- 1Department of Child & Adolescent Psychiatry, Institute of Psychiatry, Psychology and Neuroscience, King’s College London, London, United Kingdom
- 2Division of Population and Behavioral Science, University of St Andrews School of Medicine, St Andrews, United Kingdom
Introduction: The literature suggests that tobacco smoking may have a neurotoxic effect on the developing adolescent brain. Particularly, it may impair the decision-making process of early-onset smokers (<16 years), by rendering them more prone to impulsive and risky choices toward rewards, and therefore more prone to smoking relapses, in comparison to late-onset smokers (≥16 years). However, no study has ever investigated reward-based decision-making and structural brain differences between early-onset smokers and late-onset smokers.
Methods: Computerized measures of reward-based decision-making [Cambridge Gambling Task (CGT); 5-trials adjusting delay discounting task (ADT-5)] were administered to 11 early-onset smokers (mean age at regular smoking initiation = 13.2 years), 17 late-onset smokers (mean age at regular smoking initiation = 18.0 years), and 24 non-smoker controls. Voxel-based morphometry (VBM) was utilized to investigate the gray matter (GM) and white matter (WM) volume differences in fronto-cortical and striatal brain regions between early-onset smokers, late-onset smokers, and non-smokers.
Results: Early-onset smokers displayed a riskier decision-making behavior in comparison to non-smokers as assessed by the CGT (p < 0.01, Cohen’s f = 0.48). However, no significant differences (p > 0.05) in reward-based decision-making were detected between early-onset smokers and late-onset smokers. VBM results revealed early-onset smokers to present lower GM volume in the bilateral anterior insular cortex (AI) in comparison to late-onset smokers and lower WM volume in the right AI in comparison to late-onset smokers.
Conclusion: Impairments in reward-based decision-making may not be affected by tobacco smoking initiation during early adolescence. Instead, lower GM and WM volume in the AI of early-onset smokers may underline a vulnerability to develop compulsive tobacco seeking and smoking behavior during adulthood.
Introduction
The World Health Organization (WHO) defines tobacco smoking as the “leading cause of preventable death worldwide.” In fact, it is responsible for the death of approximately 8 million people each year (1). Common chronic and potentially lethal mortal diseases caused by chronic tobacco smoking include cardiopulmonary (e.g., obstructive pulmonary disease) and cerebrovascular (e.g., strokes) conditions, in addition to lung and throat cancers (2).
It is well-known that 90% of adult smokers start regular tobacco use before 21 years of age (3–6). Epidemiological studies revealed a relationship between smoking onset during early adolescence (10–15 years) and the development of higher levels of nicotine dependence compared to smoking onset during subsequent developmental stages of adolescence (7). An international longitudinal study design conducted by Hu et al. (8) revealed a significant negative correlation (p < 0.001) between tobacco smoking onset during early adolescence, smoking heaviness, and greater difficulties in quitting smoking during adulthood in 6,684 participants recruited from Australia, the US, and Finland. A longitudinal study conducted on 244 monozygotic twin pairs by Kendler et al. (9) revealed that smokers who started this maladaptive behavior at 14.6 years report higher levels of nicotine dependence and more intense craving for cigarettes compared to twins who started smoking during late adolescence (19.1 years). Another longitudinal study conducted by Paul et al. (10) on 6,559 Australian early adolescents revealed that smoking experimentation at 14–15 years of age increased significantly (p < 0.01) the risk of being a chronic smoker 20 years after baseline measurements (men, RR 2.72, 95% confidence interval [CI] 1.74–4.25; women, RR 6.39, 95% CI 2.85–14.33). The risk was higher for early adolescents who experimented with 10 or more cigarettes compared to those who experimented with less than 10 cigarettes (10). While there is consensus about the biopsychosocial factors (e.g., peer pressure, social learning, social deprivation, family members who smoke, family conflict, comorbid psychiatric illness, slow development of frontal brain regions) associated with tobacco smoking initiation during early adolescence [e.g., (5, 11–13)], there is still uncertainty, however, about the relationship between smoking onset during early adolescence and the development of chronic smoking during adulthood (8).
Animal models suggest that the above association may be due to the unique neurostructural and neurochemical alterations caused by nicotine on the developing adolescent brain [for reviews see (14–17)]. Briefly, nicotine exposure during adolescence has been shown to (a) cause an extensive upregulation of nicotinic acetylcholine receptors (nAChRs) and reduce the functioning of metabotropic glutamate type 2 receptors (mGluR2) in the PFC, to (b) influence the expression of genes involved in the neuroplasticity of frontal brain regions, and to (c) cause changes in macromolecular constituents indicative of cell loss (reduced DNA) and altered cell size (protein/DNA ratio) in the cerebral cortex, midbrain, and hippocampus of adolescent rodents (17–19). According to Del Ciampo and Del Ciampo (20), “nicotine use during adolescence has been associated with deleterious effects on development in the prefrontal cortex and hippocampal structure and can lead to irreversible decreased cognitive function, mainly attention, memory and hyperactivity, and severe addiction” (p. 1). Indeed, cognitive impairments such as increased impulsivity and decreased attention have been observed in adolescent rodents after nicotine exposure but not in adult rodents [e.g., (21)]. Heavy metals present in tobacco cigarettes (e.g., lead, arsenic, cadmium) have been also found to disrupt the formation of neural circuits in children and adolescents, and to cause cognitive impairments such as inattention, lower IQ, and learning disabilities (22–24).
In accordance with the above evidence, DeBry and Tiffany (25) proposed the “tobacco- induced neurotoxicity theory of adolescent cognitive development” (TINACD). According to the TINACD, the earlier the initiation of tobacco smoking, the greater the likelihood of suboptimal executive functioning and poor management of impulsivity would be in adult smokers (25). Particularly, deficits in brain areas associated with impulse control and decision-making such as the ventromedial prefrontal cortex (VMPFC), ventrolateral prefrontal cortex (VLPFC), orbitofrontal cortex (OFC), dorsolateral prefrontal cortex (DLPFC), and anterior cingulate cortex (ACC) should cause a poor modulation of reward-driven responses, therefore, prompting smoking relapses during quit attempts (25).
Only a handful of studies have been conducted investigating the association between early smoking initiation during adolescence and neurocognitive impairments during adulthood in chronic tobacco smokers. A study conducted by Mashhoon et al. (7) showed 10 early-onset smokers (mean age at regular smoking onset = 13.2 years) to suffer impairments in response inhibition and attentional performance, as assessed by a GO/No GO task, compared to 10 late-onset smokers (mean age at regular smoking onset = 17.7 years) and 10 non-smokers. A previous study conducted by Jacobsen et al. (26) revealed 42 chronic smokers to suffer impairments in working memory, as assessed by an auditory n-back task, in comparison to 31 non-smokers. A positive correlation was also found between earlier age at smoking initiation (mean age at regular smoking onset = 13.1 years) and performance accuracy on the working memory n-back task. Therefore, indicating “that the performance of subjects who began to smoke at older ages was less impaired than that of subjects who began to smoke at younger ages” [(26), p. 63]. An fMRI study conducted by Galván et al. (27) did not report any response inhibition differences, as assessed by a stop-signal task, between 25 late adolescent smokers (mean age = 19 years) and 25 age-matched non-smoker controls. However, a negative correlation was detected between adolescent smokers’ scores on the Heaviness of Smoking Index (a measure of smoking behavior and dependence during adolescence) and PFC cortical activation during the stop-signal task. Another study (28) reported a correlation between risk-sensitivity, as assessed by the Balloon Analog Risk Task, and neural activation in dorsolateral and ventrolateral prefrontal cortices in 18 adolescent smokers.
Findings from the above studies indicate that regular smoking initiation occurring during the earliest neuro-maturational stages of adolescence may impact negatively cognitive functions, such as working memory, attention, and response inhibition. Furthermore, adolescent smoking behavior may modulate the neural activation in prefrontal brain areas associated with response inhibition and risky decision-making.
Notably, a meta-analysis conducted by Conti et al. (29) revealed that adult chronic smokers suffer mild memory and attentional impairments in comparison to non-smokers. No differences in motor impulsivity/response inhibition were identified between chronic smokers and non-smokers (SMD = 0.105, p = 0.24). Moreover, cognitive impulsivity was found to be the most impaired (SMD: 0.881, p < 0.005) neurocognitive domain.
Cognitive impulsivity may be defined as “the inability to weigh the consequences of immediate and future events and, consequently, delay gratification” (30). It is characterized by an aberrant reward-based decision-making process, including choice impulsivity (a.k.a delay discounting) and risky decision-making, and has been consistently associated with smoking initiation and maintenance (i.e., relapse) by the literature [e.g., (31, 32)]. However, to the best of our knowledge, no study has ever investigated the possible impact of early smoking initiation on reward-based decision-making impairments (impulsive and risky choices) and their neuroanatomical correlates [gray matter (GM) and white matter (WM) volume] in adult chronic smokers.
Therefore, the aim of the current study was to test the following hypotheses:
1. Early-onset smokers (<16 years) show heightened impulsive and risky choices compared to late-onset smokers (≥16 years) and non-smokers.
2. Early-onset smokers (<16 years) present lower GM and WM volume in brain regions commonly associated with impaired reward-based decision-making (henceforth defined as a priori regions of interest) compared to late-onset smokers (≥16 years) and non-smokers. A priori regions of interest were determined based on previous studies measuring GM and WM volume differences in chronic smokers compared to non-smokers and on previous studies investigating the relationship between structural brain deficits and impaired reward-based decision-making in chronic smokers [e.g., (33–39)]. Regions of interest included: VLPFC, lOFC, DLPFC, DMPFC, VMPFC, mOFC, ACC, insula, dorsal, and ventral striatum (including adjacent white matter tracts), and anterior corpus callosum.
3. GM and WM volume in a priori regions of interest of early-onset smokers (<16 years) is correlated with the heightened risky and impulsive choices manifested by the same early-onset smokers in comparison to late-onset smokers (≥16 years) and non-smokers.
Materials and methods
Ethical and other research governance approvals
Ethical approval was granted by the London Bromley Research Ethics Committee (REC) (REC Reference Number: 19/LO/1176) and by the University of St. Andrews Teaching and Research Ethics Committee (UTREC) (UTREC Approval Code: MD14516). Research governance and management approvals were granted by the Tayside National Health System (NHS) R&D department.
Recruitment
The recruitment procedures are described in our previous study (35). Briefly, chronic smokers and non-smokers have recruited between October 2019 and March 2020 across the south-eastern regions of Scotland through convenience sampling (e.g., internet and newspaper advertisements, word of mouth). Participants needed to attend a screening session (session 1) at the University of St. Andrews School of Medicine and an experimental session (session 2) at Ninewells Hospital, Dundee, on a separate day. During session 1, participants underwent screening procedures and performed computerized measures of reward-based decision-making. During session 2, participants underwent a structural magnetic resonance imaging (MRI) procedure. No more than 3 days were allowed to occur between the two sessions.
All participants provided written informed consent prior to the beginning of the study. They were rewarded £100 for their full participation.
Inclusion and exclusion criteria
Inclusion and exclusion criteria for participants are shown in Table 1. Objective and subjective screening measures are described in detail in our previous study (35). In summary, the smoking status of participants (non-smoker vs. chronic smoker) was verified through a carbon monoxide (CO) breath test and a salivary cotinine test. Smokers needed to present a CO level ≥ 1 ppm and a salivary cotinine ≥ 2 ng/ml to be included in the study. The presence of illicit substances (e.g., heroin, methamphetamine, cocaine) in the system of the participants was investigated through urine drug analysis. Participants who were found positive for illicit substances were excluded from the study, except occasional cannabis users. In fact, previous research reported a weak association between occasional cannabis smoking and impaired reward-based decision-making, and between occasional cannabis smoking and structural brain abnormalities (40, 41). Additionally, a recent meta-analysis conducted by Lorenzetti et al. (42) did not reveal any GM and WM volume reductions in adolescent cannabis users in comparison to non-cannabis users. In the current study, occasional cannabis use was defined as smoking cannabis two or less times per week. The Mini International Neuropsychiatric Instrument (MINI) version 7.0.2 was utilized to exclude the presence of DSM-V psychiatric disorders (Axis I).
Chronic smokers were classified into subgroups: early-onset smokers (age at regular smoking onset < 16 years) and late-onset smokers (age at regular smoking onset ≥ 16 years). According to Mashhoon et al. (7), “this age cut-off has been used consistently in substance abuse research as a dividing mark for early- vs. late-onset drug use [e.g., (43–49)]” (p. 48). Age at regular smoking onset was defined as the age at which participants started smoking ≥ 5 tobacco cigarettes per day. Age at regular smoking onset, number of cigarettes smoked daily, units of alcohol consumed per day, and weekly use of cannabis and tobacco were assessed through a screening interview. Smoker participants needed to smoke ≥ 10 cigarettes per day for 2 or more years to be included in the study. Pack-years (a clinical measure of lifetime tobacco smoking exposure) were calculated by the following formula:
The severity of nicotine dependence was instead assessed by the Fagerström Test for Nicotine Dependence (FTND) (50). The pre-morbid intelligence quotient (QI) of participants was estimated through the Barona equations (51).
Instruments
Reward-based decision-making outcome measures
Computerized measures of reward-based decision-making included the 5-trials adjusting delay discounting task (ADT-5) (52) for impulsive choices and the Cambridge Gambling Task (CGT) (Cambridge Cognition, 2019) for risky choices. A detailed description of these tasks is reported in our previous study (35). Briefly, during the ADT-5, participants needed to choose between £5 available immediately or £10 available at some point in future (e.g., 1 week, 1 month) over five trials. Outcome measures consisted of effective delay 50% (ED50) values computed at the end of the task. As stated by Yoon and Higgins (53), “ED50 represents the delay that is effective in discounting the subjective value of the delayed reinforcer by 50%.” Consistently with the hypothesis proposing early-onset smokers display heightened impulsive choices compared to late-onset smokers and non-smokers, 10£ should have lost 50% of their value at a sooner time point for early-onset smokers compared to both non-smokers and late-onset smokers’ groups.
The CGT measures risky decision-making outside a learning context. It is administered through the Cambridge Neuropsychological Test Automated Battery.1 During this task, participants needed to identify a yellow token hidden inside a blue or red box. The ratio of blue and red boxes varied randomly across trials. Participants needed to bet on their decision by selecting a value presented within a circle at the center of an iPad screen. Each participant started with a score of 100 points, and for each correct guess, the value that was betted was added to their total score. On the contrary, the value that was betted was subtracted from their total score for each incorrect guess. Outcome measures for this task consisted of the different facets of the risky decision-making process that are usually impaired in substance users, such as “quality of decision-making,” “risk taking,” “overall proportion bet,” and “risk adjustment” (54–59).
Neuroimaging
Structural T1-weighted images were acquired through a Siemens 3T Prisma-FIT scanner (Siemens Healthineers, Erlangen, Germany). Images were acquired with a voxel size of 0.8 × 0.8 × 1.0 mm3 with whole-brain coverage, repetition time (TR) = 1.9 s, echo time (TE) = 2.64 ms. Flip angle = 9°, FOV = 200 mm, matrix = 256 × 256, 176 slices, slice thickness 1 mm. Scans were reported by a consultant radiologist to rule out the presence of incidental findings.
Statistical analysis
One-way ANOVAs were utilized to compare early-onset smokers, late-onset smokers, and non-smokers in relation to socio-demographic characteristics (age, social deprivation, pre-morbid IQ, and educational level), patterns of tobacco usage and dependence (cigarettes smoked per day, pack-years, FTND scores, age at regular smoking onset), and daily alcohol usage. Differences between groups in relation to the number of occasional cannabis users and biological sex were instead assessed through chi-squared (χ2) tests of associations.
ANCOVAs were conducted to test the null hypothesis of no difference between early-onset smokers, late-onset smokers, and non-smokers in relation to reward-based decision-making outcome measures. These included: ED50 scores of the ADT-5 task; risk taking, overall proportion bet, quality of decision-making, and risk adjustment scores of the CGT. Considering that statistically significant (p < 0.05) differences were identified between early-onset smokers and late-onset smokers in relation to biological sex, and between non-smokers, early-onset smokers, and late-onset smokers in relation to educational level and pre-morbid IQ (see Table 2), these socio-demographic variables were inserted as covariates in the ANCOVA analyses.
Assumptions for ANCOVAs included homogeneity of variances, homogeneity of regression slopes, and assumption of normality.
Assumptions of normality and homogeneity of variances were assessed by Shapiro–Wilk’s and Levene’s tests, respectively. Data that violated assumptions of normality and/or of homogeneity variances were log10 transformed. If data still failed assumptions of normality and/or of homogeneity of variances after log10 transformation, non-parametric Kruskal–Wallis H tests were conducted instead of ANCOVAs.
The software SPSS (version 28) was utilized to perform ANOVAs and ANCOVAs analyses. The significance threshold was set at p < 0.05. Bonferroni pairwise comparisons were employed to control for Type 1 errors. Effect sizes (Cohen’s f) were computed through the software G* Power.2
Voxel-based morphometry (VBM) was employed through the software SPM12 to analyze neuroimaging data.3 Pre-processing procedures were first conducted by segmenting T1 images into gray and white matter probability maps. Segmented images were subsequently normalized to the Montreal Neurological Institute (MNI) template and modulated to preserve the total amount of GM and WM in each probability map. The last pre-processing procedure consisted in smoothing the modulated images with an 8 mm Gaussian kernel (60). The Computational Anatomy Toolbox4 was utilized to extract the total intracranial volume (TIV) for each participant. Brain regions were identified by converting MNI coordinates into Talairach coordinates5 and by inserting the converted coordinates into the Talairach Daemon atlas.6
Whole-brain two-sample t-tests were employed to test for the null hypothesis of no differences between early-onset smokers and late-onset smokers, and between early-onset smokers and non-smokers, in relation to GM and WM volume in a priori regions of interest. Therefore, a total of four two-sample t-tests were conducted. Whole-brain voxel-wise linear regression models were employed to investigate the associations between outcome measures of reward-based decision-making (CGT and ADT-5 scores) and GM/WM volume in early-onset smokers. Brain-behavior associations were only investigated if early-onset smokers manifested heightened impulsive and/or risky choices in comparison to either late-onset smokers or non-smokers.
Exploratory analyses were also performed by computing whole-brain voxel-wise linear regression models investigating the associations between tobacco exposure variables (FTND scores, pack-years, number of cigarettes smoked per day) and GM/WM volume in early-onset smokers.
T-tests and voxel-wise linear regressions were conducted across the whole brain (but limited in scope to a priori regions of interest) by utilizing a stringent cluster-extent forming threshold of p < 0.01 with a minimum of 100 contiguous voxels per cluster (corrected for multiple comparisons by utilizing Monte-Carlo simulation) (35, 56, 61, 62). Biological sex, TIV, and age were inserted as covariates of no interest in all types of VBM analyses.
Results
Socio-demographic, smoking, and other substance use characteristics of the recruited population
The recruitment process is shown in Figure 1. Table 2 shows that the percentage of women participants was significantly (p < 0.05) higher in the early-onset smokers’ groups in comparison to both non-smokers and late-onset smokers’ groups. Non-smokers reported a higher level of education (p < 0.05) in comparison to early-onset smokers, but not to late-onset smokers (p > 0.05). Furthermore, non-smokers reported a higher pre-morbid IQ level compared to both early-onset smokers and late-onset smokers (p < 0.05). Moreover, non-smokers did not differ significantly (p > 0.05) from early-onset smokers and late-onset smokers in relation to age, Scottish Index Of Multiple Deprivation (SIMD), and units of alcohol consumed per day.
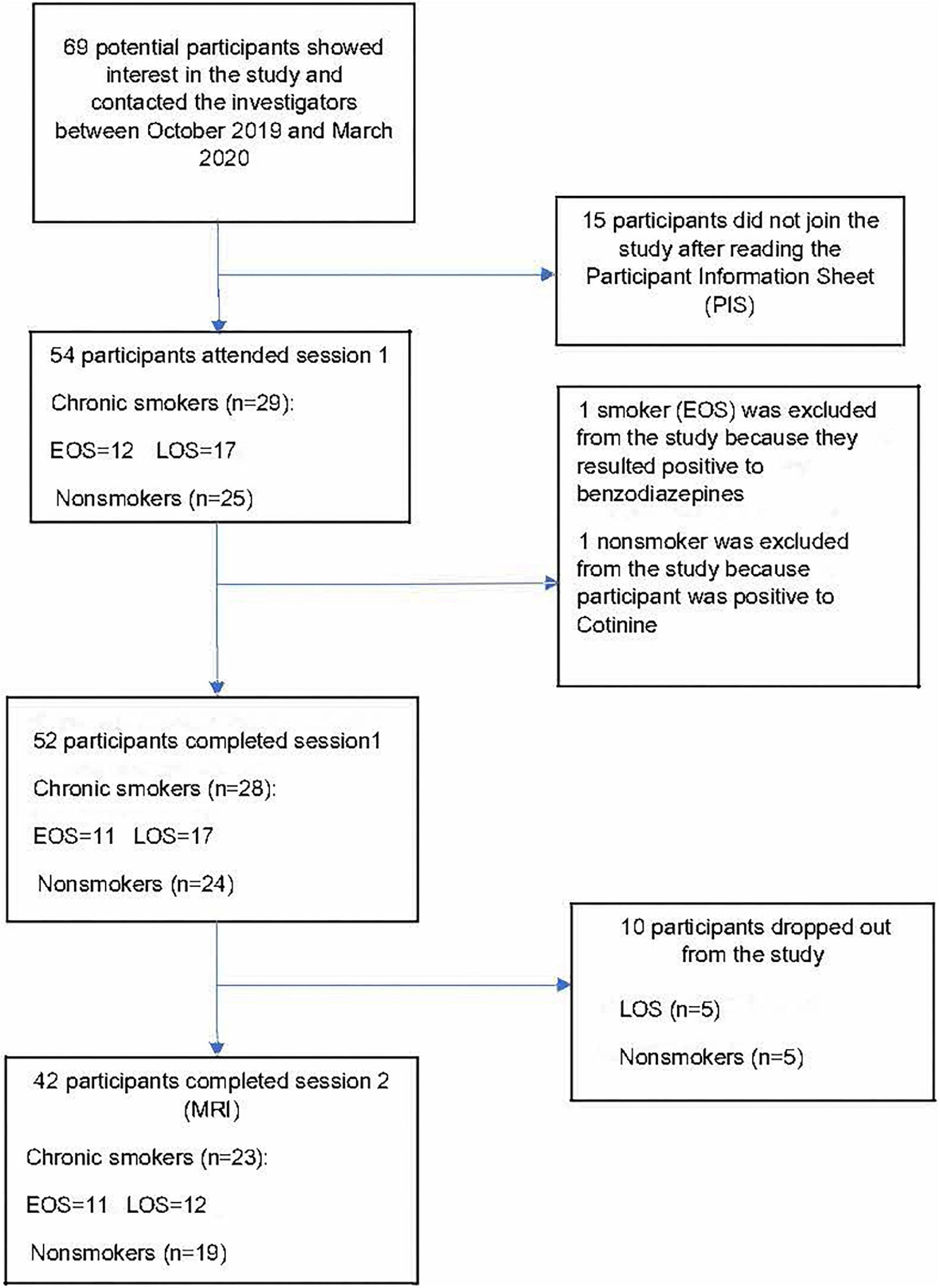
Figure 1. Recruitment flowchart between October 2019 and March 2020. EOS, early onset smokers; LOS, late onset smokers. Figure adapted from “Neuroanatomical correlates of impulsive choices and risky decision making in young chronic tobacco smokers: A voxel-based morphometry study” (35).
No statistically significant differences were detected between early-onset smokers and late-onset smokers in relation to the number of cigarettes smoked per day, pack-years, and FTND scores (p > 0.05). As expected, early-onset smokers differed significantly (p < 0.05) from late-onset smokers in relation to “age at regular smoking onset.” Particularly, early-onset smokers started regular smoking at a mean age of 13.2 years, while LOS at 18.0 years. No significant differences were detected between early-onset smokers and late-onset smokers in relation to the number of occasional cannabis smokers (p > 0.05). Two occasional cannabis smokers were present in the early-onset smokers’ group, while three occasional cannabis smokers were present in the late-onset smokers’ group. Considering the drop-out of 10 participants (five late-onset smokers, five non-smokers) prior to session 2, sensitivity analyses (ANOVAs) were conducted by just including participants who attended the MRI session. The only difference from socio-demographic comparisons conducted in session 1 consisted in that non-smokers presented a significantly higher (p < 0.05) educational level compared to both early-onset smokers and late-onset smokers subgroups. No other differences in results were detected between session 1 and session 2 for socio-demographic and smoking characteristic comparisons (see Table 2).
Reward-based decision-making outcome measures
ANCOVA analyses revealed significant main effects of group performance on the ADT-5 task (ED50 values), while controlling for biological sex, pre-morbid IQ, and level of education [F(2, 46) = 6.06, p = 0.005, partial η2 = 0.209, Cohen’s f = 0.51]. Pairwise comparisons conducted with Bonferroni adjustment revealed statistically significant (p = 0.005) higher ED50 values for non-smokers (M = 1.86; SE = 0.20) compared to late-onset smokers (M = 0.74; SE = 0.23) with a mean difference of 1.11 (95% CI, 0.29–1.94), but not for non-smokers compared to early-onset smokers (M = 0.99; SE = 0.28). Furthermore, no statistically significant differences (p > 0.05) were detected between early-onset smokers and late-onset smokers in relation to ED50 values.
Regarding risky decision-making, ANCOVA analyses revealed significant main effects of group performance on the CGT for “overall proportion bet” [F(2, 46) = 6.07, p = 0.005, partial η2 = 0.209, Cohen’s f = 0.51] and “risky taking” scores [F(2, 46) = 5.42, p = 0.008, partial η2 = 0.191, Cohen’s f = 0.48]. Pairwise comparisons conducted with Bonferroni adjustment revealed early-onset smokers (M = 0.67; SE = 0.05) to display significantly (p = 0.003) higher “overall proportion bet” scores in comparison to non-smokers (M = 0.42; SE = 0.03) with a mean difference of 0.24 (95% CI, 0.07–0.42), but not in comparison to late-onset smokers (M = 0.54; SE = 0.04). Similarly, early-onset smokers (M = 0.71; SE = 0.05) displayed significantly (p = 0.006) higher “risk-taking” scores in comparison to non-smokers (M = 0.47; SE = 0.04) with a mean difference of 0.24 (95% CI, 0.05–0.42), but not in comparison to late-onset smokers (M = 0.58; SE = 0.04). Pairwise comparisons did not reveal any statistically significant difference (p > 0.05) between non-smokers and late-onset smokers in relation to both “risk-taking” and “overall proportion bet” scores.
No main effects of group performance on CGT “risk adjustment” scores were detected [F(2, 46) = 1.74, p = 0.187, partial η2 = 0.070, Cohen’s f = 0.27]. Furthermore, the Kruskal–Wallis H tests results revealed that early-onset smokers and late-onset smokers did not differ significantly in relation to “quality of decision-making” scores [χ2(1) = 0.08, p = 0.76]. No statistically significant (p > 0.05) differences in relation to “quality of decision-making” scores were also detected between non-smokers and both early-onset smokers and late-onset smokers’ groups. Considering the drop-out of 10 participants (five late-onset smokers and five non-smokers) prior to session 2, sensitivity analyses (ANCOVAs) were conducted to ascertain differences in reward-based decision-making between the three groups of participants at the time of the MRI session. No differences in results were detected between session 1 and session 2. Impulsive choice and risky decision-making scores computed at both sessions are shown in Table 3.
Neuroimaging: Two-sample T-tests
Figures 2, 3 show that early-onset smokers displayed lower GM and WM volume compared to non-smokers in several a priori regions of interest. Particularly, early-onset smokers showed lower GM volume in the right ACC (16, 28, 24; p = 0.001; T = 3.38; k = 695; BA 32) and lower WM volume in the left anterior corpus callosum (-14, 27, 28; p = 0.003; T = 3.07; k = 149), bilateral thalamus (left thalamus: –3, 14, 12; p = 0.001; T = 3.49; k = 627) (right thalamus: 9, –18, 14; p = 0.004; T = 2.90; k = 627), and left anterior insula (AI) (-36, 30, 0; p = 0.001; T = 3.64; k = 154; BA 13) in comparison to non-smokers.
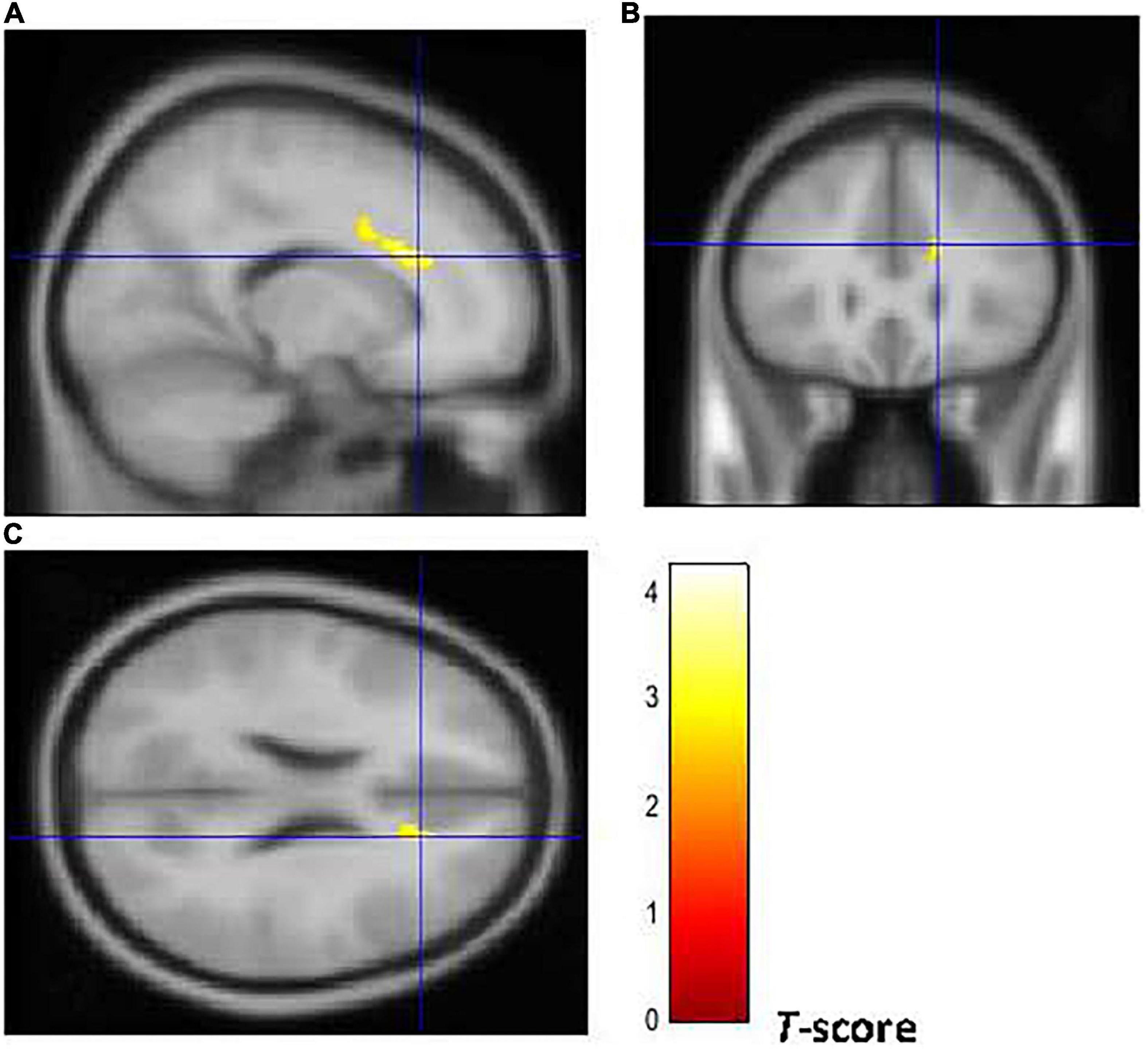
Figure 2. Brain regions of interest displaying lower GM volume in early onset smokers in comparison to non-smokers. The figure shows early onset smokers to display lower GM volume in the right ACC [region of interest centered at 16, 28, 24 MNI coordinates in sagittal (A), coronal (B), and axial (C) planes] in comparison to non-smokers. The cluster-forming threshold consisted in p < 0.01 with a minimum of 100 voxels per cluster at a whole-brain corrected level. TIV, age, and biological sex were inserted as covariates of no interest.
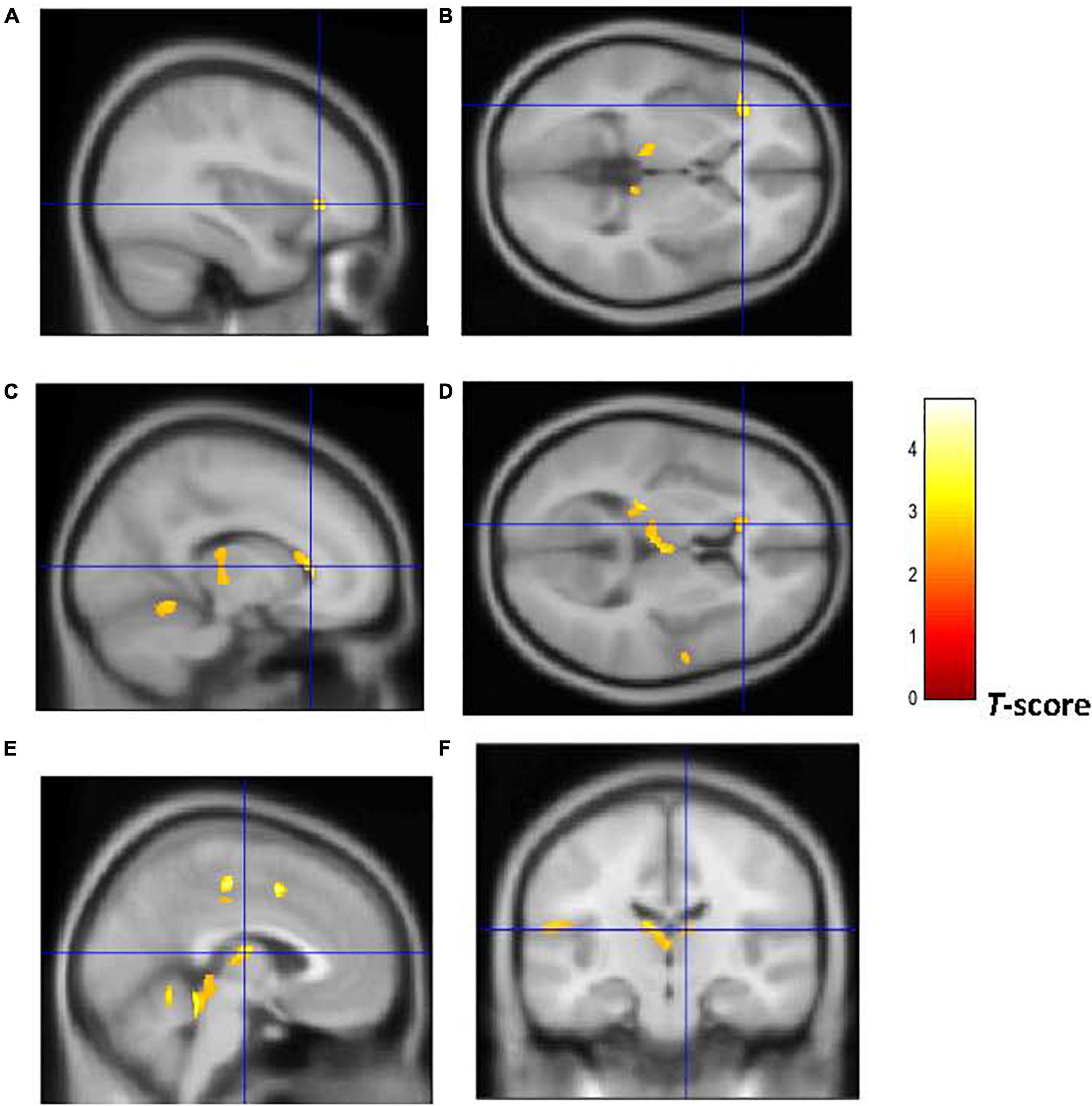
Figure 3. Brain regions of interest displaying lower WM volume in early onset smokers in comparison to non-smokers. The figure shows early onset smokers to display lower WM volume in the left AI [region of interest centered at –36, 30, 0 MNI coordinates in sagittal (A) and axial (B) planes]; left anterior corpus callosum [region of interest centered at –14, 27, 28 MNI coordinates in sagittal (C) and axial (D) planes]; and bilateral thalamus [region of interest centered at –3, –14, 12 MNI coordinates in sagittal (E) plane, and at 9, –18, 14 MNI coordinates in coronal plane (F)] in comparison to non-smokers. The cluster forming threshold consisted in p < 0.01 with a minimum of 100 contiguous voxels per cluster at a whole-brain corrected level. TIV, age, and biological sex were inserted as covariates of no interest.
Furthermore, as shown in Figures 4, 5, early-onset smokers displayed lower GM volume in the bilateral AI (right AI: 32, 15, –18; p = 0.000; T = 6.01; k = 5,428; BA 13) (left AI: –34, 14, –15; p = 0.000; T = 5.67; k = 948, BA13) and lower WM volume in the right AI (36, 18, –8; p = 0.000; T = 4.35; k = 508, BA13) in comparison to late-onset smokers.
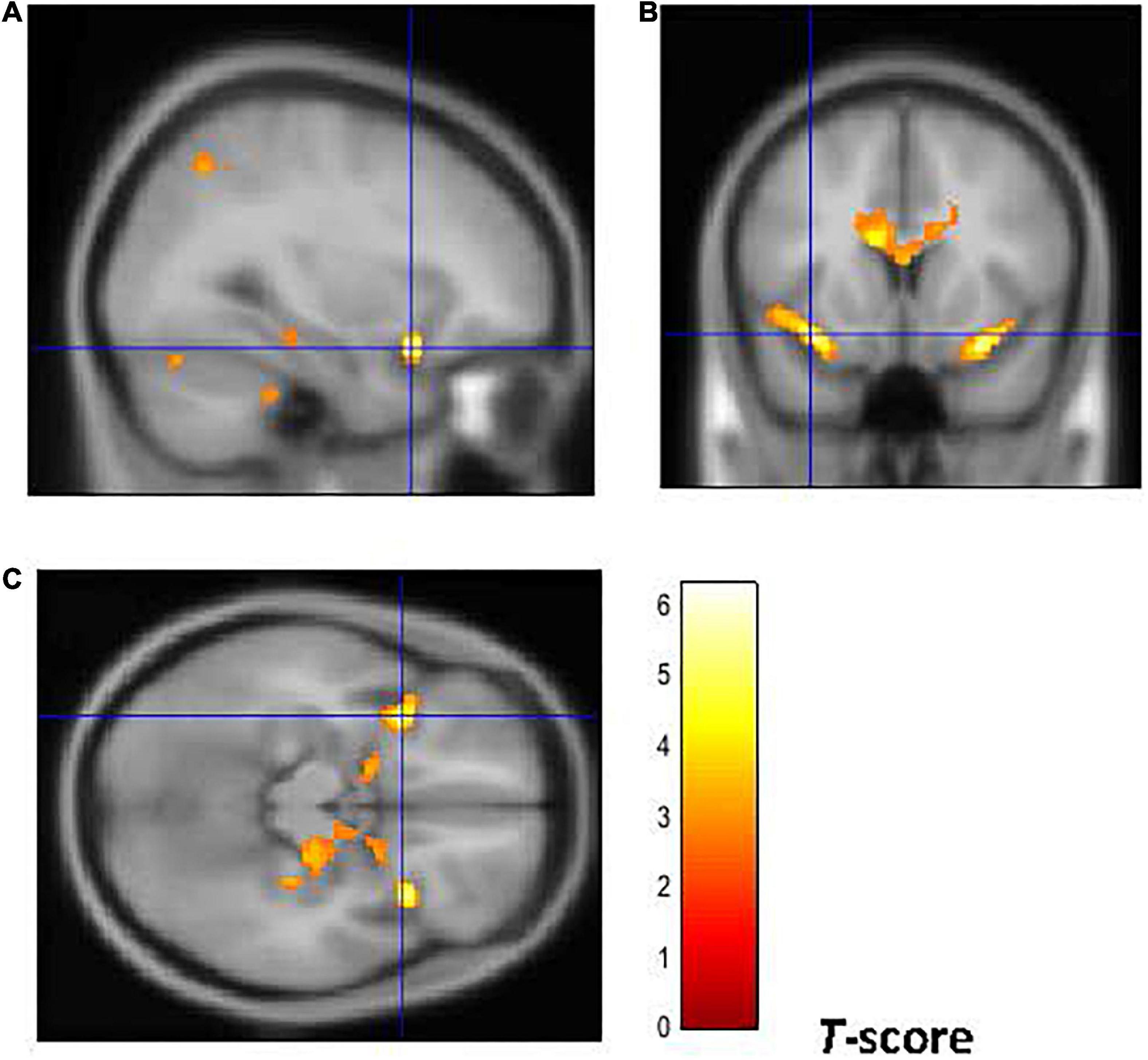
Figure 4. Brain regions of interest displaying lower GM volume in early onset smokers in comparison to late onset smokers. The figure shows early onset smokers to display lower GM volume in the bilateral AI [region of interest centered at 32, 15, –18 MNI coordinates in sagittal plane (A), and at –34, 14, –15 in coronal (B) and axial (C) planes] in comparison to late onset smokers. The cluster forming threshold consisted in p < 0.01 with a minimum of 100 contiguous voxels per cluster at a whole-brain corrected level. TIV, age, and biological sex were inserted as covariates of no interest.
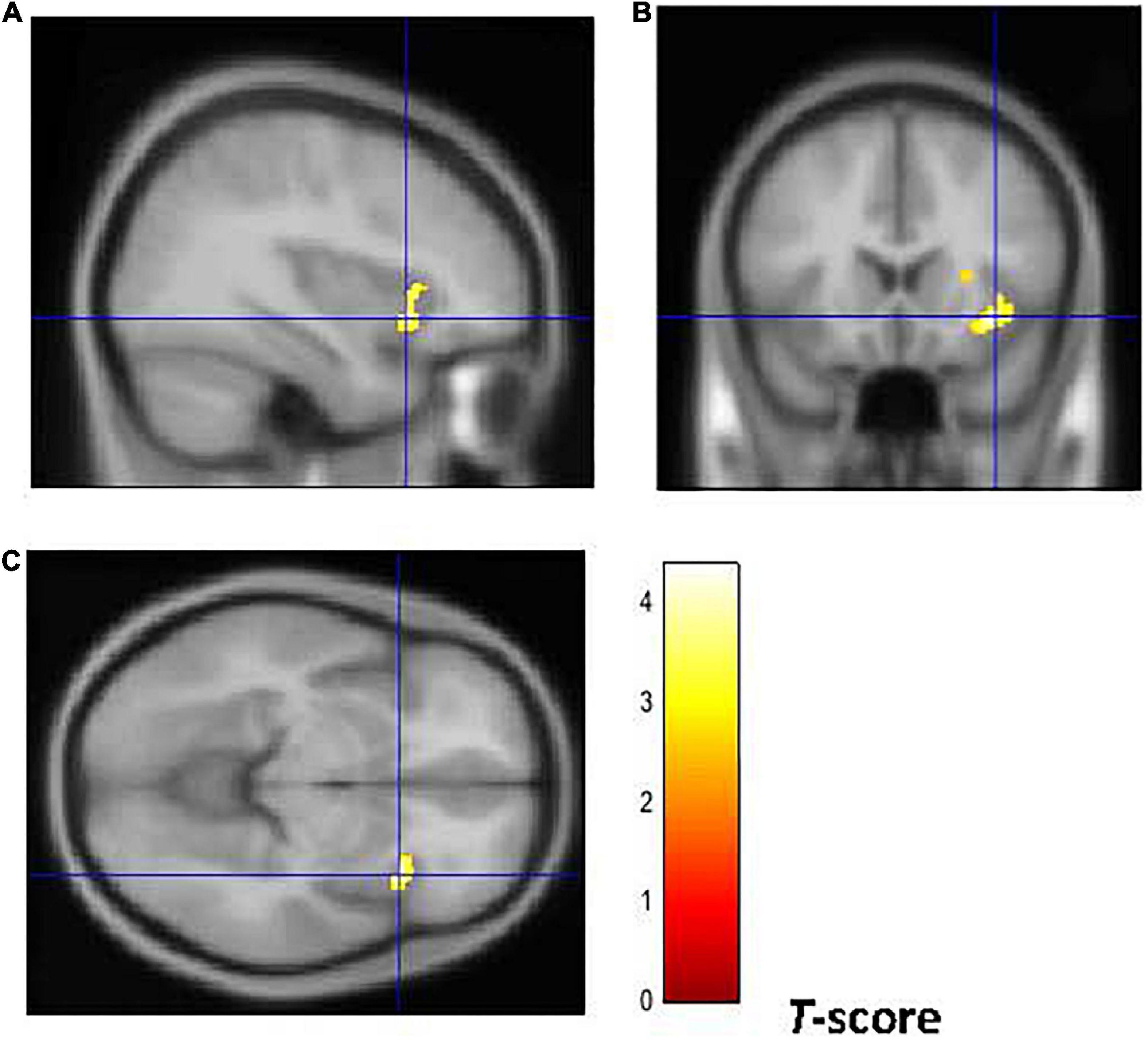
Figure 5. Brain regions of interest displaying lower WM volume in early onset smokers in comparison to late onset smokers. The figure shows early onset smokers to display lower WM volume in the right AI [region of interest centered at 36, 18, –8 MNI coordinates in sagittal (A), coronal (B), and axial (C) planes] in comparison to late onset smokers. The cluster forming threshold consisted in p < 0.01 with a minimum of 100 contiguous voxels per cluster at a whole-brain corrected level. TIV, age, and biological sex were inserted as covariates of no interest.
Neuroimaging: Voxel-wise regression models
Whole-brain voxel-wise regression results revealed negative associations between higher CGT “risk taking” and “overall proportion bet” scores manifested by early-onset smokers in comparison to non-smokers and GM/WM volume in several regions of interest, and these are shown in Table 4 (GM) and Table 5 (WM). Exploratory analyses also showed negative correlations between GM/WM volume in a priori brain regions of interest of early-onset smokers and tobacco use characteristics (FTND scores, pack-years, and number of cigarettes smoked per day) (Tables 4, 5).
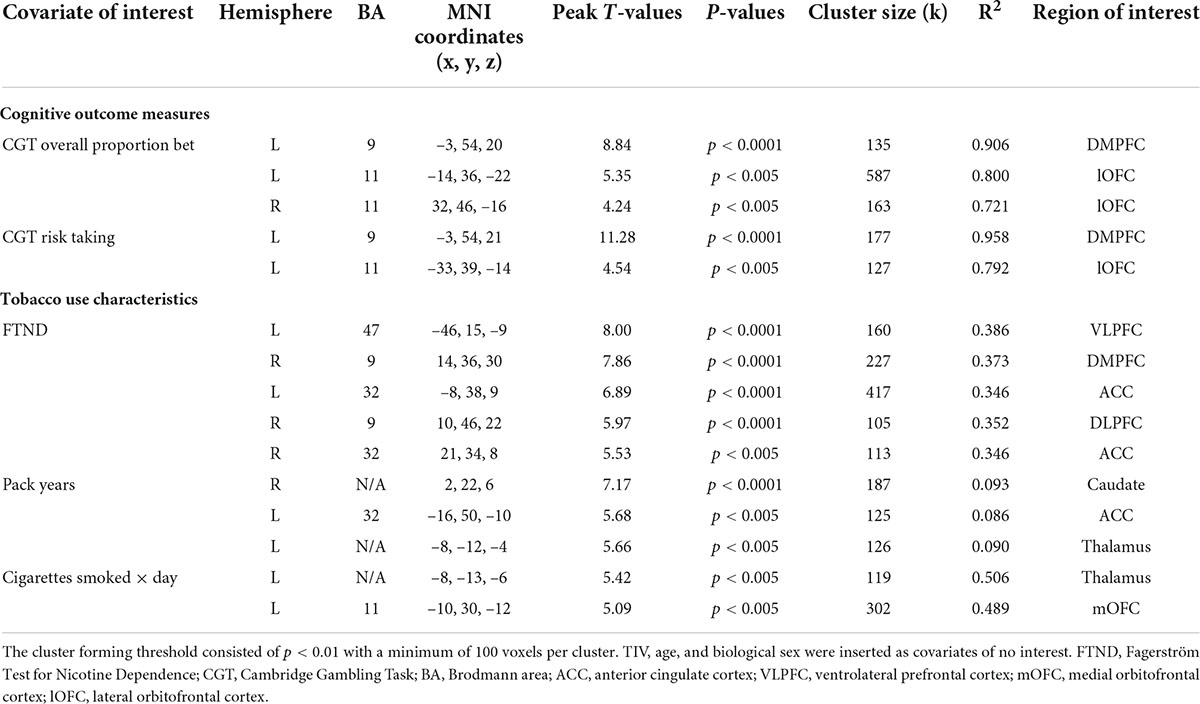
Table 4. Whole-brain voxel-wise regression models depicting negative associations between GM volume in early-onset smokers’ brain regions of interest, measures of reward-based decision-making, and tobacco use characteristics.
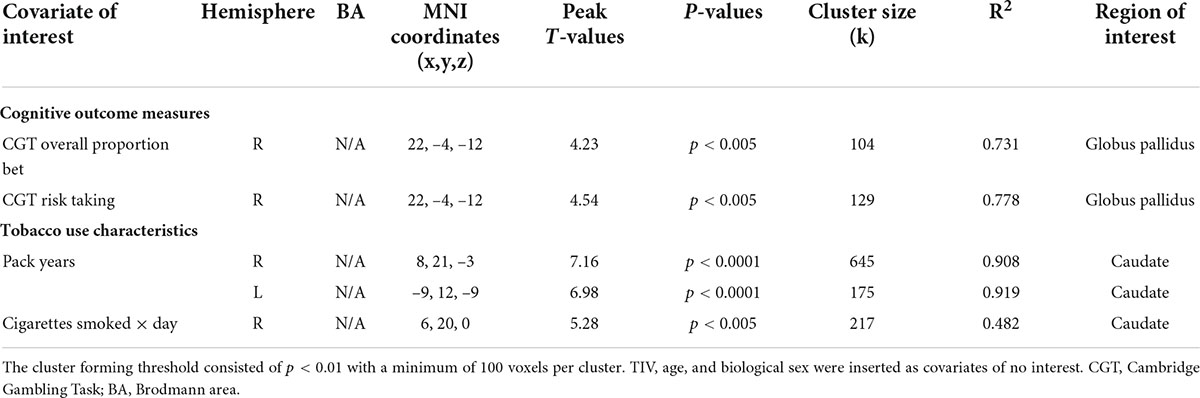
Table 5. Whole-brain voxel-wise regression models depicting negative associations between WM volume in early-onset smokers’ brain regions of interest, measures of reward-based decision-making, and tobacco use characteristics.
Discussion
Overall, the current study did not reveal any significant reward-based decision-making differences between individuals who started regular smoking at approximately 13 years of age in comparison to individuals who started smoking at 18 years of age.
Particularly, early-onset smokers showed specific facets of risky decision-making only compared to NS by betting more points overall CGT trials (overall proportion bet scores) and by betting more points when selecting the more likely outcome (risk-taking scores). No differences were detected between early-onset smokers, non-smokers, and late-onset smokers on the other CGT outcome measures (risk adjustment, quality of decision-making). Furthermore, early-onset smokers did not experience heightened choice impulsivity (ADT-5 scores) compared to both late-onset smokers and non-smokers. Therefore, hypothesis (1) of the current research was not supported. However, early-onset smokers displayed lower GM and WM volume in comparison to non-smokers in several regions of interest, such as ACC, bilateral thalamus, anterior corpus callosum, and AI. Remarkably, early-onset smokers showed lower GM volume in the bilateral AI and lower WM volume in the right AI in comparison to late-onset smokers; thus, supporting hypothesis (2) of the current research.
Negative correlations were detected between the heightened CGT “risk-taking” and “overall proportion bet” scores manifested by early-onset smokers in comparison to non-smokers and GM/WM volume in regions of interest, such as DMPFC, OFC, and white matter tracts adjacent to the globus pallidus. However, considering that CGT “risk-taking” and “overall proportion bet” scores did not differ significantly between early-onset smokers and late-onset smokers, hypothesis (3) of the current study was partially supported.
The above findings show that smoking initiation occurred during the earliest neuro-maturational stages of adolescence (13.2 years) did not impact negatively brain regions associated with impulsive and risky choices. Importantly, no differences in delay discounting rates and risk-taking scores were detected between early-onset smokers and late-onset smokers. Furthermore, early-onset smokers did not show lower GM/WM volume in prefrontal brain areas commonly associated with reward-based decision-making (e.g., VLPFC, ACC) in comparison to late-onset smokers (35, 36).
Counterintuitively, late-onset smokers (but not early-onset smokers) displayed heightened impulsive choices in contrast to non-smokers. These results seem to contradict the TINACD theory of DeBry and Tiffany (25). Indeed, evidence from genetic and twin studies in the addiction medicine literature may support the role of impaired reward-based decision-making/cognitive impulsivity as a pre-morbid trait or neurocognitive endophenotype predisposing individuals toward substance use initiation rather than as a consequence of psychoactive substance use (63–66). For example, a longitudinal-twin study design conducted by Anokhin et al. (65) on 744 adolescent twins (tested at 12 and 14 years) revealed that genetic factors predicted discounting rates at 12 years (30%) and 14 years (51%). Furthermore, discounting rates were found to be predictive of tobacco smoking initiation in the years preceding the analyses (65). Another longitudinal study conducted by Audrain-McGovern et al. (67) on 947 adolescents revealed delay discounting rates measured at baseline (15 years) to be predictive of smoking initiation. However, delay discounting rates did not increase in the years following smoking onset (67). Sparks et al. (68) revealed genetic (0.31%) and non-shared environmental effects (0.67%) to best predict impulsive choices in a sample of 791 adolescents twins aged 17 years. Importantly, “Individuals who chose the immediate reward had over three and a half times the odds of receiving a diagnosis of drug dependence and almost five times the odds of receiving a diagnosis of nicotine dependence” [(68), p. 107]. Euser et al. (69) revealed adolescents with parents suffering from substance use disorder (SUD) to make more risky choices (as assessed by the BART) in comparison to adolescents with parents not suffering from SUD. Other neuroimaging studies identified structural abnormalities in prefrontal brain areas associated with impulsive and risky choices (e.g., ACC, PFC) of substance-dependent individuals and their non-dependent biological siblings [e.g., (70, 71)].
Despite the above evidence, the postulation of impaired reward-based decision-making as a putative pre-morbid neurocognitive endophenotype/vulnerability trait for the tobacco smokers enrolled in the current study remains intuitive due to the cross-sectional nature of the current research and to the absence of genetic and/or neuroimaging data from non-smoker siblings. The most prominent findings from the current study consist in the lower GM volume identified in the bilateral AI of early-onset smokers, and in the lower WM volume identified in the right AI of early-onset smokers, in comparison to late-onset smokers. The fact that early-onset smokers and late-onset smokers were well matched in relation to tobacco exposure and nicotine dependence variables may suggest that these structural brain abnormalities were more impacted by the age of regular smoking onset rather than by chronic tobacco smoking (e.g., early-onset smokers did not report longer pack-years compared to late-onset smokers). Furthermore, no correlations were identified between tobacco exposure variables and GM/WM volume in the AI as revealed by voxel-wise regression results (Tables 4, 5).
The role of the insular cortex in addictions received substantial attention in the last decade [e.g., (72–75)]. Notably, neurofunctional and neurostructural disruptions of the insular cortex have been found to facilitate the transition from an impulsive drug intake to a compulsive drug seeking and taking behavior. This compulsive behavior is a common feature of addictions and substance use disorders (SUDs). According to Lüscher et al. (76), the symptoms listed in DSM-V for SUD reflect a compulsive behavior which is characterized by an excessive time spent searching for the drug of abuse when it is not available, by the prioritization of the search for the drug of abuse over other activities (e.g., familial, social, work-related), by a failure to avoid self-harm, and by the craving for the substance of abuse. Belin-Rauscent et al. (74), who defined the insula as “the neurobiological gate for the development of compulsive behavior,” revealed lower cortical thinness in the bilateral insula of 140 rats to be causally related to heightened motor disinhibition and compulsivity as assessed by a five-choice serial reaction time task (5-CSRTT) and by schedule-induced polydipsia (SIP) task (74). Regarding studies conducted on humans, an MRI study carried out by Grodin et al. (77) showed GM volume and thickness in the bilateral anterior insular cortex of 60 alcohol-dependent individuals (55% of alcohol-dependent individuals were also tobacco smokers) to be negatively correlated with high scores on the “Obsessive–Compulsive Drinking Scale” (OCDS). Morales et al. (78) revealed GM volume in the ventral anterior insular cortex of young cigarette smokers (mean age = 19 years) to be negatively correlated with scores on the “cigarette dependence scale” (CDS) (78). As stated by the authors, “The CDS assesses an individual’s subjective experience of symptoms, such as craving, compulsion to use, levels of stress when unable to smoke, and difficulty quitting or controlling intake” [(78), p. 1,820]. A recent activation likelihood estimation (ALE) meta-analysis conducted by Klugah-Brown et al. (79) on 144 fMRI studies revealed individuals affected by SUDs (cocaine, cannabis, alcohol, and tobacco) and obsessive–compulsive disorder (OCD) share neurofunctional alterations in the bilateral anterior insular cortex. Neurofunctional alterations in the anterior insular cortex have been also associated with impaired processing and regulation of negative moods and emotions in individuals affected by major depressive disorder (80, 81).
The importance of the insular cortex in mediating addiction to tobacco and compulsive cigarette smoking has been also emphasized by brain lesion studies. Remarkably, Naqvi et al. (82) revealed that smoking behavior was disrupted in 19 neurological patients who suffered traumatic insula damage (either left or right) but not in 50 neurological patients who suffered traumatic damage in other brain regions. Similarly, Suñer-Soler et al. (83) revealed that chronic smokers who suffered an acute stroke lesion in the insular cortex were likely to quit smoking within 1 year after brain damage. Gaznick et al. (84) also investigated prospectively (1, 3, 6, and 12 months) the effect of basal ganglia and insula damage on changes in smoking behavior and nicotine dependence in a sample of 63 neurological patients. Their results showed that patients with damages in both basal ganglia and insular cortex presented a more prominent disruption in smoking behavior and nicotine dependence in comparison to patients suffering from damages in the basal ganglia only.
The mechanistic implications of compulsive cigarette seeking and smoking behavior modulated by neurofunctional and neurostructural alterations in the insular cortex are outside the scope of the current study. However, it has been suggested that it may be related to a dysfunctional interoceptive system [see (85) and (75)]. Briefly, a dysfunctional interoceptive system may alter the perception of cue-associated conditioned responses, ultimately leading to craving for cigarettes. Indeed, several studies reported an overactivation of the insular cortex, while participants were exposed to smoking cues during acute tobacco abstinence (86–88). A recent fMRI study conducted by Ghahremani et al. (89) also reported a positive correlation between resting-state functional connectivity of the right ventral AI, craving for cigarettes, and physical symptoms of tobacco withdrawal experienced by smokers during acute abstinence (12 h). Furthermore, findings from a structural MRI study conducted by Perez Diaz et al. (90) showed a negative association between cortical thickness in the right AI and craving symptoms experienced by women smokers after overnight smoking abstinence.
Taken together, results from the current study may suggest that smoking initiation occurring during early adolescence may not be associated with impaired reward-based decision-making. Rather, lower GM and WM volume identified in the AI of early-onset smokers (compared to late-onset smokers) may indicate a vulnerability to develop a compulsive tobacco smoking behavior. This assumption is highly speculative and needs to be investigated further by neuropsychological studies employing neuroimaging techniques (e.g., VBM) and compulsivity tasks/measures.
Strengths and limitations
The current study presents several strengths. First, the findings of the current study are novel. This is the first study in the literature (to the best of our knowledge) that investigated reward-based decision-making differences and structural brain abnormalities in early-onset smokers in comparison to late-onset smokers by utilizing both cognitive and neuroimaging methods.
Second, the inclusion and exclusion criteria for the current study are stringent, and objective measures (e.g., exhaled CO, salivary cotinine, urine analysis) were employed to assess the smoking status of participants and to identify the presence of illicit substances in their system. Third, early-onset smokers and late-onset smokers were well matched in relation to tobacco exposure variables. The number of occasional cannabis smokers in all participants’ groups was also small.
Limitations of this study include the small sample size. Additionally, causality cannot be directly inferred from the current study results. Due to the cross-sectional design of the current research, it is not possible to infer if the lower GM and WM volume identified in the AI of early-onset smokers is a pre-morbid characteristic or occurred post smoking onset as a result of the neurotoxic effect of tobacco on the adolescent brain. Similarly, it is not possible to infer if impaired reward-based decision-making is a neurocognitive endophenotype/vulnerability trait for the smokers enrolled in the current study. A longitudinal study employing neuroimaging, cognitive, and genetic analyses would be needed to elucidate the causal relationship between early-onset smoking, impairments in reward-based decision-making, and compulsive tobacco seeking and smoking.
Conclusion
Early-onset smokers made more risky choices in comparison to non-smokers (as assessed by the CGT). Late-onset smokers are discounted at higher rates hypothetical 10£ in comparison to non-smokers (as assessed by the ADT-5). However, no reward-based decision-making differences (impulsive and risky choices) were detected between early-onset smokers and late-onset smokers. VBM analyses revealed early-onset smokers to present lower WM volume in the left AI and bilateral thalamus and left anterior corpus callosum in comparison to non-smokers. Lower GM volume was also detected in the right ACC of early-onset smokers in comparison to non-smokers. Remarkably, early-onset smokers presented lower GM volume in the bilateral AI in comparison to late-onset smokers in addition to lower WM volume in the right AI. Early-onset smokers did not present lower GM/WM volume in other prefrontal brain areas in comparison to late-onset smokers. These findings suggest that smoking onset occurring during the early neuro-maturational stages of adolescence may not be associated with the heightened impulsive and risky choices commonly manifested by chronic tobacco smokers (29, 32, 91, 92). However, lower GM and WM volume in the AI may indicate that early-onset smokers are more vulnerable to developing compulsive tobacco seeking and smoking behavior in comparison to late-onset smokers. This assumption needs to be investigated by future research studies.
Data availability statement
The raw data supporting the conclusions of this article will be made available by the authors, without undue reservation.
Ethics statement
The studies involving human participants were reviewed and approved by the London Bromley Research Ethics Committee (REC) (REC Reference Number: 19/LO/1176) and by the University of St. Andrews Teaching and Research Ethics Committee (UTREC) (UTREC Approval Code: MD14516). The patients/participants provided their written informed consent to participate in this study.
Author contributions
AC and AB were responsible for the study concept and design. AC contributed to the recruitment of participants, acquisition of data, performed the analysis of data, and drafted the manuscript. AB supervised recruitment, screening procedures, assisted with data analysis, interpretation of findings, and provided critical revision of the manuscript for important intellectual content. Both authors critically reviewed the content and approved the final version for publication.
Funding
This study was supported by the University of St. Andrews Endowment fund.
Acknowledgments
We wish to thank Douglas Steele and Serenella Tolomeo for the theoretical advice and support provided during the analysis of neuroimaging data. We wish to thank Jennifer McFarlane and all radiographers at the Clinical Research Center (CRC) at Ninewells Hospital, Dundee for the support provided during the neuroimaging procedures. Finally, we wish to thank all participants for their involvement in the study.
Conflict of interest
The authors declare that the research was conducted in the absence of any commercial or financial relationships that could be construed as a potential conflict of interest.
Publisher’s note
All claims expressed in this article are solely those of the authors and do not necessarily represent those of their affiliated organizations, or those of the publisher, the editors and the reviewers. Any product that may be evaluated in this article, or claim that may be made by its manufacturer, is not guaranteed or endorsed by the publisher.
Footnotes
- ^ https://www.cambridgecognition.com/cantab/cognitive-tests/executive-function/cambridge-gambling-task-cgt/
- ^ https://www.psychologie.hhu.de/arbeitsgruppen/allgemeine-psychologie-und-arbeitspsychologie/gpower
- ^ https://www.fil.ion.ucl.ac.uk/spm/
- ^ http://www.neuro.uni-jena.de/cat/
- ^ https://bioimagesuiteweb.github.io/webapp/mni2tal.html
- ^ http://www.talairach.org/
References
1. World Health Organisation [WHO]. Tobacco Key Facts. (2020). Available online at: https://www.who.int/news-room/fact-sheets/detail/tobacco (accessed May 27, 2020).
2. US Department of Health and Human Services. The Health Consequences of Smoking—50 Years of Progress A Report of the Surgeon General. Rockville, MD: Us Department of Health and Human Services. (2014).
3. Reitsma MB, Flor LS, Mullany EC, Gupta V, Hay SI, Gakidou E. Spatial, temporal, and demographic patterns in prevalence of smoking tobacco use and initiation among young people in 204 countries and territories’, 1990–2019. Lancet Public Health. (2021) 6:E472–81. doi: 10.1016/S2468-2667(21)00102-X
4. Bonnie RJ, Stratton K, Kwan LY. Public Health Implications of Raising the Minimum Age of Legal Access to Tobacco Products. Washington, DC: National Academies Press (2015).
5. Lydon DM, Wilson SJ, Child A, Geier CF. Adolescent brain maturation and smoking: what we know and where we’re headed. Neurosci Biobehav Rev. (2014) 45:323–42. doi: 10.1016/j.neubiorev.2014.07.003
6. Centre for Disease and Control Prevention. Youth and Tobacco Use. (2019). Available online at: https://www.cdc.gov/tobacco/data_statistics/fact_sheets/youth_data/tobacco_use/index.htm (accessed November 16, 2021).
7. Mashhoon Y, Betts J, Farmer SL, Lukas SE. Early onset tobacco cigarette smokers exhibit deficits in response inhibition and sustained attention. Drug Alcohol Depend. (2018) 184:48–56. doi: 10.1016/j.drugalcdep.2017.11.020
8. Hu T, Gall SL, Widome R, Bazzano LA, Burns TL, Daniels SR, et al. Childhood/adolescent smoking and adult smoking and cessation: the International Childhood Cardiovascular Cohort (i3C) Consortium. J Am Heart Associat. (2020) 9:e014381. doi: 10.1161/JAHA.119.014381
9. Kendler KS, Myers J, Damaj MI, Chen X. Early smoking onset and risk for subsequent nicotine dependence: a monozygotic co-twin control study. Am J Psychiatry. (2013) 170:408–13. doi: 10.1176/appi.ajp.2012.12030321
10. Paul SL, Blizzard L, Patton GC, Dwyer T, Venn A. Parental smoking and smoking experimentation in childhood increase the risk of being a smoker 20 years later: the Childhood Determinants of Adult Health Study. Addiction. (2008) 103:846–53. doi: 10.1111/j.1360-0443.2008.02196.x
11. Blakemore S-J, Robbins TW. Decision-making in the adolescent brain. Nat Neurosci. (2012) 15:1184–91. doi: 10.1038/nn.3177
12. Balogh KN, Mayes LC, Potenza MN. Risk-taking and decision-making in youth: relationships to addiction vulnerability. J Behav Addict. (2013) 2:1–9. doi: 10.1556/jba.2.2013.1.1
13. Talip T, Murang Z, Kifli N, Naing L. Systematic review of smoking initiation among Asian adolescents, 2005-2015: utilizing the frameworks of triadic influence and planned behavior. Asian Pac J Cancer Prev. (2016) 17:3341–55. doi: 10.14456/apjcp.2016.99/APJCP.2016.17.7.3341
14. Ren M, Lotfipour S, Leslie F. Unique effects of nicotine across the lifespan. Pharmacol Biochem Behav. (2022) 214:173343. doi: 10.1016/j.pbb.2022.173343
15. Leslie FM. Unique, long-term effects of nicotine on adolescent brain. Pharmacol Biochem Behav. (2020) 197:173010. doi: 10.1016/j.pbb.2020.173010
16. Yuan M, Cross SJ, Loughlin SE, Leslie FM. Nicotine and the adolescent brain. J Physiol. (2015) 593:3397–412. doi: 10.1113/JP270492
17. Goriounova NA, Mansvelder HD. Short-and long-term consequences of nicotine exposure during adolescence for prefrontal cortex neuronal network function. Cold Spring Harbor Perspect Med. (2012) 2:a012120. doi: 10.1101/cshperspect.a012120
18. Polesskaya OO, Fryxell KJ, Merchant AD, Locklear LL, Ker K-F, McDonald CG, et al. Nicotine causes age-dependent changes in gene expression in the adolescent female rat brain. Neurotoxicol Teratol. (2007) 29:126–40. doi: 10.1016/j.ntt.2006.11.005
19. Trauth JA, Seidler FJ, Slotkin TA. Persistent and delayed behavioral changes after nicotine treatment in adolescent rats. Brain Res. (2000) 880:167–72. doi: 10.1016/S0006-8993(00)02823-7
20. Del Ciampo LA, Del Ciampo IRL. Adolescents, e-cigarettes and nicotine: a dangerous interaction. Int J Med Rev Case Rep. (2019) 3:640–5. doi: 10.5455/IJMRCR.e-cigarettes-nicotine
21. Counotte DS, Goriounova NA, Li KW, Loos M, Van Der Schors RC, Schetters D, et al. Lasting synaptic changes underlie attention deficits caused by nicotine exposure during adolescence. Nat Neurosci. (2011) 14:417–9. doi: 10.1038/nn.2770
22. Renzetti S, Cagna G, Calza S, Conversano M, Fedrighi C, Forte G, et al. The effects of the exposure to neurotoxic elements on Italian schoolchildren behavior. Sci Rep. (2021) 11:9898. doi: 10.1038/s41598-021-88969-z
23. Rodríguez-Barranco M, Lacasaña M, Gil F, Lorca A, Alguacil J, Rohlman DS, et al. Cadmium exposure and neuropsychological development in school children in Southwestern Spain. Environ Res. (2014) 134:66–73. doi: 10.1016/j.envres.2014.06.026
24. Lidsky TI, Schneider JS. Lead neurotoxicity in children: basic mechanisms and clinical correlates. Brain. (2003) 126:5–19. doi: 10.1093/brain/awg014
25. DeBry SC, Tiffany ST. Tobacco-induced neurotoxicity of adolescent cognitive development (TINACD): a proposed model for the development of impulsivity in nicotine dependence. Nicotine Tobacco Res. (2008) 10:11–25. doi: 10.1080/14622200701767811
26. Jacobsen LK, Krystal JH, Mencl WE, Westerveld M, Frost SJ, Pugh KR. Effects of smoking and smoking abstinence on cognition in adolescent tobacco smokers. Biol Psychiatry. (2005) 57:56–66. doi: 10.1016/j.biopsych.2004.10.022
27. Galván A, Poldrack RA, Baker CM, McGlennen KM, London ED. Neural correlates of response inhibition and cigarette smoking in late adolescence. Neuropsychopharmacology. (2011) 36:970–8. doi: 10.1038/npp.2010.235
28. Galván A, Schonberg T, Mumford J, Kohno M, Poldrack RA, London ED. Greater risk sensitivity of dorsolateral prefrontal cortex in young smokers than in nonsmokers. Psychopharmacology. (2013) 229:345–55. doi: 10.1007/s00213-013-3113-x
29. Conti AA, McLean L, Tolomeo S, Steele D, Baldacchino AM. Chronic tobacco smoking and neuropsychological impairments: a systematic review and meta-analysis. Neurosci Biobehav Rev. (2019) 96:143–54. doi: 10.1016/j.neubiorev.2018.11.017
31. Syan SK, González-Roz A, Amlung M, Sweet LH, MacKillop J. Delayed reward discounting as a prognostic factor for smoking cessation treatment outcome: a systematic review. Nicotine Tobacco Res. (2021) 23:1636–45. doi: 10.1093/ntr/ntab052
32. Barlow P, McKee M, Reeves A, Galea G, Stuckler D. Time-discounting and tobacco smoking: a systematic review and network analysis. Int J Epidemiol. (2017) 46:860–9. doi: 10.1093/ije/dyw233
33. Yang Z, Zhang Y, Cheng J, Zheng R. Meta-analysis of brain gray matter changes in chronic smokers. Eur J Radiol. (2020) 132:109300. doi: 10.1016/j.ejrad.2020.109300
34. Zhong J, Shi H, Shen Y, Dai Z, Zhu Y, Ma H, et al. Voxelwise meta-analysis of gray matter anomalies in chronic cigarette smokers. Behav Brain Res. (2016) 311:39–45. doi: 10.1016/j.bbr.2016.05.016
35. Conti AA, Baldacchino AM. Neuroanatomical correlates of impulsive choices and risky decision making in young chronic tobacco smokers: a voxel-based morphometry study. Front Psychiatry. (2021) 12:708925. doi: 10.3389/fpsyt.2021.708925
36. Durazzo TC, Meyerhoff DJ, Yoder KK, Murray DE. Cigarette smoking is associated with amplified age-related volume loss in subcortical brain regions. Drug Alcohol Depend. (2017) 177:228–36. doi: 10.1016/j.drugalcdep.2017.04.012
37. Huang P, Shen Z, Wang C, Qian W, Zhang H, Yang Y, et al. Altered white matter integrity in smokers is associated with smoking cessation outcomes. Front Hum Neurosci. (2017) 11:438. doi: 10.3389/fnhum.2017.00438
38. Savjani RR, Velasquez KM, Thompson-Lake DG, Baldwin PR, Eagleman DM, De La Garza IIR, et al. Characterizing white matter changes in cigarette smokers via diffusion tensor imaging. Drug Alcohol Depend. (2014) 145:134–42. doi: 10.1016/j.drugalcdep.2014.10.006
39. Umene-Nakano W, Yoshimura R, Kakeda S, Watanabe K, Hayashi K, Nishimura J, et al. Abnormal white matter integrity in the corpus callosum among smokers: tract- based spatial statistics. PLoS One. (2014) 9:e87890. doi: 10.1371/journal.pone.0087890
40. Gillespie NA, Neale MC, Bates TC, Eyler LT, Fennema-Notestine C, Vassileva J, et al. Testing associations between cannabis use and subcortical volumes in two large population-based samples. Addiction. (2018) 113:1661–72. doi: 10.1111/add.14252
41. Figueiredo PR, Tolomeo S, Steele JD, Baldacchino A. Neurocognitive consequences of chronic cannabis use: a systematic review and meta-analysis. Neurosci Biobehav Rev. (2020) 108:358–69. doi: 10.1016/j.neubiorev.2019.10.014
42. Lorenzetti V, Kowalczyk M, Duehlmeyer L, Greenwood LM, Chye Y, Yücel M, et al. Brain anatomical alterations in young cannabis users: is it all hype? A meta- analysis of structural neuroimaging studies. Can Cannabinoid Res. (2022) [Online ahead of print]. doi: 10.1089/can.2021.0099
43. Ehrenreich H, Rinn T, Kunert HJ, Moeller MR, Poser W, Schilling L, et al. Specific attentional dysfunction in adults following early start of cannabis use. Psychopharmacology. (1999) 142:295–301. doi: 10.1007/s002130050892.pdf
44. Slade EP, Stuart EA, Salkever DS, Karakus M, Green KM, Ialongo N. Impacts of age of onset of substance use disorders on risk of adult incarceration among disadvantaged urban youth: a propensity score matching approach. Drug Alcohol Depend. (2008) 95:1–13. doi: 10.1016/j.drugalcdep.2007.11.019
45. Norberg KE, Bierut LJ, Grucza RA. Long-term effects of minimum drinking age laws on past-year alcohol and drug use disorders. Alcohol Clin Exp Res. (2009) 33:2180–90. doi: 10.1111/j.1530-0277.2009.01056.x
46. Becker B, Wagner D, Gouzoulis-Mayfrank E, Spuentrup E, Daumann J. The impact of early-onset cannabis use on functional brain correlates of working memory. Prog Neuropsychopharmacol Biol Psychiatry. (2010) 34:837–45. doi: 10.1016/j.pnpbp.2010.03.032
47. Gruber SA, Dahlgren MK, Sagar KA, Gönenc A, Killgore WD. Age of onset of marijuana use impacts inhibitory processing. Neurosci Lett. (2012) 511:89–94. doi: 10.1016/j.neulet.2012.01.039
48. Sagar KA, Dahlgren MK, Gönenç A, Racine MT, Dreman MW, Gruber SA. The impact of initiation: early onset marijuana smokers demonstrate altered Stroop performance and brain activation. Dev Cogn Neurosci. (2015) 16:84–92. doi: 10.1016/j.dcn.2015.03.003
49. Dahlgren MK, Sagar KA, Racine MT, Dreman MW, Gruber SA. Marijuana use predicts cognitive performance on tasks of executive function. J Stud Alcohol Drugs. (2016) 77:298308. doi: 10.15288/jsad.2016.77.298
50. Heatherton TF, Kozlowski LT, Frecker RC, Fagerstrom KO. The Fagerström test for nicotine dependence: a revision of the Fagerstrom Tolerance Questionnaire. Br J Addict. (1991) 86:1119–27. doi: 10.1111/j.1360-0443.1991.tb01879.x
51. Barona A, Reynolds CR, Chastain R. A demographically based index of premorbid intelligence for the WAIS—R. J Consult Clin Psychol. (1984) 52:885.
52. Koffarnus MN, Bickel WK. A 5-trial adjusting delay discounting task: accurate discount rates in less than one minute. Exp Clin Psychopharmacol. (2014) 22:222. doi: 10.1037/a0035973
53. Yoon JH, Higgins ST. Turning k on its head: comments on use of an ED50 in delay discounting research. Drug Alcohol Depend. (2008) 95:169–72. doi: 10.1016/j.drugalcdep.2007.12.011
54. Bauer IE, Meyer TD, Sanches M, Zunta-Soares G, Soares JC. Does a history of substance abuse and illness chronicity predict increased impulsivity in bipolar disorder? J Affect Disord. (2015) 179:142–7. doi: 10.1016/j.jad.2015.03.010
55. Fineberg NA, Chamberlain SR, Goudriaan AE, Stein DJ, Vanderschuren LJMJ, Gillan CM, et al. New developments in human neurocognition: clinical, genetic, and brain imaging correlates of impulsivity and compulsivity. CNS Spectr. (2014) 19:69–89. doi: 10.1017/S1092852913000801
56. Tolomeo S, Gray S, Matthews K, Steele JD, Baldacchino A. Multifaceted impairments in impulsivity and brain structural abnormalities in opioid dependence and abstinence. Psychol Med. (2016) 46:2841–53. doi: 10.1017/S0033291716001513
57. Tolomeo S, Matthews K, Steele D, Baldacchino A. Compulsivity in opioid dependence. Prog Neuropsychopharmacol Biol Psychiatry. (2018) 81:333–9. doi: 10.1016/j.pnpbp.2017.09.007
58. Baldacchino A, Balfour DJK, Matthews K. Impulsivity and opioid drugs: differential effects of heroin, methadone and prescribed analgesic medication. Psychol Med. (2015) 45:1167–79. doi: 10.1017/S0033291714002189
59. Chamberlain SR, Odlaug BL, Schreiber LR, Grant JE. Association between tobacco smoking and cognitive functioning in young adults. Am J Addict. (2012) 21:S14–9. doi: 10.1111/j.1521-0391.2012.00290.x
60. Ashburner J, Friston KJ. Unified segmentation. Neuroimage. (2005) 26:839–51. doi: 10.1016/j.neuroimage.2005.02.018
61. Slotnick SD, Moo LR, Segal JB, Hart J Jr. Distinct prefrontal cortex activity associated with item memory and source memory for visual shapes. Cogn Brain Res. (2003) 17:75–82. doi: 10.1016/S0926-6410(03)00082-X
62. Mwangi B, Matthews K, Steele JD. Prediction of illness severity in patients with major depression using structural MR brain scans. J Magn Resonan Imag. (2012) 35:64–71. doi: 10.1002/jmri.22806
63. Bickel WK. Discounting of delayed rewards as an endophenotype. Biol Psychaitry. (2015) 77:846–7.
64. Yücel M, Oldenhof E, Ahmed SH, Belin D, Billieux J, Bowden-Jones H, et al. A transdiagnostic dimensional approach towards a neuropsychological assessment for addiction: an international Delphi consensus study. Addiction. (2019) 114:1095–109. doi: 10.1111/add.14424
65. Anokhin AP, Golosheykin S, Grant JD, Heath AC. Heritability of delay discounting in adolescence: a longitudinal twin study. Behav Genet. (2010) 41:175–83. doi: 10.1007/s10519-010-9384-7
66. Anokhin AP, Grant JD, Mulligan RC, Heath AC. The genetics of impulsivity: evidence for the heritability of delay discounting. Biol Psychiatry. (2015) 77:887–94. doi: 10.1016/j.biopsych.2014.10.022
67. Audrain-McGovern J, Rodriguez D, Epstein LH, Cuevas J, Rodgers K, Wileyto EP. Does delay discounting play an etiological role in smoking or is it a consequence of smoking? Drug Alcohol Depend. (2009) 103:99–106. doi: 10.1016/j.drugalcdep.2008.12.019
68. Sparks JC, Isen JD, Iacono WG. Preference on cash-choice task predicts externalizing outcomes in 17-year-olds. Behav Genet. (2014) 44:102–12. doi: 10.1007/s10519-013-9638-2
69. Euser AS, Greaves-Lord K, Crowley MJ, Evans BE, Huizink AC, Franken IH. Blunted feedback processing during risky decision making in adolescents with a parental history of substance use disorders. Dev Psychopathol. (2013) 25(4 Pt 1):1119–36. doi: 10.1017/S0954579413000412
70. Ersche KD, Turton AJ, Pradhan S, Bullmore ET, Robbins TW. Drug addiction endophenotypes: impulsive versus sensation-seeking personality traits. Biol Psychiatry. (2010) 68:770–3. doi: 10.1016/j.biopsych.2010.06.015
71. Ersche KD, Meng C, Ziauddeen H, Stochl J, Williams GB, Bullmore ET, et al. Brain networks underlying vulnerability and resilience to drug addiction. Proc Natl Acad Sci. (2020) 117:15253–61. doi: 10.1073/pnas.2002509117
72. Campbell EJ, Flanagan J, Walker LC, Hill M, Marchant NJ, Lawrence AJ. Anterior insular cortex is critical for the propensity to relapse following punishment-imposed abstinence of alcohol seeking. J Neurosci. (2019) 39:1077–87. doi: 10.1523/JNEUROSCI.1596-18.2018
73. Ibrahim C, Rubin-Kahana DS, Pushparaj A, Musiol M, Blumberger DM, Daskalakis ZJ, et al. The insula: a brain stimulation target for the treatment of addiction. Front Pharmacol. (2019) 10:720. doi: 10.3389/fphar.2019.00720
74. Belin-Rauscent A, Daniel M-L, Puaud M, Jupp B, Sawiak S, Howett D, et al. From impulses to maladaptive actions: the insula is a neurobiological gate for the development of compulsive behavior. Mol. Psychiatry. (2015) 21:491–9. doi: 10.1038/mp.2015.140
75. Droutman V, Read SJ, Bechara A. Revisiting the role of the insula in addiction. Trends Cogn Sci. (2015) 19:414–20. doi: 10.1016/j.tics.2015.05.005
76. Lüscher C, Robbins TW, Everitt BJ. The transition to compulsion in addiction. Nat Rev Neurosci. (2020) 21:247–63. doi: 10.1038/s41583-020-0289-z
77. Grodin EN, Cortes CR, Spagnolo PA, Momenan R. Structural deficits in salience network regions are associated with increased impulsivity and compulsivity in alcohol dependence. Drug Alcohol Depend. (2017) 179:100–8. doi: 10.1016/j.drugalcdep.2017.06.014
78. Morales AM, Ghahremani D, Kohno M, Hellemann GS, London ED. Cigarette exposure, dependence, and craving are related to insula thickness in young adult smokers. Neuropsychopharmacology. (2014) 39:1816–22. doi: 10.1038/npp.2014.48
79. Klugah-Brown B, Zhou X, Pradhan BK, Zweerings J, Mathiak K, Biswal B, et al. Common neurofunctional dysregulations characterize obsessive–compulsive, substance use, and gaming disorders—An activation likelihood meta-analysis of functional imaging studies. Addict Biol. (2021) 26:e12997. doi: 10.1111/adb.12997
80. Aryutova K, Paunova R, Kandilarova S, Stoyanova K, Maes MH, Stoyanov D. Differential aberrant connectivity of precuneus and anterior insula may underpin the diagnosis of schizophrenia and mood disorders. World J Psychiatry. (2021) 11:1274–87. doi: 10.5498/wjp.v11.i12.1274
81. Sliz D, Hayley S. Major depressive disorder and alterations in insular cortical activity: a review of current functional magnetic imaging research. Front Hum Neurosci. (2012) 6:323. doi: 10.3389/fnhum.2012.00323
82. Naqvi NH, Rudrauf D, Damasio H, Bechara A. Damage to the insula disrupts addiction to cigarette smoking. Science. (2007) 315:531–4. doi: 10.1126/science.1135926
83. Suñer-Soler R, Grau A, Gras ME, Font-Mayolas S, Silva Y, Dávalos A, et al. Smoking cessation 1 year poststroke and damage to the insular cortex. Stroke. (2012) 43:131–6. doi: 10.1161/STROKEAHA.111.630004
84. Gaznick N, Tranel D, McNutt A, Bechara A. Basal ganglia plus insula damage yields stronger disruption of smoking addiction than basal ganglia damage alone. Nicotine Tobacco Res. (2014) 16:445–53. doi: 10.1093/ntr/ntt172
85. Paulus MP, Stewart JL. Interoception and drug addiction. Neuropharmacology. (2014) 76:342–50. doi: 10.1016/j.neuropharm.2013.07.002
86. Engelmann JM, Versace F, Robinson JD, Minnix JA, Lam CY, Cui Y, et al. Neural substrates of smoking cue reactivity: a meta-analysis of fMRI studies. Neuroimage. (2012) 60:252–62. doi: 10.1016/j.neuroimage.2011.12.024
87. Moran LV, Stoeckel LE, Wang K, Caine CE, Villafuerte R, Calderon V, et al. Nicotine increases activation to anticipatory valence cues in anterior insula and striatum. Nicotine Tobacco Res. (2018) 20:851–8. doi: 10.1093/ntr/ntx217
88. Janes AC, Gilman JM, Radoman M, Pachas G, Fava M, Evins AE. Revisiting the role of the insula and smoking cue-reactivity in relapse: a replication and extension of neuroimaging findings. Drug Alcohol Depend. (2017) 179:8–12. doi: 10.1016/j.drugalcdep.2017.06.012
89. Ghahremani DG, Pochon JB, Perez Diaz M, Tyndale RF, Dean AC, London ED. Functional connectivity of the anterior insula during withdrawal from cigarette smoking. Neuropsychopharmacology. (2021) 46:2083–9. doi: 10.1038/s41386-021-01036-z
90. Perez Diaz M, Pochon JB, Ghahremani DG, Dean AC, Faulkner P, Petersen N, et al. Sex differences in the association of cigarette craving with insula structure. Int J Neuropsychopharmacol. (2021) 24:624–33. doi: 10.1093/ijnp/pyab015
91. Hofmeyr A, Monterosso J, Dean AC, Morales AM, Bilder RM, Sabb FW, et al. Mixture models of delay discounting and smoking behavior. Am J Drug Alcohol Abuse. (2016) 43:271–80. doi: 10.1080/00952990.2016.1198797
Keywords: insular cortex, adolescent smokers, cognitive impulsivity, voxel based morphometry, chronic tobacco smoking, neuroimaging, addiction, reward-based decision-making
Citation: Conti AA and Baldacchino AM (2022) Chronic tobacco smoking, impaired reward-based decision-making, and role of insular cortex: A comparison between early-onset smokers and late-onset smokers. Front. Psychiatry 13:939707. doi: 10.3389/fpsyt.2022.939707
Received: 11 May 2022; Accepted: 01 August 2022;
Published: 26 August 2022.
Edited by:
Jasmin Vassileva, Virginia Commonwealth University, United StatesReviewed by:
Edythe D. London, University of California, Los Angeles, United StatesDrozdstoy Stoyanov Stoyanov, Plovdiv Medical University, Bulgaria
Copyright © 2022 Conti and Baldacchino. This is an open-access article distributed under the terms of the Creative Commons Attribution License (CC BY). The use, distribution or reproduction in other forums is permitted, provided the original author(s) and the copyright owner(s) are credited and that the original publication in this journal is cited, in accordance with accepted academic practice. No use, distribution or reproduction is permitted which does not comply with these terms.
*Correspondence: Aldo Alberto Conti, YWxkby5jb250aUBrY2wuYWMudWs=