- 1Department of Biostatistics, School of Public Health, Peking University, Beijing, China
- 2Department of Epidemiology & Biostatistics, School of Public Health, Peking University, Beijing, China
- 3Center for Intelligent Public Health, Academy for Artificial Intelligence, Peking University, Beijing, China
- 4Key Laboratory of Molecular Cardiovascular Sciences (Peking University), Ministry of Education, Beijing, China
- 5Center for Statistical Science, Peking University, Beijing, China
Coffee or caffeine consumption has been associated with neuropsychiatric disorders, implying a shared etiology. However, whether these associations reflect causality remains largely unknown. To understand the genetic structure of the association between decaffeinated coffee consumption (DCC) and neuropsychiatric traits, we examined the genetic correlation, causality, and shared genetic structure between DCC and neuropsychiatric traits using linkage disequilibrium score regression, bidirectional Mendelian randomization (MR), and genome-wide cross-trait meta-analysis in large GWAS Consortia for coffee consumption (N = 329,671) and 13 neuropsychiatric traits (sample size ranges from 36,052 to 500,199). We found strong positive genetic correlations between DCC and lifetime cannabis use (LCU; Rg = 0.48, P = 8.40 × 10−19), alcohol use disorder identification test (AUDIT) total score (AUDIT_T; Rg = 0.40, P = 4.63 × 10−13), AUDIT_C score (alcohol consumption component of the AUDIT; Rg = 0.40, P = 5.26 × 10−11), AUDIT_P score (dependence and hazardous-use component of the AUDIT; Rg = 0.28, P = 1.36 × 10−05), and strong negative genetic correlations between DCC and neuroticism (Rg = −0.15, P = 7.27 × 10−05), major depressed diseases (MDD; Rg = −0.15, P = 0.0010), and insomnia (Rg= −0.15, P = 0.0007). In the cross-trait meta-analysis, we identified 6, 5, 1, 1, 2, 31, and 27 shared loci between DCC and Insomnia, LCU, AUDIT_T, AUDIT_C, AUDIT_P, neuroticism, and MDD, respectively, which were mainly enriched in bone marrow, lymph node, cervix, uterine, lung, and thyroid gland tissues, T cell receptor signaling pathway, antigen receptor-mediated signaling pathway, and epigenetic pathways. A large of TWAS-significant associations were identified in tissues that are part of the nervous system, digestive system, and exo-/endocrine system. Our findings further indicated a causal influence of liability to DCC on LCU and low risk of MDD (odds ratio: 0.90, P = 9.06 × 10−5 and 1.27, P = 7.63 × 10−4 respectively). We also observed that AUDIT_T and AUDIT_C were causally related to DCC (odds ratio: 1.83 per 1-SD increase in AUDIT_T, P = 1.67 × 10−05, 1.80 per 1-SD increase in AUDIT_C, P = 5.09 × 10−04). Meanwhile, insomnia and MDD had a causal negative influence on DCC (OR: 0.91, 95% CI: 0.86–0.95, P = 1.51 × 10−04 for Insomnia; OR: 0.93, 95% CI: 0.89–0.99, P = 6.02 × 10−04 for MDD). These findings provided evidence for the shared genetic basis and causality between DCC and neuropsychiatric diseases, and advance our understanding of the shared genetic mechanisms underlying their associations, as well as assisting with making recommendations for clinical works or health education.
Introduction
Coffee, one of the most widely consumed beverages in the world, has received considerable attention on health consequences. Many different physiologically active compounds including caffeine, polyphenols, niacin, and others (1) have been associated with neuropsychiatric diseases. For example, coffee consumption was related to various mental diseases such as MDD (2), Alzheimer's (3) and Parkinson's disease (4), attention deficit and hyperactivity disorder (ADHD) (5), bipolar disorder (BIP) (6), and schizophrenia (SCZ) (7). One hypothesis to account for these associations is a common genetic liability. Genetic studies could provide insight into specific biological processes underlying comorbidity and disease risk.
With the increased availability of summary data from large-scale genome-wide association studies (GWAS) and methods estimating genome-wide genetic correlation by using only GWAS summary statistics (8–10), we can estimate the common genetic basis for complex traits by using genomics resources. Further, coffee consumption and neuropsychiatric diseases are heritable traits, and twin studies estimated the heritability of coffee consumption to be ~50% (11, 12). For different neuropsychiatric diseases, heritability has been estimated at varying between 50 and 80% (13–17). Recently, A GWAS of caffeine consumption in coffee consumers detected one susceptibility loci rs13107325 (SLC39A8) (18), which was also significantly identified in the GWAS of mental diseases (19, 20), suggesting potential shared genetic components between caffeine coffee consumption and neuropsychiatric diseases. Nevertheless, coffee is more than caffeine, and many other substances were found in amounts much more than that of caffeine and were thought to be responsible for health conditions (21–23). Little is known about the genetic correlations and shared genetic loci between DCC and neuropsychiatric diseases. In addition, traditional observational studies could be influenced by reverse causation or environmental confounders, and genetics investigation could help bridge these gaps from traditional epidemiological studies (24).
Therefore, we aimed to investigate the genetic correlation, causality, and shared genetic structure between decaffeinated coffee consumption and neuropsychiatric traits including insomnia, neuroticism, Alzheimer's disease (AD), BIP, anorexia nervosa (AN), MDD, SCZ, AUDIT score, ADHD, LCU, and amyotrophic lateral sclerosis (ALS) by using linkage disequilibrium (LD) score regression, bidirectional Mendelian randomization (MR), and genome-wide cross-trait meta-analysis with GWAS summary statistics, to provide more knowledge about the shared molecular mechanism of them and detect the potential genetic liability or strong evidence for a causal relationship.
Materials and Methods
Study Design and Data Sources
The workflow of our analysis was shown in Figure 1. There were three main parts in our study: genetic correlation, causality, and shared genetics analysis between DCC and neuropsychiatric traits. We selected a large GWAS of coffee type in which the consumers of decaffeinated coffee were coded as cases, with the consumers of instant, ground, and other types of coffee coded as controls. Full summary statistics for coffee consumption (64,717 cases and 264,954 controls) were downloaded from the UK Biobank (UKBB) at http://www.nealelab.is/uk-biobank/. The GWAS summary statistics of insomnia and neuroticism items scores are available at https://ctg.cncr.nl/software/summary_statistics. We also could download GWAS summary statistics of AD (71,880 cases and 383,378 controls), BIP (20,352 cases and 31,358 controls), AN (16,992 cases and 55,525 controls), MDD (170,756 cases and 329,443 controls), SCZ (40,675 cases and 64,643 controls), and AUDIT (n = 121,604) from The Psychiatric Genomics Consortium (PGC) (Available online: http://www.med.unc.edu/pgc). Particularly, we selected three GWAS analysis results for AUDIT performed with AUDIT total score, AUDIT_C score, and AUDIT_P score. GWAS data on ADHD (19,099 cases and 34,194 controls) was derived from the meta-analyses by PGC and the Lundbeck Foundation Initiative for Integrative Psychiatric Research (iPSYCH) released in June 2017. The GWAS summary statistics for LCU (43,380 cases and 118,702 controls) and ALS (12,577 cases and 23,475 controls) were downloaded from the International Cannabis Consortium (ICC; Available online: https://www.ru.nl/bsi/research/group-pages/substance-use-addiction-food-saf/vm-saf/genetics/international-cannabis-consortium-icc/) and Project MinE, an international collaboration of investigators aiming to unravel the genetic basis of ALS, respectively (Available online: http://databrowser.projectmine.com/). For all the meta-analyses of GWAS included in this study, we only focused on the data generated from the analysis of individuals from Europe. The original GWAS studies can be referred to as the publications presented in Supplementary Table 1. A more detailed description of data has been described in their original studies; in addition, we restricted the chromosome region to autosomal chromosomes and excluded single-nucleotide polymorphisms (SNPs) in the MHC region (chromosome 6, base-pair positions 29,640,000–33,120,000 from the Genome Reference Consortium Human Build 37, hg19).
Linkage Disequilibrium Score Regression Analysis
To evaluate the genetic correlation between DCC and neuropsychiatric traits, we conducted a post-GWAS genome-wide genetic correlation analysis by LD score regression using LDSC software (8, 9). The fact that the GWAS effect size estimate for each SNP represents the effects of all SNPs in LD with that SNP was met in this analysis. Thus, the SNPs in a high-LD region would show higher test statistics compared with the SNPs in a low-LD region. This method also provides a self-estimated intercept to show the overlapped subjects between studies.
Cross-Trait Meta-Analysis
To identify shared genetic loci between traits pair that showed significant genetic correlations in LD score regression analysis, we performed a genome-wide cross-trait meta-analysis using CPASSOC (25). This method uses GWAS summary statistics of multiple traits with fewer limits of characteristics, either correlated, dependent, continuous, or binary traits coming from the same or different studies. Trait heterogeneity effects, population structure, and cryptic relatedness are also allowed. This method calculates two statistics, SHet and SHom. We used SHet as the main statistic in consideration of the heterogeneity of different traits in our study. SNPs with PSHet < 5 × 10−8 and trait-specific P < 0.01 were thought to have effects on both traits.
Fine-Mapping Credible Set Analysis
To make the identified regions more precise, we conducted the fine-mapping credible set analysis. We extracted variants within 500 kb of the index SNP and identified a 99% credible set of causal variants at each of the shared loci between DCC and neuropsychiatric traits using the Bayesian likelihood fine-mapping algorithm (26). This method only maps the primary signal and uses flat prior with the steepest descent approximation. More details of the method were described in previous publications (27, 28).
Co-localization Analysis
We used R “coloc” package to perform genetic colocalization analysis to detect whether the two traits we are interested in share common genetic variants in a given region. For each locus, we extracted variants within 500 kb of the index SNP. The probability H4 was calculated in this method and the co-localized loci were defined with a probability >0.5 (29–33).
Tissue Enrichment Analysis
To calculate the tissue-specific gene enrichment and find out tissue of enriched expression of shared genes between DCC and neuropsychiatric traits, we performed a tissue enrichment analysis using the Tissue Enrich package (34, 35). The hypergeometric test was used in this analysis to determine if shared gene sets were highly enriched or specifically expressed in a tissue. The tissue-specific genes are enriched among the shared genes by processing RNA-Seq data from the Human Protein Atlas (HPA) and GTEx using the algorithm from the HPA.
Over-representation Enrichment Analysis
We used the WebGestalt tool to assess the over-represented enrichment of the identified shared gene from a cross-trait meta-analysis in Reactome pathways and GO biological processes (36, 37). Benjamini–Hochberg procedure was used to account for multiple testing.
Mendelian Randomization Analysis
We conducted a bidirectional MR analysis using a two-sample MR package to estimate if DCC and neuropsychiatric traits were causally related to each other, using inverse-variance weighted (IVW) (38) as the primary method. Other approaches including the simple median method, weighted median method, and MR-Egger method were also used to calculate the causal effect. The genetic instruments used in MR analysis must be associated with exposure and conditionally independent of the outcome given by the exposure, as well as not be the confounders between the exposure and outcome (24). To meet these consumptions, we chose SNPs with P < 1 × 10−5 from the GWAS summary statistics for interested exposure as the genetic instruments, and selected independent genetic instruments (clumping process r2 = 0.001, kb = 10,000). Considering the potential horizontal pleiotropy and heterogeneity, we used the intercept term in MR Egger regression to test a significant horizontal pleiotropy and used the IVW method and MR-Egger regression to detect heterogeneity. MR-Pleiotropy Residual Sum and Outlier (MR-PRESSO) method was also used as sensitivity analysis. We performed the MR analysis step by step as follows (39). First, we conducted an MR analysis with all the selected instruments. If the MR-PRESSO analysis indicated a significant horizontal pleiotropy, we shall perform MR analysis again after removing outlier variants with a P-value less than the threshold in the MR-PRESSO outlier test. After the above two steps, if the heterogeneity was still significant, we would remove all the SNPs with a P-value <1 in the MR-PRESSO outlier test and perform the final MR analysis. At last, we used the leave-one-out sensitivity analysis to identify potential influential SNPs and draw a robust and definitive conclusion or a conclusion with caution.
Transcriptome-Wide Association Analysis
By using the FUSION software package based on 48 GTEx (version 7) tissue expression reference weights, we performed a TWAS to identify gene expression in specific tissues for each trait (40). The P-value was corrected by the false discovery rate (FDR) and Benjamin–Hochberg procedure, and FDR <0.05 was considered significant.
Results
Genome-Wide Genetic Correlation
We estimated the genetic correlation of DCC with 13 neuropsychiatric traits of interest. There were strong positive genetic correlations between DCC and LCU (Rg = 0.48, P = 8.40 × 10−19), AUDIT_T (Rg = 0.40, P = 4.63 × 10−13), AUDIT_C (Rg = 0.40, P = 5.26 × 10−11), AUDIT_P (Rg = 0.28, P = 1.36 × 10−05), and weak positive genetic correlation between DCC and SCZ (Rg = 0.10, P = 0.0117) (Table 1). In addition, DCC was negatively associated with neuroticism (Rg = −0.15, P = 7.27 × 10−05), MDD (Rg = −0.15, P = 0.0010), and insomnia (Rg = −0.15, P = 0.0007). No significant genetic correlations were observed of DCC with AD, ALS, ADHD, BIP, and AN (Table 1).
Cross-Trait Meta-Analysis
Considering the strong genetic correlation between DCC and seven neuropsychiatric traits, we performed a cross-trait analysis to improve our power to detect shared genetic loci between them. Shared genetic loci were defined by selecting SNPs with meta-analysis P < 5 × 10−08 and single-trait P < 0.05. In this analysis, we identified 73 independent loci totally, 32 of which were not genome-wide significant in single-trait GWAS of both DCC and neuropsychiatric traits (Table 2, Supplementary Table 2).
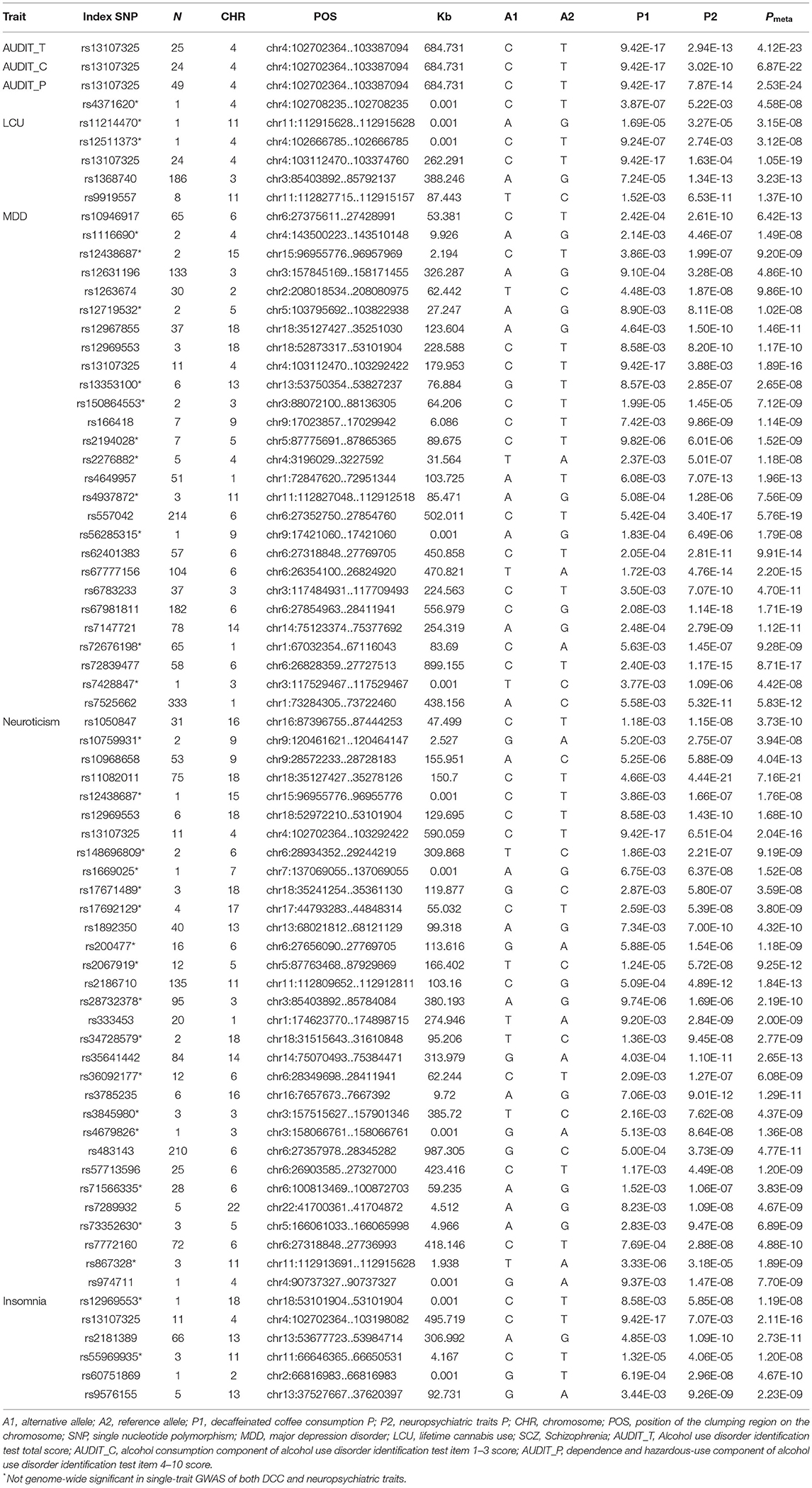
Table 2. Summary of the 73 genomic loci associated with decaffeinated coffee consumption and neuropsychiatric traits in cross-trait meta-analysis.
We identified two overlapped loci across all neuropsychiatric traits. One locus (index SNP: rs13107325) was identified in the genome-wide cross-trait meta-analysis of DCC with insomnia (Pmeta = 2.11 × 10−16), neuroticism (Pmeta = 2.04 × 10−16), LCU (Pmeta = 1.05 × 10−19), AUDIT_T (Pmeta = 4.12 × 10−23), AUDIT_C (Pmeta = 6.87 × 10−22), AUDIT_P (Pmeta = 2.53 × 10−24), and MDD (Pmeta = 1.89 × 10−16). And rs13107325 was also the strongest signal observed for insomnia, LCU, AUDIT_T, AUDIT_C, and AUDIT_P. This locus was mapped to the protein-coding gene SLC39A8, which encodes for ZIP8, a divalent metal ion transporter. The encoded protein is glycosylated and found in the plasma membrane and mitochondria, and functions in the cellular import of zinc at the onset of inflammation (41, 42). The other locus (index SNP: rs12969553) was significantly associated with both DCC and insomnia (Pmeta = 1.20 × 10−08), neuroticism (Pmeta = 1.68 × 10−10), and MDD (Pmeta = 1.17 × 10−10) in the cross-trait meta-analysis. This locus was close to gene TCF4, which encodes a helix-loop-helix transcription factor widely expressed throughout the body and during neural development and plays an important role in nervous system development. Moreover, polymorphisms in TCF4 have been associated with many psychiatric and neurological conditions (43).
In addition to two overlapped loci, we identified 29 genome-wide significantly independent loci for DCC and neuroticism. The strongest association signal was observed on chromosome 18 at the CELF4 region (index SNP rs11082011, Pmeta = 7.16 × 10−21). CELF4 was expressed primarily in excitatory neurons and encoded RNA-binding protein involved in pre- and postsynaptic neurotransmission, which played a vital role in human brain development and regulation of synaptic function (44, 45). The second strongest signal was the overlapped loci rs13107325. And the third strongest signal was mapped to NCAM1 on chromosome 11 (index SNP rs2186710, Pmeta = 1.84 × 10−13). This gene encoded a cell adhesion protein which is a member of the immunoglobulin superfamily. The encoded protein is involved in cell-to-cell interactions as well as cell-matrix interactions during development and differentiation and played a role in the development of the nervous system by regulating neurogenesis, neurite outgrowth, and cell migration, as well as in immune surveillance by the expansion of immune cells (46, 47).
In addition, we identified 27 genome-wide significant independent loci for DCC and MDD. The strongest association signal was mapped to zine finger protein genes (ZNFs), OR2B6, and NKAPL (index SNP rs67981811, Pmeta = 1.71 × 10−19). Most of ZNFs encoded the proteins that are transcription factors that function by binding to specific DNA sequences and mediating protein-protein interactions. Zinc finger genes have been reported to be associated with psychiatric disorders (48). The protein encoded by NKAPL acted as a transcriptional repressor of Notch-mediated signaling required for T-cell development (49). OR2B6 encoded Olfactory receptor proteins, which interact with odorant molecules in the nose, to initiate a neuronal response that triggers the perception of a smell. The second strongest signal was observed on chromosome 16, located near to histone family gene set (index SNP rs557042, Pmeta = 5.76 × 10−19). Histones play a central role in transcription regulation, DNA repair, DNA replication, and chromosomal stability; these gene set also affects epigenetic pathways. The overlapped loci rs13107325 was the third strongest signal for DCC and MDD. The next strongest signal observed on Butyrophilin (BTN) family genes was another common finding in the genome-wide cross-trait meta-analysis of DCC and MDD (index SNP rs67777156, Pmeta = 2.20 × 10−15). Among its related pathways are Butyrophilin (BTN) family interactions and Class I MHC-mediated antigen processing and presentation (20).
Tissue Enrichment Analysis of Shared Genes
To identify the tissue of enriched expression of shared genes between DCC and neuropsychiatric traits, we performed a tissue-specific enrichment analysis using RNA-Seq data from the Human Protein Atlas (HPA) and GTEx. A total of 13 tissues were found with an enriched expression of shared genes between DCC and neuroticism, in addition to six tissues for MDD. The mainly enriched tissues contained bone marrow, lymph node, cervix, uterine, cerebellar cortex, lung, tonsil, and thyroid gland, and main part of the nervous system, exo-/endocrine system, and hemic and immune system. We also found nine and eight enriched tissues identified for shared genes of DCC and insomnia, and LCU, respectively. Tissues like the cervix, uterine, tonsil, appendix, lung, lymph node, and thyroid gland were enriched. Meanwhile, shared genes of DCC and AUDIT were enriched in two tissues, uterine cervix, and lung (Supplementary Figures 1–5).
Over-representation Enrichment Analysis
The GO analysis showed that shared genes between DCC and neuroticism or MDD were significantly enriched in several shared biological processes, such as nucleosome organization, protein-DNA complex assembly, T cell receptor signaling pathway, and antigen receptor-mediated signaling pathway (Supplementary Table 4). Additional analysis of the Reactome pathway indicated that the association signals shared between DCC and neuroticism or MDD were significantly enriched in developmental biological, genetic, and epigenetic pathways, as well as immune-related pathways (Supplementary Table 5).
Fine-Mapping and Co-localization Analysis
In fine-mapping analysis, we got lists of credible set SNPs shown in Supplementary Table 6 for each shared loci in the cross-trait meta-analysis results. The co-localization analysis showed that two loci (rs10968658, rs28732378) contained shared casual variant between DCC/neuroticism, in addition to two loci (rs13107325, rs1368740) for DCC/LCU, and one locus (rs150864553) for DCC/MDD. The loci for DCC/AUDIT all shared casual variants, which was consistent with the cross-trait meta-analysis (Supplementary Table 7).
Mendelian Randomization (MR)
To examine whether there was evidence for the causal effect of DCC on neuropsychiatric disorders risk and vice versa, we performed a bidirectional two-sample Mendelian randomization analysis and got a robust MR analysis result shown after removing all the SNPs with a P-value <1 in the MR-PRESSO outlier test (Table 3, Supplementary Table 3). The instruments used are shown in Supplementary Tables 16–41. In our main analysis, inverse-variance-weighted (IVW) regression analysis, we found some strong evidence for a causal negative influence of DCC on MDD risk and a causal positive influence of DCC on LCU, the IVW regression odds ratio being 0.90 (95% CI: 0.85–0.95, P = 9.06 × 10−5) and 1.27 (95% CI: 1.11–1.46, P = 7.63 × 10−4), respectively. In the exploration of the causal effect of neuropsychiatric traits on DCC, we observed suggestive associations of higher genetically predicted AUDIT_T and AUDIT_C with DCC. For 1-SD increase in genetically predicted AUDIT_T and AUDIT_C, the OR was 1.83 (95% CI: 1.39–2.40, P = 1.67 × 10−05) and 1.80 (95% CI: 1.29–2.51, P = 5.09 × 10−04), respectively. Meanwhile, our results suggested that genetically predicted insomnia and MDD were negatively associated with DCC (OR: 0.91, 95% CI: 0.86–0.95, P = 1.51 × 10−04for Insomnia; OR: 0.93, 95% CI: 0.89–0.99, P = 6.02 × 10−04 for MDD). Besides, we found that there was no significant evidence for a causal relationship between DCC and other neuropsychiatric traits (Table 3, Supplementary Table 3). No significant horizontal pleiotropy and heterogeneity were detected in our last sensitivity analysis (Table 3). In addition, plots of the leave-one-out analysis supported that there was no potentially influential SNP driving the causal link and our conclusion was of stability (Supplementary Figures 6–29).
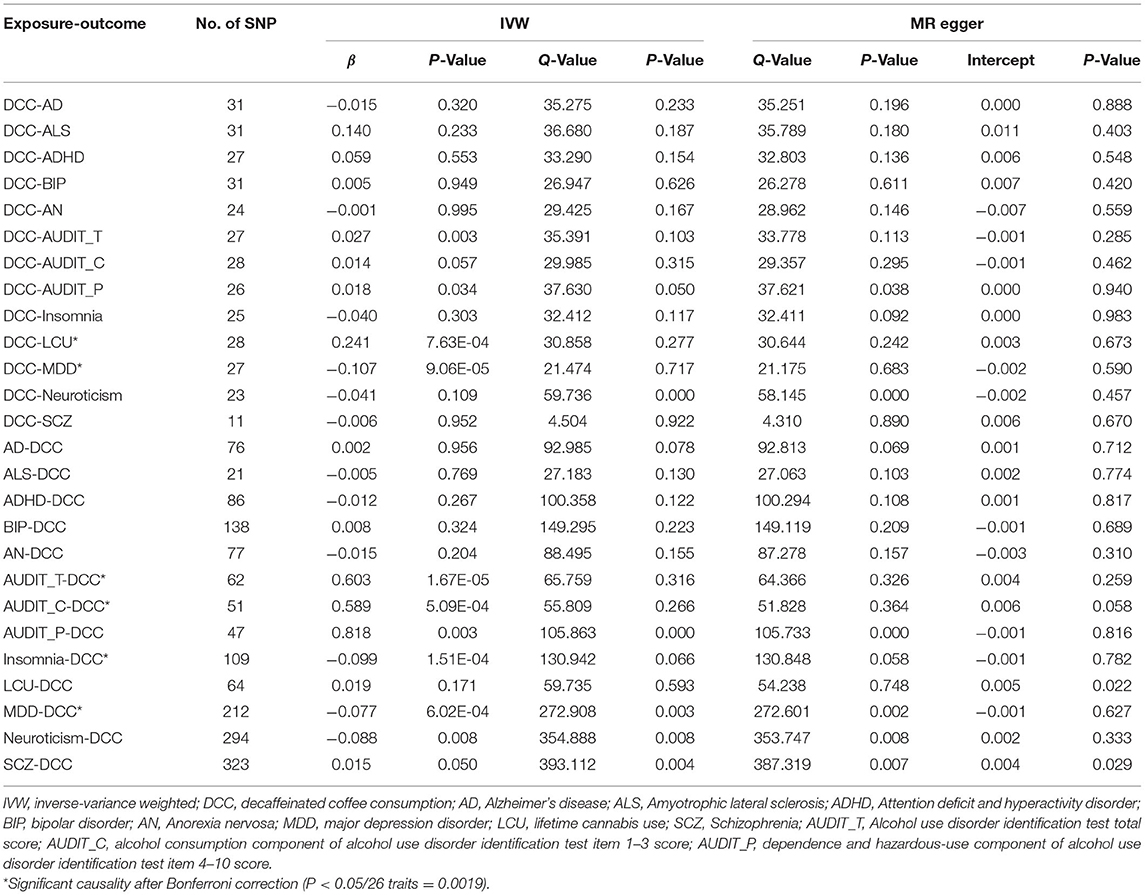
Table 3. The bidirectional MR analysis of decaffeinated coffee consumption and neuropsychiatric traits.
Single-Trait TWAS
To evaluate the genes whose expression is related to DCC or neuropsychiatric traits and the common genes shared between those single-trait TWAS significant genes for DCC and neuropsychiatric traits, we performed a TWAS analysis. In total, we identified 74 gene-tissue pairs significantly associated with DCC across 48 GTEx tissues, in addition to 1,950, 10,802, 983, 5,876, 1,542, 1,400, and 96 gene-tissue pairs with insomnia, neuroticism, LCU, MDD, AUDIT_T, AUDIT_C, and AUDIT_P, respectively (Supplementary Tables 8–15). A large of TWAS-significant associations were identified in the brain, nerve, esophagus, colon, thyroid, testis, and adipose tissue, which are part of the nervous system, digestive system, and exo-/endocrine system. TWAS suggested that neuropsychiatric traits and DCC were regulated by a complex biological network. Among these associations, two gene-tissue pairs (CYP21A2-Brain Cerebellum, ZSCAN9-Brain Cerebellum) were overlapped in TWAS for DCC and MDD.
Discussion
In the present study, we found strong genetic correlations between DCC and seven neuropsychiatric traits (insomnia, MDD, neuroticism, LCU, AUDIT_T, AUDIT_C, AUDIT_P) and identified common variants underlying these associations. Functional enrichment analysis was performed to suggest functions of the mapped genes. In addition, our bidirectional MR analysis suggested that DCC had a significant causal effect on an increased risk of LCU and a protective effect on MDD risk. Higher AUDIT_T and AUDIT_C may increase habitual DCC, but insomnia and MDD may decrease habitual DCC. These results advance our understanding of the common genetic structure between DCC and neuropsychiatric traits and reveal the causality between these traits, which demonstrated the shared etiologies and mechanisms in co-occurring DCC and neuropsychiatric traits.
Our cross-trait meta-analysis could capture the significant signals from both DCC and neuropsychiatric traits associated with GWAS summary statistics with great power. We identified 31 shared loci between DCC and neuroticism, 27 shared loci between DCC and MDD, six shared loci between DCC and insomnia, five shared loci between DCC and LCU, two shared loci between DCC and AUDIT_P, 1 shared locus between DCC and AUDIT_T, and 1 shared locus between DCC and AUDIT_C. Among them, 32 loci were not genome-wide significant in single-trait GWAS of both DCC and neuropsychiatric traits. Our results highlighted the important overlapped gene SLC39A8 (index SNP: rs13107325) identified by the cross-trait meta-analysis of seven genetically correlated trait pairs. Furthermore, our colocation analysis also showed that this locus had a great probability of containing shared causal variants of DCC and LCU (89%), as well as DCC and AUDIT (99%). SLC39A8 encodes a member of the SLC39 family of metal-ion transporters, which is glycosylated and found in the plasma membrane and mitochondria and involved in the cellular transport of zinc, the modulation of which could affect microglial inflammatory responses (50). Studies have reported that SLC39A8 was associated with caffeine consumption, alcohol consumption, and neuroticism (18, 51, 52). However, its contribution to LCU, insomnia, and MDD remains to be determined. The dysfunction of SLC39A8 had an impact on alterations in glutamate and immune function, for example, a reduction in GluN2A and GluA1/2/3 receptor surface expression, decreased BBB integrity, and increased IL-6/IL-1β protein expression (53), which may indicate that the occurrence of DCC and MDD, and LCU and insomnia is medicated by glutamate signaling and altered immune and inflammatory signals (54, 55).
Another overlapped gene TCF4, shared by three trait pairs (DCC/insomnia, DCC/neuroticism, and DCC/MDD), regulates the expression of cell adhesion molecules to control neuronal positioning during brain development (56). Polymorphisms in TCF4 were implicated in many psychiatric and neurological conditions (43). We also found several common variants (rs11214470 for LCU, rs867328 for neuroticism, rs4937872 for MDD) rarely reported in GWAS for DCC, LCU, neuroticism, and MDD. These variants were all mapped to NCAM1, encoding a cell adhesion protein. Secreted NCAM1 can bind to other extracellular molecules such as tenascin and the FGF receptor, all of which can form assemblies between different cells and guide cellular migration, neurogenesis, synaptic processes, and learning (46). So its worth further study to investigate how DCC and these three traits are similarly involved in synaptic plasticity, neurodevelopment, and neurogenesis. In addition, the strongest association in DCC/neuroticism meta-analysis was near to CELF4, expressed primarily in excitatory neurons. It regulates excitatory neurotransmission and binds to at least 15%−20% of transcriptomes, prominently playing in regulating and shaping the activity of neural circuits (45). Furthermore, our results found several genes or gene set significantly associated with DCC and MDD, including ZNFs (ZKSCAN4), BTNs (BTN1A1, BTN2A2, BTN3), and OR2B6. A large group of human ZNFs that contain a KRAB- and SCAN-box like ZKSCZN4 are thought to bind to DNA via their zinc finger domain, followed by transcriptional repression via the KRAB-box (48). BTNs are regulators of immune responses. Some of them such as BTN1A1 and BTN2A2 inhibited the proliferation of CD4+ and CD8+ T-cells and reduce the expression of IL-2 and INF-γ (57). BTN3A inhibited apoptosis for the increased survival of monocytes and dendritic cells, enhancing the synthesis of IL-1, IL-8, and IL-12. This is consistent with evidence showing the link between MDD and elevated proinflammatory cytokines (55, 58), as well as the association between coffee consumption and anti-inflammatory effects (23). Taken together, our results support that the genetic basis of DCC and neuropsychiatric traits are likely attributed to potential biological mechanisms of neurodevelopment, neural circuits, and immune response.
Our functional enrichment analysis provided several enriched tissues and biological pathways of the shared gene between DCC and neuropsychiatric traits involved. Tissue enrichment analysis showed that shared genes were enriched in nervous tissues (cerebellar cortex), exo-/endocrine tissues (thyroid gland), or hemic and immune tissues (Bone marrow, lymph node, tonsil), and lung. These findings provide clues for further study to investigate the potential common genetics in these enriched tissues. Reactome enrichment of HATs acetylate histones, HDACs deacetylate histones, HDMs demethylate histones, DNA methylation, and developmental biology together suggested the effects of both genetic and epigenetics on DCC/MDD and DCC/neuroticism. A study has revealed the shared pathway including DNA methylation between MDD and Insomnia (20). Inhibition of DNA methyltransferase 3a by polyphenol from coffee was also confirmed (59). These results imply the genetic and epigenetic contribution to both DCC and psychiatric disorders. Our GO analysis also showed pathway enrichment in the T cell receptor signaling pathway and antigen receptor-mediated signaling pathway. This is consistent with the anti-inflammatory effects of coffee and elevated proinflammatory cytokines of MDD (55, 58). Our functional analysis provides a new direction in studying epigenetic, developmental biology, or immune-related pathways in DCC and MDD or neuroticism.
In single-trait TWAS, large significant associations were identified in the brain, nerve, esophagus, colon, thyroid, testis, and adipose tissue, which are part of the nervous system, digestive system, and exo-/endocrine System. Our results suggested that neuropsychiatric traits and DCC were regulated by a complex biological network. Among these associations, two gene-tissue pairs (CYP21A2-Brain Cerebellum, ZSCAN9-Brain Cerebellum) were overlapped in TWAS of DCC and MDD. This suggested that studies need to pay more attention to the roles of the cerebellum region in DCC and MDD.
From bidirectional MR analysis, our findings indicated a causal influence of liability to DCC on LCU and low risk of MDD. The effects of coffee are commonly attributed to coffee's caffeine content. However, a study with 2,232 middle-aged men in Finland found no association between intake of caffeine and depression (60). A recent MR analysis also found little evidence to establish a causal relationship between caffeine assumption and cannabis use (61). Other compounds in coffee, such as chlorogenic acids and related compounds (quinines, caffeic acid), were found in amounts much more than that of caffeine and were thought to be responsible for antidepressant effects (62). These results provide novel insight into the role of compounds in decaffeinated coffee. On the other side, we found strong evidence for a causal relationship from high AUDIT_T and AUDIT_C to DCC but found no evidence for a causal relationship between DCC and AUDIT_P scores, confirming the different genetics between AUDIT subsets (63) and providing information for further study. Our results also suggested that insomnia and MDD had a causal negative influence on DCC. These may be explained by that the caffeine in caffeinated coffee acts primarily as a central nervous system stimulant, improving psychomotor performance, increasing vigilance, and reducing fatigue (21), which is what insomnia or MDD complainants look forward to. But it is worth drawing cautious conclusions on the influence of MDD on DCC because of the potential heterogeneity. Our MR findings provided evidence for relationships between DCC and neuropsychiatric traits, highlighting the important role of non-caffeine content in coffee. It also deserves further study to investigate whether and how caffeine affects or participates in the metabolism or mechanism of non-caffeine components in caffeinated coffee.
However, some limitations existed. First, lack of data on the amounts of compounds like chlorogenic acids present in the decaffeinated coffee regularly consumed by study participants. But qualitative research still can provide strong evidence to some extent. Second, the GWAS data from meta-analysis studies have great power but reduces the homogeneity. Fortunately, the method CPASSOC can deal with population structure and cryptic relatedness.
In conclusion, we found strong genetic correlations between DCC and neuropsychiatric disorders and identified shared common variants underlying these associations, suggesting a common genetic influence across these traits. Functional analysis showed epigenetic, developmental biology, or immune-related pathways in DCC and MDD or neuroticism, providing new directions in common biology process studies. MR analysis found strong evidence for a causal relationship between DCC and six neuropsychiatric traits (insomnia, MDD, LCU, AUDIT_T, AUDIT_C, AUDIT_P). These findings advance our understanding of the shared genetic mechanisms and causality underlying their associations, assisting with making recommendations for clinical works or health education.
Data Availability Statement
The original contributions presented in the study are included in the article/Supplementary Material, further inquiries can be directed to the corresponding authors.
Author Contributions
BY performed the statistical analysis and wrote the manuscript. All authors designed the study, helped interpret the data, reviewed and edited the final paper, and approved the submission.
Funding
The study was supported by grants from the Peking University Start-up Grant (BMU2018YJ002), the High-performance Computing Platform of Peking University. The funding organization had no role in the preparation of the manuscript.
Conflict of Interest
The authors declare that the research was conducted in the absence of any commercial or financial relationships that could be construed as a potential conflict of interest.
Publisher's Note
All claims expressed in this article are solely those of the authors and do not necessarily represent those of their affiliated organizations, or those of the publisher, the editors and the reviewers. Any product that may be evaluated in this article, or claim that may be made by its manufacturer, is not guaranteed or endorsed by the publisher.
Supplementary Material
The Supplementary Material for this article can be found online at: https://www.frontiersin.org/articles/10.3389/fpsyt.2022.910432/full#supplementary-material
References
1. Pirastu N, Kooyman M, Robino A, Van Der Spek A, Navarini L, Amin N, et al. Non-additive genome-wide association scan reveals a new gene associated with habitual coffee consumption. Sci Rep. (2016) 6:1–6. doi: 10.1038/srep31590
2. Lucas M, Mirzaei F, Pan A, Okereke OI, Willett WC, O'reilly ÉJ, et al. Coffee, caffeine, and risk of depression among women. Arch Intern Med. (2011) 171:1571–8. doi: 10.1001/archinternmed.2011.393
3. Maia L, De Mendonça A. Does caffeine intake protect from Alzheimer's disease? Eur J Neurol. (2002) 9:377–82. doi: 10.1046/j.1468-1331.2002.00421.x
4. Chu Y-F. Coffee: Emerging Health Effects and Disease Prevention. Hoboken, NJ: John Wiley and Sons. (2012).
5. Kahathuduwa C, Wakefield S, West B, Blume J, Mastergeorge A. L-theanine and caffeine improve sustained attention, impulsivity and cognition in children with attention deficit hyperactivity disorders by decreasing mind wandering (OR29-04-19). Curr Dev Nutr. (2019) 3:nzz031. OR029-004-019. doi: 10.1093/cdn/nzz031.OR29-04-19
6. Kunitake Y, Mizoguchi Y, Sogawa R, Matsushima J, Kato TA, Kawashima T, et al. Effect of excessive coffee consumption on the clinical course of a patient with bipolar disorder: a case report and literature review. Clin Neuropharmacol. (2017) 40:160–2. doi: 10.1097/WNF.0000000000000222
7. Winston AP, Hardwick E, Jaberi N. Neuropsychiatric effects of caffeine. Adv Psychiatr Treat. (2005) 11:432–9. doi: 10.1192/apt.11.6.432
8. Bulik-Sullivan B, Finucane HK, Anttila V, Gusev A, Day FR, Loh P-R, et al. An atlas of genetic correlations across human diseases and traits. Nat Genet. (2015) 47:1236–41. doi: 10.1038/ng.3406
9. Bulik-Sullivan BK, Loh P-R, Finucane HK, Ripke S, Yang J, Patterson N, et al. LD Score regression distinguishes confounding from polygenicity in genome-wide association studies. Nat Genet. (2015) 47:291–5. doi: 10.1038/ng.3211
10. Zheng J, Erzurumluoglu AM, Elsworth BL, Kemp JP, Howe L, Haycock PC, et al. LD Hub: a centralized database and web interface to perform LD score regression that maximizes the potential of summary level GWAS data for SNP heritability and genetic correlation analysis. Bioinformatics. (2017) 33:272–9. doi: 10.1093/bioinformatics/btw613
11. Laitala VS, Kaprio J, Silventoinen K. Genetics of coffee consumption and its stability. Addiction. (2008) 103:2054–61. doi: 10.1111/j.1360-0443.2008.02375.x
12. Vink JM, Staphorsius AS, Boomsma DI. A genetic analysis of coffee consumption in a sample of Dutch twins. Twin Res Hum Genet. (2009) 12:127–31. doi: 10.1375/twin.12.2.127
13. Edvardsen J, Torgersen S, Røysamb E, Lygren S, Skre I, Onstad S, et al. Heritability of bipolar spectrum disorders. Unity or heterogeneity? J Affect Disord. (2008) 106:229–40. doi: 10.1016/j.jad.2007.07.001
14. Lichtenstein P, Yip BH, Björk C, Pawitan Y, Cannon TD, Sullivan PF, et al. Common genetic influences for schizophrenia and bipolar disorder: a population-based study of 2 million nuclear families. Lancet. (2009) 373:234–9. doi: 10.1016/S0140-6736(09)60072-6
15. Franke B, Faraone S, Asherson P, Buitelaar J, Bau C, Ramos-Quiroga JA, et al. The genetics of attention deficit/hyperactivity disorder in adults, a review. Mol Psychiatry. (2012) 17:960–87. doi: 10.1038/mp.2011.138
16. Lind MJ, Aggen SH, Kirkpatrick RM, Kendler KS, Amstadter AB. A longitudinal twin study of insomnia symptoms in adults. Sleep. (2015) 38:1423–30. doi: 10.5665/sleep.4982
17. Viktorin A, Meltzer-Brody S, Kuja-Halkola R, Sullivan PF, Landén M, Lichtenstein P, et al. Heritability of perinatal depression and genetic overlap with nonperinatal depression. Am J Psychiatry. (2016) 173:158–65. doi: 10.1176/appi.ajp.2015.15010085
18. Li J, Choudhury T, Zhang M, Chen L, Wen J, Liu W, et al. Habitual coffee consumption increases risks for metabolic diseases: genome-wide association studies and a phenotype-wide two sample mendelian randomization analysis. medRxiv [Preprint]. (2021). doi: 10.1101/2021.03.08.21253114 (accessed March 08, 2021).
19. Ripke S, Neale BM, Corvin A, Walters JT, Farh K-H, Holmans PA, et al. Biological insights from 108 schizophrenia-associated genetic loci. Nature. (2014) 511:421. doi: 10.1038/nature13595
20. Lin Y-S, Wang C-C, Chen C-Y. GWAS meta-analysis reveals shared genes and biological pathways between major depressive disorder and insomnia. Genes. (2021) 12:1506. doi: 10.3390/genes12101506
21. Chou T. Wake up and smell the coffee. Caffeine, coffee, and the medical consequences. West J Med. (1992) 157:544–53.
22. Cropley V, Croft R, Silber B, Neale C, Scholey A, Stough C, et al. Does coffee enriched with chlorogenic acids improve mood and cognition after acute administration in healthy elderly? A pilot study. Psychopharmacology. (2012) 219:737–49. doi: 10.1007/s00213-011-2395-0
23. Açikalin B, Sanlier N. Coffee and its effects on the immune system. Trends Food Sci Technol. (2021) 144:625–32. doi: 10.1016/j.tifs.2021.06.023
24. Benn M, Nordestgaard BG. From genome-wide association studies to Mendelian randomization: novel opportunities for understanding cardiovascular disease causality, pathogenesis, prevention, and treatment. Cardiovasc Res. (2018) 114:1192–208. doi: 10.1093/cvr/cvy045
25. Zhu X, Feng T, Tayo BO, Liang J, Young JH, Franceschini N, et al. Meta-analysis of correlated traits via summary statistics from GWASs with an application in hypertension. Am J Hum Genet. (2015) 96:21–36. doi: 10.1016/j.ajhg.2014.11.011
26. Farh KK, Marson A, Zhu J, Kleinewietfeld M, Housley WJ, Beik S, et al. Genetic and epigenetic fine mapping of causal autoimmune disease variants. Nature. (2015) 518:337–43. doi: 10.1038/nature13835
27. Huang H, Fang M, Jostins L, Umićević Mirkov M, Boucher G, Anderson CA, et al. Fine-mapping inflammatory bowel disease loci to single-variant resolution. Nature. (2017) 547:173–8. doi: 10.1038/nature22969
28. Dadaev T, Saunders EJ, Newcombe PJ, Anokian E, Leongamornlert DA, Brook MN, et al. Fine-mapping of prostate cancer susceptibility loci in a large meta-analysis identifies candidate causal variants. Nat Commun. (2018) 9:2256. doi: 10.1038/s41467-018-04109-8
29. Plagnol V, Smyth DJ, Todd JA, Clayton DG. Statistical independence of the colocalized association signals for type 1 diabetes and RPS26 gene expression on chromosome 12q13. Biostatistics. (2009) 10:327–34. doi: 10.1093/biostatistics/kxn039
30. Wallace C. Statistical testing of shared genetic control for potentially related traits. Genet Epidemiol. (2013) 37:802–13. doi: 10.1002/gepi.21765
31. Giambartolomei C, Vukcevic D, Schadt EE, Franke L, Hingorani AD, Wallace C, et al. Bayesian test for colocalisation between pairs of genetic association studies using summary statistics. PLoS Genet. (2014) 10:e1004383. doi: 10.1371/journal.pgen.1004383
32. Zhu Z, Lee PH, Chaffin MD, Chung W, Loh PR, Lu Q, et al. A genome-wide cross-trait analysis from UK Biobank highlights the shared genetic architecture of asthma and allergic diseases. Nat Genet. (2018) 50:857–64. doi: 10.1038/s41588-018-0121-0
33. Zhuang Z, Yao M, Wong JYY, Liu Z, Huang T. Shared genetic etiology and causality between body fat percentage and cardiovascular diseases: a large-scale genome-wide cross-trait analysis. BMC Med. (2021) 19:100. doi: 10.1186/s12916-021-01972-z
34. Consortium G. The Genotype-Tissue Expression (GTEx) pilot analysis: multitissue gene regulation in humans. Science. (2015) 348:648–60. doi: 10.1126/science.1262110
35. Uhlén M, Fagerberg L, Hallström BM, Lindskog C, Oksvold P, Mardinoglu A, et al. Tissue-based map of the human proteome. Science. (2015) 347:1260419. doi: 10.1126/science.1260419
36. Ashburner M, Ball CA, Blake JA, Botstein D, Butler H, Cherry JM, et al. Gene ontology: tool for the unification of biology. Nat Genet. (2000) 25:25–9. doi: 10.1038/75556
37. Consortium GO. The gene ontology resource: 20 years and still GOing strong. Nucleic Acids Res. (2019) 47:D330–8. doi: 10.1093/nar/gky1055
38. Burgess S, Dudbridge F, Thompson SG. Combining information on multiple instrumental variables in Mendelian randomization: comparison of allele score and summarized data methods. Stat Med. (2016) 35:1880–906. doi: 10.1002/sim.6835
39. Wu F, Huang Y, Hu J, Shao Z. Mendelian randomization study of inflammatory bowel disease and bone mineral density. BMC Med. (2020) 18:1–19. doi: 10.1186/s12916-020-01778-5
40. Gusev A, Ko A, Shi H, Bhatia G, Chung W, Penninx BW, et al. Integrative approaches for large-scale transcriptome-wide association studies. Nat Genet. (2016) 48:245–52. doi: 10.1038/ng.3506
41. Aydemir TB, Liuzzi JP, Mcclellan S, Cousins RJ. Zinc transporter ZIP8 (SLC39A8) and zinc influence IFN-γ expression in activated human T cells. J Leukoc Biol. (2009) 86:337–48. doi: 10.1189/jlb.1208759
42. Liu MJ, Bao S, Gálvez-Peralta M, Pyle CJ, Rudawsky AC, Pavlovicz RE, et al. ZIP8 regulates host defense through zinc-mediated inhibition of NF-κB. Cell Rep. (2013) 3:386–400. doi: 10.1016/j.celrep.2013.01.009
43. Wirgenes KV, Sønderby IE, Haukvik UK, Mattingsdal M, Tesli M, Athanasiu L, et al. TCF4 sequence variants and mRNA levels are associated with neurodevelopmental characteristics in psychotic disorders. Transl Psychiatry. (2012) 2:e112. doi: 10.1038/tp.2012.39
44. Halgren C, Bache I, Bak M, Myatt MW, Anderson CM, Brøndum-Nielsen K, et al. Haploinsufficiency of CELF4 at 18q12.2 is associated with developmental and behavioral disorders, seizures, eye manifestations, and obesity. European Journal of Human Genetics. (2012) 20:1315–9. doi: 10.1038/ejhg.2012.92
45. Wagnon JL, Briese M, Sun W, Mahaffey CL, Curk T, Rot G, et al. CELF4 regulates translation and local abundance of a vast set of mRNAs, including genes associated with regulation of synaptic function. PLoS Genet. (2012) 8:e1003067. doi: 10.1371/journal.pgen.1003067
46. Atz ME, Rollins B, Vawter MP. NCAM1 association study of bipolar disorder and schizophrenia: polymorphisms and alternatively spliced isoforms lead to similarities and differences. Psychiatr Genet. (2007) 17:55. doi: 10.1097/YPG.0b013e328012d850
47. Guven A, Gray K, Peng KW, Klotz A, Kellogg MD, Narain NR, et al. Development and validation of an immunoassay for quantification of NCAM-1 in human plasma. J Pharm Biomed Anal. (2021) 197:113981. doi: 10.1016/j.jpba.2021.113981
48. Sun Y, Hu D, Liang J, Bao Y-P, Meng S-Q, Lu L, et al. Association between variants of zinc finger genes and psychiatric disorders: systematic review and meta-analysis. Schizophr Res. (2015) 162:124–37. doi: 10.1016/j.schres.2015.01.036
49. Pajerowski AG, Nguyen C, Aghajanian H, Shapiro MJ, Shapiro VS. NKAP is a transcriptional repressor of notch signaling and is required for T cell development. Immunity. (2009) 30:696–707. doi: 10.1016/j.immuni.2009.02.011
50. Higashi Y, Aratake T, Shimizu S, Shimizu T, Nakamura K, Tsuda M, et al. Influence of extracellular zinc on M1 microglial activation. Sci Rep. (2017) 7:1–13. doi: 10.1038/srep43778
51. Okbay A, Baselmans BM, De Neve J-E, Turley P, Nivard MG, Fontana MA, et al. Genetic variants associated with subjective well-being, depressive symptoms, and neuroticism identified through genome-wide analyses. Nat Genet. (2016) 48:624–33. doi: 10.1101/032789
52. Evangelou E, Gao H, Chu C, Ntritsos G, Blakeley P, Butts AR, et al. New alcohol-related genes suggest shared genetic mechanisms with neuropsychiatric disorders. Nat Hum Behav. (2019) 3:950–61. doi: 10.1038/s41562-019-0653-z
53. Tseng WC, Reinhart V, Lanz TA, Weber ML, Pang J, Le KXV, et al. Schizophrenia-associated SLC39A8 polymorphism is a loss-of-function allele altering glutamate receptor and innate immune signaling. Transl Psychiatry. (2021) 11:136. doi: 10.1038/s41398-021-01262-5
55. Das R, Emon MPZ, Shahriar M, Nahar Z, Islam SMA, Bhuiyan MA, et al. Higher levels of serum IL-1β and TNF-α are associated with an increased probability of major depressive disorder. Psychiatry Res. (2021) 295:113568. doi: 10.1016/j.psychres.2020.113568
56. Zhang Y, Cai Z, Hu G, Hu S, Wang Y, Li N, et al. Transcription factor 4 controls positioning of cortical projection neurons through regulation of cell adhesion. Mol Psychiatry. (2021) 26:6562–77. doi: 10.1038/s41380-021-01119-9
57. Smith IA, Knezevic BR, Ammann JU, Rhodes DA, Aw D, Palmer DB, et al. BTN1A1, the mammary gland butyrophilin, and BTN2A2 are both inhibitors of T cell activation. J Immunol. (2010) 184:3514–25. doi: 10.4049/jimmunol.0900416
58. Rhodes DA, Chen H-C, Price AJ, Keeble AH, Davey MS, James LC, et al. Activation of human γδ T cells by cytosolic interactions of BTN3A1 with soluble phosphoantigens and the cytoskeletal adaptor periplakin. J Immunol. (2015) 194:2390–8. doi: 10.4049/jimmunol.1401064
59. Rajavelu A, Tulyasheva Z, Jaiswal R, Jeltsch A, Kuhnert N. The inhibition of the mammalian DNA methyltransferase 3a (Dnmt3a) by dietary black tea and coffee polyphenols. BMC Biochem. (2011) 12:1–8. doi: 10.1186/1471-2091-12-16
60. Ruusunen A, Lehto SM, Tolmunen T, Mursu J, Kaplan GA, Voutilainen S. Coffee, tea and caffeine intake and the risk of severe depression in middle-aged Finnish men: the Kuopio ischaemic heart disease risk factor study. Public Health Nutr. (2010) 13:1215–20. doi: 10.1017/S1368980010000509
61. Chang L-H, Ong J-S, An J, Verweij KJ, Vink JM, Pasman J, et al. Investigating the genetic and causal relationship between initiation or use of alcohol, caffeine, cannabis and nicotine. Drug Alcohol Depend. (2020) 210:107966. doi: 10.1016/j.drugalcdep.2020.107966
62. Santos R, Hunter T, Lima D. Coffee, depression, alcoholism and drug abuse–a mini-review. Austin J Pharmacol Ther. (2014) 1:6.
63. Sanchez-Roige S, Palmer AA, Fontanillas P, Elson SL;, 23andMe Research Team the the Substance Use Disorder Working Group of the Psychiatric Genomics Consortium . Genome-wide association study meta-analysis of the Alcohol Use Disorders Identification Test (AUDIT) in two population-based cohorts. Am J Psychiatr. (2019) 176:107–18. doi: 10.1176/appi.ajp.2018.18040369
Keywords: coffee consumption, neuropsychiatric diseases, shared genetics, genetic correlation, Mendelian randomization, causality
Citation: Yin B, Wang X, Huang T and Jia J (2022) Shared Genetics and Causality Between Decaffeinated Coffee Consumption and Neuropsychiatric Diseases: A Large-Scale Genome-Wide Cross-Trait Analysis and Mendelian Randomization Analysis. Front. Psychiatry 13:910432. doi: 10.3389/fpsyt.2022.910432
Received: 01 April 2022; Accepted: 15 June 2022;
Published: 11 July 2022.
Edited by:
Feng Liu, Tianjin Medical University General Hospital, ChinaReviewed by:
Moinak Banerjee, Rajiv Gandhi Centre for Biotechnology, IndiaYong Wu, Wuhan Mental Health Center, China
Copyright © 2022 Yin, Wang, Huang and Jia. This is an open-access article distributed under the terms of the Creative Commons Attribution License (CC BY). The use, distribution or reproduction in other forums is permitted, provided the original author(s) and the copyright owner(s) are credited and that the original publication in this journal is cited, in accordance with accepted academic practice. No use, distribution or reproduction is permitted which does not comply with these terms.
*Correspondence: Jinzhu Jia, anpqaWFAbWF0aC5wa3UuZWR1LmNu; Tao Huang, aHVhbmd0YW90YW9AcGt1LmVkdS5jbg==