- 1Department of Child and Adolescent Psychiatry (research section), University Psychiatric Clinics, Basel, Switzerland
- 2Zurich Center for Neuroeconomics, Department of Economics, University of Zurich, Zurich, Switzerland
- 3Amsterdam UMC, Vrije Universiteit Amsterdam, Department of Child and Adolescent Psychiatry, Amsterdam Public Health, Amsterdam, Netherlands
- 4Jacobs Center for Productive Youth Development, University of Zurich, Zurich, Switzerland
- 5Department of Child and Adolescent Psychiatry, Faculty of Medicine, TU Dresden, Dresden, Germany
- 6Hospital Universitario Mutua Terrassa, IGAIN, Barcelona, Spain
- 7Biocruces Bizkaia Health Research Institute, Basurto University Hospital, University of the Basque Country, Bilbao, Spain
- 8RWTH Aachen University & JARA-Brain Institute, Aachen, Germany
- 9Child and Adolescent Psychiatry Amsterdam University Medical Centers, Amsterdam, Netherlands
- 10Child and Adolescent Psychiatry, Goethe University, Frankfurt, Germany
- 11University of Birmingham, Birmingham, United Kingdom
- 12Developmental Psychopathology, University of Bath, Bath, United Kingdom
Aims: Heart rate variability (HRV) measures have been suggested in healthy individuals as a potential index of self-regulation skills, which include both cognitive and emotion regulation aspects. Studies in patients with a range of psychiatric disorders have however mostly focused on the potential association between abnormally low HRV at rest and specifically emotion regulation difficulties. Emotion regulation deficits have been reported in patients with Conduct Disorder (CD) however, the association between these emotion regulation deficits and HRV measures has yet to be fully understood. This study investigates (i) the specificity of the association between HRV and emotion regulation skills in adolescents with and without CD and (ii) the association between HRV and grey matter brain volumes in key areas of the central autonomic network which are involved in self-regulation processes, such as insula, lateral/medial prefrontal cortices or amygdala.
Methods: Respiratory sinus arrhythmia (RSA) measures of HRV were collected from adolescents aged between 9–18 years (693 CD (427F)/753 typically developing youth (TD) (500F)), as part of a European multi-site project (FemNAT-CD). The Inverse Efficiency Score, a speed-accuracy trade-off measure, was calculated to assess emotion and cognitive regulation abilities during an Emotional Go/NoGo task. The association between RSA and task performance was tested using multilevel regression models. T1-weighted structural MRI data were included for a subset of 577 participants (257 CD (125F); 320 TD (186F)). The CerebroMatic toolbox was used to create customised Tissue Probability Maps and DARTEL templates, and CAT12 to segment brain images, followed by a 2 × 2 (sex × group) full factorial ANOVA with RSA as regressor of interest.
Results: There were no significant associations between RSA and task performance, neither during emotion regulation nor during cognitive regulation trials. RSA was however positively correlated with regional grey matter volume in the left insula (pFWE = 0.011) across all subjects.
Conclusion: RSA was related to increased grey matter volume in the left insula across all subjects. Our results thus suggest that low RSA at rest might be a contributing or predisposing factor for potential self-regulation difficulties. Given the insula’s role in both emotional and cognitive regulation processes, these brain structural differences might impact either of those.
1. Introduction
Heart Rate Variability (HRV) is a psychophysiological measure that captures beat-to beat vagal modulation of the heart rate. It has a relatively stable, trait-like character and has consistently been associated with physical and psychological wellbeing (1–7). Autonomic system adjustment to contextual demands is regulated by the Central Autonomic Network (CAN), a brain network which receives input from both the sympathetic and parasympathetic branches of the Autonomic Nervous System (ANS) underlying not only the control of autonomic responses but also visceromotor, neuroendocrine, and behavioural responses (7–12). Key components of the CAN include the anterior cingulate, insula, orbitofrontal and ventromedial prefrontal cortices, together with amygdala, thalamus, subthalamic, and brain stem nuclei (9, 11, 12). These structures are not only involved in autonomic regulation but also in the implementation of emotional and cognitive self-regulation processes (10, 13–17). Furthermore, the Neurovisceral Integration Model postulates HRV as an index of functional integrity of brain structures involved in higher executive functions including working memory, inhibitory control as well as in emotion regulation (10, 15). Measures of vagal function such as HRV, primarily mediated by parasympathetic vagal innervation, can also index inhibitory prefrontal processes involved in stress response, being therefore indicative of the ability of the autonomic system to respond and flexibly adjust to contextual demands (7, 8).
Individuals with low HRV show heightened reactivity to emotional stimuli (1) as well as difficulties in emotion regulation and impulse control in daily life (18). In line with this, recent evidence from meta-analytic studies has shown that low HRV measures are observed across several different psychiatric disorders (4, 19–21), including Conduct Disorder (CD) (22), hence suggestive of its potential transdiagnostic character. Low HRV has been associated with antisocial and aggressive behaviours (23), callous-unemotional traits (24), depressive symptoms or suicidal ideation (25–28) or anxiety disorders (29), thus supporting the idea of HRV as a potential index of deficits in emotion regulation processes and psychological well-being (7). ANS indicators have indeed long been discussed as potential biomarkers for CD (30), a highly impairing psychiatric disorder emerging in childhood or adolescence characterized by severe antisocial and aggressive behaviour. However, evidence from a recent review and meta-analyses (22, 31) have suggested a high physiological heterogeneity, where the association between ANS function and CD or antisocial behaviours might vary as a function of the clinical subtype, as well as of the ANS measures and analytical methods used. How ANS function might be associated with the phenotypic presentation of CD is thus not yet fully understood.
At the neural level, individual differences in HRV measures at rest have shown a positive association with cortical thickness in the dorsal anterior cingulate gyrus in healthy young adults (32) as well as in war veterans (33). However, in healthy adults also negative associations were found between HRV and grey matter volumes in subcortical and limbic regions including the putamen, caudate, amygdala, insula or superior temporal gyrus (34) while others found no association (35). HRV measures have been shown to be highly sensitive, influenced by a number of factors such as sex, age, SES or variations in the assessment methods used (36). Thus, the mechanism underlying the association between brain structures and HRV measures in healthy populations remains unclear.
Patients with a diagnosis of CD have been reported to have abnormal structure and function in regions including the insula, amygdala, temporal cortex and ventral striatum (30, 37–41). This association has been frequently discussed in the context of emotion regulation deficits (40, 42–45). However, children and youths diagnosed with CD show highly heterogeneous symptomatic (30), neurocognitive (46), and physiological profiles (22). The Research Domain Criteria (RDoC) (47) adopts a dimensional approach in which specific alterations in predefined biological systems lead to various symptomatic presentation. The RDoC approach could therefore contribute to improve the understanding of the high heterogeneity present in neuropsychiatric disorders, as in CD. Thus, psychophysiological measures have been suggested as having the potential to help disentangle the heterogeneity within CD populations. Given the association between HRV and emotion regulation deficits, HRV has been discussed as a potential physiological marker for CD. However, recent studies using different HRV indices such as Respiratory Sinus Arrythmia (RSA) or Pre-ejection Period (PeP) did not find significant associations between ANS activity and CD (48) or antisocial behaviours considered from a dimensional perspective (49). While different psychophysiological measures can be closely interrelated, subtle differences between groups with small effect sizes might be overlooked depending on the measure selected. Furthermore, the association between HRV and emotion regulation in patients with CD might differ significantly based on symptomatic presentations or comorbidities (31, 50). Thus, altered HRV has been shown in patients with CD and comorbid internalizing disorders, but not in those with callous-unemotional traits (31). In addition, different factors such as Body-Mass Index (BMI), socio-economic status (SES), medication intake, sports (48, 49), the type of task and analyses used or the physiological outcome investigated (22) might influence HRV measures hence contributing to the heterogeneous body of evidence.
Most of the research studies on the role of HRV in patients with CD have focused on its potential association with emotion regulation deficits (31). However, HRV might be associated with more generic self-regulation skills (51, 52). Successful self-regulation skills are crucial for goal-directed behaviour and require the implementation of selective and sustained attention, cognitive control and inhibition of inadequate emotional and behavioural responses (53). Consequently, successful self-regulation skills require the ability to regulate both emotional and cognitive processes. The available evidence seems to suggest that HRV could indeed be better understood as a general index of regulatory processes, as it has been shown to be associated with top-down self-regulation abilities (51), executive function (54), and performance during inhibition or switch tasks (52). However, the effect might be rather small and moderated by a number of variables, some inherent to the individuals such as age or sex, others related to the metrics used or the methodological approaches followed during the assessments (52).
In summary, the evidence on the association between HRV and emotion regulation capacities in the few existing studies in patients with CD is not consistent (22). Thus, the present study investigates whether HRV might be associated with more generic self-regulation deficits and with individual differences in key components of the CAN, and whether these associations differ between healthy youths and youths with CD. To test this, we capitalized on data collected as part of a European multicenter study (FemNAT-CD Project), which combines psychophysiological (with HRV operationalized via RSA), neuropsychological (performance of an Emotional Go/NoGo task) and brain structure data in a group of male and female adolescents with CD, as well as of typically developing adolescents. This allowed us to investigate whether there are group differences between adolescents with CD and healthy peers in a) the association between RSA and emotion regulation and cognitive regulation performance during an Emotional Go/NoGo task and b) the potential association between the HRV measures and grey matter volumes in those regions typically defined as part of the CAN (9). Based on the available evidence on factors that might potentially influence the association between CD and HRV, we took those variables into consideration including comorbid internalising symptoms or CU traits within the CD group (31), as well as age, sex, SES, BMI, cigarette consumption, involvement in sport or IQ (48, 55). We hypothesized that if RSA can be considered as a potential general index of self-regulation, we will find that: (1) there will be a positive association between task performance in both the emotional and cognitive regulation conditions of the Emotional Go/NoGo task and RSA measures across all participants, without significant group differences between patients and controls, and (2) RSA measures will be positively associated with cortical grey matter volumes in brain regions that are part of the CAN network across all participants, without significant group differences between patients and controls.
2. Materials and methods
2.1. Participants
Participants of this study were part of the Neurobiology and Treatment of Adolescent Female Conduct Disorder (FemNAT-CD) project1, a large European multicenter study investigating gender differences in the neurobiology underlying CD. The final sample (N = 1,446) of this study included 693 CD (427 females) and 753 TD (500 females) youth aged 9 to 18 years (M = 14.36; SD = 2.44 years) who had valid psychophysiological and neuropsychological data. In addition, structural MRI data was available for a subset of participants (N = 577, CD: n = 257, 125 females; TD: n = 320, 186 females). Further details regarding the sample characteristics are reported in Table 1.
Participants were recruited using flyers and advertisement in clinics, youth welfare centers, internet forums and schools, in Birmingham and Southampton (UK), Bilbao and Barcelona (Spain), Amsterdam (Netherlands), Aachen and Frankfurt (Germany), Szeged (Hungary), Athens (Greece), and Basel (Switzerland) between 2003 and 2013. CD youth had to fulfil DSM-5 diagnostic criteria for CD (56), while TD youth were included if they did not meet criteria for any current psychiatric disorder and had no previous DSM-IV diagnosis of CD, ODD or ADHD. Additional exclusion criteria for both groups were a diagnosis of autism spectrum disorder or schizophrenia (ICD-10, DSM-IV-TR or DSM-5), current bipolar disorder or mania, monogenetic disorder, genetic syndrome, any chronic or acute neurological disorder, treatment for epilepsy, history of traumatic brain injury, or IQ <70. The FemNAT-CD project was conducted in accordance with the Declaration of Helsinki and approved by the European Commission and local ethics committees of all participating sites. All participants and their caregivers provided written informed assent/consent.
2.2. Clinical and behavioural measures
2.2.1. Clinical interviews and questionnaires
Participants and their parents/caregivers were assessed via a semi-structured interview (Schedule for Affective Disorders and Schizophrenia for School-Age Children-Present and Lifetime version, K-SADS-PL) (57). In addition, participants and parents/caregivers completed different questionnaires and behavioural measures (for more information please (see 46)).
Internalising symptoms were assessed through the parent report version Child Behavior Checklist (CBCL) (58) and CU traits through the total score from the parent report version of the Inventory of Callous-Unemotional Traits (ICU) (59). Presence of ADHD was assessed during the interview and coded as a binary variable with 0 and 1 indicating the absence/presence of a current diagnosis of ADHD. We additionally accounted for factors with known impact on HRV measures. These included SES, BMI, number of cigarettes smoked per day and physical activity (hours/week) habits, and intake of medication that might affect parasympathetic nervous system (PNS) function (see Table 1). SES scores were computed using principal component extraction based on parental income, education and occupation (ISCO-08) (60) (ISCED) (61), and standardized within each country to avoid potential economic variation at the country level. Current medication intake of compounds that might affect PNS function was assessed by asking the participant, caretaker, therapist, or parent, and coded as a dichotomous variable (yes/no). Depending on age and language, IQ was assessed using the Wechsler Abbreviated Scale of Intelligence (WASI-II) (62), the Wechsler Intelligence Scale for Children (WISC) (63) or the Wechsler Adult Intelligence Scale (WAIS) (64) (further details on the assessments and scoring procedures can be found in (46, 48). Participants performed the emotional Go/NoGo task and underwent a psychophysiological assessment in separate study sessions.
2.2.2. Emotional Go/NoGo task
Performance in the Emotional Go/NoGo task was used to investigate the potential specificity of the association between RSA and self-regulation within the emotional or cognitive domains. This is an adapted version of the Emotional Go/NoGo task developed by Hare and colleagues (65, 66), where on each trial participants are presented with human faces, which might depict a neutral, happy or fearful expression. Trials are presented in blocks of 48, with only two types of emotions presented on each block. Participants are instructed to press a button as fast as possible when they see one of these expressions (Go trials, 73% trials in each block) and refrain from responding when presented with the second emotional expression of the block (NoGo trials, 27% trials in each block). Stimuli duration was 0.5 s, with a fixed 1 s interstimulus interval (for further details, (see 46)). The different combinations of Go/NoGo trials allows the classification of the blocks as indexing emotion regulation (emotional faces as NoGo stimuli in the context of neutral faces as Go-stimuli) or cognitive regulation (neutral faces as NoGo stimuli in the context of emotional faces as Go-stimuli) (66). The main performance measures in the task were response times to Go trials and proportion of correct response to NoGo trials. These were first z-transformed and then combined in a single measure to account for the speed-accuracy trade-off that is commonly observed in the task, with slower response in the Go trials being accompanied typically with a higher proportion of successful NoGo trials (66). Thus, the Inverse Efficiency Scores (IES) (67) were calculated indicating the ratio of z-transformed mean reaction time (Go trials) to z-transformed correct responses to NoGo stimuli (1 – incorrect responses to NoGo trials) (66).
2.2.3. Psychophysiological assessment
The procedures followed to acquire and process the electrocardiogram and respiratory rate (RR) data have been described in detail elsewhere (48). In short, to ensure familiarization with the setting and minimize potential effects of stress, application of H98SG ECG Micropore electrodes was followed by a 10 min habituation period. Next, a 5 min excerpt from an aquatic video (Coral Sea Dreaming, Small World Music Inc.) was presented on a DELL Latitude E5550 Laptop with Sennheiser HD 201 earphones, to obtain a baseline measure for HRV. Prior to the assessment, participants were asked to refrain from smoking (1 h), and from consuming alcohol or drugs (24 h) (A detailed description of the psychophysiological measurement procedure can be found in (48, 49)).
Heart and respiration rate (RR) were assessed to compute RSA. RSA is a common measure for heart-rate variability (HRV) as it indexes parasympathetic activity, which a growing body of research suggests to be linked with emotion regulation capacities (68). Respiration cycle and ECG were recorded using a VU-AMS device (Vrije Universiteit Ambulatory Monitoring System) (69). Raw data was pre-processed using automated and manual steps provided by the VU-DAMS software package version 3.9 to ensure high data quality. RSA was subject to natural-log transformation prior to the analyses to approach a normal distribution of the data (70).
2.3. MRI acquisition
Each site followed a site qualification procedure before starting data collection to ensure comparability of MRI data acquisition. Images were acquired using either a Siemens Trio (Frankfurt and Southampton), Siemens Prisma (Aachen and Basel) or Philips scanner (Birmingham) – all at 3 Tesla. Structural T1-weighted magnetization prepared rapid gradient echo (MPRAGE) images were acquired for each participant which included 192 slices, field of view 256 mm, voxel size 1 × 1 × 1 mm, repetition time 1900 ms, echo time 2.42 (Aachen and Basel), 2.74 (Frankfurt), 3.7 (Birmingham), or 4.1 ms (Southampton), flip angle 9 degrees.
2.4. Statistical analysis
2.4.1. Behavioural analysis
All analyses were conducted using R (Version 4.1.2) (71) implemented on RStudio (Version 1.4.1717). Missing values on the relevant variables to be included in the models were imputed using the “mice” packed to implement Multiple Imputation by Chained Equations (72). Details on the missing data for each variable are shown in Table 1. All behavioural and clinical variables were z-transformed before being entered in the analyses.
We examined the association between RSA and performance measures in the Emotional Go/NoGo task (IES for emotion regulation and cognitive regulation conditions), with RSA values entered as predictors and task as dependent variables with the main regressors of interest being RSA and group (CD vs. TD).
Multilevel mixed models (MLM) were used for all behavioural data analyses, with the data nested by data collection site to account for dependency in observations using the package nlme (73) and with maximised log-likelihood (‘ML’) as estimation method. Patients with CD often present with comorbid internalising symptoms and CU traits, which have been previously shown to drive psychophysiological heterogeneity (31). To further investigate whether including these variables would improve the data fit, we conducted a model comparison between models without (simple) and with (extended) these regressors. Thus, in the simple model we included the following regressors as variables of no interest: age, sex, comorbid ADHD, SES, number of cigarettes smoked per day and IQ and in the extended model we added two additional regressors to account for the presence of internalising symptoms and CU traits to compare their relative fit to the data. The two models were then compared using the anova.lme command from the nlme package (73). These analyses allowed us to identify whether there was additional variability in the data explained when adding the internalising symptoms and CU traits in the model.
To test the robustness of the results, we repeated the analyses excluding participants whose prescribed medication might have affected their PNS function and therefore, HRV measures.
2.4.2. MRI data preprocessing and analyses
Structural MRI data was analysed using the CAT12 toolbox (74) implemented in SPM12 (75) using MATLAB (v2020b). Data was preprocessed using standard CAT12 steps, and only those individuals whose data quality was classified by CAT12 as C or higher were included in the analyses. Customised tissue probability maps (TPM) across all individuals were created using the CerebroMatic toolbox (76) and used to segment individual data into the different tissues (grey/white matter and CSF) and smoothed using a 8 mm Gaussian kernel. Total Intracranial Volumes (TIV) were then calculated for each participant. The smoothed grey matter volumes were included in a 2 × 2 full factorial ANOVA, with gender and group as factors. Standardized RSA values were included as the main regressor of interest, whereas TIV, Age, IQ, and site (one regressor per site, using one-hot encoding) are used as regressors of no interest. Analyses were masked for the cortical and subcortical regions which are involved in both emotional and cognitive self-regulation, as well as those typically included as part of the CAN (9). These regions included the amygdala, insular cortex, anterior cingulate cortex and medial prefrontal cortex (Supplementary Figure S1). Results are deemed as significant at a p < 0.05, family-wise error (FWE) correction, using the Threshold-Free Cluster Enhancement technique (TFCE) with 5,000 permutations.
3. Results
3.1. Behavioural results
Univariate ANOVA comparisons showed that the group of patients with CD differed from the control participants in several demographic variables including age, IQ, SES, medication intake, and number of cigarettes per day or hours of sports per week, as well as in clinical variables including comorbid ADHD, CU traits and internalising symptoms (Table 1). The results of the initial multilevel regression analyses on RSA showed significant main effects of age, cigarette, and medication use (Supplementary Table S1) which we then included in our main analysis as covariates. When Group was included as a regressor in the model (as a factor with two levels: TD and CD), no significant effect of group was observed, suggestive of no RSA differences between the two groups when potential influencing factors (age, medication intake or cigarette consumption) are taken into consideration.
3.1.1. Emotional Go/NoGo task performance
For IES, significant group differences between CD patients and controls were observed during both task trial conditions, with the CD group showing a lower speed-accuracy trade-off than their healthy counterparts (p < 0.001; Table 2). However, we did not find an association between RSA and IES (Table 3) in either task trial condition, and excluding participants taking medication with potential PNS effects did not alter these results (Table 4).
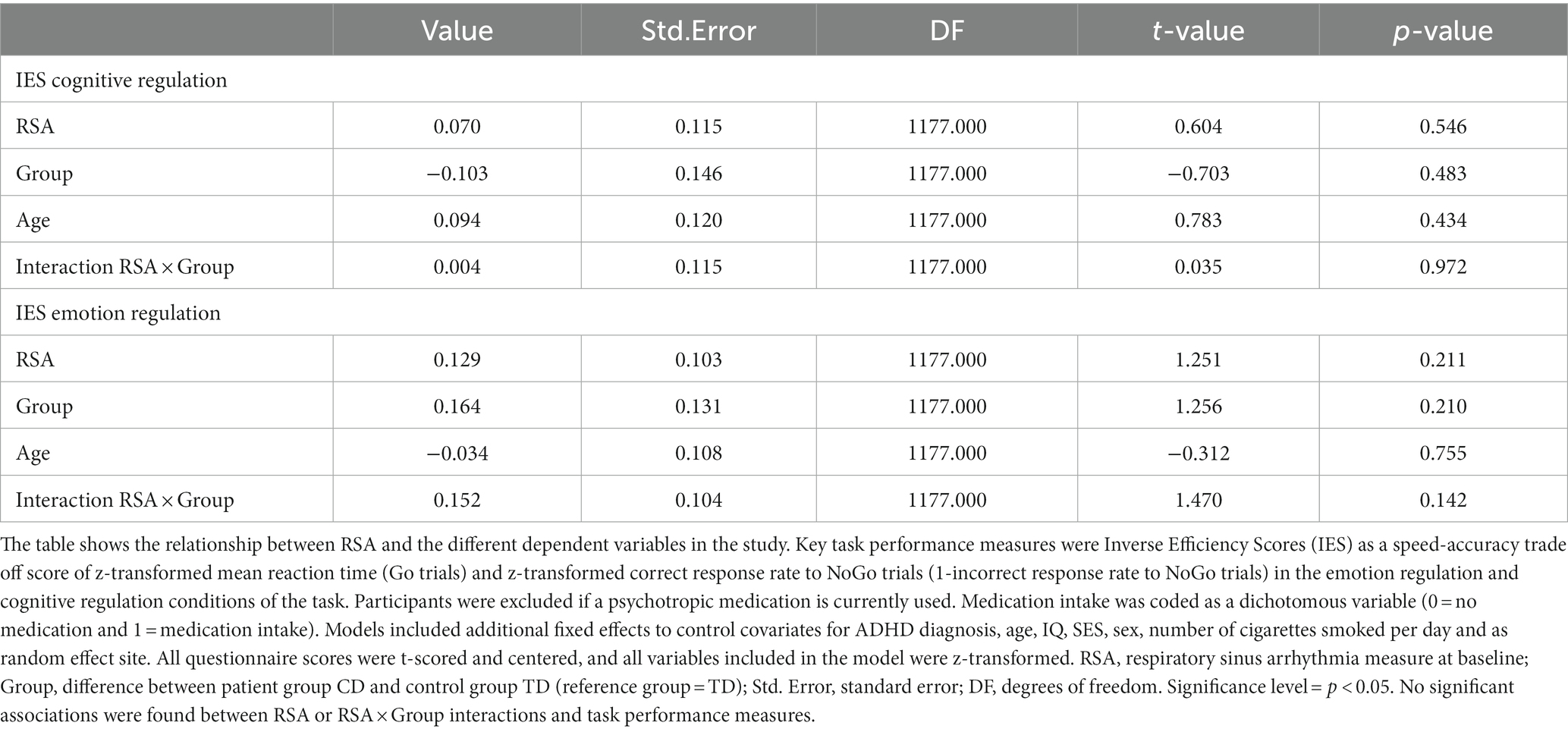
Table 4. Results of multi-level regression analyses on task performance measures after exclusion of participants with positive intake of medication with potential impact on PNS function.
To further investigate the role of internalising symptoms and CU traits in the association between RSA and emotion and cognitive regulation measures, we conducted post-hoc exploratory analyses including total subscale scores for internalising symptoms (CBCL) and total sum scores for CU traits (ICU) in the extended model (Table 5). We found no significant association between RSA and IES, and model comparison results suggested no significantly better model fit for the extended model (Table 6). Supplemental analysis comparing groups in correct response rates and reaction times revealed significant group differences (Supplementary Table S2). In the cognitive regulation condition, the TD group showed higher correct response rates and shorter reaction times than the CD group, and higher correct response rates and longer reaction times in the emotion regulation condition. Multilevel regression models in the MRI subsample (N = 577) did not find an association of RSA or group with IES (Supplementary Table S3).
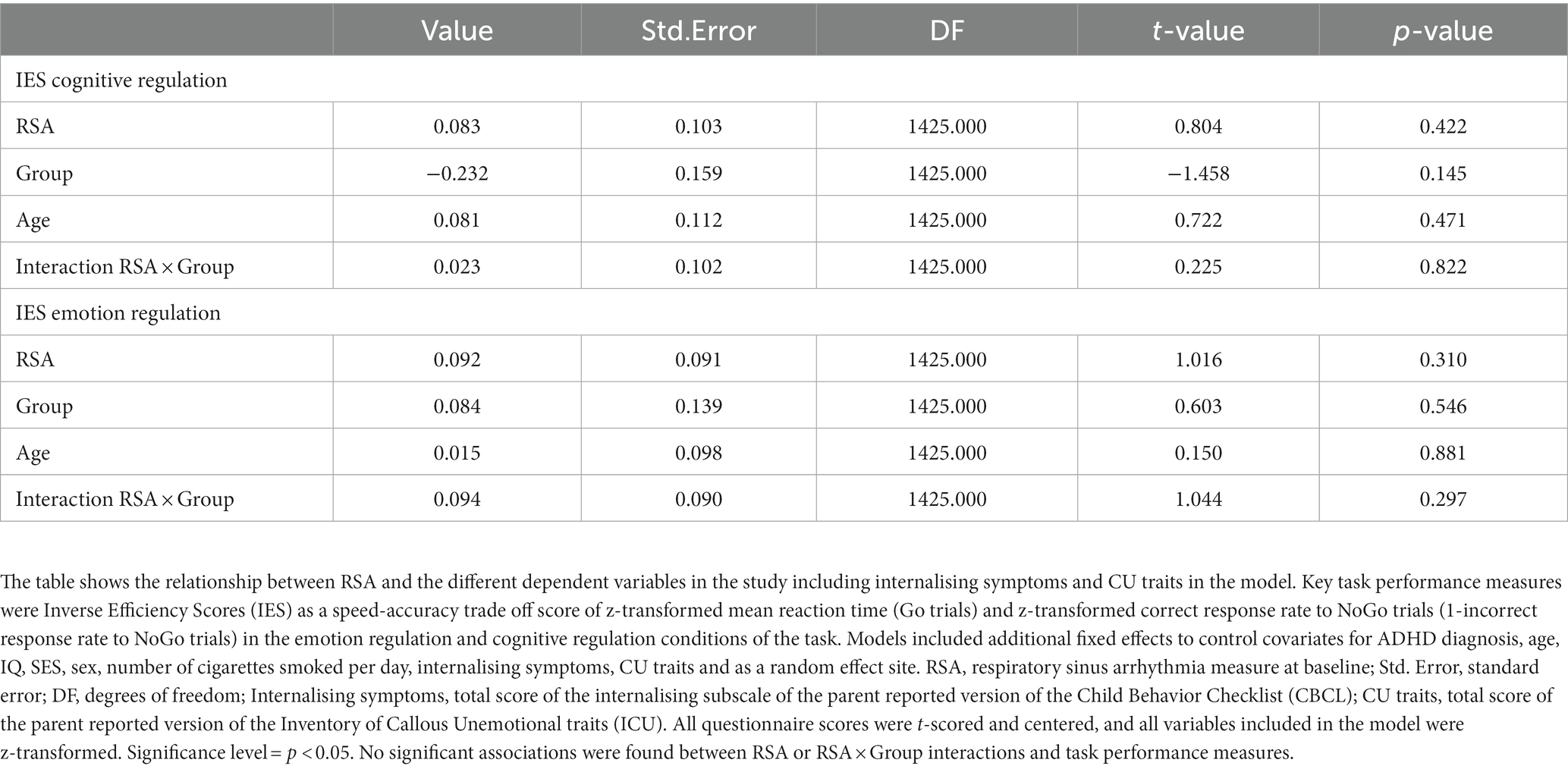
Table 5. Results of multi-level regression analyses on task performance measures including internalising symptoms and CU traits in the model.
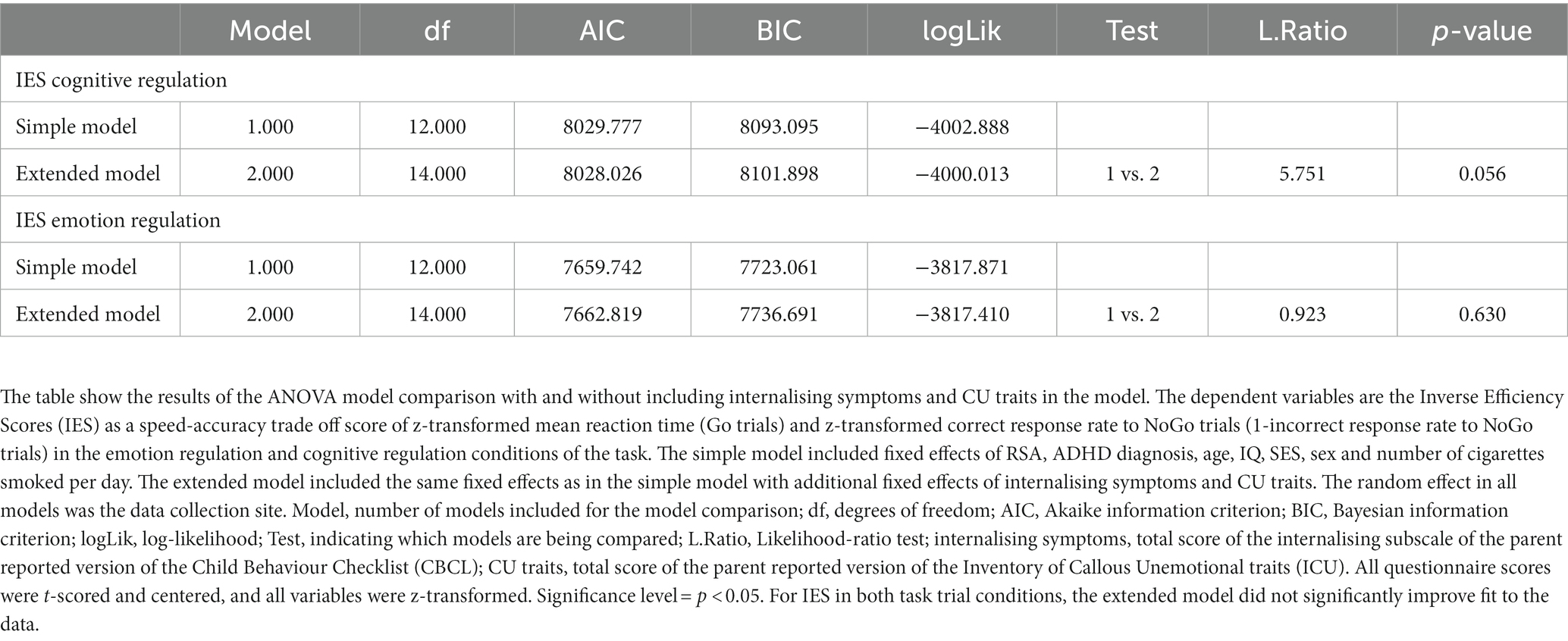
Table 6. ANOVA model comparison: with and without including internalising symptoms and CU traits in the model.
3.2. Structural imaging results
Full factorial analyses showed a significant association between grey matter volume in the left insula and RSA values across all participants (Figure 1, red cluster, pFWE = 0.011, MNI coordinates: −34; 4; 13, 3,416 mm3). There were no significant group, sex or RSA × group interaction effects. To test whether these results would remain significant when only those individuals with higher image quality were included, the analysis was repeated with those participants with image quality B or higher. Results remained essentially unchanged (Figure 1, green cluster, pFWE = 0.034, MNI coordinates: −34, 2, 15; 2,597 mm3).
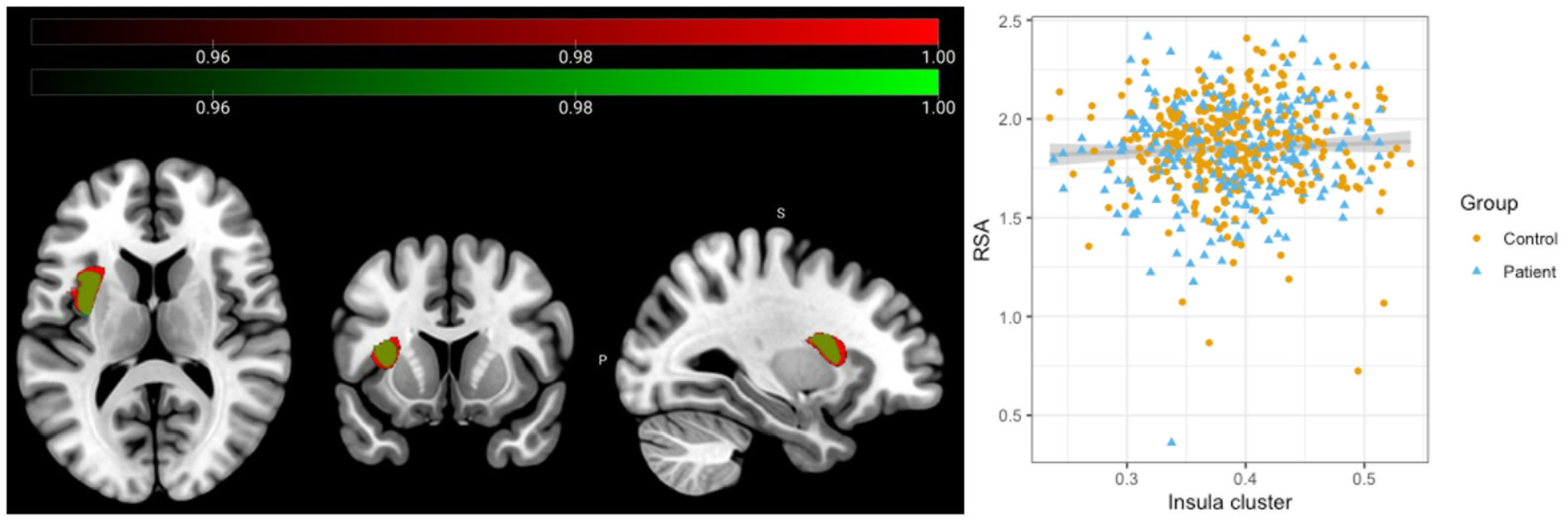
Figure 1. Association between Respiratory Sinus Arrhythmia and Insular grey matter volume across all participants. Results of the full factorial analyses with standardized RSA values included as the main regressor of interest and total intracranial volume (TIV), age, IQ and site as regressors of no interest. A significant positive association was observed across all participants between grey matter volume in the left insula and RSA values (Red cluster, N = 577, MNI coordinates: −34; 4; 13; 3,417 mm3, pFWE = 0.011). Repeating the analysis only including those participants with higher image quality did not change the results significantly (Green cluster, N = 462, MNI coordinates: −34, 2, 15; 2,597 mm3, pFWE = 0.034).
4. Discussion
The current study investigated the association between RSA (as indicator of heart rate variability) and neuropsychological measures of emotion and cognitive regulation in a sample of adolescents with and without a diagnosis of CD. Our results show that RSA was positively associated with grey matter volume in the left insular cortex across all participants, but there were no significant differences between healthy adolescents and those with diagnosis of CD in the strength of the association. However, RSA was not associated with task performance in either cognitive or emotion regulation trials.
Across all participants, individual differences in the specified measure for HRV, RSA, were positively associated with the left insula, key region for emotion and cognitive regulation. Activation in the anterior insula has been associated with sympathetic and parasympathetic activation across a variety of tasks including cognitive, affective and somato-sensory tasks (77), linked to increased autonomic arousal during task performance, and suggested as a potential major site for visceral representations (78). Our results are thus also in line with previous suggestions linking structural and functional abnormalities in the insula with psychopathology (79–81) also showing deficits in the affective and cognitive dimensions of executive function (82).
While the available evidence seems to suggest that right-lateralized neural inputs might be more relevant than their contra-lateral homologous regions for HRV regulation (32, 34, 77) our results show an association across all participants with the left anterior insular cortex volume. This brain region has been implicated in both emotion (83) and cognitive regulation processes (84). As part of the salience network, the insula mediates interactions between other large-scale networks such as the default mode network and central executive network (85). Thus, difficulties in switching between neural circuits in response to environmental demands, also linked with low HRV (14, 86) may indicate a vulnerability to generic self-regulation deficits. However, no significant differences between groups were observed. Thus, the observed association between brain structure and HRV might constitute a vulnerability factor for difficulties in self-regulation, contributing to their subsequent manifestation.
Previous studies have found significant negative associations between insular volumes or thickness and HRV measures in healthy adults (34). In addition, a recent meta-analyses has shown a significant positive association between cortical thickness and HRV measures in a number of regions including lateral orbitofrontal cortex and insular cortex, declining with age (87). However, in this study data of adolescents and young adults (18 year-olds) were analysed together under the assumption of linear association and therefore any potential quadratic trajectories in this association (potentially showing an inverted-U shape) might have been missed. We however, observed a significant positive association, potentially related to neurodevelopmental processes that are still undergoing during adolescence (88, 89). Given the role of the ANS system in supporting the development of the prefrontal cortex (55), further studies will be needed to elucidate the longitudinal differences with increasing age. The protracted maturational processes of crucial prefrontal regions for emotion and cognitive regulation including insular cortex increase the likelihood of difficulties that might contribute to the observed psychopathology (90). The insular body has indeed shown a quadratic developmental trajectory, with increasing cortical thickness during the first two decades of life and decreasing thereafter (89), a trajectory that mirrors the one described for HRV measures (55, 91). On older individuals, associations with cortical thickness on lateral OFC and ACC might be more evident (32, 87, 92). Other studies on the other hand have found no associations between HRV and cortical volumes in healthy adult samples but links with functional connectivity instead (35). This might be relevant given the prominent connectivity patterns described between the anterior and middle insula regions and dorsal anterior cingulate cortex (93).
While our results suggest no significant association between HRV and different behavioural measures of self-regulation, it might potentially be subject to several individually varying factors such as sex or age or to differences in the sensitivity of different HRV measures (52). In addition, although RSA is commonly used to quantify HRV, there is some debate about its sensitivity, whether correction for heart rate or respiration is necessary (94), with other measures of HRV as potential alternatives (36). Furthermore, there is some evidence from adolescents with self-injury behaviours where no association between baseline or reactivity of HRV measures was shown, but only on recovery processes, indicative of a poor ability to regulate response to stressors (28), or an association between HRV recovery but not at baseline with specific cognitive functions (95), a lack of association between inhibitory scores and basal HRV measures but with reactivity during inhibitory performance in preschool-aged children with early adverse experiences (96), or differentiated associations as a function of psychopathological profiles (97). One significant limitation is that our psychophysiological measures of HRV were only acquired at rest. Future studies should ideally combine measures of cardiovagal function at rest with the investigation of phasic changes in HRV within the same individuals, including both reactivity (response during stressors or challenging situations) and recovery (function after stressors) capacities (1), as well as potentially changes in reactivity over time (98). This would possibly provide a more complete picture of the association between HRV and self-regulation behaviours.
According to de Looff et al. (22), psychophysiological effects are also dependent on the experimental task, parameters, and analyses. In addition, while the Emotional Go/NoGo task has been shown to measure both emotion and cognitive regulation (66, 99), the “baseline” condition for the cognitive control condition are emotional facial expressions, therefore requiring the processing of facial emotions. The lack of differences between CD youth and healthy controls in the Emotional Go/NoGo task might be due to the inclusion of emotional faces in both emotional and cognitive regulation task conditions, which might interfere with the elicitation of these regulatory processes distinctively enough. To further investigate the specificity of the association between HRV and emotion regulation, future studies using cognitive control tasks not involving facial emotion processing would be needed.
The results of the extended model after inclusion of internalising problems and CU traits scores suggest that the clinical and symptomatologic heterogeneity of the group of CD participants might have significantly contributed to the difficulty to identify potential group differences in the task. This is in line with previous studies suggesting psychophysiological heterogeneity within patients with antisocial and aggressive behaviours (22, 31). Thus, potential differences between patients and their healthy counterparts might be easier to identify when such symptomatic differentiations within patients is taken into consideration.
In conclusion, we found a positive association between RSA and gray matter volumes in the left anterior insula. This region has been shown to be involved in emotion and cognitive regulation processes, suggesting that HRV is not solely linked with emotion regulation capacities but more with generic self-regulation processes. Since structural and functional abnormalities of the insula have been linked to many mental disorders including CD, the observed association between brain structure and HRV might constitute one risk factor that, in combination with others, might lead to self-regulation difficulties. As the insula also mediates the switching among different neural circuits in response to environmental demands (84) these processes might be affected in the case of low RSA and associated smaller grey matter volumes. Thus, further research should focus on network dysfunctions rather than individual brain regions, the additional use of HRV reactivity and recovery measures in combination with other ANS indices and the use of paradigms measuring clearly differentiated self-regulation aspects. This might then contribute to provide a clearer picture of the neural mechanisms underlying the association between individual differences in HRV and self-regulation deficits.
Data availability statement
The datasets presented in this article are not readily available because the datasets are from the FemNAT-CD multi-country consortium and are available on reasonable request in line with the consortiums’ data sharing policy. Requests to access the datasets should be directed to CS (christina.stadler@upk.ch).
Ethics statement
The studies involving human participants were reviewed and approved by the ethics committee of the medical faculty of the Goethe- University in Frankfurt am Main, Germany. After the coordinating site of the multicenter study, Goethe University Frankfurt, received the approval, local ethics committees from all other sites approved, too. Written informed consent to participate in this study was provided by the participants’ legal guardian/next of kin.
Author contributions
AT and AC designed the project and performed the analysis. HO and EU verified the analytical methods. CS supervised the project. HO, NR, GK, LN-J, AH, AF-R, KK, AP, CF, SB, and GF collected and provided the data. AT and AC wrote the paper with input from all authors. All authors contributed to the article and approved the submitted version.
Funding
This study was supported by the European Commission under the 7th Framework Programme [FemNAT-CD, coordinator CF; grant agreement number 602407]. AT was supported by the Swiss National Science Foundation (Grant no. 100014_185408/1).
Acknowledgments
We thank all members of the FemNAT-CD consortium for their contributions to the project. We would also like to thank all adolescents, families, institutions and professionals who participated in the study.
Conflict of interest
The authors declare that the research was conducted in the absence of any commercial or financial relationships that could be construed as a potential conflict of interest.
Publisher’s note
All claims expressed in this article are solely those of the authors and do not necessarily represent those of their affiliated organizations, or those of the publisher, the editors and the reviewers. Any product that may be evaluated in this article, or claim that may be made by its manufacturer, is not guaranteed or endorsed by the publisher.
Supplementary material
The Supplementary material for this article can be found online at: https://www.frontiersin.org/articles/10.3389/fpsyt.2023.1101064/full#supplementary-material
Footnotes
References
1. Balzarotti, S, Biassoni, F, Colombo, B, and Ciceri, MR. Cardiac vagal control as a marker of emotion regulation in healthy adults: a review. Biol Psychol. (2017) 130:54–66. doi: 10.1016/j.biopsycho.2017.10.008
2. Henry, BL, Minassian, A, Paulus, MP, Geyer, MA, and Perry, W. Heart rate variability in bipolar mania and schizophrenia. J Psychiatr Res. (2010) 44:168–76. doi: 10.1016/j.jpsychires.2009.07.011
3. Kemp, AH, and Quintana, DS. The relationship between mental and physical health: insights from the study of heart rate variability. Int J Psychophysiol. (2013) 89:288–96. doi: 10.1016/j.ijpsycho.2013.06.018
4. Kim, HG, Cheon, EJ, Bai, DS, Lee, YH, and Koo, BH. Stress and heart rate variability: a meta-analysis and review of the literature. Psychiatry Investig. (2018) 15:235–45. doi: 10.30773/pi.2017.08.17
5. Perna, G, Riva, A, Defillo, A, Sangiorgio, E, Nobile, M, and Caldirola, D. Heart rate variability: can it serve as a marker of mental health resilience? J Affect Disord. (2020) 263:754–61. doi: 10.1016/j.jad.2019.10.017
6. Thayer, JF, Yamamoto, SS, and Brosschot, JF. The relationship of autonomic imbalance, heart rate variability and cardiovascular disease risk factors. Int J Cardiol. (2010) 141:122–31. doi: 10.1016/j.ijcard.2009.09.543
7. Thayer, JF, Åhs, F, Fredrikson, M, Sollers, JJ, and Wager, TD. A meta-analysis of heart rate variability and neuroimaging studies: implications for heart rate variability as a marker of stress and health. Neurosci Biobehav Rev. (2012) 36:747–56. doi: 10.1016/j.neubiorev.2011.11.009
8. Appelhans, BM, and Luecken, LJ. Heart rate variability as an index of regulated emotional responding. Rev Gen Psychol. (2006) 10:229–40. doi: 10.1037/1089-2680.10.3.229
9. Benarroch, EE. The central autonomic network: functional organization, dysfunction, and perspective. Mayo Clin Proc. (1993) 68:988–1001. doi: 10.1016/S0025-6196(12)62272-1
10. Thayer, JF, Hansen, AL, Saus-Rose, E, and Johnsen, BH. Heart rate variability, prefrontal neural function, and cognitive performance: the neurovisceral integration perspective on self-regulation, adaptation, and health. Ann Behav Med. (2009) 37:141–53. doi: 10.1007/s12160-009-9101-z
11. Valenza, G, Sclocco, R, Duggento, A, Passamonti, L, Napadow, V, Barbieri, R, et al. The central autonomic network at rest: uncovering functional MRI correlates of time-varying autonomic outflow. NeuroImage. (2019) 197:383–90. doi: 10.1016/j.neuroimage.2019.04.075
12. Verberne, AJM, and Owens, NC. Cortical modulation of the cardiovascular system. Prog Neurobiol. (1998) 54:149–68. doi: 10.1016/S0301-0082(97)00056-7
13. Beauchaine, TP, and Thayer, JF. Heart rate variability as a transdiagnostic biomarker of psychopathology. Int J Psychophysiol. (2015) 98:338–50. doi: 10.1016/j.ijpsycho.2015.08.004
14. Thayer, JF, and Lane, RD. A model of neurovisceral integration in emotion regulation and dysregulation. J Affect Disord. (2000) 61:201–16. doi: 10.1016/S0165-0327(00)00338-4
15. Thayer, JF, and Lane, RD. The role of vagal function in the risk for cardiovascular disease and mortality. Biol Psychol. (2007) 74:224–42. doi: 10.1016/j.biopsycho.2005.11.013
16. Thayer, JF, and Siegle, GJ. Neurovisceral integration in cardiac and emotional regulation. IEEE Eng Med Biol Mag. (2002) 21:24–9. doi: 10.1109/MEMB.2002.1032635
17. Thayer, JF, and Sternberg, E. Beyond heart rate variability: vagal regulation of allostatic systems. Ann N Y Acad Sci. (2006) 1088:361–72. doi: 10.1196/annals.1366.014
18. Williams, DP, Cash, C, Rankin, C, Bernardi, A, Koenig, J, and Thayer, JF. Resting heart rate variability predicts self-reported difficulties in emotion regulation: a focus on different facets of emotion regulation. Front Psychol. (2015) 6:261. doi: 10.3389/fpsyg.2015.00261/abstract
19. Heiss, S, Vaschillo, B, Vaschillo, EG, Timko, CA, and Hormes, JM. Heart rate variability as a biobehavioral marker of diverse psychopathologies: a review and argument for an “ideal range”. Neurosci Biobehav Rev. (2021) 121:144–55. doi: 10.1016/j.neubiorev.2020.12.004
20. Koch, C, Wilhelm, M, Salzmann, S, Rief, W, and Euteneuer, F. A meta-analysis of heart rate variability in major depression. Psychol Med. (2019) 49:1948–57. doi: 10.1017/S0033291719001351
21. Schneider, M, and Schwerdtfeger, A. Autonomic dysfunction in posttraumatic stress disorder indexed by heart rate variability: a meta-analysis. Psychol Med. (2020) 50:1937–48. doi: 10.1017/S003329172000207X
22. de Looff, PC, Cornet, LJM, de Kogel, CH, Fernández-Castilla, B, Embregts, PJCM, Didden, R, et al. Heart rate and skin conductance associations with physical aggression, psychopathy, antisocial personality disorder and conduct disorder: an updated meta-analysis. Neurosci Biobehav Rev. (2022) 132:553–82. doi: 10.1016/j.neubiorev.2021.11.003
23. Portnoy, J, and Farrington, DP. Resting heart rate and antisocial behavior: an updated systematic review and meta-analysis. Aggress Violent Behav. (2015) 22:33–45. doi: 10.1016/j.avb.2015.02.004
24. Duindam, HM, Williams, DP, Asscher, JJ, Hoeve, M, Thayer, JF, and Creemers, HE. Heart-wired to be cold? Exploring cardiac markers of callous-unemotional traits in incarcerated offenders. Int J Psychophysiol. (2021) 170:168–77. doi: 10.1016/j.ijpsycho.2021.10.006
25. Gentzler, AL, Rottenberg, J, Kovacs, M, George, CJ, and Morey, JN. Atypical development of resting respiratory sinus arrhythmia in children at high risk for depression. Dev Psychobiol. (2012) 54:556–67. doi: 10.1002/dev.20614
26. Koenig, J, Kemp, AH, Beauchaine, TP, Thayer, JF, and Kaess, M. Depression and resting state heart rate variability in children and adolescents—a systematic review and meta-analysis. Clin Psychol Rev. (2016) 46:136–50. doi: 10.1016/j.cpr.2016.04.013
27. Rottenberg, J. Cardiac vagal control in depression: a critical analysis. Biol Psychol. (2007) 74:200–11. doi: 10.1016/j.biopsycho.2005.08.010
28. Wielgus, MD, Aldrich, JT, Mezulis, AH, and Crowell, SE. Respiratory sinus arrhythmia as a predictor of self-injurious thoughts and behaviors among adolescents. Int J Psychophysiol. (2016) 106:127–34. doi: 10.1016/j.ijpsycho.2016.05.005
29. Chalmers, JA, Quintana, DS, Abbott, MJA, and Kemp, AH. Anxiety disorders are associated with reduced heart rate variability: a meta-analysis. Front Psychiatry. (2014) 5:80. doi: 10.3389/fpsyt.2014.00080/abstract
30. Fairchild, G, Hawes, DJ, Frick, PJ, Copeland, WE, Odgers, CL, Franke, B, et al. Conduct disorder. Nat Rev Dis Primer. (2019) 5:43. doi: 10.1038/s41572-019-0095-y
31. Fanti, KA. Understanding heterogeneity in conduct disorder: a review of psychophysiological studies. Neurosci Biobehav Rev. (2018) 91:4–20. doi: 10.1016/j.neubiorev.2016.09.022
32. Winkelmann, T, Thayer, JF, Pohlack, S, Nees, F, Grimm, O, and Flor, H. Structural brain correlates of heart rate variability in a healthy young adult population. Brain Struct Funct. (2017) 222:1061–8. doi: 10.1007/s00429-016-1185-1
33. Woodward, SH. Right anterior cingulate cortical volume covaries with respiratory sinus arrhythmia magnitude in combat veterans. J Rehabil Res Dev. (2008) 45:451–64. doi: 10.1682/JRRD.2007.06.0082
34. Wei, L, Chen, H, and Wu, GR. Heart rate variability associated with grey matter volumes in striatal and limbic structures of the central autonomic network. Brain Res. (2018) 1681:14–20. doi: 10.1016/j.brainres.2017.12.024
35. Kumral, D, Schaare, HL, Beyer, F, Reinelt, J, Uhlig, M, Liem, F, et al. The age-dependent relationship between resting heart rate variability and functional brain connectivity. NeuroImage. (2019) 185:521–33. doi: 10.1016/j.neuroimage.2018.10.027
36. Shaffer, F, and Ginsberg, JP. An overview of heart rate variability metrics and norms. Front Public Health. (2017) 5:258. doi: 10.3389/fpubh.2017.00258
37. Alegria, AA, Radua, J, and Rubia, K. Meta-analysis of fMRI studies of disruptive behavior disorders. Am J Psychiatry. (2016) 173:1119–30. doi: 10.1176/appi.ajp.2016.15081089
38. Noordermeer, SDS, Luman, M, and Oosterlaan, J. A systematic review and meta-analysis of neuroimaging in oppositional defiant disorder (ODD) and conduct disorder (CD) taking attention-deficit hyperactivity disorder (ADHD) into account. Neuropsychol Rev. (2016) 26:44–72. doi: 10.1007/s11065-015-9315-8
39. Raschle, NM, Menks, WM, Fehlbaum, LV, Tshomba, E, and Stadler, C. Structural and functional alterations in right dorsomedial prefrontal and left insular cortex co-localize in adolescents with aggressive behaviour: an ALE meta-analysis. McKenna PJ, Ed. PLOS ONE. (2015); 10:e0136553, doi: 10.1371/journal.pone.0136553
40. Raschle, NM, Fehlbaum, LV, Menks, WM, Martinelli, A, Prätzlich, M, Bernhard, A, et al. Atypical dorsolateral prefrontal activity in female adolescents with conduct disorder during effortful emotion regulation. Biol Psychiatry Cogn Neurosci Neuroimaging. (2019) 4:984–94. doi: 10.1016/j.bpsc.2019.05.003
41. Rogers, JC, and De Brito, SA. Cortical and subcortical gray matter volume in youths with conduct problems: a meta-analysis. JAMA Psychiat. (2016) 73:64–72. doi: 10.1001/jamapsychiatry.2015.2423
42. Blair, RJR, Leibenluft, E, and Pine, DS. Conduct disorder and callous–unemotional traits in youth. N Engl J Med. (2014) 371:2207–16. doi: 10.1056/NEJMra1315612
43. Blair, RJR, Veroude, K, and Buitelaar, JK. Neuro-cognitive system dysfunction and symptom sets: a review of fMRI studies in youth with conduct problems. Neurosci Biobehav Rev. (2018) 91:69–90. doi: 10.1016/j.neubiorev.2016.10.022
44. Burke, JD, Hipwell, AE, and Loeber, R. Dimensions of oppositional defiant disorder as predictors of depression and conduct disorder in preadolescent girls. J Am Acad Child Adolesc Psychiatry. (2010) 49:484–92.
45. Frick, PJ, and Viding, E. Antisocial behavior from a developmental psychopathology perspective. Dev Psychopathol. (2009) 21:1111–31. doi: 10.1017/S0954579409990071
46. Kohls, G, Fairchild, G, Bernhard, A, Martinelli, A, Smaragdi, A, Gonzalez-Madruga, K, et al. Neuropsychological subgroups of emotion processing in youths with conduct disorder. Front Psych. (2020) 11:585052. doi: 10.3389/fpsyt.2020.585052
47. Insel, T, Cuthbert, B, Garvey, M, Heinssen, R, Pine, DS, Quinn, K, et al. Research domain criteria (RDoC): toward a new classification framework for research on mental disorders. Am J Psychiatry. (2010) 167:748–51. doi: 10.1176/appi.ajp.2010.09091379
48. Oldenhof, H, Prätzlich, M, Ackermann, K, Baker, R, Batchelor, M, Baumann, S, et al. Baseline autonomic nervous system activity in female children and adolescents with conduct disorder: psychophysiological findings from the FemNAT-CD study. J Crim Justice. (2019) 65:101564. doi: 10.1016/j.jcrimjus.2018.05.011
49. Prätzlich, M, Oldenhof, H, Steppan, M, Ackermann, K, Baker, R, Batchelor, M, et al. Resting autonomic nervous system activity is unrelated to antisocial behaviour dimensions in adolescents: cross-sectional findings from a European multi-Centre study. J Crim Justice. (2019) 65:101536. doi: 10.1016/j.jcrimjus.2018.01.004
50. Fanti, KA, Eisenbarth, H, Goble, P, Demetriou, C, Kyranides, MN, Goodwin, D, et al. Psychophysiological activity and reactivity in children and adolescents with conduct problems: a systematic review and meta-analysis. Neurosci Biobehav Rev. (2019) 100:98–107. doi: 10.1016/j.neubiorev.2019.02.016
51. Holzman, JB, and Bridgett, DJ. Heart rate variability indices as bio-markers of top-down self-regulatory mechanisms: a meta-analytic review. Neurosci Biobehav Rev. (2017) 74:233–55. doi: 10.1016/j.neubiorev.2016.12.032
52. Zahn, D, Adams, J, Krohn, J, Wenzel, M, Mann, CG, Gomille, LK, et al. Heart rate variability and self-control—a meta-analysis. Biol Psychol. (2016) 115:9–26. doi: 10.1016/j.biopsycho.2015.12.007
53. Robson, DA, Allen, MS, and Howard, SJ. Self-regulation in childhood as a predictor of future outcomes: a meta-analytic review. Psychol Bull. (2020) 146:324–54. doi: 10.1037/bul0000227
54. Williams, PG, Cribbet, MR, Tinajero, R, Rau, HK, Thayer, JF, and Suchy, Y. The association between individual differences in executive functioning and resting high-frequency heart rate variability. Biol Psychol. (2019) 148:107772. doi: 10.1016/j.biopsycho.2019.107772
55. Koenig, J. Neurovisceral regulatory circuits of affective resilience in youth: principal outline of a dynamic model of neurovisceral integration in development. Psychophysiology. (2020) 57:e13568. doi: 10.1111/psyp.13568
56. American Psychiatric Association. Diagnostic and statistical manual of mental disorders [internet]. 5th. American Psychiatric Association; (2013), (cited 2022 Jan 21). Available at: https://psychiatryonline.org/doi/book/10.1176/appi.books.9780890425596
57. Kaufman, J, Birmaher, B, Brent, D, Rao, U, Flynn, C, Moreci, P, et al. Schedule for affective disorders and schizophrenia for school-age children-present and lifetime version (K-SADS-PL): initial reliability and validity data. J Am Acad Child Adolesc Psychiatry. (1997) 36:980–8. doi: 10.1097/00004583-199707000-00021
58. Achenbach, TM. Rescorla LA. Manual for ASEBA school-age forms & profiles Univ Vt Res Cent Child Youth Fam (2001).
59. Frick, PJ. Inventory of callous–unemotional traits. American Psychological Association; (2017) (cited January 21, 2022). Available at: http://doi.apa.org/getdoi.cfm?doi=10.1037/t62639-000
60. ISCO - International Standard Classification of Occupations (Cited November 10, 2022). (2022), Available at: https://www.ilo.org/public/english/bureau/stat/isco/index.htm
61. International Standard Classification of Education (ISCED) [Internet] (Cited November 10, 2022). (2017), Available at: https://uis.unesco.org/en/topic/international-standard-classification-education-isced
63. Wechsler, D. Wechsler intelligence scale for children. 4th Edn. (WISC-IV San Antonio TX Psychol Corp (2003). 3 p.
64. Wechsler, D. Wechsler adult intelligence scale, 4th (WAIS-IV). San Antonio TX NCS Pearson. (2008);22:1.
65. Hare, TA, Tottenham, N, Galvan, A, Voss, HU, Glover, GH, and Casey, BJ. Biological substrates of emotional reactivity and regulation in adolescence during an emotional Go-NoGo task. Biol Psychiatry. (2008) 63:927–34. doi: 10.1016/j.biopsych.2008.03.015
66. Tottenham, N, Hare, TA, and Casey, BJ. Behavioral assessment of emotion discrimination, emotion regulation, and cognitive control in childhood, adolescence, and adulthood. Front Psychol. (2011) 2, 2:637. doi: 10.3389/fpsyg.2011.00039/abstract
67. Townsend, JT, and Ashby, FG. Stochastic modeling of elementary psychological processes CUP Archive (1983).
68. Beauchaine, TP, and Bell, ZE. Respiratory sinus arrhythmia as a transdiagnostic biomarker of emotion dysregulation. Oxf Handb Emot Dysregulation (2020) 153–166.
69. de Geus, E, van Lien, R, Neijts, M, and Willemsen, G. Genetics of autonomic nervous system activity. In T. Canli editor. The Oxford handbook of molecular psychology. Oxford University Press. (2015). 357–390.
70. Beauchaine, TP, Bell, Z, Knapton, E, McDonough-Caplan, H, Shader, T, and Zisner, A. Respiratory sinus arrhythmia reactivity across empirically based structural dimensions of psychopathology: a meta-analysis. Psychophysiology. (2019) 56:e13329. doi: 10.1111/psyp.13329
71. Team RC. R Core Team (2017). R: a language and environment for statistical computing. R Found Stat Comput Vienna Austria 2017
72. Van, Buuren S, and Groothuis-Oudshoorn, K. Multivariate imputation by chained equations in R. J Stat Softw (2011) 45. Available at: http://www.jstatsoft.org/v45/i03/
73. Pinheiro, J, Bates, D, Debroy, S, and Sarkar, D. R Core team. 2018. NLME: linear and nonlinear mixed effects models. R package version 3. 2018.
74. Gaser, C, Dahnke, R, Thompson, PM, Kurth, F, and Luders, E. Initiative A.D.N. CAT—A Computational Anatomy Toolbox for the Analysis of Structural MRI Data. BioRxiv. (2022). doi: 10.1101/2022.06.11.495736
75. Statistical parametric mapping [internet]. Elsevier; (2007) (cited January 21, 2022). Available at: https://linkinghub.elsevier.com/retrieve/pii/B9780123725608X50001
76. Wilke, M, Altaye, M, and Holland, SK. The CMIND authorship consortium. CerebroMatic: a versatile toolbox for spline-based MRI template creation. Front Comput Neurosci. (2017) 22:11. doi: 10.3389/fncom.2017.00005/full
77. Beissner, F, Meissner, K, Bar, KJ, and Napadow, V. The autonomic brain: an activation likelihood estimation meta-analysis for central processing of autonomic function. J Neurosci. (2013) 33:10503–11. doi: 10.1523/JNEUROSCI.1103-13.2013
78. Critchley, HD, Nagai, Y, Gray, MA, and Mathias, CJ. Dissecting axes of autonomic control in humans: insights from neuroimaging. Auton Neurosci. (2011) 161:34–42. doi: 10.1016/j.autneu.2010.09.005
79. Downar, J, Blumberger, DM, and Daskalakis, ZJ. The neural crossroads of psychiatric illness: an emerging target for brain stimulation. Trends Cogn Sci. (2016) 20:107–20. doi: 10.1016/j.tics.2015.10.007
80. Goodkind, M, Eickhoff, SB, Oathes, DJ, Jiang, Y, Chang, A, Jones-Hagata, LB, et al. Identification of a common neurobiological substrate for mental illness. JAMA Psychiat. (2015) 72:305. doi: 10.1001/jamapsychiatry.2014.2206
81. Ferraro, S, Klugah-Brown, B, Tench, CR, Bazinet, V, Bore, MC, Nigri, A, et al. The central autonomic system revisited – convergent evidence for a regulatory role of the insular and midcingulate cortex from neuroimaging meta-analyses. Neurosci Biobehav Rev. (2022) 142:104915. doi: 10.1016/j.neubiorev.2022.104915
82. Namkung, H, Kim, SH, and Sawa, A. The insula: an underestimated brain area in clinical neuroscience, psychiatry, and neurology. Trends Neurosci. (2017) 40:200–7. doi: 10.1016/j.tins.2017.02.002
83. Uddin, LQ, Nomi, JS, Hébert-Seropian, B, Ghaziri, J, and Boucher, O. Structure and function of the human insula. J Clin Neurophysiol. (2017) 34:300–6. doi: 10.1097/WNP.0000000000000377
84. Molnar-Szakacs, I, and Uddin, LQ. Anterior insula as a gatekeeper of executive control. Neurosci Biobehav Rev. (2022) 139:104736. doi: 10.1016/j.neubiorev.2022.104736
85. Menon, V, and D’Esposito, M. The role of PFC networks in cognitive control and executive function. Neuropsychopharmacology. (2022) 47:90–103. doi: 10.1038/s41386-021-01152-w
86. Thayer, JF, and Brosschot, JF. Psychosomatics and psychopathology: looking up and down from the brain. Psychoneuroendocrinology. (2005) 30:1050–8. doi: 10.1016/j.psyneuen.2005.04.014
87. Koenig, J, Abler, B, Agartz, I, Åkerstedt, T, Andreassen, OA, Anthony, M, et al. Cortical thickness and resting-state cardiac function across the lifespan: a cross-sectional pooled mega-analysis. Psychophysiology. (2021) 58:e13688. doi: 10.1111/psyp.13688
88. Giedd, JN, Blumenthal, J, Jeffries, NO, Castellanos, FX, Liu, H, Zijdenbos, A, et al. Brain development during childhood and adolescence: a longitudinal MRI study. Nat Neurosci. (1999) 2:861–3. doi: 10.1038/13158
89. Shaw, P, Kabani, NJ, Lerch, JP, Eckstrand, K, Lenroot, R, Gogtay, N, et al. Neurodevelopmental trajectories of the human cerebral cortex. J Neurosci. (2008) 28:3586–94. doi: 10.1523/JNEUROSCI.5309-07.2008
90. Beauchaine, TP, and Cicchetti, D. Emotion dysregulation and emerging psychopathology: a transdiagnostic, transdisciplinary perspective. Dev Psychopathol. (2019) 31:799–804. doi: 10.1017/S0954579419000671
91. Silvetti, MS, Drago, F, and Ragonese, P. Heart rate variability in healthy children and adolescents is partially related to age and gender. Int J Cardiol. (2001) 81:169–74. doi: 10.1016/S0167-5273(01)00537-X
92. Yoo, HJ, Thayer, JF, Greening, S, Lee, TH, Ponzio, A, Min, J, et al. Brain structural concomitants of resting state heart rate variability in the young and old: evidence from two independent samples. Brain Struct Funct. (2018) 223:727–37. doi: 10.1007/s00429-017-1519-7
93. Deen, B, Pitskel, NB, and Pelphrey, KA. Three systems of insular functional connectivity identified with cluster analysis. Cereb Cortex. (2011) 21:1498–506. doi: 10.1093/cercor/bhq186
94. de Geus, EJC, Gianaros, PJ, Brindle, RC, Jennings, JR, and Berntson, GG. Should heart rate variability be “corrected” for heart rate? Biological, quantitative, and interpretive considerations: XXXX. Psychophysiology. (2019) 56:e13287. doi: 10.1111/psyp.13287
95. Kimhy, D, Crowley, OV, McKinley, PS, Burg, MM, Lachman, ME, Tun, PA, et al. The association of cardiac vagal control and executive functioning – findings from the MIDUS study. J Psychiatr Res. (2013) 47:628–35. doi: 10.1016/j.jpsychires.2013.01.018
96. Skowron, EA, Cipriano-Essel, E, Gatzke-Kopp, LM, Teti, DM, and Ammerman, RT. Early adversity, RSA, and inhibitory control: evidence of children’s neurobiological sensitivity to social context: early adversity, RSA, and inhibitory control. Dev Psychobiol. (2014) 56:964–78. doi: 10.1002/dev.21175
97. Deutz, MHF, Woltering, S, Vossen, HGM, Deković, M, van Baar, AL, and Prinzie, P. Underlying psychophysiology of dysregulation: resting heart rate and heart rate reactivity in relation to childhood dysregulation. J Am Acad Child Adolesc Psychiatry. (2019) 58:589–99. doi: 10.1016/j.jaac.2018.09.434
98. Hinnant, JB, Philbrook, LE, Erath, SA, and El-Sheikh, M. Approaches to modeling the development of physiological stress responsivity. Psychophysiology. (2018) 55:e13027. doi: 10.1111/psyp.13027
Keywords: heart rate variabiity, emotion regualtion, self-regulation, brain structure, central autonomic network, conduct disorder
Citation: Cubillo A, Tkalcec A, Oldenhof H, Unternaehrer E, Raschle N, Kohls G, Nauta-Jansen L, Hervas A, Fernandez-Rivas A, Konrad K, Popma A, Freitag C, de Brito S, Fairchild G and Stadler C (2023) Linking heart rate variability to psychological health and brain structure in adolescents with and without conduct disorder. Front. Psychiatry 14:1101064. doi: 10.3389/fpsyt.2023.1101064
Edited by:
Silvia Pellegrini, University of Pisa, ItalyReviewed by:
Svenja Taubner, Heidelberg University, GermanyBrandi C. Fink, University of Oklahoma Health Sciences Center, United States
Copyright © 2023 Cubillo, Tkalcec, Oldenhof, Unternaehrer, Raschle, Kohls, Nauta-Jansen, Hervas, Fernandez-Rivas, Konrad, Popma, Freitag, de Brito, Fairchild and Stadler. This is an open-access article distributed under the terms of the Creative Commons Attribution License (CC BY). The use, distribution or reproduction in other forums is permitted, provided the original author(s) and the copyright owner(s) are credited and that the original publication in this journal is cited, in accordance with accepted academic practice. No use, distribution or reproduction is permitted which does not comply with these terms.
*Correspondence: Antonia Tkalcec, YW50b25pYS50a2FsY2VjQHVway5jaA==
†These authors have contributed equally to this work and share first authorship