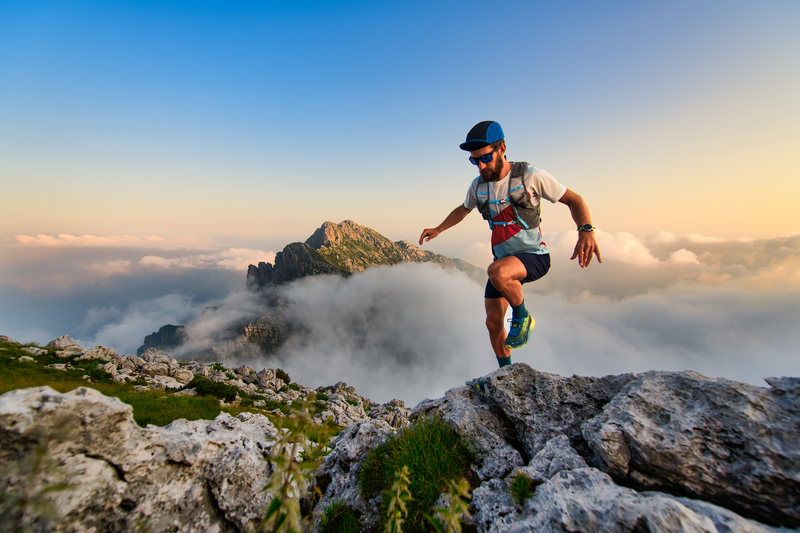
95% of researchers rate our articles as excellent or good
Learn more about the work of our research integrity team to safeguard the quality of each article we publish.
Find out more
ORIGINAL RESEARCH article
Front. Psychiatry , 16 March 2023
Sec. ADHD
Volume 14 - 2023 | https://doi.org/10.3389/fpsyt.2023.1093522
This article is part of the Research Topic Attention Deficit and Development: Brain Mechanisms, Assessment, and Intervention View all 4 articles
Introduction: Attention-deficit/hyperactivity disorder (ADHD) is a neuropsychiatric disorder whose primary symptoms are hyperactivity, impulsivity, and inattention. Historically, ADHD was recognized as a disease of childhood and adolescence. However, many patients are known to have persistent symptoms into adulthood. Many researchers consider the neuropathology of ADHD to be based on abnormalities in multiple parallel and intersecting pathways rather than a single anatomical area, but such alterations remain to be clarified.
Methods: Using diffusion tensor imaging, we investigated differences in the global network metrics estimated by graph theory and the degree of connectivity between adjacent voxels within a white matter (WM) fascicle defined by the density of the diffusing spins (connectometry) between 19 drug-naive Japanese patients with adult ADHD and 19 matched healthy controls (HCs). In adult patients with ADHD, we examined the relationships between the symptomatology of ADHD and global network metrics and WM abnormalities.
Results: Compared with HCs, adult patients with ADHD showed a reduced rich-club coefficient and decreased connectivity in widely distributed WMs such as the corpus callosum, the forceps, and the cingulum bundle. Correlational analyses demonstrated that the general severity of ADHD symptoms was associated with several global network metrics, such as lower global efficiency, clustering coefficient, small worldness, and longer characteristic path length. The connectometry revealed that the severity of hyperactive/impulsive symptoms was associated with overconnectivity in the corticostriatal, corticospinal, and corticopontine tracts, the inferior fronto-occipital fasciculus, and the extreme capsule but dysconnectivity in the cerebellum. The severity of inattentive symptoms was associated with dysconnectivity in the intracerebellar circuit and some other fibers.
Conclusion: The results of the present study indicated that patients with treatment-naive adult ADHD showed disrupted structural connectivity, which contributes to less efficient information transfer in the ADHD brain and pathophysiology of ADHD.
Trial registration: UMIN Clinical Trials Registry (UMIN-CTR) UMIN000025183, Registered: 5 January 2017.
Attention-deficit/hyperactivity disorder (ADHD) is a neuropsychiatric disease that usually appears in childhood and is characterized by hyperactivity, increased impulsivity, and developmentally inappropriate inattention. ADHD is known to affect children; however, a recent World Health Organization (WHO) survey on global mental health found that the prevalence of patients with adult ADHD was 2.8% on average (1). Growing evidence suggests that structural abnormalities in the brain may contribute to the pathophysiology of ADHD (2). However, a recent meta-analysis of functional and structural MRI studies on children and adolescents with ADHD found no significant convergence across structural and functional regional alterations in ADHD, suggesting that the pathophysiology of ADHD might be based on network interactions rather than a regional abnormality (3). Indeed, a recent study demonstrated that the neuropathology of ADHD is based on multiple parallel and intersecting pathways rather than a single anatomical area, which demonstrated altered functional connectivity in ADHD brains (2).
Diffusion tensor imaging, which is believed to be an indicator of fiber tract integrity, reflecting coherence, organization, and/or density of fiber bundles in the white matter of the brain, is a promising in vivo method that has made it possible to investigate white matter abnormalities in neuropsychiatric disorders (4). According to a recent meta-analysis, the most common WM abnormality in ADHD was a decrease in fractional anisotropy (FA) in the corpus callosum splenium (5). However, DTI studies of patients with adult ADHD have been limited, and the results are relatively inconsistent (6, 7). A possible factor contributing to inconsistent findings in adult patients with ADHD is differences between the ADHD participants with a history of stimulant treatment, such as the difference observed on the starting period of the administration of methylphenidate (MPH) (6). A previous randomized study investigating the effects of MPH treatment in male patients with ADHD reported that MPH affects FA in association tracts of the left hemisphere and the corpus callosum in children but not in adolescent and adult patients (8). Similar to the human study, an experimental study in adolescent rats reported increases in FA values in the corpus callosum after MPH administration (9). Furthermore, a study showed that the striatal genes associated with axonal myelination are upregulated by the administration of MPH in juveniles (10). Since patients with adult ADHD often consume stimulants for prolonged periods (6), such data indicated that WM integrity is affected by the treatment of MPH, and studies involving medication-naive patients with adult ADHD should be undertaken to understand the pathophysiology of adult ADHD.
However, DTI studies conducted on medication-naive patients with adult ADHD are still limited (6). Moreover, a previous DTI study estimated FA with the region of interest method and tract-based spatial statistics (6). Therefore, to conduct more comprehensive analyses to investigate WM abnormalities and its associated abnormal brain network topology in treatment-naive patients with adult ADHD, we applied conventional graph theory analysis for connectomics and the “local connectometry” (11) to investigate differences in global network metrics estimated by graph theory and the degree of connectivity between adjacent voxels within a WM fascicle defined by the density of the diffusing spins (11) between healthy controls (HCs) and patients with adult ADHD. Furthermore, we examined relationships between the symptomatology of ADHD and global network metrics and WM abnormalities. Diffusion MRI connectometry was used to derive the correlational tractography that has qualitative anisotropy (QA) correlated with the diagnosis effect and symptom severity of ADHD (11). Diffusion MRI connectometry is a method to estimate the diffusion quantities along the local tract orientation (11).
We hypothesize that, similar to untreated children with ADHD, untreated patients with adult ADHD show disintegrity of the white matter which is involved in the impairment of complex networks in the brain and contributes to the pathogenesis of adult patients with ADHD.
The call for participants was opened on 1 February 2017 and closed with the entry of the final participant on 1 June 2022. Patients with adult ADHD were recruited not only from the outpatient unit of psychiatry at the Fukushima Medical University but also from neighboring psychiatric hospitals; after explaining the purpose of the study, potential study participants were referred to the outpatient unit of psychiatry at Fukushima Medical University. In the current study, 20 patients with treatment-naive adult ADHD (M:F = 11:9) and 20 healthy controls (HCs) (M:F = 11:9) participated. From the Fukushima Medical University Hospital's outpatient service, we recruited participants with adult ADHD. At least two qualified psychiatrists diagnosed patients using the criteria outlined in the Diagnostic and Statistical Manual of Mental Disorders, Fifth Edition (DSM-5). Participants with comorbid psychiatric disorders other than ADHD were excluded using the structured clinical interview for the DSM-5 clinical version. The healthy controls were recruited through local advertisements at Fukushima Medical University. Participants having neurological or medical conditions that could potentially affect the central nervous system, such as atypical headache, history of head trauma with loss of consciousness, thyroid disease, epilepsy, seizures, substance-related disorders, or mental retardation, were excluded. Participants with a history of illicit drug use, antidepressants (at least not over the last 3 months), or other psychoactive medication were excluded. The level of intellectual performance of the participants was evaluated with the Japanese version of the Wechsler Adult Intelligence Scale III (WAIS-III) test. The WAIS-III test provides a standardized full-scale intelligence quotient (IQ) based on subtests that measure the level of verbal [verbal IQ (VIQ)] and non-verbal knowledge and reasoning [performance IQ (PIQ)]. We obtained written informed consent from all participants before participation. The presence and severity of ADHD symptoms in both ADHD and HCs were assessed using the Conners' Adult Attention-Deficit/Hyperactivity Disorder Rating Scale (CAARS). The Research Ethics Committee of Fukushima Medical University approved this study (Approval code: no. 2693, Approval data: 13 April 2016). The study was organized and carried out in line with the Declaration of Helsinki.
Due to the poor image quality of the diffusion tensor imaging, two participants (one ADHD and one HC) were excluded from the analyses.
The MRI data were acquired on a Siemens 3T Biograph mMR scanner with a 12-channel phased array coil. DTI acquisition involved a single-shot, spin-echo planar imaging sequence in contiguous axial planes that covered the whole brain. Diffusion-sensitizing gradients were applied in 30 non-collinear directions, together with acquisition without diffusion weighting (b = 0). The imaging parameters were set to the following values: TR = 10,100 ms, TE = 78 ms, average = 1, b-value = 1,000 s/mm2, slice thickness = 2.5 mm, and 60 slices. The matrix resolution was acquired at 96 × 96 and reconstructed to 96 × 96. The resolution was 2.5 × 2.5 × 2.5 mm3.
DICOM files were converted to SRC files by using DSI Studio. The SRC files were examined during the quality control procedure (12), and the data from two participants were excluded because they did not pass the quality control check. The SRC files were reconstructed in the MNI space using q-space diffeomorphic reconstruction (13) to obtain the spin distribution function (14). Since the b-value of acquired DTI was lower than 4,000 s/mm2, we applied the advanced option of “no high b for DTI.” A diffusion sampling length ratio of 1.25 was used. The output resolution in diffeomorphic reconstruction was 2.5-mm isotropic. The restricted diffusion was quantified using restricted diffusion imaging (15). The tensor metrics were calculated using DWI (11) with a b-value of lower than 1,000 s/mm2. The quantitative anisotropy (QA) was extracted as the local connectome fingerprint (16) and used in the connectometry analysis.
As the sample size of this study was relatively small, the normal distribution and equal variances were evaluated using the Shapiro–Wilk test and Levene's test, and if these were not guaranteed, then non-parametric tests such as the Mann–Whitney U-test and Spearman's rank-order correlation were used.
The chi-squared tests were used for gender and handedness, and independent two-sample t-tests were used for age, IQ, and CARRS between patients with adult ADHD and HCs. Statistical analyses were performed using R Statistical Software Version 3.1.0 (Foundation for Statistical Computing, Vienna, Austria).
The connectivity matrix (adjacency matrix) and graph theoretical analyses were conducted using DSI Studio and the brain connectivity toolbox (12, 17). The following procedures were used in the graph theoretical analysis. The first step was to create a tractography map from the DTI data, which included reading and parsing digital imaging and communications in medicine files, reconstructing it to characterize the main diffusion directions of the fibers and fiber tracking (11). The next step was to generate a connectivity matrix, which was calculated using the counts of the connecting tracts (11). The Desikan–Killiany–Tourville atlas was used for brain parcellation. This step included obtaining the whole-brain fiber tracks, placing seeding regions in the whole brain and spatial normalization, defining the region of interest, and creating a connectivity matrix (11). The graph theoretical network measures were then calculated from the connectivity matrix by using the brain connectivity toolbox (brain-connectivity-toolbox.net) (17). A graph is defined as a set of nodes and the edges or lines between them. The threshold was 0.001 to filter out matrix entries with a small number of connecting tracks. It is a ratio to the maximum connecting tracks in the connectivity matrix. The topology of graphs can be quantitatively described by a variety of measures. In this study, we used weighted graphs and evaluated the following global network metrics (17): (1) graph density, (2) global efficiency, (3) clustering coefficient, (4) characteristic path length, (5) small worldness, and (6) rich-club coefficient (18).
An independent two-sample t-test or a Mann–Whitney U-test was used to evaluate the diagnostic effect on each global network metric. Pearson's correlation coefficient or Spearman's rank-order correlation was computed to assess the relationship between scores of ADHD symptoms (T-score of CAARS, DSM-IV inattentive symptoms, DSM-IV hyperactive/impulsive, DSM-IV total symptoms, and ADHD index), and global network metrics. These statistical analyses were performed using R Statistical Software Version 3.1.0 (Foundation for Statistical Computing, Vienna, Austria).
Local connectometry was conducted using DSI Studio (11). To test the diagnostic effect, a non-parametric Spearman partial correlation was used to derive the correlation, and the effect of FIQ was removed using a multiple regression model. To explore the relationship between symptomatic severity in adult ADHD and QA, a non-parametric Spearman partial correlation was used to derive the correlation between T-scores of DSM-IV hyperactive–impulsive and DSM-IV inattentive score and QA, and the effects of FIQ, age, and sex were eliminated by using a multiple regression model. A T-score threshold of 3 was assigned and tracked using a deterministic fiber tracking algorithm (19) to obtain correlational tractography. The QA values were normalized. The seeding was placed on the whole brain. The tracks were filtered by topology-informed pruning (20) with four iteration(s). A false discovery rate (FDR) threshold of 0.05 was used to select tracks. A total of 4,000 randomized permutations were applied to the group label to obtain the null distribution of the track length to estimate the FDR.
The Shapiro–Wilk test indicated that the data were consistent with a normal distribution at a significant level of 0.05. Levene's test indicated that the equality of the error variances was assumed at a significant level of 0.05. Table 1 shows the demographic and clinical characteristics of patients with adult ADHD and HCs. All participants were unrelated Japanese. Data obtained from 19 patients with adult ADHD (mean age: 23.89, men:women = 11:8) and 19 HCs (mean age: 25.00, men:women = 11:8) were analyzed (Table 1). Regarding the type of ADHD, 11 patients belonged to the inattentive type, and eight patients belonged to the combined type. Patients with adult ADHD and HCs did not differ significantly in age, gender, and handedness (Table 1). Patients with adult ADHD exhibited significantly lower IQ and higher CAARS than those with HCs (Table 1).
Except for the clustering coefficient in the ADHD and HCs, the Shapiro–Wilk test indicated that the data were consistent with a normal distribution at a significant level of 0.05. Levene's test indicated that the equality of the error variances was assumed at a significant level of 0.05. Therefore, a comparison between adult ADHD and HCs in the clustering coefficient was done with a Mann–Whitney U-test, and a correlational analysis between the ADHD score and clustering coefficient was done with a Spearman's rank-order correlation. Other parameters were tested by parametric analysis (two-sample t-test and Pearson's correlation analysis). Table 2 shows the results of the graph theoretical analysis. We found a statistically significant difference between groups in the rich-club coefficient (k = 15) (P = 0.48, 95% CI = −0.143–−0.0001). Patients with adult ADHD had significantly lower rich-club coefficients compared with HCs. Statistically significant differences between groups in the network density, global efficiency, clustering coefficient, characteristic path length, and small worldness were not found.
There were negative correlations between the T-score of the ADHD index and global efficiency (r = −0.481, 95% CI −0.768–−0.0346, P = 0.037) (Figure 1, upper left), clustering coefficient (r = −0.56, P = 0.0127, Spearman's rank-order correlation) (Figure 1, upper right), and small worldness (r = −0.603, 95% CI −0.83–−0.205, P = 0.0062) (Figure 1, lower left). There was a positive correlation between the T-score of the ADHD index and characteristic path length (r = 0.454, 95% CI 0.000629–0.753, P = 0.0495) (Figure 1, lower left). There were no statistically significant correlations between the T-score of the ADHD index and the rich-club coefficient. There were no significant correlations between inattentive symptoms, hyperactive/impulsive, DSM-IV total scores, and global network metrics.
Figure 1. Correlations between ADHD symptoms (X: T-score of ADHD index) and global network metrics (Y) in patients with adult ADHD. (A) A significantly negative correlation between the T-score of the ADHD index and the global efficiency was noted (r = −0.481, 95% CI −0.768–−0.0346, P = 0.037). (B) A significantly negative correlation between the T-score of the ADHD index and the clustering coefficient was noted (r = −0.56, P = 0.0127). (C) A significantly negative correlation between the T-score of the ADHD index and the small worldness was noted (r = −0.603, 95% CI −0.83–−0.205, P = 0.0062). (D) A significantly positive correlation between the T-score of the ADHD index and the characteristic path length was noted (r = 0.454, 95% CI 0.000629–0.753, P = 0.0495).
Compared with HCs, the connectometry analysis identified significantly decreased connectivity in patients with adult ADHD in the body of corpus callosum, the cingulum bundle, the forceps minor, the forceps major, the left fornix, the right corticospinal tract, the right superior longitudinal fasciculus III, the right medial lemniscus, and the right corticopontine tract (Figure 2, upper). The connectometry analysis identified no track with increased connectivity in patients with adult ADHD.
Figure 2. Results of local connectometry. Upper: Compared with HCs, the connectometry analysis identified significantly decreased connectivity in patients with adult ADHD in the body of the corpus callosum, the cingulum bundle, the forceps minor, the forceps major, the left fornix, the right corticospinal tract, the right superior longitudinal fasciculus III, the right medial lemniscus, and the right corticopontine tract. Middle: Significantly positive correlations between QA and severity of hyperactive–impulsive symptoms in the right corticostriatal tract, the right corticopontine tract, the right corticospinal tract, the right inferior fronto-occipital fasciculus, and the right extreme capsule (red fibers). The QA in the middle cerebellar peduncle and the left cerebellum was found to be negatively related to the severity of hyperactive–impulsive symptoms (blue fibers). Bottom: The connectometry analysis found negative correlations between QA and severity of inattentive symptoms in the bilateral corticospinal tract, the left inferior longitudinal fasciculus, the right corticopontine tract, the middle cerebellar peduncle, the bilateral cerebellum, the right medial lemniscus, and the right dentatorubrothalamic tract.
In patients with adult ADHD, positive correlations between QA and severity of hyperactive/impulsive symptoms (T-score of DSM-IV hyperactive–impulsive symptoms) were observed in the right corticostriatal tract, the right corticopontine tract, the right corticospinal tract, the right inferior fronto-occipital fasciculus, and the right extreme capsule (Figure 2, middle; red fibers). The QA in the middle cerebellar peduncle and left cerebellum was found to be negatively related to the severity of hyperactive–impulsive symptoms (Figure 2, middle; blue fibers).
The connectometry analysis found negative correlations between QA and severity of inattentive symptoms in the bilateral corticospinal tract, the left inferior longitudinal fasciculus, the right corticopontine tract, the middle cerebellar peduncle, the bilateral cerebellum, the right medial lemniscus, and the right dentatorubrothalamic tract (Figure 2, bottom; blue fibers). There was no significant result in tracks with QA positively correlated with the severity of inattentive symptoms.
On the contrary, there was no significant result in tracks with the QA correlated with the ADHD index.
In this study, we performed comprehensive analyses of DTI data, local connectometry analysis by using QA and graph theoretical analysis, and conducted comparisons of several measurements between patients with HCs and patients with adult ADHD. We first discuss the results of global network metrics estimated by graph theory and then discuss the results of local connectometry.
Patients with adult ADHD had a significantly lower rich-club coefficient than HCs. Other indicators, such as small worldness and global efficiency, which were found to be abnormal in previous studies of children and adolescents with ADHD (21, 22), were not significantly different in the present study. Network hubs that are members of the rich club are connected to each other, establishing a central rich club that serves as a hub for interregional and global neural signaling as well as whole-brain integration and communication (18). The results of the graph theoretical analysis suggest that adults with ADHD have altered functional integration and global information communication in the brain. While children with ADHD have a deteriorated small-world network structure, with lower global efficiency and higher local efficiency (21–23), there were discrepancies between studies in the results of global network measures in patients with adult ADHD (23–27). Sidlauskaite et al. reported no differences between patients with adult ADHD and HCs in terms of global network metrics, such as small worldness, global efficiency, and clustering coefficient (24). Meanwhile, other studies reported lower global efficiency, abnormal rich-club organization and reduced hemispheric asymmetry in adult ADHD (25, 27). However, these three studies of positive results of global network metrics were reported from the same country, and two out of three studies were from the same laboratory. The results of this study, abnormal rich-club organization, and possible lower global communication in patients with adult ADHD, are similar to the results of those studies and may support the positive results of previous studies (25, 27). Correlational analyses revealed correlations between global network metrics and the overall severity of symptoms. The more severe the overall symptoms of ADHD, global efficiency, clustering coefficient, and small worldness were lower, and characteristic path length was longer, suggesting that altered functional integration and global information communication in patients with adult ADHD contribute to the general symptomatic severity of ADHD and its close relationship with the pathophysiology of ADHD. Although patients with adult ADHD showed a lower rich-club coefficient than HCs, the correlational analysis showed no correlation between the rich-club coefficient and symptomatic severity. This appears to be some sort of contradictory result. We cannot provide a clear explanation at this time, but we speculated that the rich-club coefficient abnormality may be a trait marker rather than a state marker of ADHD. Further studies will be needed to clarify such speculation.
The local connectometry revelated decreased connectivity in the corpus callosum (CC) (body) and fibers near the corpus callosum, such as the forceps minor, the forceps major, the cingulum bundle, and the left fornix in patients with adult ADHD. In addition, the right corticospinal tract, the right corticopontine tract, the right medial lemniscus, and the right superior longitudinal fasciculus III (SLF III) also showed decreased connectivity in patients with adult ADHD. We speculated that widely distributed disrupted structural connectivity including the CC revealed by local connectometry could be related to the less efficient information transfer system revealed by graph theory in patients with adult ADHD and related to overall symptoms of ADHD. Except for median structure such as the CC and the cingulum, the white matter fiber abnormalities in adult ADHD in this study were found in the right hemisphere as in previous studies (23), suggesting a contribution to the problem of hemispheric lateralization problems in ADHD (25, 27).
The CC is responsible for hemispheric lateralization and communication between cerebral hemispheres (28). Studies of connectome with agenesis of the corpus callosum demonstrated that patients with agenesis of the corpus callosum showed longer path length and lower global efficiency (29, 30). This suggests that CC abnormalities may contribute to global network metrics that reflect functional integration. The abnormalities of CC in patients with ADHD have been consistently reported by previous studies, including meta-analyses (23). The forceps major connects the bilateral occipital lobes via the splenium of the corpus callosum, and the forceps minor connects the lateral and medial surfaces of the frontal lobes via the genu of the corpus callosum (28). Several studies, including in patients with adult ADHD, showed abnormality in the forceps major and minor (23, 31). A previous study demonstrated the relationship between executive function and FA in the the right forceps minor in bilingual young adults and showed higher FA in the forceps minor predicted the high performance of a task requiring executive function (32). Moreover, a previous study reported that the disintegrity of the white matter in both forceps major and minor contributed to more declined cognitive function in patients with type-2 diabetes (33). These data suggest that impairment in the forceps contributes to cognitive disability, including executive function, which is often observed in patients with ADHD.
The cingulum bundle and fornix are important parts of the limbic system (28, 30). According to DTI studies, the cingulum takes longer to mature even after adolescence and frequently does not take on adult traits until the mid-20s or later (34, 35). Neuroimaging studies have demonstrated that functions of the cingulum bundle are related to executive/attention functions, emotion, and memory, while clinical studies have demonstrated cingulum abnormalities in neuropsychiatric diseases, including ADHD (36). The cingulum bundle and fornix are important components of the Papez circuit, which is believed to be essential for cognition, emotion, and episodic memory (36, 37). The cingulum bundle connects the anterior thalamic nucleus to the cingulate gyrus, and it also connects the cingulate gyrus to the parahippocampal region, while the fornix connects the subiculum to the mammillary bodies (36, 37). Therefore, the Papez circuit may also play a role in the pathophysiology of major depressive disorders (36, 38). Although there were no obvious psychiatric comorbidities in our sample, adult ADHD is often associated with psychiatric comorbidities and a high prevalence of depression has also been reported (39). Common genetic factors for ADHD and depression have been reported as contributing to this phenomenon (40). Therefore, we speculate that abnormalities of these regions seem to be associated with not only cognitive disability but also susceptibility to depressive disorder in adult ADHD. Indeed, in depression, abnormalities in the cingulum bundle and fornix have been observed (36, 38).
The corticopontine tract and the corticospinal tract are associated with the coordination of planned motor functions (23, 28), and abnormalities of these tracts have been considered to be associated with deficits in fine motor control in patients with ADHD (23). However, a recent study found that the corticopontine tract, a component of cortical-ponto-cerebellar pathways, also known as the cerebello-thalamo-cortical or cortico-ponto-cerebellar loop, serves as a medium for cerebro-cerebellar communication during cognitive processing (41). We speculated that abnormality in the corticopontine tract might be associated with not only motor control disorder but also cognitive disability in patients with ADHD. The SLF III had possible connections between the supramarginal gyrus and the pars opercularis and bidirectional connections between the ventral prefrontal cortex and the inferior parietal lobule (28). The SLF III may function to transfer somatosensory information, including language articulation via monitoring orofacial and hand motions (28). The abnormalities in the SLF were also reported in patients with ADHD (23).
This study found disintegrity in the right medial lemniscus in adult ADHD. To the best of our knowledge, there has been no report of abnormality in this region in patients with ADHD; however, a previous study reported decreased FA in this region in patients with autistic spectrum disorder (ASD) (42). The medial lemniscus serves as a major route for ascending sensory fibers to the ventroposterolateral thalamus (28). Since ADHD and ASD are often concurrent and shared sensory symptoms such as hyper- and hyposensitivity to various types of sensory input (43), abnormality in the medial lemniscus might be associated with sensory symptoms in ADHD.
Although global network metrics did not significantly correlate with hyperactive/impulsive or inattentive symptoms in the current study, local connectometry detected abnormal fibers associated with these symptoms. Regarding the hyperactive–impulsive symptoms, severe symptoms are associated with higher QA (suggesting overconnectivity) in the right corticostriatal tract, the corticospinal tract, vextreme capsule, and the right inferior fronto-occipital fasciculus. The corticostriatal projections are critical components of forebrain circuits that are extensively involved in motivated behavior (28). Not only decreased connectivity but also overconnectivity may also disrupt structural connectivity and information transfer, and some studies demonstrated overconnectivity in patients with ASD (40). The corticostriatal circuits have been linked to dopaminergic pathways that connect the striatum to the prefrontal cortex and other areas, and dysfunction of these circuits has been linked to impairments in cognitive functions and the ability to adapt behavior to changing circumstances (44). Considering the function of the corticostriatal tract and its contribution to the pathophysiology of ADHD, the association between abnormality in this region and hyperactive–impulsive symptoms seems to be plausible. The inferior fronto-occipital fascia (IFOF) is a WM tract that originates in the occipital and parietal lobes, ends in the inferior frontal lobe, and is connected to the inferolateral insula via the extreme and external capsules with the uncinate fasciculus. While the IFOF and extreme capsule are primarily associated with semantic language processing and transmission (28), research has shown that the IFOF connects the salience network to the executive control network, a possible serving role in goal-oriented behavior (45). We speculated that, together with the corticostriatal tract abnormalities, IFOF abnormalities may contribute to behavioral abnormalities, such as impulsiveness in ADHD-based disability of goal-oriented behaviors.
Decreased connectivity in the cerebellum was associated with both hyperactive–impulsive and inattentive symptoms. As mentioned previously, cerebro-cerebellar communication should be an important component in cognitive processing (38). Although dopamine receptors are not abundant in the cerebellum, it is involved in the indirect regulation of dopaminergic neurotransmission (44). An animal study demonstrated that the vermis and paravermal areas in the cerebellum modulate the rate of dopamine and noradrenaline turnover in the caudate and nucleus accumbens (44). Considering the intimate relationship between the dopaminergic system and the pathophysiology of ADHD, the association between abnormality in the cerebellar circuit and symptoms of ADHD seems to be plausible. Indeed, many studies have demonstrated abnormalities of the cerebellum in ADHD (23, 46).
The results for corticospinal and corticopontine tracts were ambiguous. Compared with HCs, these tracts showed significantly decreased connectivity in the adult ADHD group, but correlational analysis showed a positive correlation with the severity of hyperactive/impulsive symptoms. Furthermore, the corticospinal and corticopontine tracts showed opposite QA patterns in association with symptoms. As described earlier, higher QA in these areas was related to the severity of hyperactive–impulsive symptoms, whereas lower QA was related to the severity of inattentive symptoms. We have no clear explanation of these phenomena but speculate that different patterns of white matter abnormalities, decreased connectivity, and overconnectivity in the same region might be associated in different ways and might contribute to different symptoms in ADHD and patterns of the combination of overconnectivity/decreased connectivity of white matter fibers in each region, which might be associated with severity of clinical symptoms. However, there are no previous studies to support these speculations at this time, and further research is needed. Regarding other tracts, the inferior longitudinal fasciculus (associated with object recognition, face recognition, lexical and semantic processes, emotion, and visual memory) (47), the medial lemniscus (associated with sensory processing) (28), and the dentatorubrothalamic tract (associated with motor control and also is a known site of deep stimulation for the treatment of tremor) (28, 48), we have no clear explanation for associations between disintegrity in these fibers and severity of inattentive symptoms at present because there has been no study that reported a direct relationship between attention and these regions.
The superior point of this study is the strict selection of treatment-naive patients with adult ADHD. Since WM integrity was affected by the treatment of MPH, it was important to evaluate DTI data in treatment-naive patients with adult ADHD.
Meanwhile, there are some limitations in this study. First, as already mentioned in the Discussion section, the results for the corticospinal and corticopontine tracts were ambiguous, and we have no clear explanation for the phenomenon. Further research is needed to clarify such phenomena. The second limitation is a relatively small sample size of the study. Although Friston's loss-function analysis suggests that the optimal sample size for a neuroimaging study is 16 to 32 participants (49, 50), the small sample size in this study may underestimate the detection of changes in connectivity due to a lack of statistical power and may contribute to seemingly contradictory results, such as the difference between correlation analysis and group comparison results, and due to the fact that graph theory correlation analysis showed a correlation only with the ADHD index but no correlation with core symptoms. However, our findings are similar to previous studies with a larger sample size. Third, this study is a biological study with a small sample, and therefore, we excluded adult ADHD patients with comorbid psychiatric disorders. On the contrary, patients with adult ADHD have been reported to have a high frequency of various comorbid disorders, including affective disorders, anxiety disorders, and substance abuse (39, 51). Therefore, there are limitations in generalizing the results of this study to patients with adult ADHD, and this point should be interpreted with caution. Fourth, the maximum b-value and the number of directions in this study were lower than those recommended by DSI Studio (13). We cannot deny the possibility of reduced sensitivity in detecting WM due to our DTI acquisition parameters. However, previous studies that performed DTI like the DTI acquisition parameters in our study and performed similar analyses using DSI Studio demonstrated pathologically relevant results (52, 53). Finally, healthy control participants in the present study had high IQs. Due to the existence of the relationship between white matter development and connectome and intelligence (54, 55), control participants with high IQ affected the results of this study. However, correlational analyses were conducted only on participants with adult ADHD, and we found relevant results in terms of the pathophysiology of ADHD.
Treatment-naive patients with adult ADHD showed lower rich-club coefficients and decreased connectivity of the corpus callosum and fibers near the corpus callosum and several fibers in the right cerebral hemisphere. The widely distributed abnormal WM would contribute to the abnormal rich club and consequently less efficient information transfer in the ADHD brain. The severity of overall ADHD symptoms was associated with several global network metrics and several fibers associated with the severity of the hyperactive–impulsive symptom and the inattentive symptom, suggesting the contribution of WM abnormalities in the adult ADHD brain to the pathophysiology of adult ADHD.
The datasets presented in this article are not readily available because Due to the nature of this research, participants of this study did not agree for their data to be shared publicly, so supporting data is not available. Requests to access the datasets should be directed to dG9obmlzaDhAaXRzLmpuai5jb20=.
The studies involving human participants were reviewed and approved by Research Ethical Committee of Fukushima Medical University. The patients/participants provided their written informed consent to participate in this study.
TO: conceptualization, methodology, data analyses, writing—original draft preparation, and project administration. HY: writing—review and editing and supervision. HI, SIs, AS, and IM: data collection and writing—review and editing. JM: writing—review and editing. WT and SIt: conceptualization, data collection, and writing—review and editing.
TO has disclosed that they are a full-time employee of Janssen Pharmaceutical K.K. of Johnson and Johnson in Japan.
The remaining authors declare that the research was conducted in the absence of any commercial or financial relationships that could be construed as a potential conflict of interest.
The authors declare that this study received funding from Janssen Pharmaceutical K.K. of Johnson and Johnson in Japan. The funder had the following involvement in the study via TO: study concept, design of study, data analyses, interpretation of data and drafting manuscript.
All claims expressed in this article are solely those of the authors and do not necessarily represent those of their affiliated organizations, or those of the publisher, the editors and the reviewers. Any product that may be evaluated in this article, or claim that may be made by its manufacturer, is not guaranteed or endorsed by the publisher.
1. Fayyad J, Sampson NA, Hwang I, Adamowski T, Aguilar-Gaxiola S, Al-Hamzawi A, et al. (2017). WHO world mental health survey collaborators. The descriptive epidemiology of DSM-IV adult ADHD in the world health organization world mental health surveys. Atten Defic Hyperact Disord. 9, 47–65. doi: 10.1007/s12402-016-0208-3
2. Alexander L, Farrelly N. Attending to adult ADHD: a review of the neurobiology behind adult ADHD. Ir J Psychol Med. (2018) 35:237–44. doi: 10.1017/ipm.2017.78
3. Samea F, Soluki S, Nejati V, Zarei M, Cortese S, Eickhoff SB, et al. Brain alterations in children/adolescents with ADHD revisited: a neuroimaging meta-analysis of 96 structural and functional studies. Neurosci Biobehav Rev. (2019) 100:1–8. doi: 10.1016/j.neubiorev.2019.02.011
4. White T, Nelson M, Lim KO. Diffusion tensor imaging in psychiatric disorders. Top Magn Reson Imaging. (2008) 19:97–109. doi: 10.1097/RMR.0b013e3181809f1e
5. Zhao Y, Yang L, Gong G, Cao Q, Liu J. Identify aberrant white matter microstructure in ASD, ADHD and other neurodevelopmental disorders: A meta-analysis of diffusion tensor imaging studies. Prog Neuropsychopharmacol Biol Psychiatry. (2022) 8:113–110477. doi: 10.1016/j.pnpbp.2021.110477
6. Bouziane C, Caan MWA, Tamminga HGH, Schrantee A, Bottelier MA, de Ruiter MB, et al. ADHD and maturation of brain white matter: a DTI study in medication naive children and adults. Neuroimage Clin. (2017) 29:53–9. doi: 10.1016/j.nicl.2017.09.026
7. Schweren LJ, Hartman CA, Zwiers MP, Heslenfeld DJ, Franke B, Oosterlaan J, et al. Stimulant treatment history predicts frontal-striatal structural connectivity in adolescents with attention-deficit/hyperactivity disorder. Eur Neuropsychopharmacol. (2016) 26:674–83. doi: 10.1016/j.euroneuro.2016.02.007
8. Bouziane C, Filatova OG, Schrantee A, Caan MW, Vos FM, Reneman L. White matter by diffusion mri following methylphenidate treatment: a randomized control trial in males with attention-deficit/hyperactivity disorder. Radiology. (2019) 293:186–192. doi: 10.1148/radiol.2019182528
9. Van Der Marel K, Klomp A, Meerhoff GF, Schipper P, Lucassen PJ, Homberg JR, et al. Long-term oral methylphenidate treatment in adolescent and adult rats: differential effects on brain morphology and function. Neuropsychopharmacol. (2014) 39:263–73. doi: 10.1038/npp.2013.169
10. Adriani W, Leo D, Guarino M, Natoli A, Di Consiglio E, De Angelis G, et al. Short-term effects of adolescent methylphenidate exposure on brain striatal gene expression and sexual/endocrine parameters in male rats. Ann N Y Acad Sci. (2006) 1074:52–73. doi: 10.1196/annals.1369.005
11. Yeh FC, Badre D, Verstynen T. Connectometry: a statistical approach harnessing the analytical potential of the local connectome. Neuroimage. (2016) 125:162–171. doi: 10.1016/j.neuroimage.2015.10.053
12. Yeh FC, Zaydan IM, Suski VR, Lacomis D, Richardson RM, Maroon JC, et al. Differential tractography as a track-based biomarker for neuronal injury. Neuroimage. (2019) 202:116131. doi: 10.1016/j.neuroimage.2019.116131
13. Yeh FC, Tseng WY. NTU-90: a high angular resolution brain atlas constructed by q-space diffeomorphic reconstruction. Neuroimage. (2011) 58:91–9. doi: 10.1016/j.neuroimage.2011.06.021
14. Yeh FC, Wedeen VJ, Tseng WY. Generalized q-sampling imaging. IEEE Trans Med Imaging. (2010) 29:1626–35. doi: 10.1109/TMI.2010.2045126
15. Yeh FC, Liu L, Hitchens TK, Wu YL. Mapping immune cell infiltration using restricted diffusion MRI. Magn Reson Med. (2017) 77:603–12. doi: 10.1002/mrm.26143
16. Yeh FC, Vettel JM, Singh A, Poczos B, Grafton ST, Erickson KI, et al. Quantifying differences and similarities in whole-brain white matter architecture using local connectome fingerprints. PLoS Comput Biol 15;12. (2016) e1005203. doi: 10.1371/journal.pcbi.1005203
17. Rubinov M, Sporns O. Complex network measures of brain connectivity uses and interpretations. Neuroimage. (2010) 52:1059–69. doi: 10.1016/j.neuroimage.2009.10.003
18. Dennis EL, Jahanshad N, Toga AW, McMahon KL, de Zubicaray GI, et al. Development of the “rich club” in brain connectivity networks from 438 adolescents and adults aged 12 to 30. Proceedings of IEEE International Symposium Biomed Imaging. (2013). p. 624–7. doi: 10.1109/ISBI.2013.6556552
19. Yeh FC, Verstynen TD, Wang Y, Fernández-Miranda JC, Tseng WY. Deterministic diffusion fiber tracking improved by quantitative anisotropy. PLoS One 15;8. (2013) e80713. doi: 10.1371/journal.pone.0080713
20. Yeh FC, Panesar S, Barrios J, Fernandes D, Abhinav K, Meola A, et al. Automatic removal of false connections in diffusion MRI tractography using topology-informed pruning (TIP). Neurotherapeutics. (2019) 16:52–8. doi: 10.1007/s13311-018-0663-y
21. Cao Q, Shu N, An L, Wang P, Sun L, Xia MR, et al. Probabilistic diffusion tractography and graph theory analysis reveal abnormal white matter structural connectivity networks in drug-naive boys with attention deficit/hyperactivity disorder. J Neurosci 26;33. (2013) 10676–87. doi: 10.1523/JNEUROSCI.4793-12.2013
22. Beare R, Adamson C, Bellgrove MA, Vilgis V, Vance A, Seal ML, et al. Altered structural connectivity in ADHD: a network based analysis. Brain Imaging Behav. (2017) 11:846–58. doi: 10.1007/s11682-016-9559-9
23. Bu X, Cao M, Huang X, He Y. The structural connectome in ADHD. Psychoradiology. (2021) 1:257–271. doi: 10.1093/psyrad/kkab021
24. Sidlauskaite J, Caeyenberghs K, Sonuga-Barke E, Roeyers H, Wiersema JR. Whole-brain structural topology in adult attention-deficit/hyperactivity disorder: preserved global - disturbed local network organization. Neuroimage Clin. (2015) 13:506–12. doi: 10.1016/j.nicl.2015.10.001
25. Li D, Li T, Niu Y, Xiang J, Cao R, Liu B, et al. Reduced hemispheric asymmetry of brain anatomical networks in attention deficit hyperactivity disorder. Brain Imaging Behav. (2019) 13:669–84. doi: 10.1007/s11682-018-9881-5
26. Li D, Cui X, Yan T, Liu B, Zhang H, Xiang J, et al. Abnormal rich club organization in hemispheric white matter networks of ADHD. J Atten Disord. (2021) 25:1215–29. doi: 10.1177/1087054719892887
27. Wang B, Wang G, Wang X, Cao R, Xiang J, Yan T, et al. Rich-Club analysis in adults with ADHD connectomes reveals an abnormal structural core network. J Atten Disord. (2021) 25:1068–79. doi: 10.1177/1087054719883031
28. Wycoco V, Shroff M, Sudhakar S, Lee W. White matter anatomy: what the radiologist needs to know. Neuroimaging Clin N Am. (2013) 23:197–216. doi: 10.1016/j.nic.2012.12.002
29. Owen JP, Li YO, Ziv E, Strominger Z, Gold J, Bukhpun P, et al. The structural connectome of the human brain in agenesis of the corpus callosum. Neuroimage. (2013) 70:340–55. doi: 10.1016/j.neuroimage.2012.12.031
30. Shi M, Freitas L, Spencer-Smith MM, Kebets V, Anderson V, McIlroy A, et al. Intra- and inter-hemispheric structural connectome in agenesis of the corpus callosum. NeuroImage Clinical. (2021) 31:102709. doi: 10.1016/j.nicl.2021.102709
31. Bode MK, Lindholm P, Kiviniemi V, Moilanen I, Ebeling H, Veijola J, et al. DTI abnormalities in adults with past history of attention deficit hyperactivity disorder: a tract-based spatial statistics study. Acta Radiol. (2015) 56:990–6. doi: 10.1177/0284185114545147
32. Mamiya PC, Richards TL, Kuhl PK. Right forceps minor and anterior thalamic radiation predict executive function skills in young bilingual adults. Front Psychol. (2018) 9:118. doi: 10.3389/fpsyg.2018.00118
33. Gao S, Chen Y, Sang F, Yang Y, Xia J, Li X, et al. White matter microstructural change contributes to worse cognitive function in patients with type 2 diabetes. Diabetes. (2019) 68:2085–94. doi: 10.2337/db19-0233
34. Lebel C, Beaulieu C. Longitudinal development of human brain wiring continues from childhood into adulthood. J Neurosci. (2011) 31:10937–47. doi: 10.1523/JNEUROSCI.5302-10.2011
35. Lebel C, Gee M, Camicioli R, Wieler M, Martin W, Beaulieu C, et al. Diffusion tensor imaging of white matter tract evolution over the lifespan. Neuroimage. (2012) 60:340–52. doi: 10.1016/j.neuroimage.2011.11.094
36. Bubb EJ, Metzler-Baddeley C, Aggleton JP. The cingulum bundle: anatomy, function, and dysfunction. Neurosci Biobehav Rev. (2018) 92:104–27. doi: 10.1016/j.neubiorev.2018.05.008
37. Senova S, Fomenko A, Gondard E, Lozano AM. Anatomy and function of the fornix in the context of its potential as a therapeutic target. J Neurol Neurosurg Psychiatry. (2020) 91:547–59. doi: 10.1136/jnnp-2019-322375
38. van Velzen LS, Kelly S, Isaev D, Aleman A, Aftanas LI, Bauer J, et al. White matter disturbances in major depressive disorder: a coordinated analysis across 20 international cohorts in the ENIGMA MDD working group. Mol Psychiatry. (2020) 25:1511–25. doi: 10.1038/s41380-019-0477-2
39. Ohnishi T, Kobayashi H, Yajima T, Koyama T, Noguchi K. Psychiatric comorbidities in adult attention-deficit/hyperactivity disorder: prevalence and patterns in the routine clinical setting. Innov Clin Neurosci. (2019) 16:11–6.
40. Balogh L, Pulay AJ, Réthelyi JM. Genetics in the ADHD clinic: how can genetic testing support the current clinical practice? Front Psychol. (2022) 8:751041. doi: 10.3389/fpsyg.2022.751041
41. Palesi F, De Rinaldis A, Castellazzi G, Calamante F, Muhlert N, Chard D, et al. (2017). Contralateral cortico-ponto-cerebellar pathways reconstruction in humans in vivo: implications for reciprocal cerebro-cerebellar structural connectivity in motor and non-motor areas. Sci Rep. 7, 12841. doi: 10.1038/s41598-017-13079-8
42. Kleinhans NM, Pauley G, Richards T, Neuhaus E, Martin N, Corrigan NM, et al. (2012). Age-related abnormalities in white matter microstructure in autism spectrum disorders. Brain Res. 15, 1479.1–16. doi: 10.1016/j.brainres.2012.07.056
43. Ohta H, Aoki YY, Itahashi T, Kanai C, Fujino J, Nakamura M, et al. (2020). White matter alterations in autism spectrum disorder and attention-deficit/hyperactivity disorder in relation to sensory profile. Mol Autism. 19, 11.77. doi: 10.1186/s13229-020-00379-6
44. Solso S, Xu R, Proudfoot J, Hagler DJ, Campbell K, Venkatraman V, et al. Diffusion tensor imaging provides evidence of possible axonal overconnectivity in frontal lobes in autism spectrum disorder toddlers. Biol Psychiatry. (2016) 79:676–84. doi: 10.1016/j.biopsych.2015.06.029
45. Conner AK, Briggs RG, Sali G, Rahimi M, Baker CM, Burks JD, et al. A Connectomic atlas of the human cerebrum-chapter 13: tractographic description of the inferior fronto-occipital fasciculus. Operative Neurosurgery. (2018) 15:S436–43. doi: 10.1093/ons/opy267
46. Ding L, Pang G. Identification of brain regions with enhanced functional connectivity with the cerebellum region in children with attention deficit hyperactivity disorder: a resting-state fMRI study. Int J Gen Med. (2021) 27:14. doi: 10.2147/IJGM.S303339
47. Herbet G, Zemmoura I, Duffau H. Functional anatomy of the inferior longitudinal fasciculus: from historical reports to current hypotheses. Front Neuroanat. (2018) 12:77. doi: 10.3389/fnana.2018.00077
48. Fenoy AJ, Schiess MC. Deep brain stimulation of the dentato-rubro-thalamic tract: outcomes of direct targeting for tremor. Neuromodulation. (2017) 20:429–36. doi: 10.1111/ner.12585
49. Friston KJ, Holmes AP, Worsley KJ. How many subjects constitute a study? Neuroimage10. (1999) 1–5. doi: 10.1006/nimg.1999.0439
50. Friston K. Ten ironic rules for non-statistical reviewers. Neuroimage 16;61. (2012) 1300–10. doi: 10.1016/j.neuroimage.2012.04.018
51. Kooij JJS, Bijlenga D, Salerno L, Jaeschke R, Bitter I, Balázs J, et al. Updated European consensus statement on diagnosis and treatment of adult ADHD. Eur Psychiatry. (2019) 56:14–34. doi: 10.1016/j.eurpsy.2018.11.001
52. Bigham B, Zamanpour SA, Zare H. Alzheimer's disease neuroimaging initiative. Features of the superficial white matter as biomarkers for the detection of Alzheimer's disease and mild cognitive impairment: A diffusion tensor imaging study. Heliyon. (2022) 8:8.e08725. doi: 10.1016/j.heliyon.2022.e08725
53. Zdanovskis N, Platkājis A, Kostiks A, Karelis G, Grigorjeva O. Brain structural connectivity differences in patients with normal cognition and cognitive impairment. Brain Sci. (2021) 11:943. doi: 10.3390/brainsci11070943
54. Northam GB, Liégeois F, Chong WK, Wyatt JS, Baldeweg T. Total brain white matter is a major determinant of IQ in adolescents born preterm. Ann Neurol. (2011) 69:702–11. doi: 10.1002/ana.22263
Keywords: adult ADHD, diffusion tensor imaging, connectome, connectivity, graph theory
Citation: Ohnishi T, Toda W, Itagaki S, Sato A, Matsumoto J, Ito H, Ishii S, Miura I and Yabe H (2023) Disrupted structural connectivity and less efficient network system in patients with the treatment-naive adult attention-deficit/hyperactivity disorder. Front. Psychiatry 14:1093522. doi: 10.3389/fpsyt.2023.1093522
Received: 09 November 2022; Accepted: 17 February 2023;
Published: 16 March 2023.
Edited by:
Yulia Solovieva, Meritorious Autonomous University of Puebla, MexicoReviewed by:
Weizhao Lu, Shandong First Medical University, ChinaCopyright © 2023 Ohnishi, Toda, Itagaki, Sato, Matsumoto, Ito, Ishii, Miura and Yabe. This is an open-access article distributed under the terms of the Creative Commons Attribution License (CC BY). The use, distribution or reproduction in other forums is permitted, provided the original author(s) and the copyright owner(s) are credited and that the original publication in this journal is cited, in accordance with accepted academic practice. No use, distribution or reproduction is permitted which does not comply with these terms.
*Correspondence: Takashi Ohnishi, dG9obmlzaDhAaXRzLmpuai5jb20=
Disclaimer: All claims expressed in this article are solely those of the authors and do not necessarily represent those of their affiliated organizations, or those of the publisher, the editors and the reviewers. Any product that may be evaluated in this article or claim that may be made by its manufacturer is not guaranteed or endorsed by the publisher.
Research integrity at Frontiers
Learn more about the work of our research integrity team to safeguard the quality of each article we publish.