- 1Department of Psychiatry, Chang’an Hospital, Xi’an, China
- 2Department of Psychiatry, Xijing Hospital, Air Force Medical University, Xi’an, China
Lipidomics has been established as a potential tool for the investigation of mental diseases. However, the composition analysis and the comparison of the peripheral lipids regarding adult women with major depressive depression (MDD) or bipolar depression (BPD) has been poorly addressed. In the present study, age-matched female individuals with MDD (n = 28), BPD (n = 22) and healthy controls (HC, n = 25) were enrolled. Clinical symptoms were assessed and the plasma samples were analyzed by comprehensive lipid profiling based on liquid chromatography-mass spectrometry (LC/MS). We found that the composition of lipids was remarkably changed in the patients with MDD and BPD when compared to HC or compared to each other. Moreover, we identified diagnostic potential biomarkers comprising 20 lipids that can distinguish MDD from HC (area under the curve, AUC = 0.897) and 8 lipids that can distinguish BPD from HC (AUC = 0.784), as well as 13 lipids were identified to distinguish MDD from BPD with moderate reliability (AUC = 0.860). This study provides further understanding of abnormal lipid metabolism in adult women with MDD and BPD and may develop lipid classifiers able to effectively discriminate MDD from BPD and HC.
Introduction
Major depressive disorder (MDD) and bipolar disorder (BD) are highly prevalent affective disorders, leading to increased adverse sequelae of other common comorbid medical conditions and suicide risk and a heavy social burden (1–3). The lifetime prevalence of MDD is evaluated to be around 20% and is predicted to be the leading cause of global disability by the year 2030 according to the World Health Organization (4, 5), whereas the lifetime prevalence range of BD is from 0.5 to 2.4% worldwide (6, 7). Although hypomania and mania are the most recognizable characteristics, patients with BD were hypomanic or manic less than 10% of the time, and depression is its most frequent clinical presentation (8, 9). Meanwhile, the overlapping symptomology between MDD and bipolar depression (BPD) is very common (10, 11). Although the pharmacotherapeutic strategies in these two disorders are substantially different (12, 13), patients with BPD are often initially misdiagnosed with depression (14). In addition, diagnosis may change from depression to BPD over the long-term disease progression (15), which may lead to inappropriate treatment and greater healthcare costs (16). Meanwhile, the benefit of drug treatment is still unsatisfactory for patients even with a definite diagnosis. For instance, the summary effect sizes of antidepressants are mostly modest (17) and approximately 30% of MDD do not respond to standard pharmacological therapies (18). On the other hand, the efficiency of drug treatments for BD is about only 40–60% and usually causes side effects (19, 20). As a result, the underlying molecular basis and correct diagnosis for MDD and BPD remain largely concerned (21, 22).
Women are nearly twice as likely as men to develop depression during their lifetime (23, 24) and women with MDD also report greater illness severity and increased suicide attempts than men (25–27). On the other hand, although there is no gender difference in the incidence rate of BD, women are more likely to have depressive episodes and bring more burden of disease than men (28–30) and are at high risk of having a serious episode of illness during childbirth and pregnancy (31, 32). However, the objective biomarkers that can distinguish women with MDD from BD have not been identified. Thus, clarifying the pathological features of MDD and BD in women might be able to provide a basis for the development of new diagnostic methods and treatment strategies.
The use of peripheral blood as the sample source for identifying diagnostic, prognostic, and predictive biomarkers for MDD and BD has been already reported (33–35). Correspondingly, lipids from peripheral blood already represent a target for preventive medicine for MDD and BD (36). Recently, lipidomics analyses have also demonstrated the role of serum lipid abnormalities in BD and MDD (37, 38). For example, targeted lipidomics reveals that high plasma ceramides in patients with MDD and BD are indicative of a high metabolic burden (39). Although previous plasma lipidomics analyses have revealed the characteristic lipid molecules of antenatal depression and female bipolar disorder (40, 41), thus far, there are only limited understandings of the role of lipids in the pathophysiology and the direct comparison of lipid compositions between adult MDD and BPD in women has not been reported. Such investigations might shed a light on the identifications of peripheral biomarkers for these two disorders.
Considering the above, the present study performed a case-control study of lipidomic analysis of plasma samples (n = 75) from age-matched female individuals with MDD (n = 28) and BPD (n = 22) compared with healthy controls (HC, n = 25) by using liquid chromatography-mass spectrometry (LC/MS). Next, we identified female MDD- and BPD-related lipidomic signatures compared to HC by orthogonal partial least-squares discrimination analysis (OPLS-DA) as well as discriminative lipids panels that could distinguish MDD, BPD, and HC by random forest and receiver operating characteristic (ROC) analysis. Finally, correlation analysis based on the relative abundance of altered lipids and clinical symptom scores was performed to explore the potential lipid function.
Materials and Methods
Subjects and Plasma Sampling
In total, 28 female patients who met the DSM-5 criteria for MDD (24–46 years of age) or 22 female patients for BPD (26–58 years of age) were recruited from the Department of Psychiatry at Chang’an Hospital, Xi’an, China, along with 25 healthy female (25–53 years of age) individuals, all of which were Han nationality and underwent a physical examination. The Mini-International Neuropsychiatric Interview was used to screen for pre-existing psychiatric disorders. Diagnosis of MDD and BPD was performed by two senior psychiatrists based on a Structured Clinical Interview for Diagnostic and Statistical Manual of Mental Disorders, 5th Edition’s (DSM-5) criteria. All the patients with BPD were recruited with a current depressive episode. Meanwhile, psychiatrists who had attended a training session on how to administer the tests before the start of the study and were blinded to the clinical status of the participants evaluated the clinical symptoms by the Hamilton Anxiety Scale (HAM-A), Hamilton Depression Rating Scale (HAM-D) and Positive and Negative Syndrome Scale (PANSS) scores. The exclusion criteria include: patients with inflammatory bowel disease, Crohn’s disease or other diseases of the digestive system, patients with other organic diseases; hypertension; obesity, defined as a body-mass index (BMI) ≥ 28.0; pregnancy, lactation or menstrual period; severely unbalanced diet, such as high-fat diet or long-term vegetarians; the presence of other mental disorders according to the DSM-5 criteria.
Blood samples were collected in anticoagulant tubes and centrifuged at 1,600 rpm for 15 min, and then aliquoted into sterile cryopreservation tubes and stored in liquid nitrogen until analysis. Blood samples were collected between 8 and 10 a.m. with all individuals under fasting conditions.
Lipidomics Analyses
The lipidomics analyses were supported by Shanghai Applied Protein Technology Co., Ltd., which were performed as previously described (42, 43). Briefly, plasma (100 μL) was spiked with internal lipid standards (SPLASH® LIPIDOMIX® Mass Spec Standard, methanol solution, AVANTI, 330707-1EA, Merck, United States) and homogenized with water and methanol, then 800 μL of methyl tert-butyl ether (MTBE, Thermo Fisher, United States) was added. After centrifuging, the supernatant was separated for analysis. For LC-MS, samples were separated by a UHPLC Nexera LC-30A ultra-high-performance liquid chromatography system with a C18 column (ACQUITY UPLC CSH C18, 1.7 μm, 2.1 mm × 100 mm, Waters, United States). The lipid extracts were re-dissolved in 200 μL 90% isopropanol/acetonitrile (Thermo Fisher Scientific, United States), centrifuged at 14,000 g for 15 min, and finally, 3 μL of the sample was injected. Mass spectra were acquired by Q-Exactive Plus (Thermo Fisher Scientific, United States) in positive and negative modes, respectively. Electrospray ionization (ESI) parameters were optimized and preset for all measurements as follows: Source temperature, 300°C; capillary temperature, 350°C, the ion spray voltage was set at 3000V, S-Lens RF Level was set at 50% and the scan range of the instruments was set at m/z 200–1,800. Identification of lipids was performed by LipidSearch™ software (Thermo Fisher Scientific, United States), which contains more than 30 lipid classes and more than 1,500,000 fragment ions in the database. Single-point internal standard calibrations were used to estimate absolute concentrations for unique lipids identified by accurate mass, MS/MS spectral match, and retention times (44).
Statistical Analyses
Differences in continuous variables were assessed with the one-way Analysis of Variance (ANOVA) or Kruskal Wallis test. The data of lipids was further converted through log10 and standardized by Pareto scaling. Then the data quality was evaluated by the partial least squares discriminant analysis (PLS-DA) and the orthogonal partial least squares discriminant analysis (OPLS-DA) method was applied to remove irrelevant variables. The quality of the OPLS-DA was validated by the permutation test.
In addition, the contribution of variables was evaluated by the variable importance in the projection (VIP) values and the student’s t-test which was followed by the adjustment of the false discovery rate (FDR) by multiple hypothesis tests. The differential lipids were determined by a combination of FDR, fold change (FC) and VIP values, and a 10-fold cross-validation method was used to verify the accuracy of important lipids. Moreover, the correlation between the clinical parameters and lipid concentrations was analyzed by Pearson correlation. The potential biomarkers were evaluated by receiver operating characteristic (ROC) analysis after transformation by a logistic regression model to the predicted probability scale.
Results
Clinical Characteristics of the Recruited Participants
A total of 75 individuals were included in this study. No significant difference was found among the three groups in terms of age (P = 0.239) and BMI (P = 0.693). The scale score of HAMD, HAMA, and PANSS in MDD and BPD groups were higher than those of HC group (Table 1), and a significant difference in score of HAMA was also observed between MDD and BPD groups. Moreover, duloxetine and venlafaxine are the main therapeutic drugs used in MDD group and olanzapine or quetiapine combined with lithium or valproate are the main therapeutic drugs used in BPD group. It is worth noting that the recruited participants did not or occasionally drink alcohol and smoke. Detailed clinical and demographic characteristics of the studied samples are displayed in Supplementary Table 1.
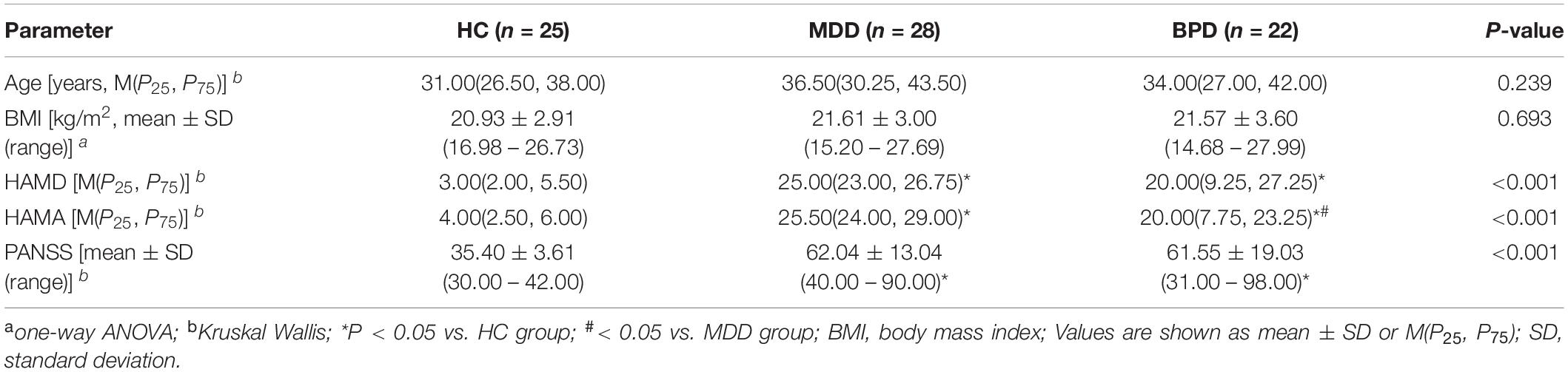
Table 1. Comparison of clinical characteristics data and symptom scale assessment among the three groups.
Alternation of Lipid at Class Level and Differential Carbon Chain Lengths and Degree of Saturation of Fatty Acyls in Major Depressive Disorder and Bipolar Depression
A total of 31 lipid classes and 884 lipid species were identified in the samples of each group (Supplementary Table 2). Lipidomic analyses revealed that there are significant differences on the levels of several classes including wax esters (WE, H = 8.789, P = 0.012) and acylcarnitine (AcCa, H = 35.991, P < 0.001) (Figure 1A), monogalactosyldiacylglycerol (MGDG, F = 9.030, P < 0.001) and coenzymeQ10 (Co, H = 10.578, P = 0.005) (Figure 1B), sphingomyelin (SM, H = 9.671, P = 0.008), phytosphingomyelin (phSM, F = 4.995, P = 0.010), CeramideG2GNAc1 (Cer G2GNAc1 H = 8.795, P = 0.012) and GM2 (H = 25.646, P < 0.001) (Figure 1C), triglyceride (TG, H = 7.790, P = 0.020) (Figure 1D), phosphatidylserine (PS, F = 11.727, P < 0.001), phosphatidylethanolamine (PE, F = 9.885, P < 0.001), lysophosphatidylethanolamine (LPE, F = 33.952, P < 0.001), lysophosphatidylinositol (LPI, H = 13.570, P = 0.001), phosphatidylinositol (PIP, H = 15.034, P = 0.001) and phosphatidylinositol 4,5-bisphosphate (PIP2, H = 11.919, P = 0.003) (Figure 1E) among MDD, BPD and HC. Intercomparison further showed that levels of MGDG, phSM, CerG2GNAc1, AcCa and PS were decreased while GM2 and PIP were increased in MDD when compared with HC. Meanwhile, levels of MGDG, Co, SM, phSM, WE, AcCa, PS, and PE were decreased while GD2, GM2, TG, LPE, PIP, and PIP2 were increased in BPD when compared with HC. Moreover, levels of LPE and LPI were decreased while PE were increased in MDD when compared with BPD.
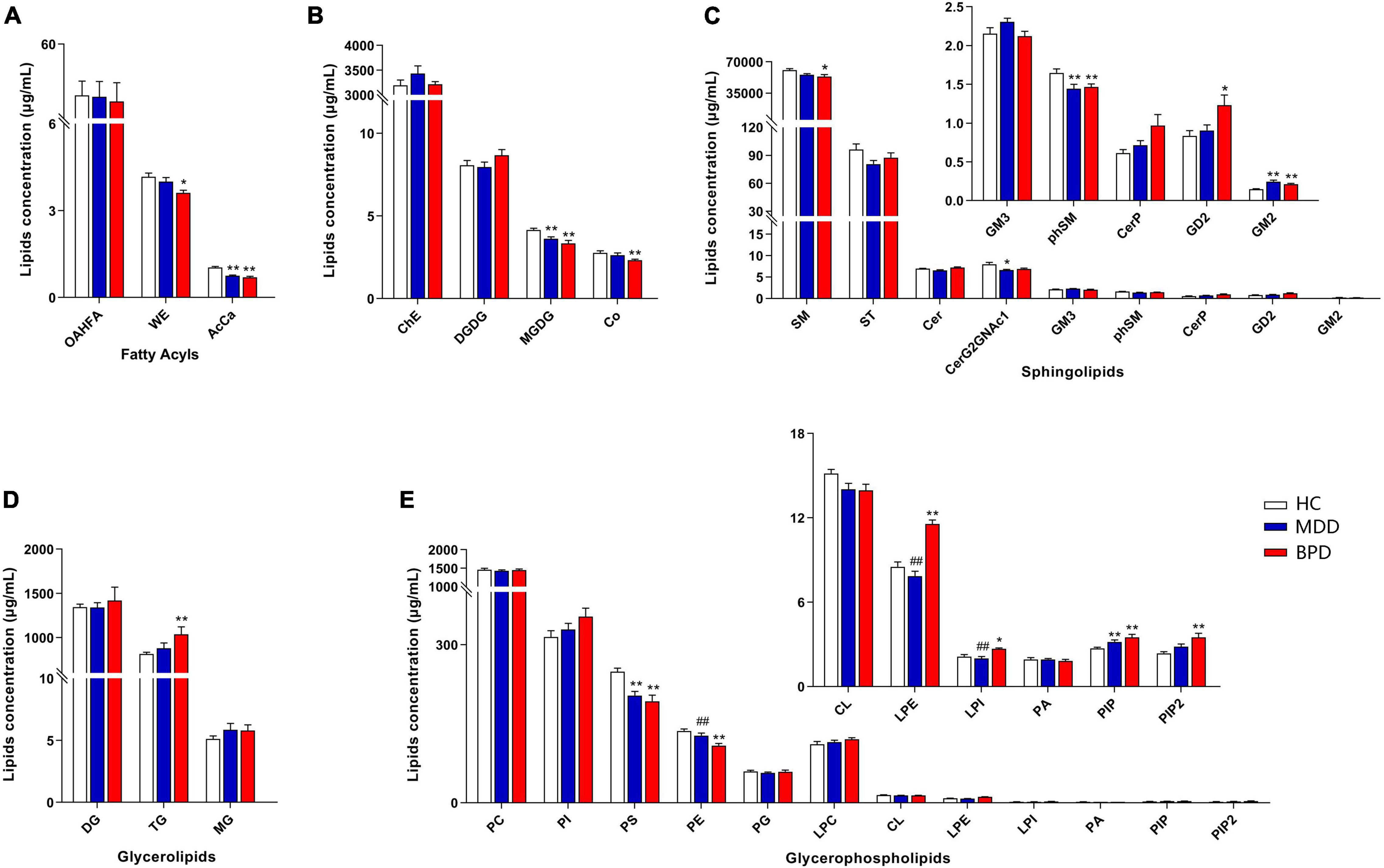
Figure 1. Differential concentration of lipid class among major depressive disorder (MDD), bipolar depression (BPD), and healthy controls (HC). (A) Fatty acyls, (B) ChE, Co, DGDG, and MGDG, (C) sphingolipids, (D) glycerolipids, (E) glycerophospholipids. HC, healthy controls; MDD, major depressive disorder; BPD, bipolar depression; OAHFA, (O-acyl)-1-hydroxy fatty acid; WE, wax esters; AcCa, acylcarnitine; ChE, cholesterol ester; Co, coenzyme; DGDG, digalactosyldiacylglycerol; MGDG, monogalactosyldiacylglycerol; SM, sphingomyelin; ST, sulfatide; Cer, ceramides; CerP, ceramides phosphate; phSM, phytosphingomyelin; DG, diglyceride; TG, triglyceride; MG, monoglyceride; PC, phosphatidylcholine; PI, phosphatidylinositol; PS, phosphatidylserine; PE, phosphatidylethanolamine; PG, phosphatidylglycerol; CL, cardiolipin; LPC, lysophosphatidylcholine; LPE, lysophosphatidylethanolamine; LPI, lysophosphatidylinositol; PA, phosphatidic acid; PIP, phosphatidylinositol; PIP2, phosphatidylinositol 4,5-bisphosphate. *P < 0.05 vs. HC; **P < 0.01 vs. HC, ##P < 0.01 vs. BPD.
To further investigate the difference in lipid composition, we compared the concentrations of lipids with different carbon chain lengths and the degree of saturation of the fatty acyls among MDD, BPD, and HC. As shown in Figure 2, we observed significant alterations in the fatty acyl chain profile of lipids in the plasma. Levels of long-chain fatty acyls with 40 carbons (40C) and levels of saturated fatty acid, polyunsaturated fatty acyls with 5 double bonds and 9 double bonds were decreased, while levels of long-chain fatty acyls with 16 carbons (16C) were increased in both the MDD (MDD vs. HC, P < 0.05) and the BPD group (BPD vs. HC, P < 0.05). Meanwhile, levels of long-chain fatty acyls with 26 carbons (26C) were increased, while level of long-chain fatty acyls with 28 carbons (28C) was decreased in the MDD group (MDD vs. HC, P < 0.05). Levels of long-chain fatty acyls with 41 carbons (41C), 37 carbons (37C), 35 carbons (35C), 33 carbons (33C) and polyunsaturated fatty acyls with 1 double bond, 4 double bonds and 8 double bonds were decreased, while levels of long-chain fatty acyls more 47 carbons (>47C) and polyunsaturated fatty acyls more than 9 double bonds were increased in the BPD group (BPD vs. HC, P < 0.05). Notably, a significant difference in the levels of long-chain fatty acyls with 26 carbons (26C), 22 carbons (22C), 37 carbons (37C), and polyunsaturated fatty acyls with 8 double bonds were also observed between the MDD and BPD group (MDD vs. BPD, P < 0.05). Taken together, there were obvious changes in lipid composition not only at lipid class level but also in the structure of fatty acyls among the three groups.
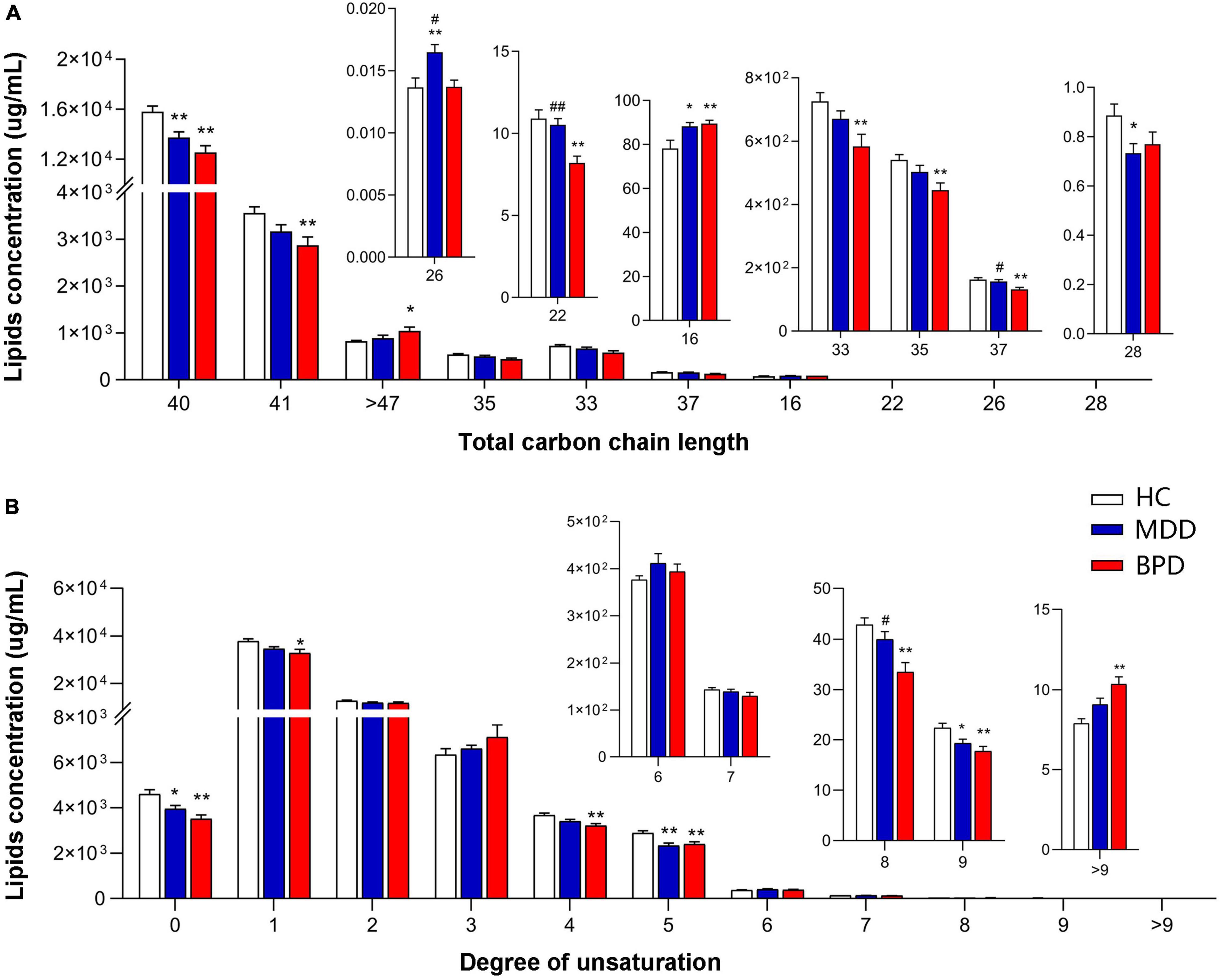
Figure 2. Groupwise alterations in fatty acid composition in the plasma. Results of analysis of fatty acyl composition by (A) chain length (number of carbons) and (B) degree of unsaturation. Lipids showing low levels results have been enlarged in insets. HC, health control; MDD, major depressive disorder; BPD, bipolar depression; *P < 0.05 vs. HC; **P < 0.01 vs. HC; #P < 0.05 vs. BD; ##P < 0.01 vs. BPD.
Characteristic Lipid Species Between Major Depressive Disorder and Healthy Controls
According to the characteristics of the OPLS-DA model, the data of plasma lipids could be well distinguished between HC and MDD (Figure 3A). The OPLS-DA model was reliable to screen lipid biomarkers between HC and MDD (Figure 3B). The differential lipids were determined based on the following criteria: (1) FC > 1.5 or < 0.067, (2) VIP > 1, and (3) FDR value < 0.05. A total of 66 differential lipid species were identified (36 up-regulated and 30 down-regulated in MDD) between MDD and HC (Figure 3C and Supplementary Table 3). Meanwhile, the above molecules were selected to construct a random forest model, and 10-fold cross-validation showed that the lipids with the top 20 importance had the lowest model error rate (Figure 3D). These 20 lipids covered 7 different lipid class and showed good sensitivity and specificity [AUC = 0.897, 95% CI (0.807, 0.978)] (Figure 3E). Moreover, among these characteristic species, levels of Cer(d15:1/25:2), Cer(d18:1/16:0), Cer(d18:1/24:0), Cer(d40:1), Cer(d41:1 + O), Cer(m34:0 + O), Cer(m38:2 + O) and PI(16:1) were negatively while levels of Cer(d32:4), CerG2GNAc1(d32:1), CerG2GNAc1(d36:1), CerG2GNAc1(d38:4), GM2(d34:5), GM3(t39:6), PC(18:0/16:0), PC(20:1e/18:2), PC(26:2e), PC(40:10), TG(18:1/18:2/22:4) and TG(20:0/18:1/18:1) were positively correlated with the scores of HAMA, HAMD and PANSS (Figure 3F), indicating those lipids in the plasma might be a combinational biomarker for women with MDD.
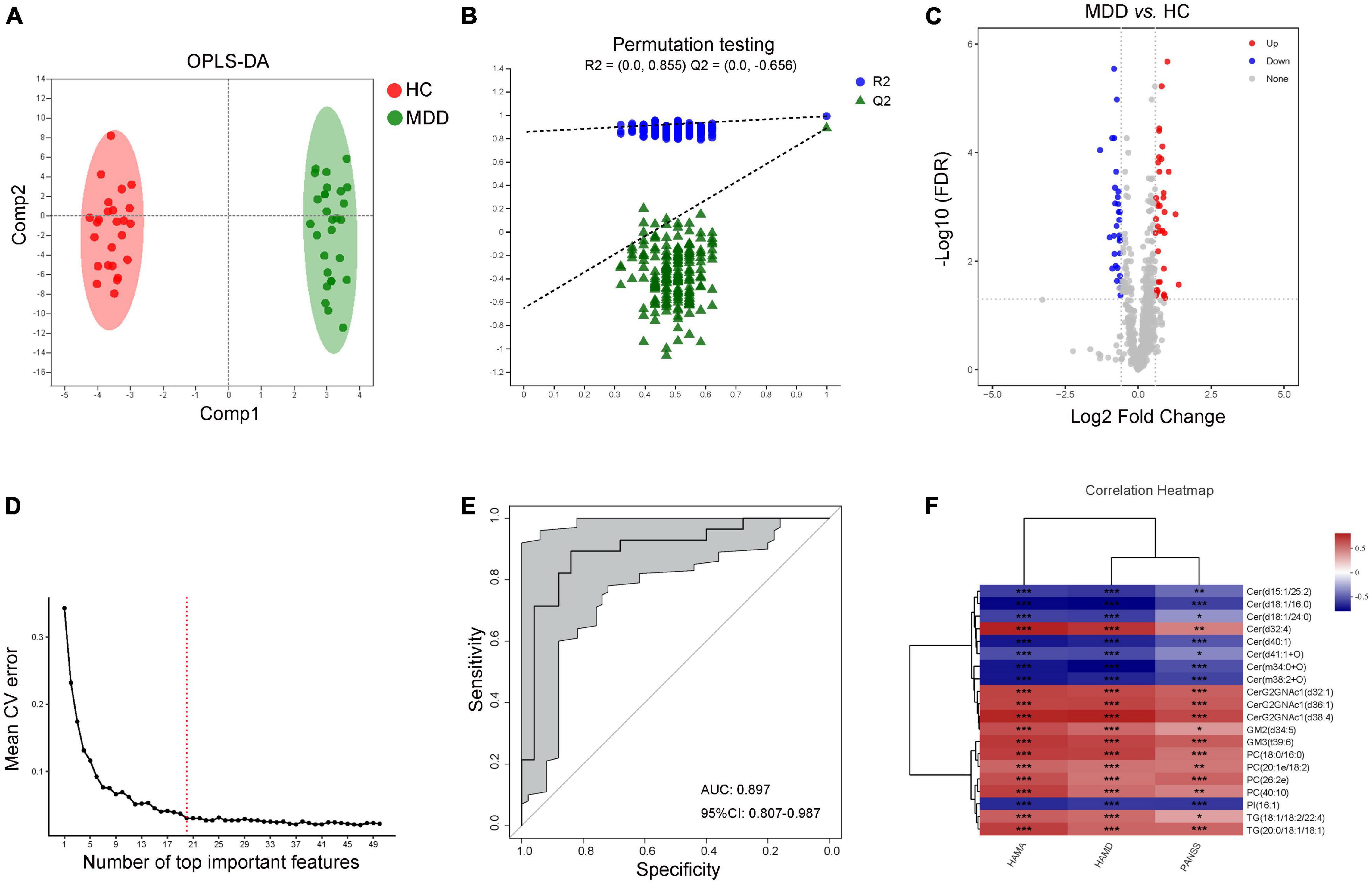
Figure 3. Characteristic lipid species in MDD. (A) Scatter plot of OPLS-DA model and (B) validation model of permutation test, (C) volcano map reveals the increased (red dots) and decreased (blue dots) lipid species between the MDD and HC, (D) random forest model and 10-fold cross-validation showed that the lipids with the top 20 importance had the lowest model error rate, (E) ROC analysis for the combinational lipids, (F) correlation between clinical parameters and levels of 20 lipid species. *P < 0.05; **P < 0.01, ***P < 0.001.
Characteristic Lipid Species Between Bipolar Depression and Healthy Controls
Data of plasma lipids could also be well distinguished and reliable to screen lipid biomarkers between HC and BPD (Figures 4A,B). A total of 172 differential lipid species were identified (138 up-regulated and 34 down-regulated in BPD) between BPD and HC (Figure 4C and Supplementary Table 4). 10-fold cross-validation further showed that the lipids with the top 8 importance had the lowest model error rate (Figure 4D). These 8 lipids covered 4 different lipid class and showed good sensitivity and specificity [AUC = 0.784, 95% CI (0.642, 0.925)] (Figure 4E). Moreover, among these characteristic species, levels of PC(36:6e) and PS(42:9e) were negative while levels of LPE(16:0), LPE(18:0), PC(38:8), PC(8:0e/6:0) and TG(22:4/17:1/18:2) were positively correlated with the scores of HAMA, HAMD and PANSS (Figure 4F). Meanwhile, levels of TG(16:0/16:1/22:6) were also positively correlated with the scores of HAMA and HAMD. Taken together, these 8 lipids might be a combinational plasma biomarker for women with BPD.
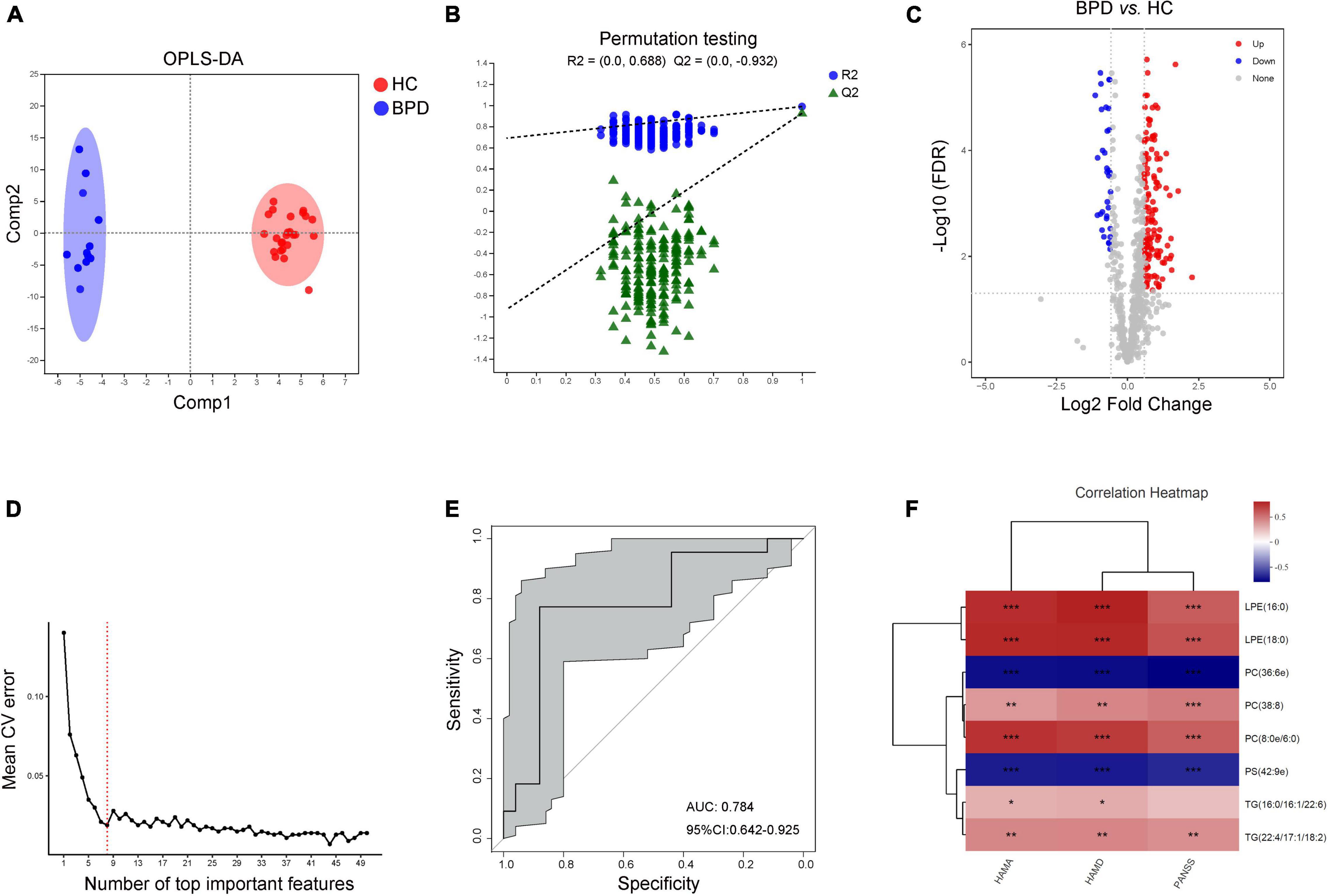
Figure 4. Characteristic lipid species in BPD. (A) Scatter plot of OPLS-DA model and (B) validation model of permutation test, (C) volcano map reveals the increased (red dots) and decreased (blue dots) lipid species between the BPD and HC, (D) random forest model and 10-fold cross-validation showed that the lipids with the top 8 importance had the lowest model error rate, (E) ROC analysis for the combinational lipids, (F) correlation between clinical parameters and levels of 8 lipid species. *P < 0.05; **P < 0.01, ***P < 0.001.
Characteristic Lipid Species Between Major Depressive Disorder and Bipolar Depression
Moreover, data of plasma lipids could be well distinguished and reliable to screen lipid biomarkers between MDD and BPD as well (Figures 5A,B). A total of 77 differential lipid species were identified (25 up-regulated and 52 down-regulated in MDD) between MDD and BPD (Figure 5C and Supplementary Table 5). Meanwhile, 10-fold cross-validation showed that the lipids with the top 13 importance had the lowest model error rate (Figure 5D). These 13 lipids covered 5 different lipid class and showed good sensitivity and specificity [AUC = 0.860, 95% CI (0.756, 0.964)] (Figure 5E). Moreover, among these characteristic species, levels of Cer(d18:1/24:1), Cer(m18:0/20:0) and Cer(m34:0 + O) were negatively while levels of DG(21:5e), PC(16:1e/16:0), PE(20:0p/18:2) and PE(37:2e) were positively correlated with the scores of HAMA and HAMD (Figure 5F). Meanwhile, levels of Cer(d18:1/16:0) and PE(8:0p/12:3) were negatively correlated with the scores of HAMA and levels of PE(16:0p/22:6) were positively correlated with the scores of HAMD. However, there was no difference in correlation analysis between levels of these species and scores of PANSS. Thus, there are differences in lipidomics between women with depression and bipolar depression, and these 13 lipids might be a potential combinational plasma biomarker to distinguish the two diseases.
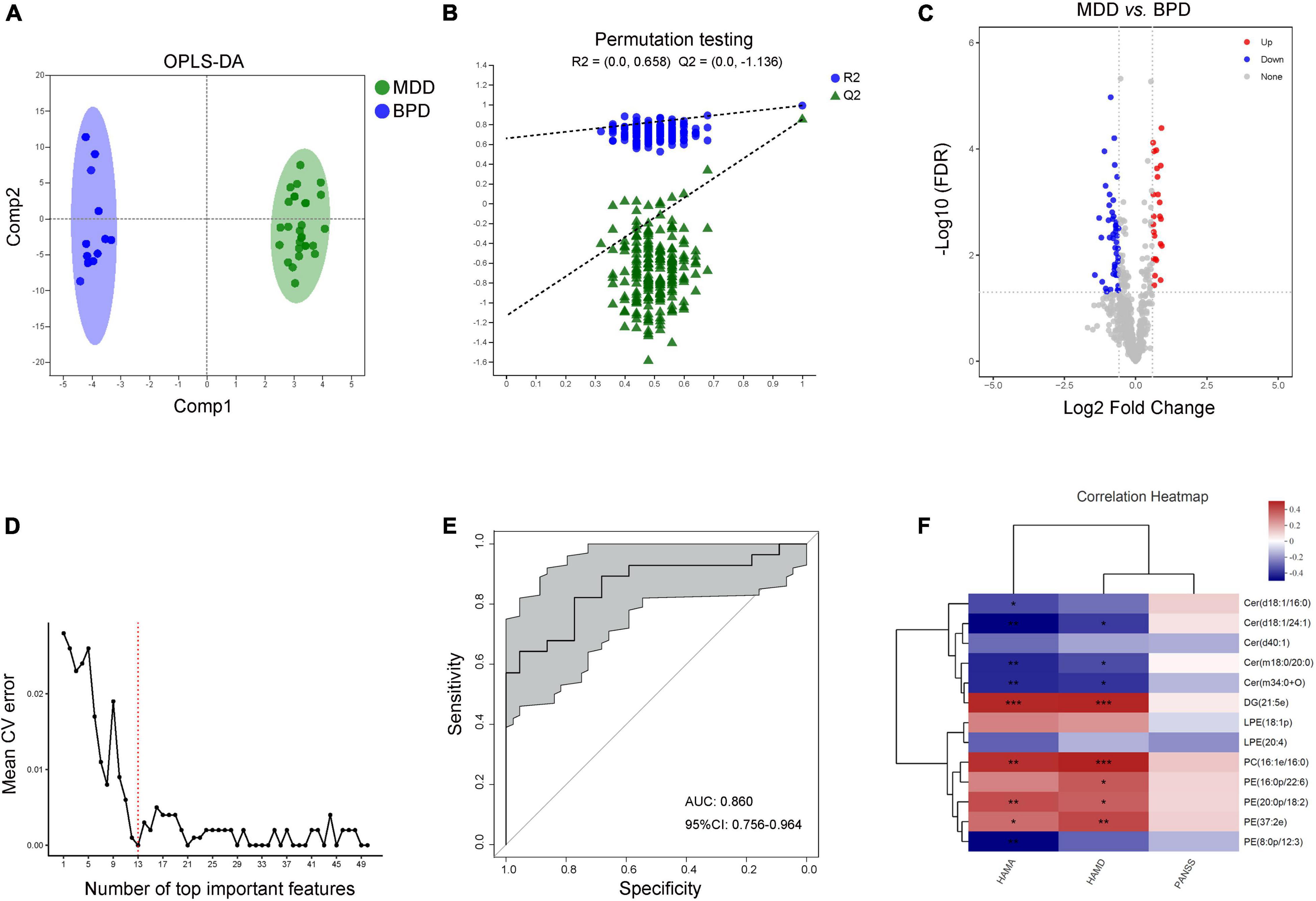
Figure 5. Characteristic lipid species between MDD and BPD. (A) Scatter plot of OPLS-DA model and (B) validation model of permutation test, (C) volcano map reveals the increased (red dots) and decreased (blue dots) lipid species between the MDD and BPD, (D) random forest model and 10-fold cross-validation showed that the lipids with the top 13 importance had the lowest model error rate, (E) ROC analysis for the combinational lipids, (F) correlation between clinical parameters and levels of 13 lipid species. *P < 0.05; **P < 0.01, ***P < 0.001.
Discussion
In this study, we characterized the peripheral lipid composition of adult women with MDD and BPD and identified unique lipids signatures of subjects with MDD and BPD relative to HC. Meanwhile, we identified diagnostic potential biomarkers comprising several lipids, which may distinguish MDD from BPD, and each from HC, with moderate reliability. Although the result is worth further exploration in clinical trials with large sample size, these results may help identify novel clinical diagnostic and therapeutic targets for adult women with MDD and BPD.
Changes in lipid composition have been reported in several neuropsychiatric diseases (45, 46), such as MDD and BD (47, 48). However, thus far, the potential lipids that can distinguish women with MDD from BPD have not been identified. Such investigations would be particularly valuable to understand the shared and distinct lipid characteristics between these two disorders. Herein, we found that the levels of MGDG, phSM, AcCa and PS were decreased while GM2 and PIP were increased both in MDD and BPD when compared with HC. Supporting this finding, previous studies found that plasma SM was negatively correlated with depressive symptoms (49) and lower concentrations of AcCa were observed in the plasma or serum in MDD and BD patients when compared with healthy control previously (50–52). Moreover, alterations in AcCa were also involved in the action of citalopram/escitalopram in major depression (53). On the other hand, although there is no direct evidence, MGDG shows a strong anti-inflammatory property (54, 55) and PS acts as an engulfment signal for phagocytosis in apoptotic cells and participates in immune regulation in non-apoptotic cells (56, 57). Meanwhile, disrupted GM2/GD2 synthase was associated with neurodegeneration disease (58) and GM2 elevation was even related to glial activation induced damage in the developing brain (59). In addition, PI and its array of inositol-phosphorylated products PIP and PIP2, play fundamental roles in the regulation of membrane trafficking and cytoskeletal dynamics as well as cell biology that include membrane-delineated signal transduction, as well as (60, 61), and serum PI might be a biomarker for bipolar disorder (62). These results suggest that there are some similar lipid changes in depression and BPD, and these lipids are related to energy metabolism and oxidative stress, inflammation and neurodegenerative diseases (63, 64). Notably, levels of LPE and LPI were decreased while PE was increased in MDD when compared with BPD. Given that lysophospholipids inhibited N-methyl-D-aspartate (NMDA) responses (65) whereas PE influenced events for the remission of acute inflammation and energy metabolism (66, 67), this difference may be related to the peripheral inflammatory response and excitability regulation between the unipolar and bipolar depression.
It should be noted that the changed lipids between the BPD and HC were more obvious than that of MDD vs. HC. Previous works found that PE was decreased whereas TG was elevated in the plasma of BD (68, 69). Due to PS biosynthesis via the serine base-exchange reaction using PE (70), the decrease in PS levels may be related to the reduction of PE. Meanwhile, LPE and PI showed pronounced positive relationships with depression severity (71). Similarly, CoQ10 might be considered as an effective strategy for the treatment of bipolar depression (72) whereas lithium significantly decreased PIP2 levels in the platelet membrane of BD (73, 74). Likewise, here we found that levels of CoQ10, SM, PS, and PE were decreased while TG, LPE, PIP, and PIP2 were increased in BPD when compared with HC. It has been reported that plasma Cer levels increase in patients with major depression and bipolar disorder (39) and the Cer might be a novel potential antidepressant target (75, 76). Unfortunately, we did not observe a difference in Cer levels between MDD and HC, as well as did in BPD vs. HC. This inconsistency may be related to the gender, age and dietary structure of the subjects included in this study. Of course, the interference of drugs cannot be ruled out. Furthermore, as the carbon chain length and the degree of saturation of the fatty acids influence biophysical properties of lipids (77, 78), the present study also observed the alterations in the concentrations of different carbon chains and unsaturated fatty acids in the plasma of each group. Although the potential mechanism for this difference is not clear, this result may further explain the difference in lipid molecular structure and function in female MDD and BPD.
In terms of lipid species, data of plasma lipids could be distinguished into the three groups (Supplementary Figure 1A). By the trends of changes in lipids class, a total of 66 differential lipid species were identified between MDD and HC whereas 172 differential lipid species were identified between BPD and HC. Meanwhile, 77 differential lipid species were identified between MDD and BPD. Coverage of lipids in all three comparisons showed that there are 20 unique lipids found in MDD vs. HC and 28 unique lipids for MDD vs. BPD (Supplementary Figure 1A and Supplementary Table 6). However, only 37 differentially regulated lipids were identified for MDD in drug-free patients and 121 statistically differential lipids between the BPD and HC in serum lipidomic analysis in previous studies (37, 38). Therefore, the present study newly reports some previously unidentified lipid signatures associated with female MDD or BPD and lays a foundation from which to further characterize the distinct plasma lipids underpinnings of MDD and BPD. Besides identifying the lipid compositions that characterized each disease, the present study also identified a signature of 20 lipids that could distinguish patients with MDD from HC, with an AUC value of 0.897 and a signature of 8 lipids that could distinguish patients with BPD from HC, with an AUC value of 0.784. Notably, a signature of 13 lipids was identified that could distinguish MDD from BPD, with an AUC value of 0.860. We believe this method has potential as a tool for distinguishing MDD from BPD (and both from HC) based on subjects’ plasma lipidomics. However, the identified potential lipid biomarkers were also inconsistent with previous works (71, 79), this discrepancy may be related to gender and symptom characteristics, sample type and analysis method which needs to be verified by larger samples in the future.
The dysfunction of lipid metabolism in peripheral blood may contribute to the etiopathology of MDD and BD (79, 80), and the use of other treatments aimed at modulating the function and regulation of the lipids such as phospholipid and sphingolipid represent a means to improve or prevention in individuals suffering from psychiatric disorders (36, 81, 82). However, the crosstalk between brain and peripheral lipid homeostasis is still largely unknown. Analysis of lipids and associated enzymes in cerebrospinal fluid might be provided further insight to explain the relationship between central lipid homeostasis and symptom characteristics of MDD and BPD. Furthermore, the most probable period of the first episode of MDD extends from mid-adolescence to mid-40s (83). In comparison, the prevalence of BD was evident from 10 years of age and peaked in the early 20s (84). Although the average age range of patients included in this study was 30–40 years old, the influence of age factors on lipid composition is partly excluded. In particular, menopause is likely to affect lipid metabolism (85, 86) and there were 9 cases over 45 years old in this study, the effect of menopause on plasma lipidomics needs to be further considered. On the other hand, other signatures that can discriminate unipolar from bipolar depression including gut microbiota, imaging features and metabolomics were reported recently (87–89), the advantages, disadvantages and interaction between lipidomic characteristics and those signatures still need to be further explored. Finally, the potential limitations of the study should be mentioned. (i) The number of recruited samples is relatively small; (ii) environmental or regional-specific biases on subjects’ lipids compositions cannot be ignored; (iii) the influence of drugs on lipid composition cannot be excluded. It is well known that antipsychotic drugs could affect lipid metabolism and olanzapine and quetiapine were used in the present study (90); (iv) our findings cannot show a causal relationship between the identified differential lipid compositions in BPD or MDD. A discovery and validation cohort research with larger sample sizes should be operated to verify the potential use of these lipid markers and it may be useful to further identify the potential biomarkers which could reflect the differences with the duration of disease or age group in the future.
Conclusion
In summary, the present study characterized and identified different plasma lipids compositions in adult women with MDD vs. BPD, and in both vs. HC. Furthermore, lipid classifiers that could be able to discriminate MDD from BPD and HC were developed. Our findings lay the potential foundation for further development of plasma lipidomic-based clinical diagnostic assay for MDD and BPD.
Data Availability Statement
The original contributions presented in this study are included in the article/Supplementary Material, further inquiries can be directed to the corresponding authors.
Ethics Statement
The study involving humans was reviewed and approved by the Ethics Committee of Chang’an Hospital (approval number: ChANLL2020001). The patients/participants provided their written informed consent to participate in this study.
Author Contributions
TZ, LG, Z-WP, and Q-RT designed the experiment protocol and responsible for the data collection. RL, FW, and W-MY were responsible for the clinical evaluation. J-BY and Z-QC were responsible for the data management. C-HZ, Y-HC, and HY analyzed the data. TZ and LG wrote the first draft. Z-WP and Q-RT financed the study and revised the manuscript. All authors contributed to the article and approved the submitted version.
Funding
This study was funded by the National Natural Science Foundation of China (grant numbers 81630032 and 82171512).
Conflict of Interest
The authors declare that the research was conducted in the absence of any commercial or financial relationships that could be construed as a potential conflict of interest.
Publisher’s Note
All claims expressed in this article are solely those of the authors and do not necessarily represent those of their affiliated organizations, or those of the publisher, the editors and the reviewers. Any product that may be evaluated in this article, or claim that may be made by its manufacturer, is not guaranteed or endorsed by the publisher.
Acknowledgments
We thank Jun-biao Zhang and Jian-hua Zhao from the Majorbio Bio-pharm Technology Co., Ltd., for technological assistance.
Supplementary Material
The Supplementary Material for this article can be found online at: https://www.frontiersin.org/articles/10.3389/fpsyt.2022.927817/full#supplementary-material
Supplementary Figure 1 | (A) Scatter plot of OPLS-DA model for three groups and (B) coverage of lipids in all three comparisons.
References
1. Vancampfort D, Firth J, Schuch FB, Rosenbaum S, Mugisha J, Hallgren M, et al. Sedentary behavior and physical activity levels in people with schizophrenia, bipolar disorder and major depressive disorder: a global systematic review and meta-analysis. World Psychiatry. (2017) 16:308–15. doi: 10.1002/wps.20458
2. Malhi GS, Das P, Outhred T, Bryant RA, Calhoun V, Mann JJ. Default mode dysfunction underpins suicidal activity in mood disorders. Psychol Med. (2020) 50:1214–23. doi: 10.1017/S0033291719001132
3. McIntyre RS, Berk M, Brietzke E, Goldstein BI, Lopez-Jaramillo C, Kessing LV, et al. Bipolar disorders. Lancet. (2020) 396:1841–56. doi: 10.1016/S0140-6736(20)31544-0
4. Bromet E, Andrade LH, Hwang I, Sampson NA, Alonso J, de Girolamo G, et al. Cross-national epidemiology of DSM-IV major depressive episode. BMC Med. (2011) 9:90. doi: 10.1186/1741-7015-9-90
5. Global Burden of Disease Study 2013 Collaborators. Global, regional, and national incidence, prevalence, and years lived with disability for 301 acute and chronic diseases and injuries in 188 countries, 1990-2013: a systematic analysis for the Global Burden of Disease Study 2013. Lancet. (2015) 386:743–800. doi: 10.1016/S0140-6736(15)60692-4
6. Grande I, Berk M, Birmaher B, Vieta E. Bipolar disorder. Lancet. (2016) 387:1561–72. doi: 10.1016/S0140-6736(15)00241-X
7. Merikangas KR, Akiskal HS, Angst J, Greenberg PE, Hirschfeld RM, Petukhova M, et al. Lifetime and 12-month prevalence of bipolar spectrum disorder in the National Comorbidity Survey replication. Arch Gen Psychiatry. (2007) 64:543–52. doi: 10.1001/archpsyc.64.5.543
8. Judd LL, Akiskal HS, Schettler PJ, Endicott J, Maser J, Solomon DA, et al. The long-term natural history of the weekly symptomatic status of bipolar I disorder. Arch Gen Psychiatry. (2002) 59:530–7. doi: 10.1001/archpsyc.59.6.530
9. Hirschfeld RM. Differential diagnosis of bipolar disorder and major depressive disorder. J Affect Disord. (2014) 169(Suppl 1):S12–6. doi: 10.1016/S0165-0327(14)70004-7
10. Douglas KM, Gallagher P, Robinson LJ, Carter JD, McIntosh VV, Frampton CM, et al. Prevalence of cognitive impairment in major depression and bipolar disorder. Bipolar Disord. (2018) 20:260–74. doi: 10.1111/bdi.12602
11. Dervaux A, Laqueille X. Bipolar disorder: defining symptoms and comorbidities. Lancet. (2016) 388:869. doi: 10.1016/S0140-6736(16)31430-1
12. Ghaemi SN, Rosenquist KJ, Ko JY, Baldassano CF, Kontos NJ, Baldessarini RJ. Antidepressant treatment in bipolar versus unipolar depression. Am J Psychiatry. (2004) 161:163–5. doi: 10.1176/appi.ajp.161.1.163
13. Shim IH, Woo YS, Kim MD, Bahk WM. Antidepressants and mood stabilizers: novel research avenues and clinical insights for bipolar depression. Int J Mol Sci. (2017) 18:2406. doi: 10.3390/ijms18112406
14. Hirschfeld RM, Lewis L, Vornik LA. Perceptions and impact of bipolar disorder: how far have we really come? Results of the national depressive and manic-depressive association 2000 survey of individuals with bipolar disorder. J Clin Psychiatry. (2003) 64:161–74.
15. Angst J, Sellaro R, Stassen HH, Gamma A. Diagnostic conversion from depression to bipolar disorders: results of a long-term prospective study of hospital admissions. J Affect Disord. (2005) 84:149–57. doi: 10.1016/S0165-0327(03)00195-2
16. Pacchiarotti I, Bond DJ, Baldessarini RJ, Nolen WA, Grunze H, Licht RW, et al. The International Society for Bipolar Disorders (ISBD) task force report on antidepressant use in bipolar disorders. Am J Psychiatry. (2013) 170:1249–62. doi: 10.1176/appi.ajp.2013.13020185
17. Cipriani A, Furukawa TA, Salanti G, Chaimani A, Atkinson LZ, Ogawa Y, et al. Comparative efficacy and acceptability of 21 antidepressant drugs for the acute treatment of adults with major depressive disorder: a systematic review and network meta-analysis. Lancet. (2018) 391:1357–66. doi: 10.1016/S0140-6736(17)32802-7
18. Akil H, Gordon J, Hen R, Javitch J, Mayberg H, McEwen B, et al. Treatment resistant depression: a multi-scale, systems biology approach. Neurosci Biobehav Rev. (2018) 84:272–88. doi: 10.1016/j.neubiorev.2017.08.019
19. Jadhav S, Russo S, Cottier S, Schneiter R, Cowart A, Greenberg ML. Valproate induces the unfolded protein response by increasing ceramide levels. J Biol Chem. (2016) 291:22253–61. doi: 10.1074/jbc.M116.752634
20. Henry CA, Zamvil LS, Lam C, Rosenquist KJ, Ghaemi SN. Long-term outcome with divalproex in children and adolescents with bipolar disorder. J Child Adolesc Psychopharmacol. (2003) 13:523–9. doi: 10.1089/104454603322724913
21. Smith DJ, Griffiths E, Kelly M, Hood K, Craddock N, Simpson SA. Unrecognised bipolar disorder in primary care patients with depression. Br J Psychiatry. (2011) 199:49–56. doi: 10.1192/bjp.bp.110.083840
22. Pradier MF, Hughes MC, McCoy TH Jr., Barroilhet SA, Doshi-Velez F, Perlis RH. Predicting change in diagnosis from major depression to bipolar disorder after antidepressant initiation. Neuropsychopharmacology. (2021) 46:455–61. doi: 10.1038/s41386-020-00838-x
23. Kuehner C. Why is depression more common among women than among men? Lancet Psychiatry. (2017) 4:146–58. doi: 10.1016/S2215-0366(16)30263-2
24. Sassarini DJ. Depression in midlife women. Maturitas. (2016) 94:149–54. doi: 10.1016/j.maturitas.2016.09.004
25. Kornstein SG, Schatzberg AF, Thase ME, Yonkers KA, McCullough JP, Keitner GI, et al. Gender differences in chronic major and double depression. J Affect Disord. (2000) 60:1–11. doi: 10.1016/s0165-0327(99)00158-5
26. Seney ML, Huo Z, Cahill K, French L, Puralewski R, Zhang J, et al. Opposite molecular signatures of depression in men and women. Biol Psychiatry. (2018) 84:18–27. doi: 10.1016/j.biopsych.2018.01.017
27. Marcus SM, Young EA, Kerber KB, Kornstein S, Farabaugh AH, Mitchell J, et al. Gender differences in depression: findings from the STAR*D study. J Affect Disord. (2005) 87:141–50. doi: 10.1016/j.jad.2004.09.008
28. Clark CT, Wisner KL. Treatment of peripartum bipolar disorder. Obstet Gynecol Clin North Am. (2018) 45:403–17. doi: 10.1016/j.ogc.2018.05.002
29. Huang Y, Wang Y, Wang H, Liu Z, Yu X, Yan J, et al. Prevalence of mental disorders in China: a cross-sectional epidemiological study. Lancet Psychiatry. (2019) 6:211–24. doi: 10.1016/S2215-0366(18)30511-X
30. Miller LJ, Ghadiali NY, Larusso EM, Wahlen KJ, Avni-Barron O, Mittal L, et al. Bipolar disorder in women. Health Care Women Int. (2015) 36:475–98. doi: 10.1080/07399332.2014.962138
31. Di Florio A, Forty L, Gordon-Smith K, Heron J, Jones L, Craddock N, et al. Perinatal episodes across the mood disorder spectrum. JAMA Psychiatry. (2013) 70:168–75. doi: 10.1001/jamapsychiatry.2013.279
32. Wesseloo R, Kamperman AM, Munk-Olsen T, Pop VJ, Kushner SA, Bergink V. Risk of postpartum relapse in bipolar disorder and postpartum psychosis: a systematic review and meta-analysis. Am J Psychiatry. (2016) 173:117–27. doi: 10.1176/appi.ajp.2015.15010124
33. Preece RL, Han SYS, Bahn S. Proteomic approaches to identify blood-based biomarkers for depression and bipolar disorders. Expert Rev Proteomics. (2018) 15:325–40. doi: 10.1080/14789450.2018.1444483
34. Pu J, Liu Y, Zhang H, Tian L, Gui S, Yu Y, et al. An integrated meta-analysis of peripheral blood metabolites and biological functions in major depressive disorder. Mol Psychiatry. (2021) 26:4265–76. doi: 10.1038/s41380-020-0645-4
35. Shi Y, Song R, Wang L, Qi Y, Zhang H, Zhu J, et al. Identifying Plasma Biomarkers with high specificity for major depressive disorder: a multi-level proteomics study. J Affect Disord. (2020) 277:620–30. doi: 10.1016/j.jad.2020.08.078
36. Schneider M, Levant B, Reichel M, Gulbins E, Kornhuber J, Muller CP. Lipids in psychiatric disorders and preventive medicine. Neurosci Biobehav Rev. (2017) 76:336–62. doi: 10.1016/j.neubiorev.2016.06.002
37. Ribeiro HC, Klassen A, Pedrini M, Carvalho MS, Rizzo LB, Noto MN, et al. A preliminary study of bipolar disorder type I by mass spectrometry-based serum lipidomics. Psychiatry Res. (2017) 258:268–73. doi: 10.1016/j.psychres.2017.08.039
38. Kim EY, Lee JW, Lee MY, Kim SH, Mok HJ, Ha K, et al. Serum lipidomic analysis for the discovery of biomarkers for major depressive disorder in drug-free patients. Psychiatry Res. (2018) 265:174–82. doi: 10.1016/j.psychres.2018.04.029
39. Brunkhorst-Kanaan N, Klatt-Schreiner K, Hackel J, Schroter K, Trautmann S, Hahnefeld L, et al. Targeted lipidomics reveal derangement of ceramides in major depression and bipolar disorder. Metabolism. (2019) 95:65–76. doi: 10.1016/j.metabol.2019.04.002
40. Wu Z, Zhao P, Long Z, Li J, Yang G, Zhang Q, et al. Biomarker screening for antenatal depression in women who underwent caesarean section: a matched observational study with plasma Lipidomics. BMC Psychiatry. (2019) 19:259. doi: 10.1186/s12888-019-2241-1
41. Guo L, Zhang T, Li R, Cui ZQ, Du J, Yang JB, et al. Alterations in the plasma lipidome of adult women with bipolar disorder: a mass spectrometry-based lipidomics research. Front Psychiatry. (2022) 13:802710. doi: 10.3389/fpsyt.2022.802710
42. Fu Y, Chen N, Wang Z, Luo S, Ding Y, Lu B. Degradation of lipid droplets by chimeric autophagy-tethering compounds. Cell Res. (2021) 31:965–79. doi: 10.1038/s41422-021-00532-7
43. Sun Y, Song K, Liu L, Sun L, Qin Q, Jiang T, et al. Silencing of sulfoquinovosyl diacylglycerol synthase 1 impairs the glycolipids accumulation and photosynthesis in phosphate-deprived rice. J Exp Bot. (2021) 72:6510–23. doi: 10.1093/jxb/erab300
44. Cajka T, Smilowitz JT, Fiehn O. Validating quantitative untargeted lipidomics across nine liquid chromatography-high-resolution mass spectrometry platforms. Anal Chem. (2017) 89:12360–8. doi: 10.1021/acs.analchem.7b03404
45. Bornstein SR, Voit-Bak K, Rosenthal P, Tselmin S, Julius U, Schatz U, et al. Extracorporeal apheresis therapy for Alzheimer disease-targeting lipids, stress, and inflammation. Mol Psychiatry. (2020) 25:275–82. doi: 10.1038/s41380-019-0542-x
46. Kim DD, Barr AM, Fredrikson DH, Honer WG, Procyshyn RM. Association between serum lipids and antipsychotic response in schizophrenia. Curr Neuropharmacol. (2019) 17:852–60. doi: 10.2174/1570159X17666190228113348
48. Erfurth A, Kuhn G. Topiramate monotherapy in the maintenance treatment of bipolar I disorder: effects on mood, weight and serum lipids. Neuropsychobiology. (2000) 42(Suppl. 1):50–1. doi: 10.1159/000054854
49. Demirkan A, Isaacs A, Ugocsai P, Liebisch G, Struchalin M, Rudan I, et al. Plasma phosphatidylcholine and sphingomyelin concentrations are associated with depression and anxiety symptoms in a Dutch family-based lipidomics study. J Psychiatr Res. (2013) 47:357–62. doi: 10.1016/j.jpsychires.2012.11.001
50. Yokoyama S, Yasui-Furukori N, Nakagami T, Miyazaki K, Ishioka M, Tarakita N, et al. Association between the serum carnitine level and ammonia and valproic acid levels in patients with bipolar disorder. Ther Drug Monit. (2020) 42:766–70. doi: 10.1097/FTD.0000000000000778
51. Maldonado C, Vazquez M, Fagiolino P. Potential therapeutic role of carnitine and acetylcarnitine in neurological disorders. Curr Pharm Des. (2020) 26:1277–85. doi: 10.2174/1381612826666200212114038
52. Ahmed AT, MahmoudianDehkordi S, Bhattacharyya S, Arnold M, Liu D, Neavin D, et al. Acylcarnitine metabolomic profiles inform clinically-defined major depressive phenotypes. J Affect Disord. (2020) 264:90–7. doi: 10.1016/j.jad.2019.11.122
53. MahmoudianDehkordi S, Ahmed AT, Bhattacharyya S, Han X, Baillie RA, Arnold M, et al. Alterations in acylcarnitines, amines, and lipids inform about the mechanism of action of citalopram/escitalopram in major depression. Transl Psychiatry. (2021) 11:153. doi: 10.1038/s41398-020-01097-6
54. Lenti M, Gentili C, Pianezzi A, Marcolongo G, Lalli A, Cancedda R, et al. Monogalactosyldiacylglycerol anti-inflammatory activity on adult articular cartilage. Nat Prod Res. (2009) 23:754–62. doi: 10.1080/14786410802456956
55. Ulivi V, Lenti M, Gentili C, Marcolongo G, Cancedda R, Descalzi Cancedda F. Anti-inflammatory activity of monogalactosyldiacylglycerol in human articular cartilage in vitro: activation of an anti-inflammatory cyclooxygenase-2 (COX-2) pathway. Arthritis Res Ther. (2011) 13:R92. doi: 10.1186/ar3367
56. Sharma B, Kanwar SS. Phosphatidylserine: a cancer cell targeting biomarker. Semin Cancer Biol. (2018) 52:17–25. doi: 10.1016/j.semcancer.2017.08.012
57. Chang W, Fa H, Xiao D, Wang J. Targeting phosphatidylserine for Cancer therapy: prospects and challenges. Theranostics. (2020) 10:9214–29. doi: 10.7150/thno.45125
58. Sheikh KA, Sun J, Liu Y, Kawai H, Crawford TO, Proia RL, et al. Mice lacking complex gangliosides develop Wallerian degeneration and myelination defects. Proc Natl Acad Sci USA. (1999) 96:7532–7. doi: 10.1073/pnas.96.13.7532
59. Saito M, Wu G, Hui M, Masiello K, Dobrenis K, Ledeen RW, et al. Ganglioside accumulation in activated glia in the developing brain: comparison between WT and GalNAcT KO mice. J Lipid Res. (2015) 56:1434–48. doi: 10.1194/jlr.M056580
60. Di Paolo G, De Camilli P. Phosphoinositides in cell regulation and membrane dynamics. Nature. (2006) 443:651–7. doi: 10.1038/nature05185
61. Kim SJ, Jeong MJ, Jo HJ, Jung JH, Kaang BK, Choi YB, et al. Identification of postsynaptic phosphatidylinositol-4,5-bisphosphate (PIP2) roles for synaptic plasticity using chemically induced dimerization. Sci Rep. (2017) 7:3351. doi: 10.1038/s41598-017-03520-3
62. Knowles EE, Meikle PJ, Huynh K, Goring HH, Olvera RL, Mathias SR, et al. Serum phosphatidylinositol as a biomarker for bipolar disorder liability. Bipolar Disord. (2017) 19:107–15. doi: 10.1111/bdi.12468
63. Kler RS, Jackson S, Bartlett K, Bindoff LA, Eaton S, Pourfarzam M, et al. Quantitation of acyl-CoA and acylcarnitine esters accumulated during abnormal mitochondrial fatty acid oxidation. J Biol Chem. (1991) 266:22932–8.
64. Maceyka M, Spiegel S. Sphingolipid metabolites in inflammatory disease. Nature. (2014) 510:58–67. doi: 10.1038/nature13475
65. Casado M, Ascher P. Opposite modulation of NMDA receptors by lysophospholipids and arachidonic acid: common features with mechanosensitivity. J Physiol. (1998) 513(Pt 2):317–30. doi: 10.1111/j.1469-7793.1998.317bb.x
66. Eros G, Varga G, Varadi R, Czobel M, Kaszaki J, Ghyczy M, et al. Anti-inflammatory action of a phosphatidylcholine, phosphatidylethanolamine and N-acylphosphatidylethanolamine-enriched diet in carrageenan-induced pleurisy. Eur Surg Res. (2009) 42:40–8. doi: 10.1159/000167856
67. van der Veen JN, Kennelly JP, Wan S, Vance JE, Vance DE, Jacobs RL. The critical role of phosphatidylcholine and phosphatidylethanolamine metabolism in health and disease. Biochim Biophys Acta Biomembr. (2017) 1859:1558–72. doi: 10.1016/j.bbamem.2017.04.006
68. Modica-Napolitano JS, Renshaw PF. Ethanolamine and phosphoethanolamine inhibit mitochondrial function in vitro: implications for mitochondrial dysfunction hypothesis in depression and bipolar disorder. Biol Psychiatry. (2004) 55:273–7. doi: 10.1016/s0006-3223(03)00784-4
69. Otoki Y, Hennebelle M, Levitt AJ, Nakagawa K, Swardfager W, Taha AY. Plasma phosphatidylethanolamine and triacylglycerol fatty acid concentrations are altered in major depressive disorder patients with seasonal pattern. Lipids. (2017) 52:559–71. doi: 10.1007/s11745-017-4254-1
70. Vance JE, Steenbergen R. Metabolism and functions of phosphatidylserine. Prog Lipid Res. (2005) 44:207–34. doi: 10.1016/j.plipres.2005.05.001
71. Liu X, Li J, Zheng P, Zhao X, Zhou C, Hu C, et al. Plasma lipidomics reveals potential lipid markers of major depressive disorder. Anal Bioanal Chem. (2016) 408:6497–507. doi: 10.1007/s00216-016-9768-5
72. Mehrpooya M, Yasrebifar F, Haghighi M, Mohammadi Y, Jahangard L. Evaluating the effect of Coenzyme Q10 augmentation on treatment of bipolar depression: a double-blind controlled clinical trial. J Clin Psychopharmacol. (2018) 38:460–6. doi: 10.1097/JCP.0000000000000938
73. Soares JC, Mallinger AG, Dippold CS, Forster Wells K, Frank E, Kupfer DJ. Effects of lithium on platelet membrane phosphoinositides in bipolar disorder patients: a pilot study. Psychopharmacology (Berl). (2000) 149:12–6. doi: 10.1007/s002139900341
74. Soares JC, Dippold CS, Wells KF, Frank E, Kupfer DJ, Mallinger AG. Increased platelet membrane phosphatidylinositol-4,5-bisphosphate in drug-free depressed bipolar patients. Neurosci Lett. (2001) 299:150–2. doi: 10.1016/s0304-3940(00)01775-4
75. Kornhuber J, Muller CP, Becker KA, Reichel M, Gulbins E. The ceramide system as a novel antidepressant target. Trends Pharmacol Sci. (2014) 35:293–304. doi: 10.1016/j.tips.2014.04.003
76. Kurz J, Parnham MJ, Geisslinger G, Schiffmann S. Ceramides as novel disease biomarkers. Trends Mol Med. (2019) 25:20–32. doi: 10.1016/j.molmed.2018.10.009
77. Carta M, Lanore F, Rebola N, Szabo Z, Da Silva SV, Lourenco J, et al. Membrane lipids tune synaptic transmission by direct modulation of presynaptic potassium channels. Neuron. (2014) 81:787–99. doi: 10.1016/j.neuron.2013.12.028
78. Oliveira TG, Chan RB, Bravo FV, Miranda A, Silva RR, Zhou B, et al. The impact of chronic stress on the rat brain lipidome. Mol Psychiatry. (2016) 21:80–8. doi: 10.1038/mp.2015.14
79. D’Ambrosio V, Salvi V, Bogetto F, Maina G. Serum lipids, metabolic syndrome and lifetime suicide attempts in patients with bipolar disorder. Prog Neuropsychopharmacol Biol Psychiatry. (2012) 37:136–40. doi: 10.1016/j.pnpbp.2011.12.009
80. Scola G, Versace A, Metherel AH, Monsalve-Castro LA, Phillips ML, Bazinet RP, et al. Alterations in peripheral fatty acid composition in bipolar and unipolar depression. J Affect Disord. (2018) 233:86–91. doi: 10.1016/j.jad.2017.12.025
81. Liao Y, Xie B, Zhang H, He Q, Guo L, Subramanieapillai M, et al. Efficacy of omega-3 PUFAs in depression: a meta-analysis. Transl Psychiatry. (2019) 9:190. doi: 10.1038/s41398-019-0515-5
82. Robinson DG, Gallego JA, John M, Hanna LA, Zhang JP, Birnbaum ML, et al. A potential role for adjunctive omega-3 polyunsaturated fatty acids for depression and anxiety symptoms in recent onset psychosis: results from a 16week randomized placebo-controlled trial for participants concurrently treated with risperidone. Schizophr Res. (2019) 204:295–303. doi: 10.1016/j.schres.2018.09.006
83. Moffitt TE, Caspi A, Taylor A, Kokaua J, Milne BJ, Polanczyk G, et al. How common are common mental disorders? Evidence that lifetime prevalence rates are doubled by prospective versus retrospective ascertainment. Psychol Med. (2010) 40:899–909. doi: 10.1017/S0033291709991036
84. Ferrari AJ, Stockings E, Khoo JP, Erskine HE, Degenhardt L, Vos T, et al. The prevalence and burden of bipolar disorder: findings from the Global Burden of Disease Study 2013. Bipolar Disord. (2016) 18:440–50. doi: 10.1111/bdi.12423
85. Ko SH, Jung Y. Energy metabolism changes and dysregulated lipid metabolism in postmenopausal women. Nutrients. (2021) 13:4556. doi: 10.3390/nu13124556
86. Ko SH, Kim HS. Menopause-associated lipid metabolic disorders and foods beneficial for postmenopausal women. Nutrients. (2020) 12:202. doi: 10.3390/nu12010202
87. Zheng P, Yang J, Li Y, Wu J, Liang W, Yin B, et al. Gut microbial signatures can discriminate unipolar from bipolar depression. Adv Sci (Weinh). (2020) 7:1902862. doi: 10.1002/advs.201902862
88. Gong J, Wang J, Qiu S, Chen P, Luo Z, Wang J, et al. Common and distinct patterns of intrinsic brain activity alterations in major depression and bipolar disorder: voxel-based meta-analysis. Transl Psychiatry. (2020) 10:353. doi: 10.1038/s41398-020-01036-5
89. Hashimoto K. Metabolomics of major depressive disorder and bipolar disorder: overview and future perspective. Adv Clin Chem. (2018) 84:81–99.
Keywords: lipidomics, women, depression, bipolar depression, plasma lipid
Citation: Zhang T, Guo L, Li R, Wang F, Yang W-m, Yang J-b, Cui Z-q, Zhou C-h, Chen Y-h, Yu H, Peng Z-w and Tan Q-r (2022) Alterations of Plasma Lipids in Adult Women With Major Depressive Disorder and Bipolar Depression. Front. Psychiatry 13:927817. doi: 10.3389/fpsyt.2022.927817
Received: 25 April 2022; Accepted: 21 June 2022;
Published: 18 July 2022.
Edited by:
Karen Tabb, University of Illinois at Urbana-Champaign, United StatesCopyright © 2022 Zhang, Guo, Li, Wang, Yang, Yang, Cui, Zhou, Chen, Yu, Peng and Tan. This is an open-access article distributed under the terms of the Creative Commons Attribution License (CC BY). The use, distribution or reproduction in other forums is permitted, provided the original author(s) and the copyright owner(s) are credited and that the original publication in this journal is cited, in accordance with accepted academic practice. No use, distribution or reproduction is permitted which does not comply with these terms.
*Correspondence: Zheng-wu Peng, cGVuZ3poZW5nd3UxNDQ2QDE2My5jb20=; Qing-rong Tan, dGFucWluZ3JAZm1tdS5lZHUuY24=
†These authors have contributed equally to this work