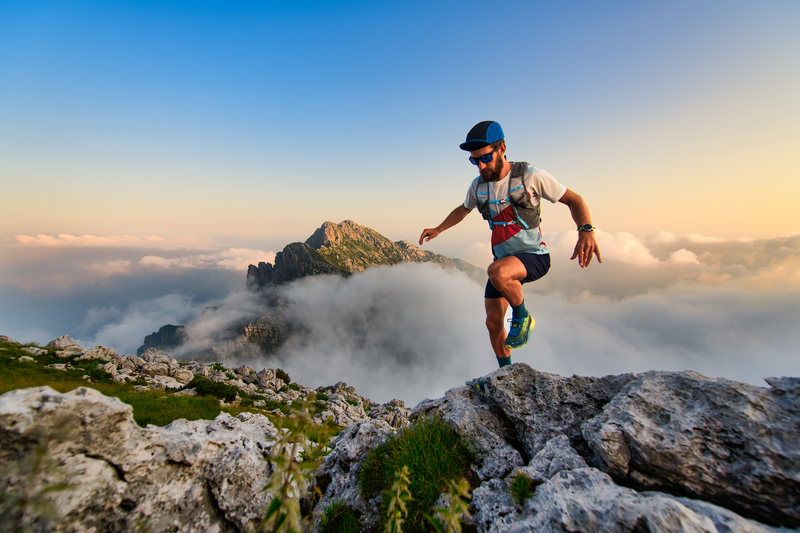
95% of researchers rate our articles as excellent or good
Learn more about the work of our research integrity team to safeguard the quality of each article we publish.
Find out more
ORIGINAL RESEARCH article
Front. Psychiatry , 15 May 2023
Sec. Psychopathology
Volume 14 - 2023 | https://doi.org/10.3389/fpsyt.2023.1048791
This article is part of the Research Topic Developmental Trajectories in Mental Health between Adolescence and Adulthood View all 5 articles
Introduction: Policies to legalize possession and use of marijuana have been increasingly supported across the United States. Although there are restrictions on use in minors, many substance abuse scientists anticipate that these policy changes may alter use patterns among adolescents due to its wider availability and a softening of beliefs about its potentially harmful consequences. Despite the possibility that these policies may increase the prevalence of use among adolescents, the effects of marijuana on neurodevelopment remain unclear, clouding arguments in favor of or opposition to these policies.
Methods: The present prospective, longitudinal study was designed to isolate the neurodevelopmental consequences of marijuana use from its precursors during adolescence—a period of heightened vulnerability for both substance use and disrupted development due to environmental insults. Early adolescents who were substance-naïve at baseline (N = 529, aged 10–12) were recruited and tracked into adolescence when a subgroup initiated marijuana use during one of three subsequent waves of data collection, approximately 18 months apart.
Results: Results suggest that marijuana use may be specifically related to a decline in verbal learning ability in the short term and in emotion recognition, attention, and inhibition in the longer-term.
Discussion: These preliminary findings suggest that marijuana use has potential to adversely impact vulnerable neurodevelopmental processes during adolescence. Intensive additional investigation is recommended given that state-level policies regulating marijuana use and possession are rapidly shifting in the absence of good scientific information.
Adolescence is considered a transitional developmental period between childhood and adulthood during which significant changes in biological, social, and psychological functioning occur in the context of greater social demands and increasing autonomy (1). Due to adolescents’ heightened susceptibility to environmental influences, these changes pose challenges for developing teens who are attempting to navigate a rapidly evolving landscape of newfound independence, drives, and abilities. To do so successfully, ongoing maturation of higher order cognitive skills and emotion regulatory functions are relied upon. However, there is a disconnect between the phasic development of cognitive and emotional systems that would otherwise enable adaptive decision-making, impulse control, and self-regulation of behavior. The heuristic “dual systems model” suggests that the neural substrates of executive cognition (e.g., prefrontal cortex) are more easily overridden by a relatively more mature limbic system that undergirds emotional responses (2). Especially pronounced in the presence of peers, cognitive controls are not as effective in modulating emotions during adolescence as in adulthood (3), leading to somewhat higher rates of risky behaviors; a phenomenon characterized over the centuries as normative (4). However, youth with nonnormative maturational delays in prefrontal cognitive systems and their circuitry with lower regions are at heightened risk for a range of more serious risky behaviors, such as substance misuse (3).
In addition to increased risk for using abusable substances during this period, there is some evidence that these emergent systems may be more vulnerable to damage potentially caused by substance use (5). The effects of substance use may, thus, be observed in a maturational delay in those cognitive functions that have not yet reached their peak of maturity. Furthermore, in adolescents exhibiting a nonnormative lag in developing prefrontal regions, those “weaker” systems may be most adversely impacted by subsequent use. Studies have yet to fully establish whether and to what extent substance use during adolescence impedes development of various dimensions of executive cognitive functioning, with potential for enduring effects into adulthood. Also not well defined is the drug-specific nature of these impacts.
These questions are particularly salient when considering substances that are widely used in adolescence. Several national surveys consistently document that marijuana use substantially rises when children reach their adolescent years; adolescence is the stage when initiation most commonly occurs and marijuana is the most prevalent drug used, other than alcohol (6). Data from the Monitoring The Future (MTF) study concluding in 2019 affirms that rates of marijuana use rise sharply in adolescence, with 11.8% of the 8th graders, 28.8% of the 10th graders, and 35.7% of the 12th graders surveyed nationally reporting marijuana use in the past year (7). And more recently, both adolescents and young adults are reporting a historically even higher level of marijuana use (8, 9), perhaps due to decreased beliefs in the negative consequences of marijuana use due to legalization trends (10, 11).
Although these prevalence rates are alarming given the heightened vulnerability of the adolescent brain, there is a paucity of longitudinal efforts to isolate effects of marijuana on development, particularly across different levels of use and individual characteristics. The Adolescent Brain Cognitive Development (ABCD) study will eventually reveal both precursors and consequences of marijuana and other substance use in adolescents (12). Until then, there is an urgency to elucidate the impacts of marijuana use on neurodevelopment during this critical period of time in light of policy changes that are already directly influencing prevalence rates. Accurate and timely information is needed to ensure policies are grounded in science, and to educate parents, teachers, and adolescents about scientific findings and implications of policy change for their own decision-making.
The adolescent brain undergoes dramatic neural reorganization, including synaptic pruning and myelination (13), thereby facilitating greater differentiation across brain regions and increasing the speed of processing. This progression of brain remodeling occurs throughout adolescence into young adulthood, resulting in enhanced structural neural integration (14), which is integrally related to the fine-tuning of executive functioning, such as decision-making and emotion regulation (14–16). During remodeling, sensitivity to environmental stimuli is also heightened, as seen in augmented neural and physiological responses to negative peer interaction, mass media, and exposure to neighborhood influences (17, 18).
In similar fashion, the adolescent brain is more sensitive to the rewarding properties of abusable substances than adults (5, 19, 20), with potential for use to alter the course of structural and functional maturation (21). Repeated activation of the “reward” dopaminergic neural circuity occurs in response to substance use, sensitizing those neural systems and, in turn, reinforcing drug taking and seeking behaviors (22). The effects of marijuana on neural maturation are a focus of this research because it is most often initiated in adolescence and stimulates the dopaminergic reward system to achieve its desirable effects. Thus, a determination of whether and how tetrahydrocannabinol (THC) exposure, the primary active component of marijuana (23–25), impacts the developing brain is crucial. Studies suggest that THC alters the structure and function of the hippocampus and orbitofrontal cortex, potentially impairing memory, attention, and thinking and learning ability (26, 27). Additionally, similar to other abusable substances, adolescent exposure to THC sensitizes the brain to stimulation from various other drugs—known as cross-sensitization—heightening reward system responsivity and reinforcing further drug-taking (18).
The neural networks affected by THC subserve neurocognitive development; thus, marijuana-induced alterations in the functioning of those circuits are likely to be expressed in altered cognitive functioning, acutely and possibly sustainably. Chronic marijuana use has been linked later in life to aberrations in neural architecture that undergird aspects of neurocognition, including decreased white matter integrity and cortical thickness, associated with inattention and cognitive instability. This relationship is stronger with early initiation during adolescence and greater severity of use (14, 28). Heavy marijuana users also exhibit attenuated emotional responses to negative affective stimuli compared to healthy controls (29, 30) as well as impairments in memory and attention (18, 31, 32). And early initiators exhibit poorer executive cognitive functioning (33, 34). For example, early onset before age 16 is associated with a decline in verbal learning and verbal working memory compared to non-users (35, 36). There is some evidence that these adverse effects may be irreversible (18).
The evidence is not yet clear about whether these deficits are due to residual effects of active marijuana ingredients remaining in the system or potentially longstanding, direct neurotoxic effects of marijuana (37). Scott and his colleagues conducted a meta-analysis of studies that focused on heavy marijuana use in adolescents and young adults (38). Overall, results showed a small significant effect on cognitive functioning; however, that effect becomes insignificant when marijuana users were abstinent for longer than 72 h. A finer grained analysis by Hanson and her colleagues found an interesting pattern that suggests, after marijuana use is discontinued, some cognitive functions recover with time, while others appear to be more sustainably in deficit (35). Crean, Crane, and Mason reported a similar pattern where impaired executive functioning was found immediately after use, whereas in the long term, many of the residual effects diminished and functioning returned to baseline level (39). Very few of these studies included a substance-naïve baseline and many examined cognitive functioning only during acute administration conditions, negating the ability to ascertain whether deficits existed prior to use or were exacerbated by or entirely a consequence of use.
Whether adolescent marijuana use contributes to executive cognitive function and emotion recognition deficits requires prospective, longitudinal investigations, uniquely capable of delineating different dimensions of these processes and their developmental trends potentially impacted by marijuana use during adolescence. Also needed is research that accounts for the comorbid use of marijuana and other substances; few previous studies include other substance use as a covariate or an interaction term is used. Isolating the impact of marijuana from other substances is necessary to determine how it may alter the process of neurocognitive development. Policies can then be more appropriately and safely formulated based on the evidence and the public can be informed of any potential risks prior to the enactment of policy reforms.
This preliminary investigation analyzed data from the Longitudinal Study of Adolescent Marijuana Use and Neurodevelopment, funded by the National Institute on Drug Abuse (1R01DA022321-01A1; MPIs Drs. Diana Fishbein and Christopher Krebs), a prospective, longitudinal study designed to elucidate neurodevelopmental and psychosocial risk factors that predict marijuana initiation and escalation, and evaluate the impact of subsequent marijuana and other substance use on adolescent neurocognitive development. Benefitting from a substance-naïve baseline, the study was able to partial out the consequences of marijuana use on neurodevelopment from the precursors in early adolescence. Several tasks measuring executive cognitive function and emotion recognition were administered for a comprehensive examination of the specific dimensions predictive of and impacted by marijuana initiation and continuous use. Based on previous research, we anticipated that marijuana initiation and repeated use have different etiologies and may bi-directionally influence the development of executive function and emotional recognition (40–42). Therefore, we first hypothesized that youth who reported marijuana use in subsequent data collection waves would exhibit lower levels of functioning at baseline relative to non-users. Second, we expected marijuana use to exert negative impacts on neurodevelopment in both executive cognitive functioning and emotion recognition domains, controlling for baseline levels of functioning. As THC appears to exert its effects largely in the hippocampus and dorsolateral prefrontal cortex (43), we expected to find impairments in attention and working memory ability, leading to deficits in the ability to learn and perform complicated tasks.
In 2018, adolescents (N = 529) were recruited from a working class, medium-sized city in northern Kentucky characterized by a high rate of early marijuana initiation compared to state and national rates (44). Administrators of local schools were contacted for access to information on enrolled students who were 10–12 years old (public domain information). Eligibility criteria included (a) willingness and ability to provide parental consent and youth assent; (b) English speaking; (c) not emotionally disturbed or severely learning disabled as reported by teachers or parents; and (d) have never consumed more than small amounts of alcohol (e.g., one standard drink) and no use of illicit substances by the time of the baseline interview. Study staff used a variety of strategies to recruit students, including: (i) posters and flyers distributed in schools; (ii) mailings to households with a study package that included a Superintendent’s endorsement letter and study brochure; and (iii) direct contact with custodial caregivers (44). Parents were compensated with cash and youth were given gift cards. This study was granted approval from the Institutional Review Boards (IRBs) of RTI International, University of Maryland School of Medicine, and The Pennsylvania State University. Data collection concluded at the end of 2013.
Well-trained Master’s level research associates conducted separate sessions with caregiver and child within the household in a private location. Interviews were computer-assisted and sensitive content questions were asked using Audio Computer-Assisted Self-Interview (ACASI) technology. Survey measures were administered to both the parent or child and a battery of psychiatric and neurocognitive measures was administered to the child.
A detailed survey of substance use was adapted from three large national surveys: the National Survey on Drug Use and Health (formerly known as the National Household Survey on Drug Abuse), the Monitoring The Future (MTF) Survey, and the Youth Risk Behavior Survey (YRBS) (44). Participants completed the survey in a private location in their homes and were repeatedly reminded that their responses were confidential and anonymous. Audio Computer-Assisted technology (ACASI) was used to increase accuracy of their responses (45). The range of substances gaged included marijuana, alcohol, tobacco, powder cocaine, crack, hallucinogens, heroin, inhalants, prescription pain relievers, Salvia, and stimulants (i.e., a range commonly abused amphetamines), with open-ended questions querying about any other substance use not listed. For each of them, ever use, past 30 days and cumulative days of use were collected to discern patterns of substance use overtime. A dummy variable representing “ever use” of all measured substances other than marijuana was created.
All tasks are developmentally appropriate and have been validated in this age group and have shown minimal repeated measures effects (see references cited in each subsection).
The Vigilance Continuous Performance Test (CPT) is a measure of sustained attention and the ability to inhibit a prepotent impulse (46, 47). The screen presents a 2 × 2 matrix comprising two letters and two solid blocks. Participants are shown a sequence of letters on different positions in the matrix at a rate of 900 ms. Primary outcomes are the number of times the participant correctly responds to a nontarget letter, the number of misses and the number of incorrect responding.
The Rey Auditory-Verbal Learning Test (RAVLT) is a straightforward paper-and-pencil test which aims to measure the capacity of short-term memory and learning ability under proactive interference (48). The task starts with a list of 15 unrelated words at the rate of one word per second, followed by four additional trials. Participants are asked to remember what they see on the first trial and the fifth trail. Immediately and 30 min later (delayed recall), participants are given a story and asked to circle the words which appeared in each of the two trials. Number of correct recall and errors were used.
The Motor Restraint Task (MRT) measures inhibition of impulsive motor reactivity while executing a controlled slow motoric response (49). During this task, a narrow 108-degree circular arc is displayed across the screen. Participants are asked to trace the arc with stylus, and continuously move forward without going outside the lines, stopping or moving backward. The primary outcomes of the task are the time to traverse the arc and the time spent in the arc (number of stoppages).
The Iowa Gambling Task (50–52) is a computerized measure of ability to develop a decision-making strategy based on previously learned information and sensitivity to consequences. The “Wheel of Fortune” (WoF) version (53, 54) was adapted to be developmentally appropriate for young adolescents. Players must develop a strategy to maximize gain, balancing between rewards and penalties; the disadvantageous strategy is the selection of large rewards with greater odds of losing those rewards. Outcome measures included percentage of risky selections, percentage of safe selections, and the risky/safe ratio.
An Emotional Stroop Task assesses cognitive performance in the context of emotional stimuli (55). Respondents are presented with 45 words, 15 in each of positive, negative and control categories. The words are carefully chosen to represent positive and negative emotional states. Respondents are asked to state the color of the word while disregarding its content. Mean reaction times are calculated for each of the three categories. The differences in reaction times for positive and negative categories compared to the control category are primary outcome measures.
The Facial Recognition Task was developed at NIMH and uses facial pictures to measure the ability of emotion recognition (56, 57). Pictures of six emotional expressions are presented for participants to identify: happiness, sadness, anger, surprise, disgust, and fear. An additional category of neutral faces is also presented, which shows plain and alert faces. Participants are asked to recognize and label the emotion expressions in each of the seven categories. Number of correct identifications for each category and a total score were generated.
The Trail Making Test (TMT) provides information about speed for attention, mental flexibility, and executive functioning (58, 59). Fifteen circles containing numbers are randomly arranged on the screen. In trial A, respondents are asked to connect dots in an ascending pattern, and in trail B, they are asked to connect in the reversing order. The time spent in both trials was recorded.
All analyses were performed in R (version 3.6.1, 2019).
An exploratory factor analysis (EFA) was conducted to assess the relationship and structure of the large set of measures included in this study. Using principal axis factoring with oblique rotation, EFA identified latent constructs underlying the measures and indicated dimensions of executive functioning and emotion recognition that clustered together. Eigenvalue and scree plot were used to determine the number of factors, and the “constructs” were interpreted based on the measures within each factor. A threshold of 0.4 was set for the minimum loading coefficient for items under each factor loading (60).
The EFA generated a seven-factor model solution with eigenvalues higher than 1. However, the scree plot suggested that a five-factor model was a better fit (see details in Figure 1). These five factors were labeled accordingly as: (1) Emotion Recognition (Facial Recognition Task); (2) Verbal Learning (RAVLT); (3) Mental Flexibility (MRT); (4) Emotion Repression (Emotional Stroop Task); and (5) Attention and Impulsivity (CPT).
Figure 1. Scree plot from exploratory factor analysis on executive functioning and emotion recognition measures.
Exploratory regression analyses were performed to assess the relationship between the dichotomous measure of marijuana use at baseline and five factors from the EFA at baseline. Adolescents who later reported marijuana use were better able to accurately attribute emotion at baseline, compared to non-users over the course of this project.
Analysis of covariance (ANCOVA) was used to examine whether cognitive performance reflected by each factor differs between the marijuana user group and non-user group by the end of wave 4 of data collection. Baseline differences between the two groups, with users exhibiting lower cognitive ability, might suggest a preexisting susceptibility to marijuana use in early adolescence (21). An ANCOVA was then conducted, controlling for baseline cognitive ability.
The outcomes of interest included the dimensions of executive function and emotion recognition measured in this study. The primary analysis examined the relationship between marijuana use and level of executive functioning. An interaction term between marijuana and age was added to the model to test our hypothesis that there may be time varying effects on cognitive development for marijuana users. The model is specified as:
Previous research emphasizes the criticality of accurately measuring the propensity and severity of marijuana use (61). Therefore, marijuana use was coded in two ways: “ever use” and severity of use. For the ever use measure, participants who reported using marijuana at any wave were designated as users. For severity of use, the cumulative days of use by the time of assessment were coded into seven categories: 1 day, 2 days, 3–5 days, 6–9 days, 10–19 days, 20–39 days, and 40 or more days.
All models included two covariates: the reported use of substances other than marijuana to isolate the effects of marijuana and household income, measured on an 11-point scale, ranges from less than $5,000 to $100,000–$149,999 per year. Previous studies have reported inconsistencies in the role of income. Some studies have found that marijuana use is negatively correlated with income level (62, 63), while others are contradictory, suggesting that higher household income is associated with higher rates of marijuana use (64, 65). Although comparisons between groups on household income at baseline were not significantly different, we included it in the model to avoid a possible confounding effect.
Initial analyses included all participants with complete data for waves 1 and 2 (see below); full data for the cognitive tasks were required to render an accurate assessment. Fewer participants were included in the study beginning in wave 3 due to locating difficulties and budget constraints: 57.2% for wave 3 and 53.1% for wave 4. Chi-square tests showed no differences for sex or ethnicity between participants retained and participants lost to follow up for both waves 3 and 4. Thus, we used Multivariate Imputation by Chained Equation (MICE) which replaces missing values based on patterns in the overall dataset and their responses in the first two waves to compensate for their “loss.” MICE considers uncertainty in predicting missing values, reducing possible attrition bias, to provide a better prediction of developmental trajectories (66, 67).
Of the 529 participants recruited, 64 participants were excluded due to incomplete data in waves 1 and 2; therefore, 465 participants were included in the final analysis. Demographic characteristics of the full sample are presented in Table 1, including age, sex, ethnicity, and income. User status was determined based on whether participants reported using marijuana during wave 2, 3, or 4. The number of marijuana users was 148, while 317 were categorized as non-users. At baseline, marijuana users and non-users were similar in background characteristics, except for age; the user group was, on average, older at baseline (W = 20,600, p = 0.024) compared to the non-user group. Expectedly, as the prevalence rates of marijuana increases with age, an older age is associated with higher probability of having used marijuana by the end of the study; thus, no adjustment was needed. Group differences were examined using nonparametric statistics, given that they differed in size. Additionally, 137 of the 148 marijuana users indicated the number of days of “ever used” at wave 4: 1 day = 27; 2 days = 12; 3–5 days = 12; 6–9 days = 8; 10–19 = 10; 20–39 days = 10; and 40 or more days = 58. No significant differences in sex or ethnicity were found for any number of days of marijuana use.
Table 2 presents results from the exploratory regression analyses. Emotion recognition was significantly different at baseline between the two groups (t = 0.23, p < 0.05), with adjustments for an age effect (68).
One-way ANCOVAs were conducted to identify statistical differences between marijuana users and non-users in the level of executive cognitive function, controlling for other substance use, household income, and the function itself at baseline. Results are presented in Table 3. A significant effect of any marijuana use on verbal learning emerged [F (1,460) = 4.474, p < 0.05].
Additional one-way ANCOVAs were conducted to further explore whether days of use have differential effects on adolescent cognitive functioning (Table 4). We found a significant effect of days of marijuana use on emotion recognition [F(7,234) = 2.52, p < 0.05].
Table 5 presents results of growth models predicting cognitive and emotion functioning by adolescent marijuana use. The age variable was centered such that the zero-point represents the age of 10 which is the youngest age of participants when entering the study. Our five factors were each tested for both main effect of cumulative days of marijuana use and the interaction between age and cumulative days of marijuana use. Both the main effects of level of marijuana use and interaction effects of marijuana use and age were significantly predictive of Emotion Recognition (tmain = 0.64, p < 0.05; tint = −0.05, p < 0.05) and Attention & Impulsivity (tmain = −0.45, p < 0.05; tint = 0.03, p < 0.05).
The present study examined the relationship between level of neurocognitive functioning and marijuana use from a substance naïve baseline at age 10–12 until age 15–17. Researchers interested in a variety of problems extending from the use of marijuana have focused extensively on this period of development when adolescents are susceptible to use and may eventually misuse substances due to both environmental influences and neurodevelopmental vulnerability (2). Although most previous studies have not been able to isolate the neurocognitive consequences of marijuana due to the lack of a substance-naïve baseline, there is a growing number of investigations that will shed light on this outstanding question (69). At present, however, there are no longitudinal studies in the extant literature that employ growth models to ascertain both short-term and long-term effects of marijuana. In contrast with traditional analyses, growth modeling provides smooth trajectory estimation of developmental changes and is capable of incorporating time-varying predictors (70, 71). Further, the high concurrence between marijuana and other substance use demands adjustments for possible additive or synergistic effects of the use of more than one substance on developmental trajectories. Greater understanding of the relationship between marijuana use and neurocognitive development through adolescence can be achieved with the appropriate design features.
In the present investigation, EFA generated five latent constructs representing executive and emotion recognition dimensions—emotion recognition, verbal learning, mental flexibility, emotion repression, and attention and impulsivity—that were expected to show significant relative differences in their developmental progression between marijuana users and nonusers. Our purpose was to identify individual level functional indicators that are predictive of both marijuana initiation and consequences of use as an essential step in determining interventions and policies best suited to promote healthy neurodevelopmental trajectories.
To begin to address these outstanding scientific questions, our first hypothesis was that marijuana initiators will perform less well prior to onset on neurocognitive tasks compared to non-users. Instead, rather than weaker cognitive functioning prior to substance use initiation, we found only that adolescents with early onset of marijuana use were better able to accurately attribute emotion. Previous studies have reported that emotion recognition, particularly in response to negative emotional stimuli, is associated with marijuana initiation (44, 72). A parallel body of literature further suggests that history of child maltreatment, often accompanied by a higher sensitivity to negative emotions (73), has been associated with initiation of marijuana (74). Also potentially relevant is that adolescents who misuse marijuana have been found to report a higher level of aggression than non-initiators (75), which is further associated with over-attributing anger (76). In the present study, however, participants who began to use marijuana exhibited slightly higher scores for all emotions, with the total score reaching statistical significance. Further scrutiny is needed to evaluate the predictive value, significance, and mechanistic explanations for the relationship between emotion recognition and marijuana use.
Additional analyses tested the hypothesis that adolescents who initiate marijuana use will subsequently exhibit neurocognitive delays relative to adolescents who do not report use of marijuana. Because irreversible negative impacts on the developing brain and neurocognitive functioning may be attributable to early substance use (77), we anticipated that lower levels of neurocognitive functioning would be related to marijuana use. Partially consistent with expectations, the adolescent marijuana users in our sample exhibited slight deficits primarily in verbal learning ability. Compared to non-marijuana substance users and non-users, adolescent users recalled fewer words both immediately after learning and after a delay. This finding is supported by previous studies which suggest that the residual effect of marijuana is associated with reduced ability to memorize a word list (37).
In a third set of analyses to examine impacts of extent of marijuana use on development, two factors appeared to be affected. The growth model prediction suggested that repeated marijuana use may have hindered the development of attention and impulse control, beginning in late adolescence which is consistent with previous findings (78). With less attention to the environment, frequent marijuana users more often act without thinking and are less patient, potentially increasing unplanned risky behaviors including spontaneous substance use and violence (79). There is also ample evidence indicating that impulsivity is highly associated with vulnerability to addiction (80).
We further found a significant negative interaction effect between level of marijuana use and age on emotion recognition accuracy that may represent a long-term effect of frequent marijuana use. Emotion recognition is the focus of numerous previous studies to characterize marijuana users, however, they lack a substance naïve baseline (81–83). Nevertheless, based on those previous findings, we expected chronic marijuana users to exhibit difficulties in recognizing emotions, making the present findings intriguing. Although the marijuana user group performed better in the facial recognition task prior to initiation, this negative interaction over time suggests a decline in emotion recognition ability among frequent marijuana users that could become increasingly consequential in late adolescence into emerging adulthood. In effect, frequent marijuana users might experience difficulty in expressing empathy and attention, leading to peer relationship problems; accurately attributing facial expressions is important in interpersonal communications. There is also some evidence to suggest that marijuana users may consider consumption of substances as a substitute for friendship or a coping mechanism when relationship building is a challenge (84).
Overall, these findings implicate impaired acquisition and storage ability and heightened emotion recognition in adolescent marijuana users. However, unexpectedly, verbal learning ability was not identified in the growth modeling, suggesting that verbal learning is only influenced by marijuana in the short term. Lower levels of verbal learning ability, even when transient, may be expressed in poorer academic performance (85), potentially compounding risk for problematic substance use and other behavioral issues. It is possible that, with each use of marijuana, there is a short period of time when verbal learning ability is impaired, thereby affecting the ability to recall new words and discern connections between the words and their meaning (37), leading to difficulties in academic performance. The combination of academic challenges and heightened impulsivity during adolescence can have long-lasting consequences extending into adulthood, further increasing risk for ongoing or escalating use of marijuana and other substances.
These suppositions require further study to better understand how specific dimensions of EF predict and are impacted by initiation and patterns of use, and the potential for confounding effects when other substances are simultaneously used. Also of interest is the distinction between hot and cool EF, with the former involving emotional aspects of cognition (e.g., emotion regulation, reward-seeking, and impulsivity) and the latter involving neutral or decontextualized processes (e.g., verbal learning, cognitive flexibility, and working memory). In our study, both hot and cool EF were implicated in our measures of marijuana use at baseline and change over time. However, they played different roles. As aptly pointed out by Moriguchi and Phillips (86), these functions vary and begin to differentiate across development, showing different associations with behavioral patterns as well as neurobiological substrates. Understanding marijuana use precursors and consequences in this context is, thus, deemed an important line of inquiry.
There are a few implications of our findings for the development of intervention components that more specifically target these neurocognitive vulnerabilities to marijuana misuse and escalation. For example, programs to address trauma and bullying have potential to normalize attributions of negative emotions, thereby reducing aggressive behaviors and, in turn, may attenuate marijuana adverse effects on social interaction skills. Improving attention and reducing impulsivity are two additional skills that may offer some protection against using substances and experiencing their adverse effects in adolescence and beyond. Also, social emotional learning strategies build skills that tamp down on emotion reactivity, increase accuracy of emotion attribution in others and self, and improve classroom behavior and academic achievement (87). Information regarding neural circuits that undergird these abilities might further guide the selection of program components that act to strengthen precortical controls over emotion reactivity. Behavioral interventions that include focused meditation or other mindfulness practices and pharmacological approaches have been found to exert a beneficial effect at both the neural and behavioral levels (88, 89), improving the ability to cope with stress, strengthen cognitive control over emotional reactions, normalize emotion recognition, and calm aggressive tendencies, and may thus be useful in preventing marijuana use and dependence.
There are several limitations in the present study. First, the sample size declined significantly across waves, particularly by wave 3. Relatedly, the reduced sample in waves 3 and 4 may have created selection bias, leading to a narrowing in the gap between developmental trajectories of executive function and emotion recognition of marijuana users and non-users in this study. Although we applied MICE strategy in treating missing data and to compensate for the decreased power of our analyses, the ability to detect true effects is compromised. Third, due to the high comorbidity between marijuana and other substances such as alcohol and tobacco, participants who only used marijuana without exposure to these other substances represented a relatively smaller number of marijuana-only users (20 out of 148). The small sample of marijuana-only users compromised the power of our analyses when controlling for other substance use. On the other hand, the use of other potentially more harmful substances (e.g., cocaine, opioids, and methamphetamine) was relatively rare. Additionally, discrepancies occurred when participants reported marijuana use in an earlier wave and then denied ever using in a later wave. We considered participants who reported ever use in any of the two waves marijuana users, even if use was denied in a subsequent wave.
Clearly, future research should focus on extending the span of time and number of participants to better depict the developmental trajectories of executive cognitive function and emotion recognition and ways in which marijuana use impacts developmental trajectories. Regardless, our results suggest a pathway for future studies to determine whether the decline we observed in a few of these processes among adolescent marijuana users holds. Furthermore, longitudinal studies from adolescence into early adulthood are needed to trace the widening or narrowing of the gap in functioning relative to typically developing control participants. Assessing the combined effects of multiple substance use will also enhance our understanding of how adolescent development is influenced specifically by marijuana. One possible direction is the application of a creative design (e.g., twin study with clean baseline) to disentangle the unique influence of marijuana, while another option is to focus on the polysubstance use effect rather than an individual drug.
During the past few years, legalization of recreational marijuana use has received an increasing amount of support from the public and policymakers. Nineteen states, two territories and the District of Columbia have legalized small amounts for recreational use as of May 2022 (90). Research suggests that, similar to alcohol and tobacco, legalization of marijuana may have negative effects on adolescent development; even though the sale to underage youth is illegal, such reforms can directly or indirectly influence use in young people (91). Concerns among substance abuse experts revolve largely around research consistently establishing that beliefs regarding negative consequences of the use of any given substance are directly and inversely related to risk for initiation among adolescents (10). Evidence is emerging that, indeed, there has been a clear decline in adolescents’ perception that marijuana use is hazardous in recent years (8). And in states where marijuana has been legalized, a precipitous rise in use among adolescents has been reported (11). Given these trends in perceptions that correspond with changes in marijuana laws, whether and how marijuana usage and neurodevelopment are affected would be an important consideration in policymaking. Policies based on evidence are inherently more effective in protecting public health. The same applies to marijuana laws, where well-informed policies will be more appropriately and safely formulated, and the public can be informed of any potential risks prior to the enactment of any policy reforms. In essence, understanding the impact of adolescent marijuana use on cognitive development is of great value to provide insights into both the benefits and harms of the legalization process. That information can inform the decision-making of policymakers enabling them to take into account the potential consequences of legalizing marijuana use for recreational purposes and ensure that appropriate safeguards are in place.
The raw data supporting the conclusions of this article will be made available by the authors, without undue reservation. Requests should be directed to ZGZpc2hiZWluQHVuYy5lZHU=.
The studies involving human participants were reviewed and approved by RTI International. Written informed consent to participate in this study was provided by the participants’ legal guardian/next of kin.
The parent study was conceived of and executed by DF, who also contributed to the conceptual framework for the present investigation and wrote and edited the manuscript. WR developed the hypotheses, selected variables for and conducted the analyses, contributed to interpretations, and co-wrote the paper. All authors contributed to the article and approved the submitted version.
This investigation was supported by the National Institute on Drug Abuse (1R01DA022321-01A1).
Our gratitude is extended to the study teams at RTI International, the University of Maryland School of Medicine, The Pennsylvania State University, and our collaborators in the Kentucky school district. We also want to thank the participants and their families for their time and commitment to this study.
The authors declare that the research was conducted in the absence of any commercial or financial relationships that could be construed as a potential conflict of interest.
All claims expressed in this article are solely those of the authors and do not necessarily represent those of their affiliated organizations, or those of the publisher, the editors and the reviewers. Any product that may be evaluated in this article, or claim that may be made by its manufacturer, is not guaranteed or endorsed by the publisher.
1. Christie, D, and Viner, R. Adolescent development. BMJ. (2005) 330:301–4. doi: 10.1136/bmj.330.7486.301
2. Steinberg, L. A dual systems model of adolescent risk-taking. Dev Psychobiol. (2010) 52:24. doi: 10.1002/dev.20445
3. Steinberg, L. A social neuroscience perspective on adolescent risk-taking. Dev Rev. (2008) 28:78–106. doi: 10.1016/j.dr.2007.08.002
4. Albert, D, Chein, J, and Steinberg, L. Peer influences on adolescent decision making: peer influences on adolescent decision making. Curr Dir Psychol Sci. (2013) 22:114–20. doi: 10.1177/0963721412471347
5. Casey, BJ, and Jones, RM. Neurobiology of the adolescent brain and behavior: implications for substance use disorders. J Am Acad Child Adolesc Psychiatry. (2010) 49:1189–285. doi: 10.1097/00004583-201012000-00005
6. Chen, X, Yu, B, Lasopa, SO, and Cottler, LB. Current patterns of marijuana use initiation by age among US adolescents and emerging adults: implications for intervention. Am J Drug Alcohol Abuse. (2017) 43:261–70. doi: 10.3109/00952990.2016.1165239
7. Miech, RA, Johnston, LD, O’Malley, PM, Bachman, JG, Schulenberg, JE, and Patrick, ME. Monitoring the future national survey results on drug use, 1975–2019: Volume I, secondary school students. (2020) University of Michigan Institute for Social Research.
8. Johnston, LD, Miech, RA, O’Malley, PM, Bachman, JG, Schulenberg, JE, and Patrick, ME. Monitoring the future national survey results on drug use 1975–2021: Overview, key findings on adolescent drug use. (2022) University of Michigan Institute for Social Research.
9. Patrick, ME, Schulenberg, JE, Miech, RA, Johnston, LD, O’Malley, PM, and Bachman, JG Monitoring the future panel study annual report: National data on substance use among adults ages 19 to 60, 1976–2021. (2022) University of Michigan Institute for Social Research.
10. Cleveland, MJ, Feinberg, ME, Bontempo, DE, and Greenberg, MT. The role of risk and protective factors in substance use across adolescence. J Adolesc Health. (2008) 43:157–64. doi: 10.1016/j.jadohealth.2008.01.015
11. Lachance, A, Bélanger, RE, Riva, M, and Ross, NA. A systematic review and narrative synthesis of the evolution of adolescent and young adult Cannabis consumption before and after legalization. J Adolesc Health. (2022) 70:848–63. doi: 10.1016/j.jadohealth.2021.11.034
12. Volkow, ND, Koob, GF, Croyle, RT, Bianchi, DW, Gordon, JA, Koroshetz, WJ, et al. The conception of the ABCD study: from substance use to a broad NIH collaboration. Dev Cogn Neurosci. (2018) 32:4–7. doi: 10.1016/j.dcn.2017.10.002
13. Spear, LP. Adolescent neurodevelopment. J Adolesc Health. (2013) 52:S7–S13. doi: 10.1016/j.jadohealth.2012.05.006
14. Jacobus, J, Courtney, KE, Hodgdon, EA, and Baca, R. Cannabis and the developing brain: what does the evidence say? Birth Defects Res. (2019) 111:1302–7. doi: 10.1002/bdr2.1572
15. Sisk, CL, and Zehr, JL. Pubertal hormones organize the adolescent brain and behavior. Front Neuroendocrinol. (2005) 26:163–74. doi: 10.1016/j.yfrne.2005.10.003
16. Sotiras, A, Toledo, JB, Gur, RE, Gur, RC, Satterthwaite, TD, and Davatzikos, C. Patterns of coordinated cortical remodeling during adolescence and their associations with functional specialization and evolutionary expansion. Proc Natl Acad Sci U S A. (2017) 114:3527–32. doi: 10.1073/pnas.1620928114
17. Griffin, A. Adolescent neurological development and implications for health and well-being. Healthcare. (2017) 5:62. doi: 10.3390/healthcare5040062
18. Volkow, ND, Baler, RD, Compton, WM, and Weiss, SRB. Adverse health effects of marijuana use. N Engl J Med. (2014) 370:2219–27. doi: 10.1056/NEJMra1402309
19. Lubman, DI, Yücel, M, and Hall, WD. Substance use and the adolescent brain: a toxic combination? J Psychopharmacol. (2007) 21:792–4. doi: 10.1177/0269881107078309
21. Squeglia, LM, Jacobus, J, and Tapert, SF. The influence of substance use on adolescent brain development. Clin EEG Neurosci. (2009) 40:31–8. doi: 10.1177/155005940904000110
22. Bava, S, and Tapert, SF. Adolescent brain development and the risk for alcohol and other drug problems. Neuropsychol Rev. (2010) 20:398–413. doi: 10.1007/s11065-010-9146-6
23. Sharma, S, Arain,, Mathur,, Rais,, Nel, W, Sandhu,, et al. Maturation of the adolescent brain. Neuropsychiatr Dis Treat. (2013) 9:449–61. doi: 10.2147/NDT.S39776
24. Paus, T. How environment and genes shape the adolescent brain. Horm Behav. (2013) 64:195–202. doi: 10.1016/j.yhbeh.2013.04.004
25. Russo, EB. Cannabis and Cannabinoids: Pharmacology, Toxicology, and Therapeutic Potential. New York, NY: Routledge (2013).
26. Shollenbarger, SG, Price, J, Wieser, J, and Lisdahl, K. Impact of cannabis use on prefrontal and parietal cortex gyrification and surface area in adolescents and emerging adults. Dev Cogn Neurosci. (2015) 16:46–53. doi: 10.1016/j.dcn.2015.07.004
27. Yücel, M, Lorenzetti, V, Suo, C, Zalesky, A, Fornito, A, Takagi, MJ, et al. Hippocampal harms, protection and recovery following regular cannabis use. Transl Psychiatry. (2016) 6:e710. doi: 10.1038/tp.2015.201
28. Schweinsburg, AD, Brown, SA, and Tapert, SF. The influence of marijuana use on neurocognitive functioning in adolescents. Curr Drug Abuse Rev. (2008) 1:99–111. doi: 10.2174/1874473710801010099
29. Gruber, SA, Rogowska, J, and Yurgelun-Todd, DA. Altered affective response in marijuana smokers: an FMRI study. Drug Alcohol Depend. (2009) 105:139–53. doi: 10.1016/j.drugalcdep.2009.06.019
30. Metrik, J, Aston, ER, Kahler, CW, Rohsenow, DJ, McGeary, JE, and Knopik, VS. Marijuana’s acute effects on cognitive bias for affective and marijuana cues. Exp Clin Psychopharmacol. (2015) 23:339–50. doi: 10.1037/pha0000030
31. Bartholomew, J, Holroyd, S, and Heffernan, TM. Does cannabis use affect prospective memory in young adults? J Psychopharmacol. (2010) 24:241–6. doi: 10.1177/0269881109106909
32. Gonzalez, R, Pacheco-Colón, I, Duperrouzel, JC, and Hawes, SW. Does cannabis use cause declines in neuropsychological functioning? A review of longitudinal studies. J Int Neuropsychol Soc. (2017) 23:893–902. doi: 10.1017/S1355617717000789
33. Fontes, MA, Bolla, KI, Cunha, PJ, Almeida, PP, Jungerman, F, Laranjeira, RR, et al. Cannabis use before age 15 and subsequent executive functioning. Br J Psychiatry. (2011) 198:442–7. doi: 10.1192/bjp.bp.110.077479
34. Sagar, KA, Dahlgren, MK, Gönenç, A, Racine, MT, Dreman, MW, and Gruber, SA. The impact of initiation: early onset marijuana smokers demonstrate altered Stroop performance and brain activation. Dev Cogn Neurosci. (2015) 16:84–92. doi: 10.1016/j.dcn.2015.03.003
35. Hanson, KL, Winward, JL, Schweinsburg, AD, Medina, KL, Brown, SA, and Tapert, SF. Longitudinal study of cognition among adolescent marijuana users over three weeks of abstinence. Addict Behav. (2010) 35:970–6. doi: 10.1016/j.addbeh.2010.06.012
36. Schuster, RM, Hoeppner, SS, Evins, AE, and Gilman, JM. Early onset marijuana use is associated with learning inefficiencies. Neuropsychology. (2016) 30:405–15. doi: 10.1037/neu0000281
37. Pope, HG Jr, and Yurgelun-Todd, D. The residual cognitive effects of heavy marijuana use in college students. JAMA. (1996) 275:521–7. doi: 10.1001/jama.1996.03530310027028
38. Scott, JC, Slomiak, ST, Jones, JD, Rosen, AFG, Moore, TM, and Gur, RC. Association of cannabis with cognitive functioning in adolescents and young adults: a systematic review and Meta-analysis. JAMA Psychiat. (2018) 75:585–95. doi: 10.1001/jamapsychiatry.2018.0335
39. Crean, RD, Crane, NA, and Mason, BJ. An evidence based review of acute and long-term effects of cannabis use on executive cognitive functions. J Addict Med. (2011) 5:1–8. doi: 10.1097/ADM.0b013e31820c23fa
40. Allan, JL, McMinn, D, and Daly, M. A bidirectional relationship between executive function and health behavior: evidence, implications, and future directions. Front Neurosci. (2016) 10:386. doi: 10.3389/fnins.2016.00386
41. Gillespie, NA, Neale, MC, and Kendler, KS. Pathways to cannabis abuse: a multi-stage model from cannabis availability, cannabis initiation and progression to abuse. Addiction. (2009) 104:430–8. doi: 10.1111/j.1360-0443.2008.02456.x
42. Verweij, KJH, Zietsch, BP, Lynskey, MT, Medland, SE, Neale, MC, Martin, NG, et al. Genetic and environmental influences on cannabis use initiation and problematic use: a meta-analysis of twin studies. Addiction. (2010) 105:417–30. doi: 10.1111/j.1360-0443.2009.02831.x
43. Jager, G, van Hell, HH, de Win, MML, Kahn, RS, van den Brink, W, van Ree, JM, et al. Effects of frequent cannabis use on hippocampal activity during an associative memory task. Eur Neuropsychopharmacol. (2007) 17:289–97. doi: 10.1016/j.euroneuro.2006.10.003
44. Fishbein, DH, Novak, SP, Ridenour, TA, Thornburg, V, Hammond, J, and Brown, J. Neurocognitive characteristics of early marijuana use initiation in adolescents: a signature mapping analysis. J Stud Alcohol Drugs. (2016) 77:431–40. doi: 10.15288/jsad.2016.77.431
45. Richter, L, and Johnson, PB. Current methods of assessing substance use: a review of strengths, problems, and developments. J Drug Issues. (2001) 31:809–32. doi: 10.1177/002204260103100401
46. Ehrenreich, H, Kunert, HJ, Moeller, MR, Poser, W, Schilling, L, Gigerenzer, G, et al. Specific attentional dysfunction in adults following early start of cannabis use. Psychopharmacology. (1999) 142:295–301. doi: 10.1007/s002130050892
47. Riccio, CA, Reynolds, CR, and Lowe, PA. Clinical Applications of Continuous Performance Tests: Measuring Attention and Impulsive Responding in Children and Adults. Nashville, TN: John Wiley & Sons (2008).
48. Schmidt, M. Rey Auditory Verbal Learning Test: A Handbook, vol. 17. Los Angeles, CA: Western Psychological Services (1996).
49. Parsons, OA, Tarter, RE, and Edelberg, R. Altered motor control in chronic alcoholics. J Abnorm Psychol. (1972) 80:308–14. doi: 10.1037/h0033740
50. Bechara, A, Damasio, AR, Damasio, H, and Anderson, SW. Insensitivity to future consequences following damage to human prefrontal cortex. Cognition. (1994) 50:7–15. doi: 10.1016/0010-0277(94)90018-3
51. Bechara, A, Damasio, H, Tranel, D, and Damasio, AR. Deciding advantageously before knowing the advantageous strategy. Science. (1997) 275:1293–5. doi: 10.1126/science.275.5304.1293
52. Whitlow, CT, Liguori, A, Brooke Livengood, L, Hart, SL, Mussat-Whitlow, BJ, Lamborn, CM, et al. Long-term heavy marijuana users make costly decisions on a gambling task. Drug Alcohol Depend. (2004) 76:107–11. doi: 10.1016/j.drugalcdep.2004.04.009
53. Ernst, M, Grant, SJ, London, ED, Contoreggi, CS, Kimes, AS, and Spurgeon, L. Decision making in adolescents with behavior disorders and adults with substance abuse. Am J Psychiatry. (2003) 160:33–40. doi: 10.1176/appi.ajp.160.1.33
54. Shad, MU, Bidesi, AS, Chen, L-A, Thomas, BP, Ernst, M, and Rao, U. Neurobiology of decision-making in adolescents. Behav Brain Res. (2011) 217:67–76. doi: 10.1016/j.bbr.2010.09.033
55. Williams, JMG, Mathews, A, and MacLeod, C. The emotional Stroop task and psychopathology. Psychol Bull. (1996) 120:3–24. doi: 10.1037/0033-2909.120.1.3
56. Ekman, P, and Friesen, P. Unmasking the Face: A Guide to Recognizing Emotions From Facial. Vol. 10. Ishk. (2003).
57. Tottenham, N, Tanaka, JW, Leon, AC, McCarry, T, Nurse, M, Hare, TA, et al. The NimStim set of facial expressions: judgments from untrained research participants. Psychiatry Res. (2009) 168:242–9. doi: 10.1016/j.psychres.2008.05.006
58. Bowie, CR, and Harvey, PD. Administration and interpretation of the trail making test. Nat Protoc. (2006) 1:2277–81. doi: 10.1038/nprot.2006.390
59. Lezak, MD, Howieson, DB, Loring, DW, Hannay, HJ, and Fischer, JS. Neuropsychological Assessment. 4th ed. New York, NY: Oxford University Press (2004).
60. Cudeck, R, and O’Dell, LL. Applications of standard error estimates in unrestricted factor analysis: significance tests for factor loadings and correlations. Psychol Bull. (1994) 115:475–87. doi: 10.1037//0033-2909.115.3.475
61. Conway, KP, Levy, J, Vanyukov, M, Chandler, R, Rutter, J, Swan, GE, et al. Measuring addiction propensity and severity: the need for a new instrument. Drug Alcohol Depend. (2010) 111:4–12. doi: 10.1016/j.drugalcdep.2010.03.011
62. Carliner, H, Mauro, PM, Brown, QL, Shmulewitz, D, Rahim-Juwel, R, Sarvet, AL, et al. The widening gender gap in marijuana use prevalence in the U.S. during a period of economic change, 2002–2014. Drug Alcohol Depend. (2017) 170:51–8. doi: 10.1016/j.drugalcdep.2016.10.042
63. Rodondi, N, Pletcher, MJ, Liu, K, Hulley, SB, and Sidney, S. Coronary artery risk development in young adults (CARDIA) study. Marijuana use, diet, body mass index, and cardiovascular risk factors (from the CARDIA study). Am J Cardiol. (2006) 98:478–84. doi: 10.1016/j.amjcard.2006.03.024
64. Humensky, JL. Are adolescents with high socioeconomic status more likely to engage in alcohol and illicit drug use in early adulthood? Subst Abuse Treat Prev Policy. (2010) 5:19. doi: 10.1186/1747-597X-5-19
65. Patrick, ME, Wightman, P, Schoeni, RF, and Schulenberg, JE. Socioeconomic status and substance use among young adults: a comparison across constructs and drugs. J Stud Alcohol Drugs. (2012) 73:772–82. doi: 10.15288/jsad.2012.73.772
66. van Buuren, S, and Groothuis-Oudshoorn, K. Multivariate imputation by chained equations in R. J Stat Softw. (2011) 45:1–67. doi: 10.18637/jss.v045.i03
67. White, IR, Royston, P, and Wood, AM. Multiple imputation using chained equations: issues and guidance for practice. Stat Med. (2011) 30:377–99. doi: 10.1002/sim.4067
68. Crone, EA. Executive functions in adolescence: inferences from brain and behavior: executive functions in adolescence. Dev Sci. (2009) 12:825–30. doi: 10.1111/j.1467-7687.2009.00918.x
69. Ajmera, N, Collins, PF, Weiss, H, and Luciana, M. Initiation of moderately frequent cannabis use in adolescence and young adulthood is associated with declines in verbal learning and memory: a longitudinal comparison of pre- versus post-initiation cognitive performance. J Int Neuropsychol Soc. (2021) 27:621–36. doi: 10.1017/S1355617721000631
70. Curran, PJ, Obeidat, K, and Losardo, D. Twelve frequently asked questions about growth curve modeling. J Cogn Dev. (2010) 11:121–36. doi: 10.1080/15248371003699969
71. Hesser, H. Modeling individual differences in randomized experiments using growth models: recommendations for design, statistical analysis and reporting of results of internet interventions. Internet Interv. (2015) 2:110–20. doi: 10.1016/j.invent.2015.02.003
72. Ernst, M, Luckenbaugh, DA, Moolchan, ET, Temple, VA, Jenness, J, Korelitz, KE, et al. Decision-making and facial emotion recognition as predictors of substance-use initiation among adolescents. Addict Behav. (2010) 35:286–9. doi: 10.1016/j.addbeh.2009.10.014
73. Assed, MM, Khafif, TC, Belizario, GO, Fatorelli, R, Rocca CC de, A, and de Pádua Serafim, A. Facial emotion recognition in maltreated children: a systematic review. J Child Fam Stud. (2020) 29:1493–509. doi: 10.1007/s10826-019-01636-w
74. Dubowitz, H, Thompson, R, Arria, AM, English, D, Metzger, R, and Kotch, JB. Characteristics of child maltreatment and adolescent marijuana use: a prospective study: a prospective study. Child Maltreat. (2016) 21:16–25. doi: 10.1177/1077559515620853
75. Ernst, M, Luckenbaugh, DA, Moolchan, ET, Leff, MK, Allen, R, Eshel, N, et al. Behavioral predictors of substance-use initiation in adolescents with and without attention-deficit/hyperactivity disorder. Pediatrics. (2006) 117:2030–9. doi: 10.1542/peds.2005-0704
76. Clifford, ME, Nguyen, AJ, and Bradshaw, CP. Emotion processing associated with aggression in early adolescents: a focus on affective theory of mind. Aggress Behav. (2021) 47:173–82. doi: 10.1002/ab.21936
77. Gruber, SA, Sagar, KA, Dahlgren, MK, Racine, M, and Lukas, SE. Age of onset of marijuana use and executive function. Psychol Addict Behav. (2012) 26:496–506. doi: 10.1037/a0026269
78. Paige, KJ, and Colder, CR. Long-term effects of early adolescent marijuana use on attentional and inhibitory control. J Stud Alcohol Drugs. (2020) 81:164–72. doi: 10.15288/jsad.2020.81.164
79. Romer, D. Adolescent risk taking, impulsivity, and brain development: implications for prevention. Dev Psychobiol. (2010) 52:263–76. doi: 10.1002/dev.20442
80. Verdejo-García, A, Lawrence, AJ, and Clark, L. Impulsivity as a vulnerability marker for substance-use disorders: review of findings from high-risk research, problem gamblers and genetic association studies. Neurosci Biobehav Rev. (2008) 32:777–810. doi: 10.1016/j.neubiorev.2007.11.003
81. Bayrakçı, A, Sert, E, Zorlu, N, Erol, A, Sarıçiçek, A, and Mete, L. Facial emotion recognition deficits in abstinent cannabis dependent patients. Compr Psychiatry. (2015) 58:160–4. doi: 10.1016/j.comppsych.2014.11.008
82. Fluharty, ME, Heron, J, and Munafò, MR. Longitudinal associations of social cognition and substance use in childhood and early adolescence: findings from the Avon longitudinal study of parents and children. Eur Child Adolesc Psychiatry. (2018) 27:739–52. doi: 10.1007/s00787-017-1068-x
83. Spechler, PA, Orr, CA, Chaarani, B, Kan, K-J, Mackey, S, Morton, A, et al. Cannabis use in early adolescence: evidence of amygdala hypersensitivity to signals of threat. Dev Cogn Neurosci. (2015) 16:63–70. doi: 10.1016/j.dcn.2015.08.007
84. Foster, K, and Spencer, D. “It’s just a social thing”: drug use, friendship and borderwork among marginalized young people. Int J Drug Policy. (2013) 24:223–30. doi: 10.1016/j.drugpo.2012.12.005
85. Solowij, N, Jones, KA, Rozman, ME, Davis, SM, Ciarrochi, J, Heaven, PCL, et al. Verbal learning and memory in adolescent cannabis users, alcohol users and non-users. Psychopharmacology. (2011) 216:131–44. doi: 10.1007/s00213-011-2203-x
86. Moriguchi, Y, and Phillips, S. Evaluating the distinction between cool and hot executive function during childhood. Brain Sci. (2023) 13:313. doi: 10.3390/brainsci13020313
87. Mahoney, JL, Durlak, JA, and Weissberg, RP. An update on social and emotional learning outcome research. Phi Delta Kappan. (2018) 100:18–23. doi: 10.1177/0031721718815668
88. Menezes, CB, de Paula Couto, MC, Buratto, LG, Erthal, F, Pereira, MG, and Bizarro, L. The improvement of emotion and attention regulation after a 6-week training of focused meditation: a randomized controlled trial. Evid Based Complement Alternat Med. (2013) 2013:984678. doi: 10.1155/2013/984678
89. Ramey, T, and Regier, PS. Cognitive impairment in substance use disorders. CNS Spectr. (2019) 24:102–13. doi: 10.1017/S1092852918001426
90. Hanson, K, and Garcia, A (2022). State medical cannabis laws. Ncslorg. Available at: https://www.ncsl.org/research/health/state-medical-marijuana-laws.aspx (Accessed September 19, 2022)
Keywords: adolescence, marijuana use, neurocognitive functioning, emotion recognition, longitudinal, substance-naïve baseline, legalization
Citation: Ren W and Fishbein D (2023) Prospective, longitudinal study to isolate the impacts of marijuana use on neurocognitive functioning in adolescents. Front. Psychiatry. 14:1048791. doi: 10.3389/fpsyt.2023.1048791
Received: 20 September 2022; Accepted: 19 April 2023;
Published: 15 May 2023.
Edited by:
Antoine Bechara, University of Southern California, United StatesReviewed by:
Elissa Weitzman, Harvard Medical School, United StatesCopyright © 2023 Ren and Fishbein. This is an open-access article distributed under the terms of the Creative Commons Attribution License (CC BY). The use, distribution or reproduction in other forums is permitted, provided the original author(s) and the copyright owner(s) are credited and that the original publication in this journal is cited, in accordance with accepted academic practice. No use, distribution or reproduction is permitted which does not comply with these terms.
*Correspondence: Diana Fishbein, ZGZpc2hiZWluQHVuYy5lZHU=
Disclaimer: All claims expressed in this article are solely those of the authors and do not necessarily represent those of their affiliated organizations, or those of the publisher, the editors and the reviewers. Any product that may be evaluated in this article or claim that may be made by its manufacturer is not guaranteed or endorsed by the publisher.
Research integrity at Frontiers
Learn more about the work of our research integrity team to safeguard the quality of each article we publish.