J Environ Res Public Health. (2015) 12:10587–601. doi: 10.3390/ijerph120910587
Keywords: depression, quality of life, guardians, hospitalized psychiatric patients, network
Citation: Zhao Y-J, Zhang L, Feng Y, Sha S, Lam MI, Wang Y-Y, Li J-X, Su Z, Cheung T, Ungvari GS, Jackson T, An F-R and Xiang Y-T (2023) Prevalence of depression and its association with quality of life among guardians of hospitalized psychiatric patients during the COVID-19 pandemic: a network perspective. Front. Psychiatry. 14:1139742. doi: 10.3389/fpsyt.2023.1139742
Received: 07 January 2023; Accepted: 03 April 2023;
Published: 12 May 2023.
Edited by:
Luca Steardo, Magna Græcia University of Catanzaro, ItalyReviewed by:
Yaoyao Sun, Peking University Sixth Hospital, ChinaCopyright © 2023 Zhao, Zhang, Feng, Sha, Lam, Wang, Li, Su, Cheung, Ungvari, Jackson, An and Xiang. This is an open-access article distributed under the terms of the Creative Commons Attribution License (CC BY). The use, distribution or reproduction in other forums is permitted, provided the original author(s) and the copyright owner(s) are credited and that the original publication in this journal is cited, in accordance with accepted academic practice. No use, distribution or reproduction is permitted which does not comply with these terms.
*Correspondence: Feng-Rong An, YWZyeWxtQHNpbmEuY29t; Yu-Tao Xiang, eHl1dGx5QGdtYWlsLmNvbQ==
†These authors have contributed equally to this work
Disclaimer: All claims expressed in this article are solely those of the authors and do not necessarily represent those of their affiliated organizations, or those of the publisher, the editors and the reviewers. Any product that may be evaluated in this article or claim that may be made by its manufacturer is not guaranteed or endorsed by the publisher.
Research integrity at Frontiers
Learn more about the work of our research integrity team to safeguard the quality of each article we publish.