- 1Department of Psychiatry, The First Affiliated Hospital of Chongqing Medical University, Chongqing, China
- 2National Health Commission (NHC) Key Laboratory of Diagnosis and Treatment on Brain Functional Diseases, The First Affiliated Hospital of Chongqing Medical University, Chongqing, China
- 3Department of Neurology, The First Affiliated Hospital of Chongqing Medical University, Chongqing, China
Background: Children and adolescents are at a high risk of major depressive disorder (MDD) with known sex differences in epidemiology. However, there are currently no objective laboratory-based sex-specific biomarkers available to support the diagnoses of male and female patients with MDD.
Methods: Here, a male set of 42 cases and 27 healthy controls (HCs) and a female set of 42 cases and 22 HCs were recruited. This study investigated the sex differences of plasma metabolite biomarkers in young patients with MDD by the application of ultra-high-performance liquid chromatography equipped with quadrupole time-of-flight mass spectrometry.
Results: The metabolic profiles showed clear separations in both male and female sets. In total, this study identified 57 male-related and 53 female-related differential metabolites. Compared with HCs, both male and female subjects with MDD displayed four significantly altered pathways. Notably, biliverdin was selected as an independent diagnostic male-specific biomarker with an area under the receiver operating characteristic curve of 0.966, and phosphatidylcholine (10:0/14:1) was selected as a female-specific biomarker, achieving an area under the curve (AUC) of 0.957.
Conclusion: This metabolomics study may aid in the development of a plasma-based test for the diagnosis of male and female children and adolescents with MDD, as well as give new insight into the pathophysiology of sex differences in children and adolescents with MDD.
Introduction
Major depressive disorder (MDD) is a common mental health problem worldwide, with 322 million people suffering from depression, and an estimated prevalence of 3.2% from 3 to 17 years of age (1, 2). Globally, MDD has become the top cause of illness and disability during adolescence, and more than half of adolescent suicide victims exhibit MDD at the time of death (3, 4). The sex difference in depression rates begins in childhood and persists into old age, with females having a strong prevalence for MDD compared with males (approximately 2:1), and this gender gap becomes smaller in adults compared with younger ages (5, 6). Notably, the sex difference peaks in adolescence (odds ratio = 3.02 for ages 13–15 years, and uncorrected effect size = 0.47 for 16 years of age) in depression (7). Several hypotheses have attempted to explain this phenomenon. Research indicates that increases in sex steroids are linked to organizational effects on neural structure and function (8). A high level of testosterone was associated with higher levels of adolescent depression in girls (9). One circuit, which has high concentrations of sex steroid receptors, connects the amygdala to the hippocampus and ventral expanses of the prefrontal cortex. This circuit is linked to hypothalamic-pituitary-adrenal axis activity and may provide a biological mechanism for why girls have higher risk of depression than boys (10). However, these hypotheses need to be tested and verified by research. According to previous studies, metabolism differences between boys and girls with MDD, like sex steroids, may affect neural function or the activity of the hypothalamic-pituitary-adrenal axis, which has a different impact on the epidemiology, symptomatology, and pathogenesis of depression (11). Therefore, sex-specific molecular characteristics are required to clarify the sex differences in MDD.
Metabolomics, which identifies and quantifies metabolites in various biological fluids such as plasma and urine, has been extensively applied to determine the metabolic perturbations in various disease states (12). In earlier studies, metabolomics was successfully used to identify novel biomarkers for neuropsychiatric disorders, such as schizophrenia and bipolar disorder (13, 14). Previously, we identified some candidate diagnostic metabolite biomarkers for MDD in adults, as well as in children and adolescents (15, 16), and analyzed sex-specific metabolites of MDD in adults (17). Other researchers also found blood metabolomic signatures of major depression before and after antidepressant treatment (18). However, none of these studies have taken sex differences of MDD in children and adolescents into account, which may limit the universal applicability of these potential metabolite biomarkers to some extent. In our previous study, adults with MDD had disruptions in methionine and tryptophan metabolism, and tryptophan was identified as a key biomarker for discriminating adult MDD (16). Children and adolescents with MDD had substantial purine metabolism disturbances, and inosine was selected as an independent diagnostic biomarker. Past studies reported that MDD in children and adolescents was different from adults in pathophysiology, treatment response and neuroimaging (19–21). Thus, it is likely that sex differences are prominent for MDD in children and adolescents and may provide an understanding of the mechanisms underlying the pathoetiology in both males and females.
In this investigation, ultra-high-performance liquid chromatography equipped with quadrupole time-of-flight mass spectrometry (UPLC-Q-TOF/MS) analysis was applied to profile 84 subjects with MDD (42 males and 42 females) and 49 healthy controls (HCs, 27 males and 22 females) in children and adolescents with the aim of identifying sex differences in plasma metabolic signatures, and obtain altered metabolic pathways would help to understand the differences in mechanisms between boys and girls. We also explored sex-specific metabolite biomarkers in males and females with MDD separately, which could contribute to future clinical practice for more efficient diagnosis of depression in different populations.
Materials and Methods
Ethics Statement
The samples obtained from these subjects had been previously used to explore potential biomarkers of depression in children and adolescents with MDD (16). Ethics approval was obtained from the Institutional Review Board of Children’s Hospital of Chongqing Medical University (No. 2016121). Prior to the collection of plasma samples, written informed consent and detailed clinical information were obtained from participants (if they were 18 years old) or legal guardians (if aged 17 years or less). All procedures were conducted according to the principles expressed in the Declaration of Helsinki (22).
Subject Recruitment
For this study, HCs and patients with MDD were recruited from routine medical examinations in two schools, a primary school in Yuzhong district and a middle school in Liangping district, both in Chongqing, China, and the Children’s Hospital of Chongqing Medical University (Chongqing, China) between November 2016 and May 2017. The diagnosis of MDD was confirmed by trained psychiatrists according to criteria in the Diagnostic and Statistical Manual of Mental Disorders-version 4 (DSM-IV). The severity of depression was assessed by two standardized and validated depression scales, i.e., Children’s Depression Rating Scale-Revised (CDRS-R) for children and younger adolescents (aged < 15 years) (23) and Hamilton Depression Scale (17-Items) (HAMD-17) for older adolescents (aged ≥ 15 years) (24). Patients aged from 6 to 18 years with moderate to severe depression (i.e., CDRS-R scores ≥ 40 or HAMD-17 scores ≥ 17), without any comorbid physical, neurological or psychiatric disorders and/or illicit drug use, were included in the study. Both first-episode drug-naive patients with MDD (DN-MDD) and drug-treated patients with MDD (DT-MDD) were recruited. For HCs, subjects with no previous neurological, DSM-IV Axis I/II or medical illness were also recruited.
Sample Collection and Preparation
The fasting blood of subjects was taken in 5 mL vacutainer tubes containing heparin lithium, then centrifuged for 15 min (1,500 × g, 4°C). Plasma samples were stored at –80°C in equal aliquots (150 μL) until later analysis. Before UPLC-Q-TOF/MS analysis, 100 μL aliquots of thawed plasma samples were mixed with 400 μL of cold methanol/acetonitrile (1:1, v/v) for protein removal. The mixture was centrifuged for 15 min (14,000 × g, 4°C) and the supernatant dried in a vacuum centrifuge. The samples were redissolved in 100 μL acetonitrile/water (1:1, v/v) solvent. To monitor the stability and repeatability of instrumental analysis, 10 μL of each sample was combined into quality control (QC) samples. The QC samples were inserted regularly and analyzed in every eight samples.
Ultra-High-Performance Liquid Chromatography Equipped With Quadrupole Time-of-Flight Mass Spectrometry Analysis
An Agilent 1290 Infinity LC system (Agilent Technologies, Santa Clara, California, United States) coupled with an AB SCIEX Triple TOF 6600 system (AB SCIEX, Framingham, MA, United States) was used to undertake metabolic profiling of plasma samples. ACQUITY HSS T3 1.8 μm (2.1 × 100 mm) columns were used for chromatographic separation in both positive and negative models. The column temperature was maintained at 25°C. The mobile phases of 0.1% formic acid in water (A) and 0.1% formic acid in acetonitrile (B) were used in the positive ionization mode, while 0.5 mM ammonium fluoride in water (C) and acetonitrile (D) were used in the negative ionization mode. In the positive model, the elution gradient initially started from 1% B and increased linearly to 100% B between 1 and 8 min, and was maintained for 2 min, then returned to 1% B for 2 min to equilibrate the systems. This elution gradient was replicated in the negative model using reagents C and D. The sample injection volume was 2 μL and the flow rate was set to 300 μL/min.
The mass spectrometric data were collected using the UPLC system equipped with an electrospray ionization source operating in either positive or negative ion mode. The optimal conditions were set as follows: ion source gas 1, 40 psi; ion source gas 2, 60 psi; curtain gas, 30 psi; ion-spray voltage floating, 5,000 V in positive mode and –4,500 V in negative mode, respectively, normalized collision energy, 20–40–60 V rolling for MS/MS. Data acquisition was performed with Information-Dependent Acquisition (IDA) mode, and parameters of IDA included a declustering potential [60 V (+) and –60 V (–)], collision energy [50 V (+) and –20 V (–)], exclusion of isotopes within 4 Da, and 10 candidate ions to monitor per cycle.
Metabolomics Data Analysis
After non-targeted UPLC-Q-TOF/MS analyses, the raw data were converted to mzXML files using the Proteo Wizard MSconventer tool and then processed using XCMS online software. The parameters in XCMS were set as follows: centWave settings for feature detection [Δm/z = 25 ppm, peakwidth = c (10, 60)]; obiwarp settings for retention time correction (profStep = 1); and parameters including minfrac = 0.5, bw = 5 and mzwid = 0.025 for chromatogram alignment. Normalized and integrated data were then uploaded into MetaboAnalyst 5.0 software for further processing.1 Principal component analysis (PCA) using an unsupervised method was applied to obtain an overview of the metabolic data, general clustering, trends, or outliers. Orthogonal partial least squares discriminate analysis (OPLS-DA) was used for statistical analysis to determine global metabolic changes between comparable groups. Two matrices were involved in the OPLS-DA analysis: the X matrix was the sample-variable observation matrix, and the Y matrix was the sample category attribution matrix. R2X and R2Y represented the interpretation rate of the X and Y matrices by the model, and Q2 represented the predictive ability of the model. If both R2Y and Q2 were greater than 0.5, the model could predict well and avoided the risk of over-fitting. Variable importance in the projection (VIP) were calculated in the OPLS-DA model. P-values were estimated using Student’s t-test (subject to normal distribution) or Mann-Whitney U-test (not subject to normal distribution). Finally, metabolites with a VIP value > 1.0 and P < 0.05 were identified as metabolites exhibiting different levels and responsible for sample differentiation. Then, we analyzed the correlations between metabolites and clinical characteristics. Because only patients with MDD were assessed with CDRS-R or HAMD-17, correlations between biomarkers and depression scales scores were done in patients only. To facilitate uniform comparison, we applied the COD-FISH Tool to transform all HAMD-17 scores to CDRS-R scores (25).
Statistical Analysis
Continuous variables were presented as mean with standard deviation. As appropriate, comparisons of demographic characteristics between groups were performed on SPSS 21.0 (IBM Corp., Armonk, NY, United States) using the non-parametric Mann-Whitney U test. Normal distribution was evaluated by Kolmogorov-Smirnov test. Spearman’s correlation was applied to analyze the correlations between metabolites and CDRS-R scores. Random forest analysis was used to select the best-fit model. Receiver operating characteristic analysis was applied to determine the diagnostic performance of the best-fit model. A P-value < 0.05 was considered to be statistically significant.
Results
Clinical Characteristics for the Metabolomic Model of Major Depressive Disorder vs. Healthy Controls
The data from our previous published study was reanalyzed for this study (16). One female healthy control was omitted from the research because of the lack of metabolome data. The demographic and clinical characteristics of all the study participants are summarized in Table 1. A total of 133 participants were enrolled in the current study as children and adolescents. The male set consisted of 27 patients with first-episode DN-MDD, 15 patients with DT-MDD and 27 HCs. Among male patients with MDD, the average HAMD-17 score was 22.00, and the average CDRS-R score was 45.00. In the female set, 25 patients with DN-MDD, 17 patients with DT-MDD and 22 HCs were recruited. The average HAMD-17 score in female patients with MDD was 22.10, and the average CDRS-R score was 52.69. Between the male and female sets, there were no significant differences in the demographic variables of age and body mass index. The number of participants with MDD and on medication did not differ significantly across the male and female sets.
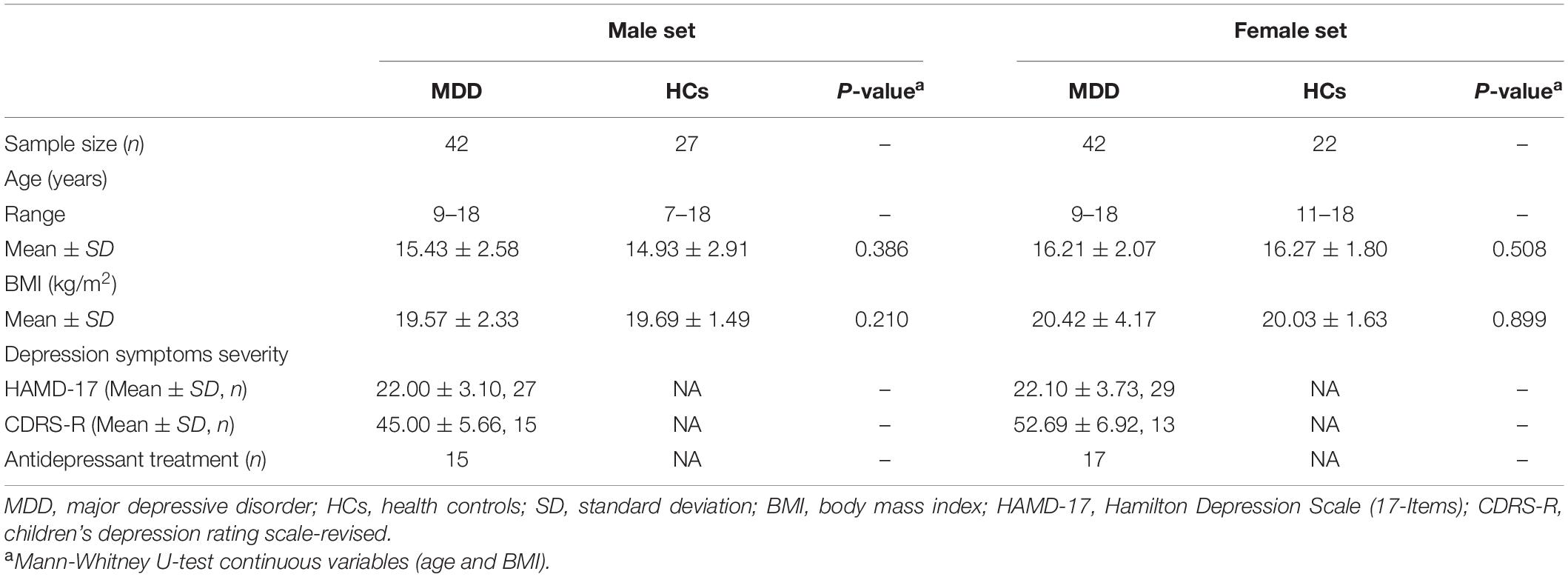
Table 1. Clinical characteristics of the recruited patients with major depressive disorder and healthy controls.
Metabolomic Analysis of Plasma From Patients With Major Depressive Disorder and Healthy Controls
There were 5,353 positive-mode features and 1,680 negative-mode features identified in the metabolic profiling. In the PCA and OPLS-DA analyses, all metabolites of the subjects were utilized. Both male and female patients with MDD were distinctly separated from their respective HCs in PCA score plots, indicating satisfactory reproducibility (Supplementary Figure 1). OPLS-DA score plots demonstrated a striking distinction between male MDD and HC subjects, as well as female MDD and HC subjects [Figure 1A; male: R2X cumulative (cum) = 0.224, R2Y cum = 0.965, Q2 cum = 0.889; Figure 1B; female: R2X cum = 0.296, R2Y cum = 0.967, Q2 cum = 0.894]. In addition, the permutation test revealed that the two OPLS-DA models developed were both valid and were not over-fitted (Figures 1C,D). The metabolites responsible for sample differentiation were identified as those having VIP values of at least 1 and a P-value of less than 0.05. In total, by analyzing the OPLS-DA loading coefficient plot, we identified 57 differential metabolites in the male set and 53 in the female set (Table 2). Among them, 40 metabolites were significantly altered in both males and females with MDD. The results of Spearman’s correlation analysis between metabolites and CDRS-R scores were shown in Supplementary Table 1.
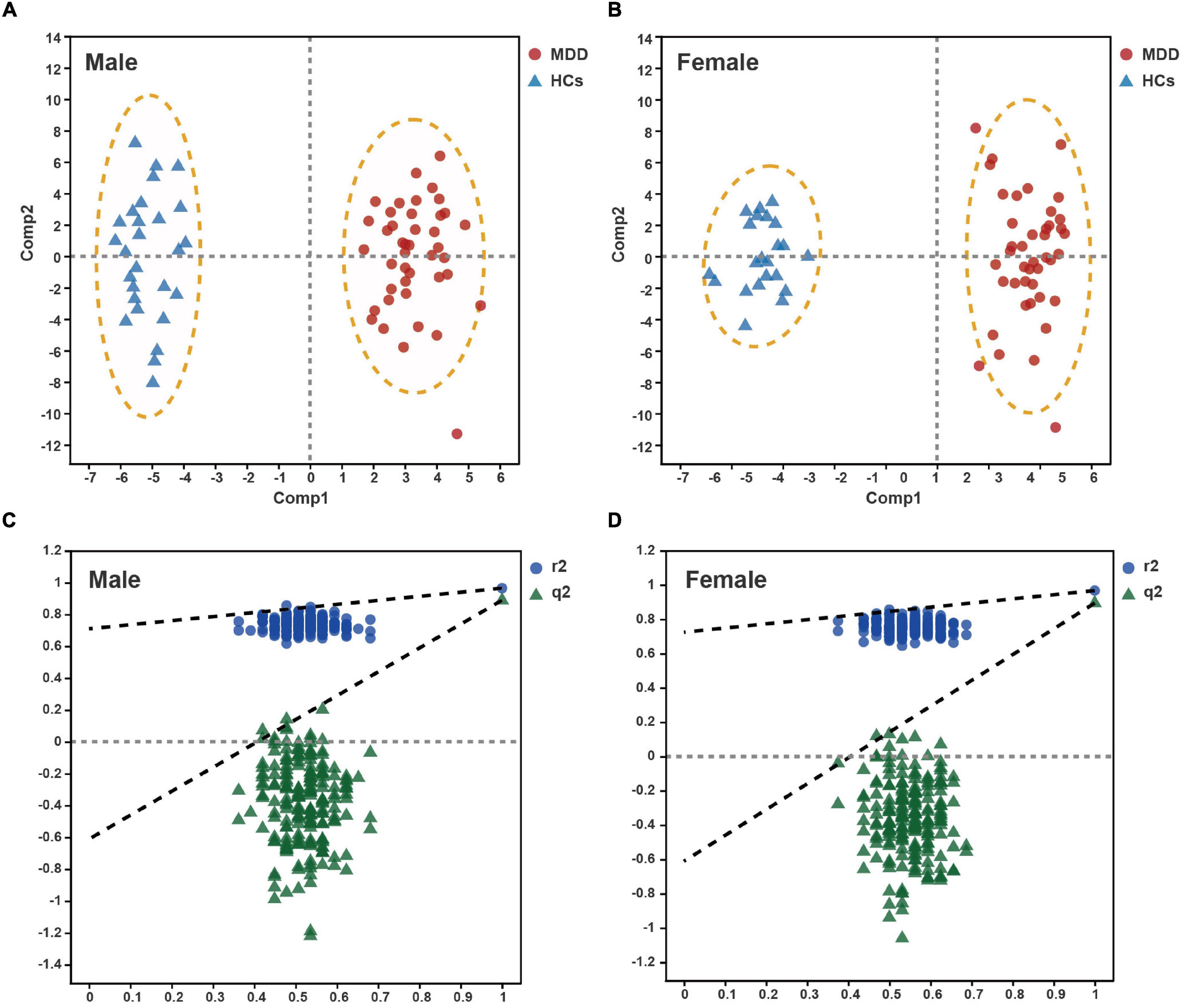
Figure 1. Metabolomic analysis of plasma from male and female patients with major depressive disorder (MDD) vs. healthy controls (HCs). Orthogonal partial least squares discriminate analysis (OPLS-DA) score plots displayed clear discrimination between male (A) and female (B) subjects with MDD from their respective HCs. Permutation test for male (C) and female (D) OPLS-DA models.
Pathway Analysis of Sex Differences in Altered Plasma Metabolites
To further explore the biological functions of these differential metabolites in both male and female sets, MetaboAnalyst 5.0 was performed. The pathways that were considerably affected are listed in Supplementary Table 1. In the male set, there were four significantly altered pathways (P-value < 0.05), including aminoacyl-tRNA biosynthesis, biosynthesis of unsaturated fatty acids, linoleic acid metabolism and alpha-linolenic acid metabolism (Figure 2A). In the female set, there were also four significantly altered pathways, including biosynthesis of unsaturated fatty acids, linoleic acid metabolism, alpha-linolenic acid metabolism and arginine and proline metabolism (Figure 2B). Among the three shared pathways, in comparison to HCs, both male and female subjects with MDD exhibited lower levels of fatty acids (FAs), such as palmitic acid and polyunsaturated fatty acids (PUFAs), including linoleic acid, arachidonic acid, eicosapentaenoic acid, alpha-linolenic acid, and phosphatidylcholine (PC) (16:0/18:2). Male subjects with MDD showed lower levels of L-phenylalanine, L-methionine and L-tryptophan, and higher levels of L-histidine, L-arginine and L-valine, in aminoacyl-tRNA biosynthesis. Female subjects with MDD had lower levels of creatine and D-proline, and a higher level of L-arginine, in arginine and proline metabolism.
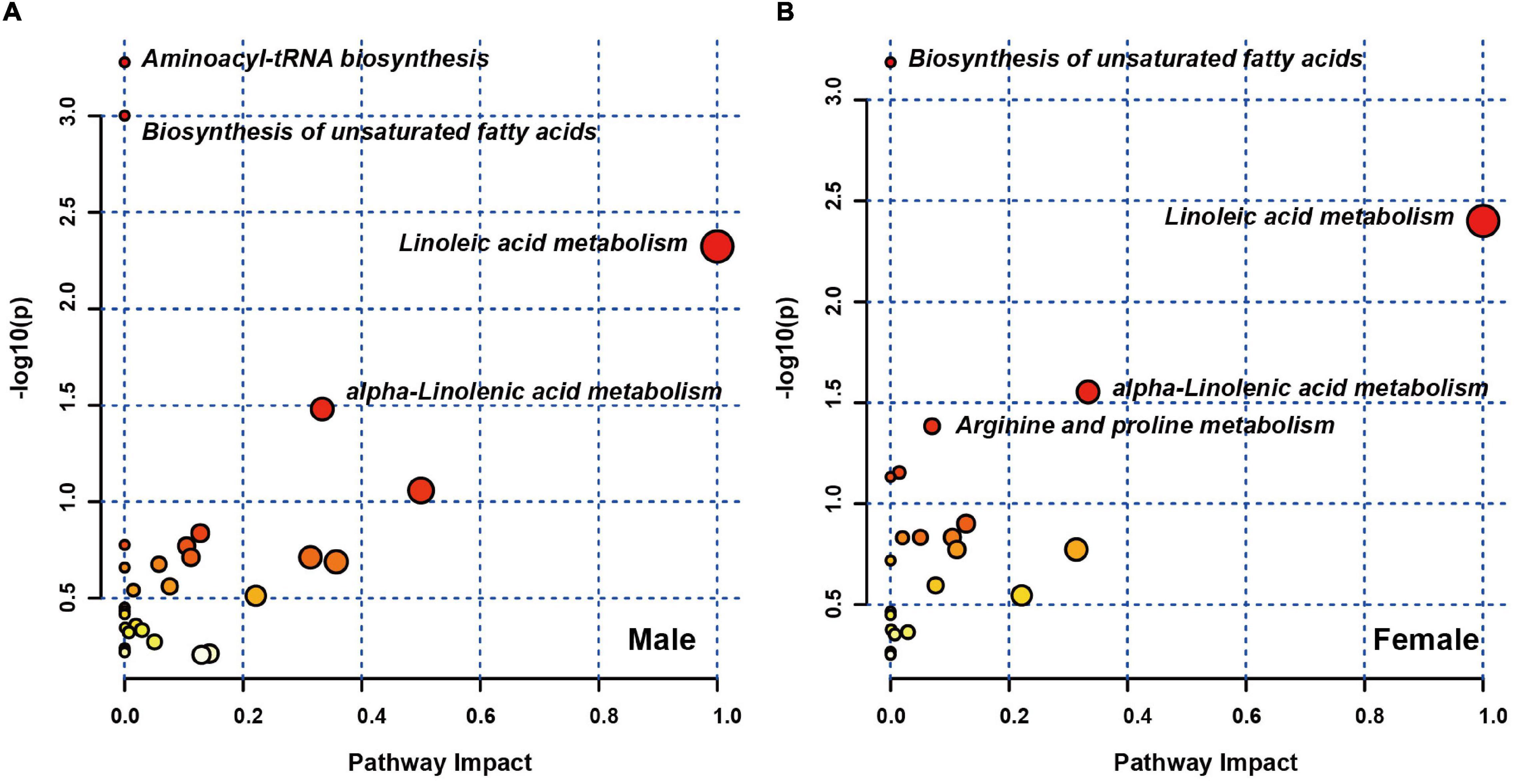
Figure 2. Pathway analysis of sex differences in differential plasma metabolites. Metabolic pathway analysis plot displaying the four affected metabolic pathways in male (A) and female (B) sets.
Simplified Sex-Specific Metabolite Biomarker Panel for Diagnosis of Major Depressive Disorder
In clinical practice, measuring 57 or 53 plasma metabolites simultaneously to diagnose MDD is inconvenient and costly. Considering that a diagnostic panel based on the quantification of a small number of parameters would be more feasible and cost-effective, random forest analysis was used to simplify candidate metabolite biomarkers, and to describe the significant deviations between the MDD and HC samples. For a sex-specific metabolite biomarker, we selected a metabolite with the highest importance value (Supplementary Table 2). We found that a male-specific biomarker panel including biliverdin could effectively capture the significant divergence between MDD and HCs. Receiver operating characteristic analysis was performed to quantify the diagnostic performance with an area under the curve (AUC) of 0.966 (Figure 3A; 95% confidence interval: 0.921–1; sensitivity: 88.9%; specificity: 97.6%). A female-specific biomarker panel that included PC (10:0/14:1) was shown to be effective in capturing the significant difference between MDD and HC samples, with an AUC of 0.957 (Figure 3B; 95% confidence interval: 0.896–1; sensitivity: 90.9%; specificity: 100.0%). Because an AUC of 0.5 indicates a valueless test and an AUC of 1.0 indicates an excellent test, these results suggest that the two plasma metabolite panels are good classifiers of male and female subjects with MDD for both children and adolescents.
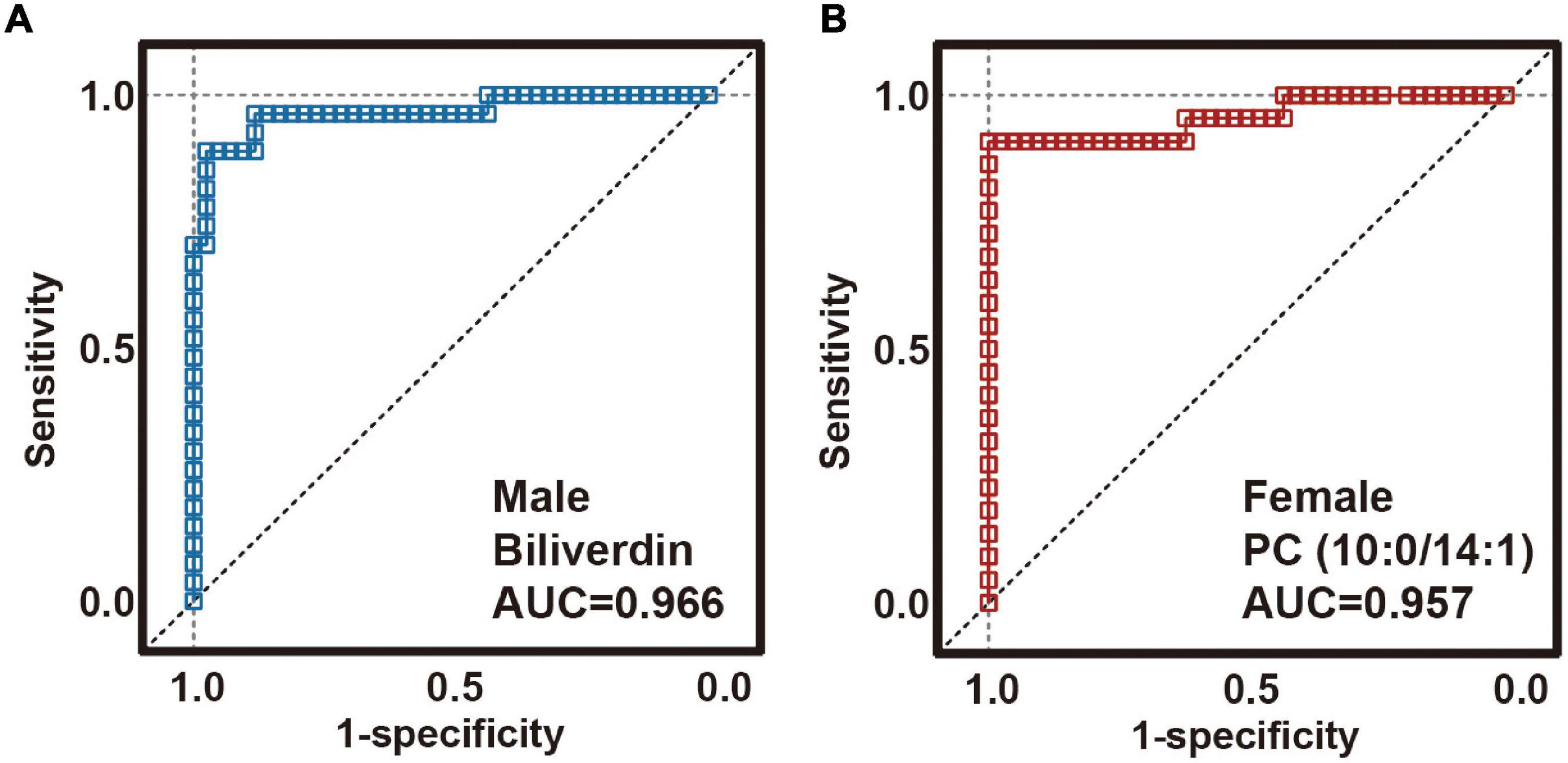
Figure 3. Diagnostic performance of sex-specific plasma biomarkers. Receiver operating characteristic (ROC) analysis showing outstanding diagnostic performances of these sex-specific biomarkers. (A) The area under the curve (AUC) of the male-specific biomarker was 0.966. (B) AUC of the female-specific biomarker was 0.966.
Discussion
To the best of our knowledge, this is the first investigation of potential sex-specific biomarkers of MDD in children and adolescents through the metabolic profiling of plasma samples. Here, the coefficient loading plots of the OPLS-DA model identified 57 and 53 differential metabolites that can discriminate male and female patients with MDD, respectively, from HCs. Results of pathway analysis showed that there were four significantly altered pathways in both male and female patients with MDD, three of which were common to both male and female patients. Finally, this study identified two panels of plasma metabolite biomarkers, one with biliverdin for males and the other with PC (10:0/14:1) for females, allowing MDD and HC samples to be distinguished effectively with an AUC of 0.966 (male) and 0.957 (female). These two panels may aid in the development of a plasma-based diagnostic test for males and females with MDD in children and adolescents, as well as give new insight into the pathophysiology of MDD in both males and females.
Previous studies have found gender differences in lipid and glucose metabolism between males and females in healthy individuals (26). There is accumulating evidence suggesting that the contribution of long-chain polyunsaturated fatty acids to the fatty acid composition of plasma lipids may be higher in females than in males (27). As for patients with MDD, in adults, there were sex-specific modules in plasma mainly involved in bile acid biosynthesis and the carnitine shuttle for males and in glycosphingolipid metabolism and tryptophan metabolism for females; additionally, the porphyrin metabolism was a common pathway in both sexes (17). And in urine, significantly affected metabolic pathways mainly involved in pyrimidine metabolism and synthesis and degradation of ketone bodies for women and taurine and hypotaurine metabolism and aminoacyl-tRNA biosynthesis for men (28). Here, by comparing boys and girls with MDD with their respective HCs, we found that the types of fatty acids (FAs) that changed in the plasma of females with MDD were not identical to those of males with MDD. In comparison to the healthy population, the levels of the same fatty acids in female patients decreased more than male patients, although girls also had higher levels of long-chain polyunsaturated fatty acids in their plasma than boys. Compared with adult patients, altered metabolic pathways in boys and girls with MDD are very different, which deserves to be further studied.
By investigating the plasma metabolite signature of subjects with MDD, we found that the female MDD metabolic signatures were significantly different from that in male. The results showed that 17 metabolites were found to be notably changed in male patients. For example, the decrease in stearoylcarnitine was only shown in boys with MDD. Previous studies in male mice investigated the influence of stearoylcarnitine on energy metabolism in a hippocampal extracellular flux analyzer, which may lead to depression (29). Whereas 13 metabolites were found to be changed only in female patients. For instance, the decrease in creatine was only shown in girls with MDD. Females have been reported to have 70–80% lower endogenous creatine stores than males, and consume significantly lower amounts of dietary creatine compared to males (30). Dietary creatine supplementation has been shown to be beneficial for antidepressant treatment, possibly providing an energizing effect in brain chemistry through the efficient regeneration of intracellular high-energy phosphates in females (31, 32). And forty metabolites were significantly altered in both males and females with MDD. The physiopathological heterogeneity of MDD was reflected in the relatively diverse function of these altered metabolites. MetaboAnalyst 5.0 was performed to examine the metabolic pathways significantly affected by these differential metabolites (33). In both male and female sets, there were three significantly altered pathways consisting of the biosynthesis of unsaturated fatty acids, linoleic acid metabolism and alpha-linolenic acid metabolism. Compared with HCs, concentrations of FAs (e.g., cis-9-palmitoleic acid, dodecanoic acid and palmitic acid) were lower in children and adolescents with MDD, and these FAs determine membrane fluidity and peroxidizability as the major components of neuronal membranes (34). In depression, FA metabolism and its interaction with the hypothalamic-pituitary-adrenal axis are disrupted (35). Also, depression is associated with unique FA compositions in certain brain locations (36). Given these findings, together with a decrease in carnitine (10:0) that promotes fatty acids oxidation, we theorized that child–adolescent patients with MDD would experience a decline in fatty acid β-oxidation. In addition, a reduction of some polyunsaturated fatty acids [PUFAs, e.g., linoleic acid, arachidonic acid, eicosapentaenoic acid, alpha-linolenic acid, and PC (16:0/18:2)] has been found and reported in other study (37). The levels of PUFAs, adenosine, hypoxanthine and inosine were considerably lower in child–adolescent patients with MDD, demonstrating that PUFAs may play a role in the pathophysiology of depression through modulating the purine metabolism pathway (38). In the etiology of depression, depletion of omega-3 PUFAs has previously been linked to inflammatory processes and lipid peroxidation (39).
Although some biological functions are the same in both males and females with MDD (e.g., biosynthesis of unsaturated fatty acids), there are different changes in other pathways. For males, aminoacyl-tRNA biosynthesis (e.g., L-histidine, L-phenylalanine, L-arginine, L-methionine, L-valine, and L-tryptophan) was a significantly altered metabolic pathway in the current study. Perturbation of the aminoacyl-tRNA biosynthesis in peripheral blood appears to be prominently associated with patients exhibiting MDD (40–42). Depletion of L-phenylalanine and its derivative DL-O-tyrosine causes a reduction in dopamine levels, which is linked to the occurrence of depression (43). In humans, the reaction between L-methionine and adenosine triphosphate produces S-adenosyl-L-methionine, which has antidepressant effects, and a decrease in methionine would reduce its effect (44). Accumulation of kynurenine, an intermediate metabolite of tryptophan, in the brain is linked to the development of depression (45). For the female set, arginine and proline metabolism (e.g., L-arginine, creatine, and D-proline) were altered in the present study. It was reported that decreased arginine levels led to increased arginase activity, which affects the severity of depression (46). Arginine and proline metabolism is associated with the nitric oxide (NO) cycle (47). High level of NO in the brain was associated with elevated levels of interleukin-6 and tumor necrosis factor-α, both of which can contribute to cognitive impairment through tau phosphorylation (48). Thus, arginine and proline metabolism may have a role in MDD by mediating the inflammatory response.
Because of the following limitations, the findings should be regarded with caution. First, this work reanalyzed the data from our published study, which contained 133 child–adolescent participants, and only one metabolite was identified to distinguish MDD from HCs in male or female set. Follow up studies should expand the sample size, particularly the number of healthy controls, and apply targeted metabolomics to validate the diagnostic efficacy of the two biomarkers. Second, both drug-naive and drug-treated MDD participants were enrolled in this study, and female MDD participants showed higher CDRS-R score, which may have the potential to influence metabolomics results. Third, this work analyzed the metabolites in plasma; however, we neglected to record blood routine test, liver function, kidney function, and blood lipids despite the subjects’ self-reported absence of somatic diseases, which might not better explain the results. Fourth, clinical locations in a single city (Chongqing, China) were investigated in the current study, and dietary disparities (including the lower body mass index of individuals in China) between China and European nations may have an impact on metabolomic data (49, 50). Multiple centers are needed to validate the diagnostic efficacy of these metabolite biomarkers in further studies. Last, because of the diverse physicochemical properties and widely varying concentrations of metabolites, future research should employ more metabolomics platforms and methods.
Conclusion
In conclusion, we identified different plasma metabolic profiles between male and female children and adolescents with MDD by a UPLC-Q-TOF/MS metabolomics approach. Seventeen metabolites were altered only in male patients with MDD, while 13 metabolites were altered only in female patients with MDD, and yet 40 metabolites changed in both. Four altered pathways were revealed by pathway analysis in male and female patients with MDD, three of which were shared. Sex-specific plasma metabolite biomarkers were identified for the diagnosis of MDD. Both male-specific (biliverdin) and female-specific [PC (10:0/14:1)] biomarkers were able to distinguish male and female subjects with MDD from their corresponding HCs. These results may serve as a valid diagnostic tool for male and female patients with MDD vs. healthy controls, and will facilitate future research into the different etiologies of MDD in male and female children and adolescents.
Data Availability Statement
The datasets generated and/or analyzed during the current study are not publicly available because the datasets relate to other unpublished projects, but are available from the corresponding author on reasonable request.
Ethics Statement
The studies involving human participants were reviewed and approved by Institutional Review Board of Children’s Hospital of Chongqing Medical University. Written informed consent to participate in this study was provided by the participants’ legal guardian/next of kin.
Author Contributions
PX, XZ, YJ, and MQ designed the research. YJ, MQ, TT, XL, and YY performed the research. YJ, MQ, JW, HW, and YH analyzed the data. YJ, MQ, TT, XZ, and PX drafted the manuscript. All authors contributed to the article and approved the submitted version.
Funding
This research was supported by the National Key Research and Development Program of China (Grant no. 2017YFA0505700), the Non-profit Central Research Institute Fund of Chinese Academy of Medical Sciences (Grant no. 2019PT320002), and the Natural Science Foundation Project of China (Grant no. 81820108015).
Conflict of Interest
The authors declare that the research was conducted in the absence of any commercial or financial relationships that could be construed as a potential conflict of interest.
Publisher’s Note
All claims expressed in this article are solely those of the authors and do not necessarily represent those of their affiliated organizations, or those of the publisher, the editors and the reviewers. Any product that may be evaluated in this article, or claim that may be made by its manufacturer, is not guaranteed or endorsed by the publisher.
Acknowledgments
Our sincere gratitude is extended to all the staff from the Children’s Hospital of Chongqing Medical University (Chongqing, China) and two schools (one primary school in Yuzhong district and one middle school in Liangping district, both in Chongqing, China) for their efforts in subject recruitment and sample collection.
Supplementary Material
The Supplementary Material for this article can be found online at: https://www.frontiersin.org/articles/10.3389/fpsyt.2022.929207/full#supplementary-material
Footnotes
References
1. World Health Organization [WHO]. Depression and Other Common Mental Disorders: Global Health Estimates. Geneva: World Health Organization (2017).
2. Ghandour RM, Sherman LJ, Vladutiu CJ, Ali MM, Lynch SE, Bitsko RH, et al. Prevalence and treatment of depression, anxiety, and conduct problems in US children. J Pediatr. (2019) 206:256–67.e3. doi: 10.1016/j.jpeds.2018.09.021
3. World Health Organization [WHO]. Health for the World’s Adolescents: A Second Chance in the Second Decade: Summary. Geneva: World Health Organization (2014).
4. Hawton K, van Heeringen K. Suicide. Lancet. (2009) 373:1372–81. doi: 10.1016/S0140-6736(09)60372-X
5. Bromet E, Andrade LH, Hwang I, Sampson NA, Alonso J, de Girolamo G, et al. Cross-national epidemiology of DSM-IV major depressive episode. BMC Med. (2011) 9:90. doi: 10.1186/1741-7015-9-90
6. Patten SB, Williams JV, Lavorato DH, Wang JL, Bulloch AG, Sajobi T. The association between major depression prevalence and sex becomes weaker with age. Soc Psychiatry Psychiatr Epidemiol. (2016) 51:203–10. doi: 10.1007/s00127-015-1166-3
7. Salk RH, Hyde JS, Abramson LY. Gender differences in depression in representative national samples: meta-analyses of diagnoses and symptoms. Psychol Bull. (2017) 143:783–822. doi: 10.1037/bul0000102
8. Herting MM, Gautam P, Spielberg JM, Kan E, Dahl RE, Sowell ER. The role of testosterone and estradiol in brain volume changes across adolescence: a longitudinal structural MRI study. Hum Brain Mapp. (2014) 35:5633–45. doi: 10.1002/hbm.22575
9. Copeland WE, Worthman C, Shanahan L, Costello EJ, Angold A. Early pubertal timing and testosterone associated with higher levels of adolescent depression in girls. J Am Acad Child Adolesc Psychiatry. (2019) 58:1197–206. doi: 10.1016/j.jaac.2019.02.007
10. Nelson EE, Leibenluft E, McClure EB, Pine DS. The social re-orientation of adolescence: a neuroscience perspective on the process and its relation to psychopathology. Psychol Med. (2005) 35:163–74. doi: 10.1017/s0033291704003915
11. Thapar A, Collishaw S, Pine DS, Thapar AK. Depression in adolescence. Lancet. (2012) 379:1056–67. doi: 10.1016/S0140-6736(11)60871-4
12. Nielsen J. Systems biology of metabolism. Annu Rev Biochem. (2017) 86:245–75. doi: 10.1146/annurev-biochem-061516-044757
13. Yang J, Chen T, Sun L, Zhao Z, Qi X, Zhou K, et al. Potential metabolite markers of schizophrenia. Mol Psychiatry. (2013) 18:67–78. doi: 10.1038/mp.2011.131
14. Lan MJ, McLoughlin GA, Griffin JL, Tsang TM, Huang JT, Yuan P, et al. Metabonomic analysis identifies molecular changes associated with the pathophysiology and drug treatment of bipolar disorder. Mol Psychiatry. (2009) 14:269–79. doi: 10.1038/sj.mp.4002130
15. Zheng P, Chen JJ, Huang T, Wang MJ, Wang Y, Dong MX, et al. A novel urinary metabolite signature for diagnosing major depressive disorder. J Proteome Res. (2013) 12:5904–11. doi: 10.1021/pr400939q
16. Zhou X, Liu L, Lan X, Cohen D, Zhang Y, Ravindran AV, et al. Polyunsaturated fatty acids metabolism, purine metabolism and inosine as potential independent diagnostic biomarkers for major depressive disorder in children and adolescents. Mol Psychiatry. (2019) 24:1478–88. doi: 10.1038/s41380-018-0047-z
17. Pu J, Liu X, Liu Y, Gui S, Zhong X, Tian L, et al. Sex-specific plasma metabolome signatures in major depressive disorder. Psychiatry Clin Neurosci. (2019) 73:713–4. doi: 10.1111/pcn.12925
18. Ciocan D, Cassard AM, Becquemont L, Verstuyft C, Voican CS, El Asmar K, et al. Blood microbiota and metabolomic signature of major depression before and after antidepressant treatment: a prospective case-control study. J Psychiatry Neurosci. (2021) 46:E358–68. doi: 10.1503/jpn.200159
19. Cipriani A, Zhou X, Del Giovane C, Hetrick SE, Qin B, Whittington C, et al. Comparative efficacy and tolerability of antidepressants for major depressive disorder in children and adolescents: a network meta-analysis. Lancet. (2016) 388:881–90. doi: 10.1016/S0140-6736(16)30385-3
20. Cipriani A, Furukawa TA, Salanti G, Chaimani A, Atkinson LZ, Ogawa Y, et al. Comparative efficacy and acceptability of 21 antidepressant drugs for the acute treatment of adults with major depressive disorder: a systematic review and network meta-analysis. Lancet. (2018) 391:1357–66. doi: 10.1016/S0140-6736(17)32802-7
21. Schmaal L, Hibar DP, Sämann PG, Hall GB, Baune BT, Jahanshad N, et al. Cortical abnormalities in adults and adolescents with major depression based on brain scans from 20 cohorts worldwide in the ENIGMA major depressive disorder working group. Mol Psychiatry. (2017) 22:900–9. doi: 10.1038/mp.2016.60
22. World Medical Association. World medical association declaration of helsinki. Ethical principles for medical research involving human subjects. Bull WHO. (2001) 79:373–4.
23. Poznanski E, Mokros H. Children’s Depression Rating Scale, Revised (CDRS-R). Los Angeles, CA: Western Psychological Services (1996).
25. Cumpston M, Li T, Page MJ, Chandler J, Welch VA, Higgins JP, et al. Updated guidance for trusted systematic reviews: a new edition of the cochrane handbook for systematic reviews of interventions. Cochrane Database Syst Rev. (2019) 10:Ed000142. doi: 10.1002/14651858.ED000142
26. Varlamov O, Bethea CL, Roberts CT Jr. Sex-specific differences in lipid and glucose metabolism. Front Endocrinol. (2014) 5:241. doi: 10.3389/fendo.2014.00241
27. Decsi T, Kennedy K. Sex-specific differences in essential fatty acid metabolism. Am J Clin Nutr. (2011) 94:1914s–9s. doi: 10.3945/ajcn.110.000893
28. Zheng P, Chen JJ, Zhou CJ, Zeng L, Li KW, Sun L, et al. Identification of sex-specific urinary biomarkers for major depressive disorder by combined application of NMR- and GC-MS-based metabonomics. Transl Psychiatry. (2016) 6:e955. doi: 10.1038/tp.2016.188
29. Aichler M, Borgmann D, Krumsiek J, Buck A, MacDonald PE, Fox JEM, et al. N-acyl taurines and acylcarnitines cause an imbalance in insulin synthesis and secretion provoking β cell dysfunction in type 2 diabetes. Cell Metab. (2017) 25:1334–47.e4. doi: 10.1016/j.cmet.2017.04.012
30. Brosnan JT, Brosnan ME. Creatine: endogenous metabolite, dietary, and therapeutic supplement. Annu Rev Nutr. (2007) 27:241–61. doi: 10.1146/annurev.nutr.27.061406.093621
31. Renshaw PF, Parow AM, Hirashima F, Ke Y, Moore CM, Frederick Bde B, et al. Multinuclear magnetic resonance spectroscopy studies of brain purines in major depression. Am J Psychiatry. (2001) 158:2048–55. doi: 10.1176/appi.ajp.158.12.2048
32. Kondo DG, Sung YH, Hellem TL, Fiedler KK, Shi X, Jeong EK, et al. Open-label adjunctive creatine for female adolescents with SSRI-resistant major depressive disorder: a 31-phosphorus magnetic resonance spectroscopy study. J Affect Disord. (2011) 135:354–61. doi: 10.1016/j.jad.2011.07.010
33. Pang Z, Chong J, Zhou G, de Lima Morais DA, Chang L, Barrette M, et al. MetaboAnalyst 5.0: narrowing the gap between raw spectra and functional insights. Nucleic Acids Res. (2021) 49:W388–96. doi: 10.1093/nar/gkab382
34. Bazinet RP, Layé S. Polyunsaturated fatty acids and their metabolites in brain function and disease. Nat Rev Neurosci. (2014) 15:771–85. doi: 10.1038/nrn3820
35. Mocking RJ, Verburg HF, Westerink AM, Assies J, Vaz FM, Koeter MW, et al. Fatty acid metabolism and its longitudinal relationship with the hypothalamic-pituitary-adrenal axis in major depression: associations with prospective antidepressant response. Psychoneuroendocrinology. (2015) 59:1–13. doi: 10.1016/j.psyneuen.2015.04.027
36. Assies J, Mocking RJ, Lok A, Ruhé HG, Pouwer F, Schene AH. Effects of oxidative stress on fatty acid- and one-carbon-metabolism in psychiatric and cardiovascular disease comorbidity. Acta Psychiatr Scand. (2014) 130:163–80. doi: 10.1111/acps.12265
37. Tsuchimine S, Saito M, Kaneko S, Yasui-Furukori N. Decreased serum levels of polyunsaturated fatty acids and folate, but not brain-derived neurotrophic factor, in childhood and adolescent females with depression. Psychiatry Res. (2015) 225:187–90.
38. Mizushina Y, Dairaku I, Yanaka N, Takeuchi T, Ishimaru C, Sugawara F, et al. Inhibitory action of polyunsaturated fatty acids on IMP dehydrogenase. Biochimie. (2007) 89:581–90. doi: 10.1016/j.biochi.2007.01.009
39. Owen C, Rees AM, Parker G. The role of fatty acids in the development and treatment of mood disorders. Curr Opin Psychiatry. (2008) 21:19–24.
41. Francklyn CS, Mullen P. Progress and challenges in aminoacyl-tRNA synthetase-based therapeutics. J Biol Chem. (2019) 294:5365–85. doi: 10.1074/jbc.REV118.002956
42. Yang J, Yan B, Zhao B, Fan Y, He X, Yang L, et al. Assessing the causal effects of human serum metabolites on 5 major psychiatric disorders. Schizophr Bull. (2020) 46:804–13.
43. Roiser JP, McLean A, Ogilvie AD, Blackwell AD, Bamber DJ, Goodyer I, et al. The subjective and cognitive effects of acute phenylalanine and tyrosine depletion in patients recovered from depression. Neuropsychopharmacology. (2005) 30:775–85. doi: 10.1038/sj.npp.1300659
44. Galizia I, Oldani L, Macritchie K, Amari E, Dougall D, Jones TN, et al. S-adenosyl methionine (SAMe) for depression in adults. Cochrane Database Syst Rev. (2016) 10:CD011286.
45. Chen Y, Guillemin GJ. Kynurenine pathway metabolites in humans: disease and healthy States. Int J Tryptophan Res. (2009) 2:1–19. doi: 10.4137/IJTR.S2097
46. Baranyi A, Amouzadeh-Ghadikolai O, Rothenhäusler HB, Theokas S, Robier C, Baranyi M, et al. Nitric oxide-related biological pathways in patients with major depression. PLoS One. (2015) 10:e0143397. doi: 10.1371/journal.pone.0143397
47. Morris SM Jr. Arginine: beyond protein. Am J Clin Nutr. (2006) 83:508s–12s. doi: 10.1093/ajcn/83.2.508S
48. Faraco G, Hochrainer K, Segarra SG, Schaeffer S, Santisteban MM, Menon A, et al. Dietary salt promotes cognitive impairment through tau phosphorylation. Nature. (2019) 574:686–90. doi: 10.1038/s41586-019-1688-z
49. O’Sullivan A, Gibney MJ, Brennan L. Dietary intake patterns are reflected in metabolomic profiles: potential role in dietary assessment studies. Am J Clin Nutr. (2011) 93:314–21. doi: 10.3945/ajcn.110.000950
Keywords: major depressive disorder, children and adolescents, sex specific, biomarker, metabolomics, plasma
Citation: Jiang Y, Qin M, Teng T, Li X, Yu Y, Wang J, Wu H, He Y, Zhou X and Xie P (2022) Identification of Sex-Specific Plasma Biomarkers Using Metabolomics for Major Depressive Disorder in Children and Adolescents. Front. Psychiatry 13:929207. doi: 10.3389/fpsyt.2022.929207
Received: 26 April 2022; Accepted: 22 June 2022;
Published: 14 July 2022.
Edited by:
Li Yang, Peking University Sixth Hospital, ChinaReviewed by:
Yang Chen, Peking University, ChinaYiping Tang, Taizhou Second People’s Hospital, China
Copyright © 2022 Jiang, Qin, Teng, Li, Yu, Wang, Wu, He, Zhou and Xie. This is an open-access article distributed under the terms of the Creative Commons Attribution License (CC BY). The use, distribution or reproduction in other forums is permitted, provided the original author(s) and the copyright owner(s) are credited and that the original publication in this journal is cited, in accordance with accepted academic practice. No use, distribution or reproduction is permitted which does not comply with these terms.
*Correspondence: Xinyu Zhou, emhvdXhpbnl1QGNxbXUuZWR1LmNu; Peng Xie, eGllcGVuZ0BjcW11LmVkdS5jbg==
†These authors have contributed equally to this work and share first authorship