- 1School of Mental Health, Jining Medical University, Jining, China
- 2Shandong Mental Health Center, Shandong University, Jinan, China
- 3Cheeloo College of Medicine, Shandong University, Jinan, China
- 4School of Psychology, Shandong Normal University, Jinan, China
Introduction: Superior longitudinal fasciculus (SLF) is a white matter (WM) tract that connects the frontal, parietal and temporal lobes. SLF integrity has been widely assessed in neuroimaging studies of psychiatric disorders, such as schizophrenia (SZ), bipolar disorder (BD), and attention-deficit/hyperactivity disorder (ADHD). However, prior studies have revealed inconsistent findings and comparisons across disorders have not been fully examined.
Methods: Here, we obtained data for 113 patients (38 patients with SZ, 40 with BD, 35 with ADHD) and 94 healthy controls from the UCLA Consortium for Neuropsychiatric Phenomic LA5c dataset. We assessed the integrity of 20 major WM tracts with a novel segmentation method by automating fiber tract quantification (AFQ). The AFQ divides each tract into 100 equal parts along the direction of travel, with fractional anisotropy (FA) of each part taken as a characteristic. Differences in FA among the four groups were examined.
Results: Compared to healthy controls, patients with SZ showed significantly lower FA in the second half (51–100 parts) of the SLF. No differences were found between BD and healthy controls, nor between ADHD and healthy controls. Results also demonstrated that patients with SZ showed FA reduction in the second half of the SLF relative to patients with BP. Moreover, greater FA in patients in SLF was positively correlated with the manic-hostility score of the Brief Psychiatry Rating scale.
Discussion: These findings indicated that differences in focal changes in SLF might be a key neurobiological abnormality contributing to characterization of these psychiatric disorders.
Introduction
Psychiatric disorders such as schizophrenia (SZ), bipolar disorder (BD), and attention-deficit/hyperactivity disorder (ADHD) are substantial causes of the global growing disability and morality. Among these mental disorders, similar neurocognitive deficits (1) and underlying genetic basis (2, 3) have been found. For instance, differences in brain networks associated with memory and cognitive control have been reported in these disorders, particularly those in prefrontal, default mode, and limbic networks (4, 5). Notably, symptom profiles differ across these disorders, with SZ high in psychotic features, BD increased in emotional dysfunction and ADHD high in externalizing behaviors. Exploring the neuropathological characteristics across psychiatric disorders is critical for understanding their pathophysiology. Brain neuroimaging studies have identified imbalanced connectivity within and between the attention, frontoparietal, default, and reword networks among psychiatric disorders (6). Investigating structural connectivity abnormalities could help understand dysfunction of these brain networks. Structural connectivity refers to brain white matter (WM), which consists of myelinated fiber bundles and structurally connects regions that are not physically proximal. In human brain, major long WM tracts, such as superior longitudinal fasciculus (SLF), are largely responsible for communications between various areas of the cerebral cortex. Disturbances in these tracts or neural networks are considered important etiopathogenetic factors of psychiatric disorders. Previous studies have found altered WM tracts among the three psychiatric disorders, such as the prefrontal lobe, temporal lobe, and anterior corona radiata fiber tract (7–12). However, to what extent these three psychiatric disorders have shared and distinct WM abnormalities remains elusive. There is a need to explore the microstructure of WM integrity across psychiatric disorders.
Structural connectivity disturbances are considered an important etiopathogenetic factors of major psychoses (13), including SZ and BD. They were identified within large-scale disconnected brain networks (14). Among WM tracts, the SLF has been widely reported to show reduced fractional anisotropy (FA) in many studies (15–18). SLF is a large WM bundle that connects and allows communication among the frontal, parietal, and temporal lobes (Figure 1). The main function of the SLF consists of attention, executive control, visual and spatial cognition, control of motor processes, and language functions (19). Decreased FA has also been reported in patients at high risk of psychosis (20–25). Prior WM in vivo magnetic resonance imaging (MRI) studies of SZ and BD have demonstrated decreased FA in SLF in both disorders (16) with greater heterogeneity in findings of BD (26–28).
Attention-deficit/hyperactivity disorder has also been found related to defects in brain networks involving in cognitive, affective, and motor behaviors (29). Similar to SZ and BD, cognitive deficits of ADHD consist of attention, memory, response control, emotional instability, and impulsive behaviors. Abnormality in the SLF, which showed associations with these symptoms, has been reported in patients with ADHD (30–35). For example, in a longitudinal follow-up study, Cortese et al. (34) suggested that decreased FA may be a lasting characteristic change in patients with ADHD. The decline of FA in SLF continued into adulthood and did not recover as symptoms got better. While Silk et al. (36) found increased FA in the SLF in ADHD patients, some other studies found no significant difference compared to healthy controls (37–41). Further studies are needed to investigate abnormalities in the SLF in ADHD patients.
Previous inconsistent findings in psychiatric disorders might be partly due to methodological differences across studies. The region of interest (ROI) based approach and the tract-based spatial statistics were frequently utilized to analyze Diffusion-weighted images (DWI). The two methods analyze WM microstructures across the whole brain. However, they were of limited utility in investigating the integrity of specific WM tracts. Tractography might be a more suitable method to systematically identify and quantify WM tracts in the human brain. In recent years, Automated Fiber Quantification (AFQ) shows up as an advance in tractography and has been successfully applied into psychiatric disorders. This allows researchers to quantify diffusion measures at sample positions from the start to the end of each reconstructed tract. Some studies have applied AFQ to reveal microstructural changes in WM tracts in SZ, BD, and patients with multiple sclerosis, obsessive-compulsive disorder, and Alzheimer’s disease (42–46). Importantly, all these above studies found that microstructural abnormalities in WM tracts were focal, not entire.
Considering inconsistent findings and fewer comparisons across psychiatric disorders, we used the novel AFQ method to automatically reconstruct WM fibers in patients with SZ, BD, and ADHD. We aimed to identify focal changes of the SLF in these three disorders by comparing healthy controls to each patient group. The shared and distinct alterations of FA in SLF were then explored in patient groups. Correlation analyses were also performed to test whether any focal abnormalities in the patient groups were associated with clinical symptoms. We hypothesized that patients with SZ, BD, and ADHD would show abnormal FA in SLF relative to healthy adults and that differences in FA would be associated with severity of clinical symptoms.
Materials and methods
Participants
Participants of this study were from the UCLA Consortium for Neuropsychiatric Phenomics (CNP). The CNP dataset is available via the OpenfMRI project1 and is comprised of healthy controls and patients with SZ, BD, and ADHD. The detailed information on demographic, clinical, and neuroimaging data of participants has been described in the public data descriptor (1). Both T1-weighted imaging and DWI for all participants were inspected by two authors (FX and KW) and 22 participants with excessive head motion and missing image data were excluded. The analysis sample of this study included 207 participants, which consisted of 113 patients including 38 adults with SZ (mean age = 35.68 ± 9.32 years; F/M = 9/29), 40 adults with BD (mean age = 34.50 ± 8.95 years; F/M = 18/22), and 35 adults with ADHD (mean age = 32.11 ± 10.45 years; F/M = 16/19), and 94 healthy participants (mean age = 31.56 ± 8.13 years; F/M = 43/51). Demographic and clinical information of the analysis sample is shown in Table 1.
All participants were aged 21–50 years and right-handed. As shown in the data descriptor (1), all of them were interviewed using the Diagnostic and Statistical Manual of Mental Disorders, Fourth Edition, and were based on the Structured Clinical Interview for DSM-IV supplemented by the Adult ADHD Interview (a structured interview form derived from the Kiddie Schedule for Affective Disorders and SZ, Present and Lifetime Version). ADHD criteria were assessed using Adult ADHD Interview.
Exclusion criteria of healthy group were any lifetime diagnosis of major psychiatric disorder. For the patient groups (SZ, BD, and ADHD), patients with other diagnoses or having used stable medications were excluded. For MRI scans, we excluded participants who were left-handed, who were pregnant, and those had other contraindications to scanning (e.g., claustrophobia, metal in the body). The UCLA CNP Study was approved by the UCLA institutional review board and was conducted following the Declaration of Helsinki. The current study was approved by the institutional review boards at the Shandong Normal University.
Symptom assessment
Clinical symptoms in patient groups were assessed using clinical rating scales and self-report questionnaires. Psychotic symptoms were evaluated using the Brief Psychiatric Rating Scale (BPRS). Affective states were assessed with the Young Mania Rating Scale (YMRS) and Hamilton Psychiatric Rating Scale for Depression (HAMD). The adult ADHD Self-Report Scale (ASRS) was a self-report screening scale for ADHD. For more details about these four scales, see Supplementary Material.
Magnetic resonance imaging acquisition parameters
MRI data were obtained using 3-T Siemens Trio scanner, which was located at UCLA, with the parameters for the T1-weighted high-resolution structural MPRAGE images: slice thickness, 1 mm; 176 slices; repetition time (TR), 1900 ms; echo time (TE), 2.26 ms; matrix, 256 × 256; FOV, 250 × 250 mm2. DWI were scanned with the following parameters: 64 directions; slice thickness, 2 mm; TR, 9000 ms; TE, 93 ms; 1 average; matrix, 96 × 96; flip angle, 90 degree; b-value, 1000 s/mm2.
Image processing
All the DWI images were preprocessed by the VISTA pipeline,2 consisting of eddy-current correction, motion correction, T1 image alignment. Then, reconstruction of tractography was performed using the freely available AFQ package,3 which was a MATLAB toolbox and used to identify and quantify WM tracts in each subject’s brain. AFQ is a multi-step reconstructing procedure, which includes (1) all fibers are tracked within a WM mask using deterministic tractography (47); (2) each fiber was then qualified by using Wakana’s waypoint ROI procedure (48); (3) fiber tract refinement was performed according to the track probability maps (49); (4) after refinement, fiber tract cleaning applies the outlier rejection algorithm (47); (5) quantification of the diffusion measurements along each fiber track at 100 equidistant nodes. The FA values were averaged in cross-sections (i.e., segments) along the WM tracts (42) in each participant. While we focused on the SLF in this study, additional 18 major WM tracts were also examined, including bilateral anterior thalamic radiation (ATR), corticospinal tract (CST), cingulum cingulate (CCing), cingulum hippocampus (CHippo), inferior frontal-occipital fasciculus (IFOF), inferior longitudinal fasciculus (ILF), uncinated fasciculus (UF), arcuate fasciculus (AF), and corpus callosum (CC) (48). For a detailed characterization of FA along the SLF, we used the 100 segments as prior studies suggested (42, 45, 46).
Statistical analysis
All statistical analyses were performed using IBM SPSS Statistics 26. Demographic and clinical variables were evaluated by analysis of variance and χ2 analysis between diagnostic groups. Using a three-way mixed-design analysis of covariance (ANCOVA), we examined the main effect of four groups on FA of the left and right SLF. Particularly, LATERALITY (1. Left; 2. Right) and SEGMENTS (1. 1–50 segments; 2. 51–100 segments) were regarded as within subject factors. Then, post-hoc comparisons were tested across these four groups. Finally, partial correlation analyses were conducted to determine the correlations between the mean FA values of each part of the SLF (1. 1–50 segments of left SLF; 2. 51–100 segments of left SLF; 3. 1–50 segments of right SLF; 4. 51–100 segments of right SLF) and seven clinical symptoms in patient groups. Given the effect of age and gender on WM, we included them as covariates in all above analyses. Here, we corrected the significance with Bonferroni method for multiple comparisons. The significance threshold was set at p = 0.05/7.
Additional analyses
To validate our main findings, we firstly examined influence of medication status on the tracts. In each patient group, we classified subjects into subgroups according to their medication status (i.e., use of antipsychotics or other treatments for SZ; use of mood stabilizers or other treatments and antidepressants or other treatments for BD; and the use of psycho-stimulants of other treatments for ADHD). Then, two-sample t-tests were performed between each of the corresponding pairs of subgroups for the FA values of segments.
Second, in our current sample, 16 patients were diagnosed comorbidity with a secondary diagnosis (SZ patients comorbid with ADHD, n = 6; BD patients comorbid with ADHD, n = 7; SZ patients comorbid with BD, n = 2; ADHD patients comorbid with BD, n = 1; no patients comorbid with SZ). To partial out the impact of comorbidity, we excluded these 16 patients and performed the same statistical analyses to verify the results.
Results
Demographic and clinical characteristics
Demographic and clinical characteristics of the four groups are shown in Table 1. There were no significant differences in age or gender among the SZ, BD, ADHD, and HC groups. As expected, significant differences were observed in positive symptom, negative symptom and anxiety-depression among the three patient groups (all ps < 0.05).
Differences in fractional anisotropy between patient groups
After accounting for the effects of age and gender, a three-way mixed-design ANCOVA revealed a significant main effect of GROUP (between-subject effect: F [3, 201] = 3.687; p = 0.013) on FA in the second part (51–100 consecutive segments) of bilateral SLF. This analysis also revealed a significant effect of LATERALITY (within-subjects effect; F [1, 201] = 16.028; p < 0.05) and an effect of SEGMENT (within-subjects effect; F [49, 9849] = 36.192; p < 0.05). There were no significant group differences in the first part (1–50 consecutive segments) of SLF (Table 2).
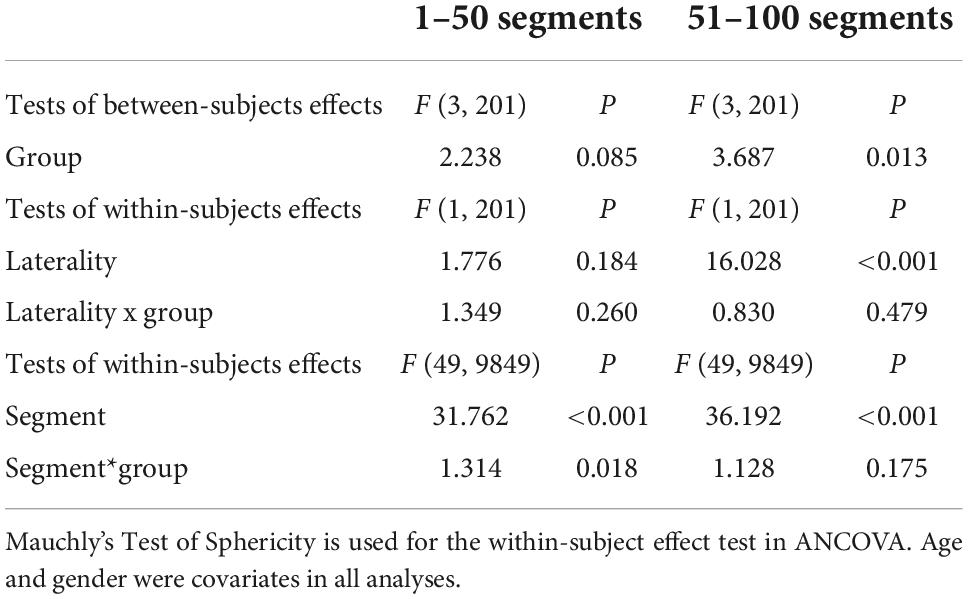
Table 2. Three-way mixed design analysis of covariance (ANCOVA) in Superior longitudinal fasciculus in three kinds of psychiatric disorders and healthy controls.
Post-hoc analyses revealed that patients with SZ exhibited lower FA in the bilateral SLF than patients with BD in 51–100 segments (p < 0.05). There were no significant differences in FA between patients with BD and ADHD and healthy controls (ps > 0.05) (Figures 2, 3). Additionally, analyses for FA values of another 18 WM tracts found no significant differences between groups.
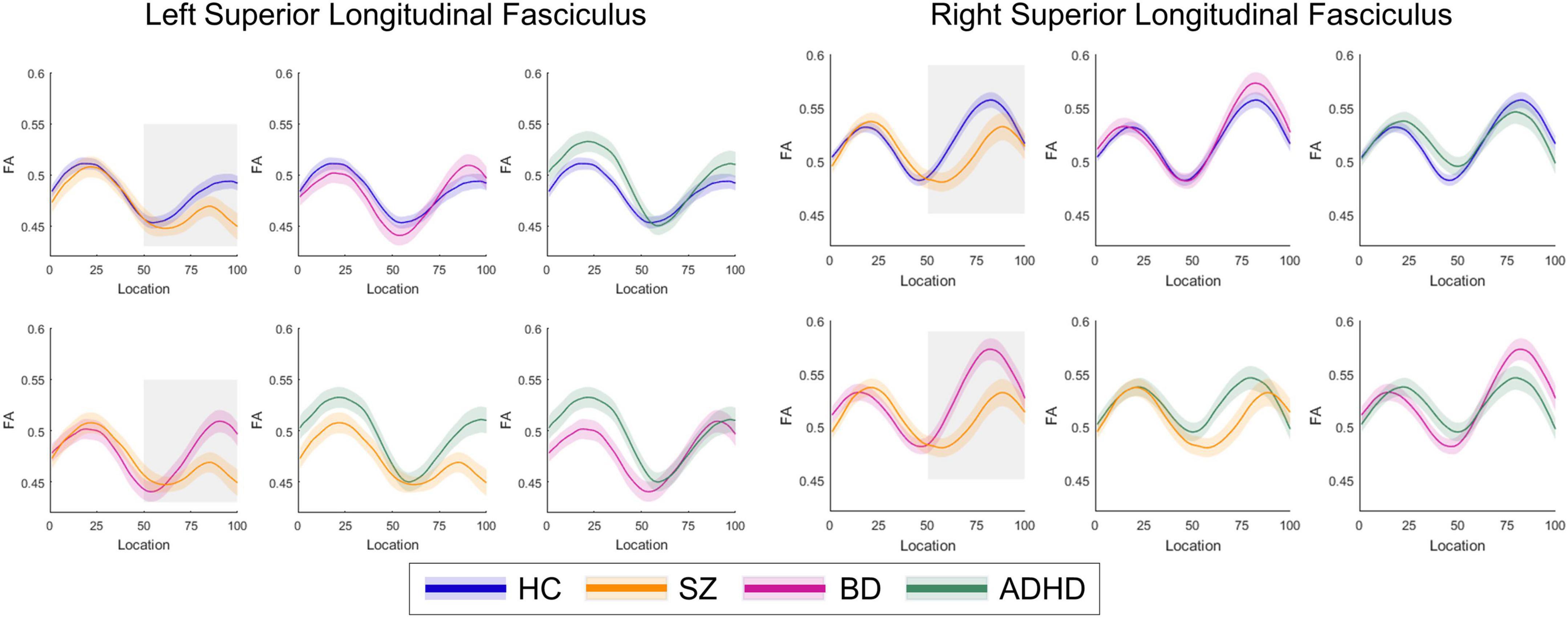
Figure 2. The plots of fractional anisotropy (FA) profiles of bilateral superior longitudinal fasciculus from healthy control individuals and patient subgroups.
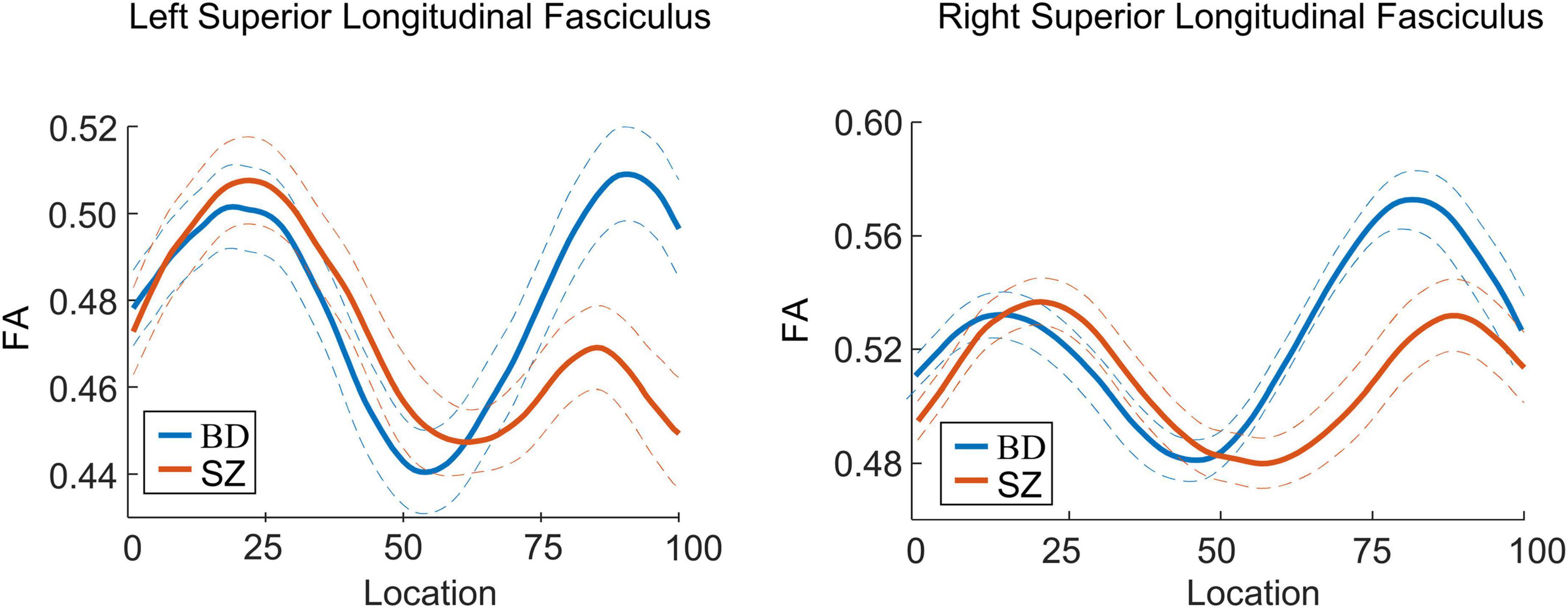
Figure 3. Curve-trajectory plots represent the mean fractional anisotropy (FA) along the left and right superior longitudinal fasciculus in schizophrenia (SZ) vs. bipolar disorder (BD).
Clinical relationships
Partial correlation analyses were carried out to assess the relationships between mean FA values of the SLF and clinical characteristics in each patient group and all patients. Analyses across all patients found a significantly positive relationship between the mean FA value in the second part (51–100 segments) of the right SLF and the manic-hostility score of the BPRS (r = 0.285, p < 0.05). This positive association was also significant in patients with SZ (r = 0.391, p < 0.05). In patients with BD, higher mean FA values in the second part (51–100 segments) of the left SLF were associated with higher negative scores of the BPRS (r = 0.323, p < 0.05). No significant association was found in the ADHD group.
Additional analyses
First, significant differences were observed in the first and second parts of the left SLF in patients with BD who had used mood stabilizers and other treatments. In SZ and ADHD groups, we did not observe any significant differences between unmedicated and medicated statuses (Supplementary Table 1).
Analyses excluding the 16 patients with comorbidity revealed same results. We found a significant main effect of GROUP (between-subject effect: F [3, 185] = 4.086; p = 0.008) on FA in the second part (51–100 consecutive segments) of bilateral SLF, and significant effects of LATERALITY (within-subjects effect; F [1, 185] = 14.050; p < 0.05) and SEGMENT (within-subjects effect; F [49,9065] = 33.449; p < 0.05). No significant group differences were found in the first part (1–50 consecutive segments) of SLF (Supplementary Table 2).
Discussion
The present study aimed to examine whether the WM bundles were focal or extended in patients with SZ, BD, and ADHD by using the tract-profile approach. We found lower collinearity of fibers in posterior bilateral SLF tracts in adults with SZ compared to the healthy group, but no differences in adults with BD or ADHD. Comparisons between disorders revealed that patients with SZ had lower FA in half of parts of bilateral SLF compared to those with BD. Additionally, FA of the posterior right SLF was correlated with the manic-hostility score of the BPRS across all patients.
White matter impairments in patients with SZ were found in SLF, which was in line with prior studies (50–53). Our findings further demonstrated that lower FA in the SLF was focal, not in the entire tract. Reduced FA may indicate impaired myelination, altered fiber organization, or aberrant axon morphology. The focal abnormalities in this tract probably reflected abnormalities in the collinearity/integrity of specific fibers that connected the frontal lobe with occipital, parietal, and temporal lobes. Regarding BD patients, a prior study found a trend toward increased FA in posterior of bilateral SLF compared to healthy controls (27). Here, we showed that patients with SZ had lower FA in the left and right posterior SLF when compared to BD patients. By contrast, a previous study reported no significant group differences in structural connectivity of SLF between SZ and BD groups (27). The difference in patient characteristics such as age, gender, medical status, and the tractography method in the previous study may be reasons for the inconsistency. Further research using various tractography methods and a homogenous patient sample are required to verify findings of this study. Given the results of our study, SLF may be a distinct neural marker for the two disorders, and this tract might be more affected and disrupted in SZ than in BD. A previous diffusion study has employed similar tractography approaches and compared children patients with BD and patients with ADHD (11). As in this study, the authors found no diffusion imaging abnormalities in the SLF. In fact, within the attentional network model, the microstructure of the SLF might be an important mechanism underlying poor attention in patients with ADHD (54). Further research with a larger sample size is needed to elucidate the distinction in SLF between ADHD and healthy group.
We also found that higher FA values in the posterior right SLF were associated with higher manic-hostility scores across the patients. This suggested that the partial SLF abnormalities may contribute to manic-hostility symptoms. A previous study reported that greater thickness in the frontoparietal network was positively correlated with hostility score in patients with BD (55). There was no published literature on the association between manic-hostility symptoms and microstructural changes of SLF in mental disorders. Our research might serve as an explorative finding, and potential associations warrant further research.
Several limitations should be considered. First, this is a cross-sectional study and thus the causal relationship between focal SLF abnormalities and SZ remains to be explored. Longitudinal research are wanted in future to examine the development trajectories of SLF tracts among these psychiatric disorders. Second, impact of the age of onset has not been considered due to lack of data. Kelly et al. (15) found that the duration of illness could be an important factor in WM impairments in SZ. They also found that several brain regions showed a negative association between FA values and duration of illness. Third, type of medications might influence the microstructural integrity of the WM tracts. A prior study found that a higher proportion of patients taking antipsychotics was associated with smaller FA reduction in patients with first-episode psychosis (56). Although we examined impact of medication and did not find any significant differences in SZ, our results need to be validated in future studies in drug-naive first-episode patients.
In summary, using a tract-profile approach in patients with SZ, BD, and ADHD, we demonstrated focal abnormalities in the posterior of the SLF in patients with SZ. In contrast, no significant differences were found in patients with BD and ADHD when compared to the healthy group. The posterior of the SLF links the regions between frontal and parietal cortices and has been proposed to have a key role in the pathophysiology of SZ. Correlation between focal abnormalities of the right SLF and the manic-hostility symptom was also found. Our finding may encourage further research on focal WM abnormalities and the common and distinct pathophysiology of psychiatric disorders. Future research is needed to validate our results and further examine the effect of confounding factors such as medications and duration of illness.
Data availability statement
The datasets presented in this study can be found in online repositories. The names of the repository/repositories and accession number(s) can be found below: http://openfmri.org.
Ethics statement
The studies involving human participants were reviewed and approved by the Institutional Review Board of the Shandong Mental Health Center. The patients/participants provided their written informed consent to participate in this study. Written informed consent was obtained from the individual(s) for the publication of any potentially identifiable images or data included in this article.
Author contributions
KW and YY designed the study. KW, FX, and CJ analyzed the data. FX and CJ drafted the manuscript. KW, YY, FX, CJ, TZ, and RW revised the manuscript. All authors approved the final version of the manuscript.
Funding
This research was supported by the National Natural Science Foundation of China (32000760) to KW, China Postdoctoral Science Foundation Funded Project to KW (2019M662433), and Postdoctoral Innovation Project (239735) in Shandong Province to KW.
Conflict of interest
The authors declare that the research was conducted in the absence of any commercial or financial relationships that could be construed as a potential conflict of interest.
Publisher’s note
All claims expressed in this article are solely those of the authors and do not necessarily represent those of their affiliated organizations, or those of the publisher, the editors and the reviewers. Any product that may be evaluated in this article, or claim that may be made by its manufacturer, is not guaranteed or endorsed by the publisher.
Supplementary material
The Supplementary Material for this article can be found online at: https://www.frontiersin.org/articles/10.3389/fpsyt.2022.999384/full#supplementary-material
Footnotes
- ^ http://openfmri.org
- ^ https://web.stanford.edu/group/vista/cgi-bin/wiki/index.php/DTI_Preprocessing
- ^ https://github.com/yeatmanlab/AFQ/wiki
References
1. Poldrack R, Congdon E, Triplett W, Gorgolewski K, Karlsgodt K, Mumford J, et al. A phenome-wide examination of neural and cognitive function. Sci Data. (2016) 3:160110. doi: 10.1038/sdata.2016.110
2. Pettersson E, Lichtenstein P, Larsson H, Song J, Agrawal A, Børglum A, et al. Genetic influences on eight psychiatric disorders based on family data of 4 408 646 full and half-siblings, and genetic data of 333 748 cases and controls. Psychol Med. (2019) 49:1166–73.
3. Gandal M, Haney J, Parikshak N, Leppa V, Ramaswami G, Hartl C, et al. Shared molecular neuropathology across major psychiatric disorders parallels polygenic overlap. Science. (2018) 359:693–7.
4. Cao H. Prefrontal-cerebellar dynamics during post-success and post-error cognitive controls in major psychiatric disorders. Psychol Med. (2022) 1–8. doi: 10.1017/S0033291722001829
5. Barron D, Gao S, Dadashkarimi J, Greene A, Spann M, Noble S, et al. Transdiagnostic, connectome-based prediction of memory constructs across psychiatric disorders. Cereb Cortex. (2021) 31:2523–33. doi: 10.1093/cercor/bhaa371
6. Dong D, Wang Y, Chang X, Luo C, Yao D. Dysfunction of large-scale brain networks in schizophrenia: a meta-analysis of resting-state functional connectivity. Schizophr Bull. (2018) 44:168–81. doi: 10.1093/schbul/sbx034
7. McIntosh A, Job D, Moorhead T, Harrison L, Lawrie S, Johnstone E. White matter density in patients with schizophrenia, bipolar disorder and their unaffected relatives. Biol Psychiatry. (2005) 58:254–7. doi: 10.1016/j.biopsych.2005.03.044
8. Adler C, Holland S, Schmithorst V, Wilke M, Weiss K, Pan H, et al. Abnormal frontal white matter tracts in bipolar disorder: a diffusion tensor imaging study. Bipolar Disord. (2004) 6:197–203. doi: 10.1111/j.1399-5618.2004.00108.x
9. Beyer J, Taylor W, MacFall J, Kuchibhatla M, Payne M, Provenzale J, et al. Cortical white matter microstructural abnormalities in bipolar disorder. Neuropsychopharmacology. (2005) 30:2225–9. doi: 10.1038/sj.npp.1300802
10. Bruno S, Cercignani M, Ron M. White matter abnormalities in bipolar disorder: a voxel-based diffusion tensor imaging study. Bipolar Disord. (2008) 10:460–8. doi: 10.1111/j.1399-5618.2007.00552.x
11. Pavuluri M, Yang S, Kamineni K, Passarotti A, Srinivasan G, Harral E, et al. Diffusion tensor imaging study of white matter fiber tracts in pediatric bipolar disorder and attention-deficit/hyperactivity disorder. Biol Psychiatry. (2009) 65:586–93. doi: 10.1016/j.biopsych.2008.10.015
12. Okugawa G, Sedvall G, Agartz I. Reduced grey and white matter volumes in the temporal lobe of male patients with chronic schizophrenia. Eur Arch Psychiatry Clin Neurosci. (2002) 252:120–3. doi: 10.1007/s00406-002-0370-9
13. Friston K, Brown H, Siemerkus J, Stephan K. The dysconnection hypothesis (2016). Schizophr Res. (2016) 176:83–94. doi: 10.1016/j.schres.2016.07.014
14. Brandl F, Avram M, Weise B, Shang J, Simões B, Bertram T, et al. Specific substantial dysconnectivity in schizophrenia: a transdiagnostic multimodal meta-analysis of resting-state functional and structural magnetic resonance imaging studies. Biol Psychiatry. (2019) 85:573–83. doi: 10.1016/j.biopsych.2018.12.003
15. Kelly S, Jahanshad N, Zalesky A, Kochunov P, Agartz I, Alloza C, et al. Widespread white matter microstructural differences in schizophrenia across 4322 individuals: results from the ENIGMA schizophrenia DTI working group. Mol Psychiatry. (2018) 23:1261–9.
16. Dong D, Wang Y, Chang X, Jiang Y, Klugah-Brown B, Luo C, et al. Shared abnormality of white matter integrity in schizophrenia and bipolar disorder: a comparative voxel-based meta-analysis. Schizophr Res. (2017) 185:41–50. doi: 10.1016/j.schres.2017.01.005
17. Joo S, Kim H, Jo Y, Ahn S, Choi Y, Park S, et al. White matter impairments in patients with schizophrenia: a multisite diffusion MRI study. Prog Neuropsychopharmacol Biol Psychiatry. (2021) 111:110381. doi: 10.1016/j.pnpbp.2021.110381
18. Vitolo E, Tatu M, Pignolo C, Cauda F, Costa T, Ando A, et al. White matter and schizophrenia: a meta-analysis of voxel-based morphometry and diffusion tensor imaging studies. Psychiatry Res Neuroimaging. (2017) 270:8–21. doi: 10.1016/j.pscychresns.2017.09.014
19. Nakajima R, Kinoshita M, Shinohara H, Nakada M. The superior longitudinal fascicle: reconsidering the fronto-parietal neural network based on anatomy and function. Brain Imaging Behav. (2020) 14:2817–30. doi: 10.1007/s11682-019-00187-4
20. Bloemen O, de Koning M, Schmitz N, Nieman D, Becker H, de Haan L, et al. White-matter markers for psychosis in a prospective ultra-high-risk cohort. Psychol Med. (2010) 40:1297–304. doi: 10.1017/S0033291709991711
21. Carletti F, Woolley J, Bhattacharyya S, Perez-Iglesias R, Fusar Poli P, Valmaggia L, et al. Alterations in white matter evident before the onset of psychosis. Schizophr Bull. (2012) 38:1170–9. doi: 10.1093/schbul/sbs053
22. Karlsgodt K, Niendam T, Bearden C, Cannon T. White matter integrity and prediction of social and role functioning in subjects at ultra-high risk for psychosis. Biol Psychiatry. (2009) 66:562–9. doi: 10.1016/j.biopsych.2009.03.013
23. Krakauer K, Nordentoft M, Glenthøj B, Raghava J, Nordholm D, Randers L, et al. White matter maturation during 12 months in individuals at ultra-high-risk for psychosis. Acta Psychiatr Scand. (2018) 137:65–78. doi: 10.1111/acps.12835
24. Kristensen T, Mandl R, Raghava J, Jessen K, Jepsen J, Fagerlund B, et al. Widespread higher fractional anisotropy associates to better cognitive functions in individuals at ultra-high risk for psychosis. Hum Brain Mapp. (2019) 40:5185–201. doi: 10.1002/hbm.24765
25. Wang C, Ji F, Hong Z, Poh J, Krishnan R, Lee J, et al. Disrupted salience network functional connectivity and white-matter microstructure in persons at risk for psychosis: findings from the LYRIKS study. Psychol Med. (2016) 46:2771–83. doi: 10.1017/S0033291716001410
26. Diaz A, Fernandes B, Teixeira A, Mwangi B, Hasan K, Wu M, et al. White matter microstructure associated with anhedonia among individuals with bipolar disorders and high-risk for bipolar disorders. J Affect Disord. (2022) 300:91–8. doi: 10.1016/j.jad.2021.12.037
27. Mamah D, Ji A, Rutlin J, Shimony J. White matter integrity in schizophrenia and bipolar disorder: tract- and voxel-based analyses of diffusion data from the Connectom scanner. Neuroimage Clin. (2019) 21:101649. doi: 10.1016/j.nicl.2018.101649
28. Squarcina L, Bellani M, Rossetti M, Perlini C, Delvecchio G, Dusi N, et al. Similar white matter changes in schizophrenia and bipolar disorder: a tract-based spatial statistics study. PLoS One. (2017) 12:e0178089. doi: 10.1371/journal.pone.0178089
29. Albajara Sáenz A, Villemonteix T, Massat I. Structural and functional neuroimaging in attention-deficit/hyperactivity disorder. Dev Med Child Neurol. (2019) 61:399–405. doi: 10.1111/dmcn.14050
30. Hyde C, Sciberras E, Efron D, Fuelscher I, Silk T. Reduced fine motor competence in children with ADHD is associated with atypical microstructural organization within the superior longitudinal fasciculus. Brain Imaging Behav. (2021) 15:727–37. doi: 10.1007/s11682-020-00280-z
31. Wu W, McAnulty G, Hamoda H, Sarill K, Karmacharya S, Gagoski B, et al. Detecting microstructural white matter abnormalities of frontal pathways in children with ADHD using advanced diffusion models. Brain Imaging Behav. (2020) 14:981–97. doi: 10.1007/s11682-019-00108-5
32. van Ewijk H, Heslenfeld D, Zwiers M, Faraone S, Luman M, Hartman C, et al. Different mechanisms of white matter abnormalities in attention-deficit/hyperactivity disorder: a diffusion tensor imaging study. J Am Acad Child Adolesc Psychiatry. (2014) 53:790–9.e3. doi: 10.1016/j.jaac.2014.05.001
33. Wu Z, Bralten J, Cao Q, Hoogman M, Zwiers M, An L, et al. White matter microstructural alterations in children with ADHD: categorical and dimensional perspectives. Neuropsychopharmacology. (2017) 42:572–80. doi: 10.1038/npp.2016.223
34. Cortese S, Imperati D, Zhou J, Proal E, Klein R, Mannuzza S, et al. White matter alterations at 33-years follow-up in adults with childhood attention-deficit/hyperactivity disorder. Biol Psychiatry. (2013) 74:591–8. doi: 10.1016/j.biopsych.2013.02.025
35. Makris N, Buka S, Biederman J, Papadimitriou G, Hodge S, Valera E, et al. Attention and executive systems abnormalities in adults with childhood ADHD: a DT-MRI study of connections. Cereb Cortex. (2008) 18:1210–20. doi: 10.1093/cercor/bhm156
36. Silk T, Vance A, Rinehart N, Bradshaw J, Cunnington R. White-matter abnormalities in attention deficit hyperactivity disorder: a diffusion tensor imaging study. Hum Brain Mapp. (2009) 30:2757–65. doi: 10.1002/hbm.20703
37. Versace A, Jones N, Joseph H, Lindstrom R, Wilson T, Lima Santos J, et al. White matter abnormalities associated with ADHD outcomes in adulthood. Mol Psychiatry. (2021) 26:6655–65. doi: 10.1038/s41380-021-01153-7
38. Onnink A, Zwiers M, Hoogman M, Mostert J, Dammers J, Kan C, et al. Deviant white matter structure in adults with attention-deficit/hyperactivity disorder points to aberrant myelination and affects neuropsychological performance. Prog Neuropsychopharmacol Biol Psychiatry. (2015) 63:14–22. doi: 10.1016/j.pnpbp.2015.04.008
39. Davenport N, Karatekin C, White T, Lim K. Differential fractional anisotropy abnormalities in adolescents with ADHD or schizophrenia. Psychiatry Res Neuroimaging. (2010) 181:193–8. doi: 10.1016/j.pscychresns.2009.10.012
40. Chiang H, Hsu Y, Shang C, Tseng W, Gau S. White matter endophenotype candidates for ADHD: a diffusion imaging tractography study with sibling design. Psychol Med. (2020) 50:1203–13. doi: 10.1017/S0033291719001120
41. Zhao Y, Yang L, Gong G, Cao Q, Liu J. Identify aberrant white matter microstructure in ASD, ADHD and other neurodevelopmental disorders: a meta-analysis of diffusion tensor imaging studies. Prog Neuropsychopharmacol Biol Psychiatry. (2022) 113:110477. doi: 10.1016/j.pnpbp.2021.110477
42. Yeatman J, Richie-Halford A, Smith J, Keshavan A, Rokem A. A browser-based tool for visualization and analysis of diffusion MRI data. Nat Commun. (2018) 9:940. doi: 10.1038/s41467-018-03297-7
43. Versace A, Graur S, Greenberg T, Lima Santos J, Chase H, Bonar L, et al. Reduced focal fiber collinearity in the cingulum bundle in adults with obsessive-compulsive disorder. Neuropsychopharmacology. (2019) 44:1182–8. doi: 10.1038/s41386-019-0353-4
44. Zhang X, Sun Y, Li W, Liu B, Wu W, Zhao H, et al. Characterization of white matter changes along fibers by automated fiber quantification in the early stages of Alzheimer’s disease. Neuroimage Clin. (2019) 22:101723. doi: 10.1016/j.nicl.2019.101723
45. Sun H, Lui S, Yao L, Deng W, Xiao Y, Zhang W, et al. Two Patterns of white matter abnormalities in medication-naive patients with first-episode schizophrenia revealed by diffusion tensor imaging and cluster analysis. JAMA Psychiatry. (2015) 72:678–86. doi: 10.1001/jamapsychiatry.2015.0505
46. Deng F, Wang Y, Huang H, Niu M, Zhong S, Zhao L, et al. Abnormal segments of right uncinate fasciculus and left anterior thalamic radiation in major and bipolar depression. Prog Neuropsychopharmacol Biol Psychiatry. (2018) 81:340–9. doi: 10.1016/j.pnpbp.2017.09.006
47. Yeatman J, Dougherty R, Myall N, Wandell B, Feldman H. Tract profiles of white matter properties: automating fiber-tract quantification. PLoS One. (2012) 7:e49790. doi: 10.1371/journal.pone.0049790
48. Wakana S, Caprihan A, Panzenboeck M, Fallon J, Perry M, Gollub R, et al. Reproducibility of quantitative tractography methods applied to cerebral white matter. Neuroimage. (2007) 36:630–44. doi: 10.1016/j.neuroimage.2007.02.049
49. Hua K, Zhang J, Wakana S, Jiang H, Li X, Reich D, et al. Tract probability maps in stereotaxic spaces: analyses of white matter anatomy and tract-specific quantification. Neuroimage. (2008) 39:336–47. doi: 10.1016/j.neuroimage.2007.07.053
50. Jones D, Catani M, Pierpaoli C, Reeves S, Shergill S, O’Sullivan M, et al. Age effects on diffusion tensor magnetic resonance imaging tractography measures of frontal cortex connections in schizophrenia. Hum Brain Mapp. (2006) 27:230–8. doi: 10.1002/hbm.20179
51. Rowland L, Spieker E, Francis A, Barker P, Carpenter W, Buchanan R. White matter alterations in deficit schizophrenia. Neuropsychopharmacology. (2009) 34:1514–22. doi: 10.1038/npp.2008.207
52. Karlsgodt K, van Erp T, Poldrack R, Bearden C, Nuechterlein K, Cannon T. Diffusion tensor imaging of the superior longitudinal fasciculus and working memory in recent-onset schizophrenia. Biol Psychiatry. (2008) 63:512–8. doi: 10.1016/j.biopsych.2007.06.017
53. Szeszko P, Tan E, Uluğ A, Kingsley P, Gallego J, Rhindress K, et al. Investigation of superior longitudinal fasciculus fiber complexity in recent onset psychosis. Prog Neuropsychopharmacol Biol Psychiatry. (2018) 81:114–21. doi: 10.1016/j.pnpbp.2017.10.019
54. Makris N, Biederman J, Monuteaux M, Seidman L. Toward conceptualizing a neural systems-based anatomy of attention-deficit/hyperactivity disorder. Dev Neurosci. (2009) 31:36–49. doi: 10.1159/000207492
55. Simonetti A, Kurian S, Saxena J, Verrico C, Restaino A, Di Nicola M, et al. Cortical correlates of impulsive aggressive behavior in pediatric bipolar disorder. Front Psychiatry. (2021) 12:674707. doi: 10.3389/fpsyt.2021.674707
Keywords: schizophrenia, bipolar disorder, ADHD, superior longitudinal fasciculus, automated fiber quantification
Citation: Xu F, Jin C, Zuo T, Wang R, Yang Y and Wang K (2022) Segmental abnormalities of superior longitudinal fasciculus microstructure in patients with schizophrenia, bipolar disorder, and attention-deficit/hyperactivity disorder: An automated fiber quantification tractography study. Front. Psychiatry 13:999384. doi: 10.3389/fpsyt.2022.999384
Received: 21 July 2022; Accepted: 21 November 2022;
Published: 06 December 2022.
Edited by:
Jeffrey A. Stanley, Wayne State University, United StatesReviewed by:
Piotr Podwalski, Pomeranian Medical University, PolandYuyang Luo, Massachusetts Eye and Ear Infirmary and Harvard Medical School, United States
Copyright © 2022 Xu, Jin, Zuo, Wang, Yang and Wang. This is an open-access article distributed under the terms of the Creative Commons Attribution License (CC BY). The use, distribution or reproduction in other forums is permitted, provided the original author(s) and the copyright owner(s) are credited and that the original publication in this journal is cited, in accordance with accepted academic practice. No use, distribution or reproduction is permitted which does not comply with these terms.
*Correspondence: Ying Yang, doctoryy2022@163.com; Kangcheng Wang, wangkangcheng@sdnu.edu.cn
†These authors have contributed equally to this work