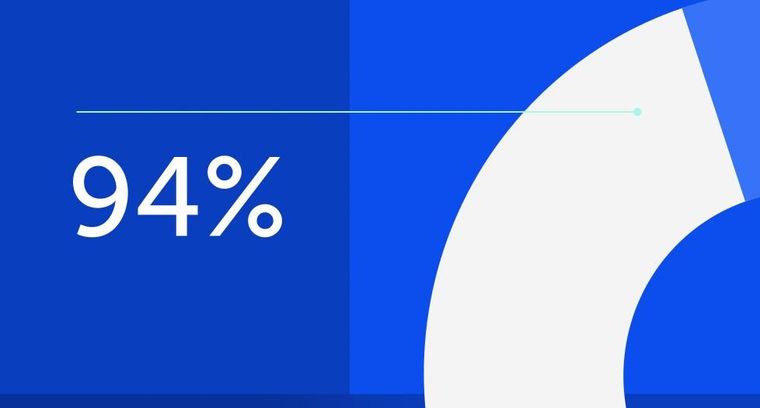
94% of researchers rate our articles as excellent or good
Learn more about the work of our research integrity team to safeguard the quality of each article we publish.
Find out more
BRIEF RESEARCH REPORT article
Front. Psychiatry, 21 June 2022
Sec. Public Mental Health
Volume 13 - 2022 | https://doi.org/10.3389/fpsyt.2022.891479
This article is part of the Research TopicCardiometabolic Disease and Psychiatric DisordersView all 7 articles
Background: High prevalence of Metabolic Syndrome (MS) was found in patients with schizophrenia and bipolar disorders. Insulin Resistance (IR) seems to mediate MS role in developing cardiometabolic consequences.
Aims: To investigate the prevalence of MS, and the role of MS components and IR surrogate indexes in determining MS in adult ADHD outpatients.
Methods: In the present cross-sectional study, MS, defined according to the Expert Panel on Detection, Evaluation and Treatment of High Blood Cholesterol in Adults (ATP III), and IR surrogate indexes were assessed on a consecutive sample of adult ADHD outpatients. Logistic regression analysis was performed to evaluate the effect of each ATP III component and IR surrogate index in determining MS.
Results: Seventeen out of 158 patients (10.8%, 95%CI = 0.064/0.167) fulfilled the ATP-III criteria for MS. A comprehensive comparison with prevalence in the reference population was hindered by the lack of patients over 60 in the study sample, however under this age no significant differences were found. Among MS components, blood triglycerides level (OR = 1.02, 95%CI=1.01/1.03, p = 0.001) was the main predictor for MS, followed by diastolic blood pressure (OR = 1.08, 95%CI=1.01/1.16, p = 0.024) and waist circumference (OR = 1.06, 95%CI=1.01/1.13, p = 0.029). Lipid Accumulation Product (LAP, OR = 1.0006, 95%CI=1.0003/1.0009, p < 0.001) outperformed Triglyceride-Waist Circumference (TG-WC, OR=1.03, 95%CI=1.01/1.04, p < 0.001) in predicting MS.
Conclusions: More attention should be paid not only to MS but also to each ATP III component of MS and LAP in ADHD patients both at first assessment and during follow-up process.
Metabolic Syndrome (MS) is a widespread cluster of cardiometabolic risk factors (1) with a great impact on global health, and high morbidity, mortality rate, and public costs (2–4).
The worldwide prevalence of MS is difficult to assess because of the deep variation in habits and mean age shown by different populations. Data about the U.S. population suggest a prevalence of almost 35% mostly driven by a high central obesity rate (5, 6). Consistently, a study conducted in Italy on patients referring to their general practitioner's office for miscellaneous complaints found a prevalence of almost 33%. Patients with MS were noticeably older (mean age = 61.5, SD = 9.3 years) than those who did not meet the criteria (mean age = 56.3, SD = 12.6 years) (7). Ten years before, a prevalence of 18% in men and 15% in women was detected in the Italian general population. Even then, age played an important role because the MS rate changed from 3%, in people between 18 and 25, to 25% in those over 70 (8). A recent epidemiological survey conducted on the European general population (9) has confirmed that the prevalence of MS steadily increases with age.
A comprehensive meta-analysis showed that MS is 58% more frequent in patients with any psychiatric disorder than in the general population (10). High prevalence of MS was detected especially in patients with schizophrenia (11), bipolar disorder (12, 13), and major depressive disorder (14), mostly due to unhealthy lifestyles (15, 16), sleep-wake rhythm disruption (1), and side effects of pharmacological treatments (11, 17, 18). Attention-Deficit/Hyperactivity Disorder (ADHD) is one of the conditions whose correlation with MS has recently been investigated.
ADHD is a pervasive neurodevelopmental disorder resulting in a persistent pattern of inattention, hyperactivity, and impulsivity severe enough to interfere with social, academic, and occupational functioning (19). The onset of ADHD is during childhood with an estimated worldwide prevalence of 3.4% (95%CI = 2.6/4.5) (20), but diagnostic criteria seem to persist in 57% of patients during adulthood when the estimated prevalence is 2.8% (IQR = 1.8–4.1%) (21). A meta-analysis on prospective longitudinal studies has suggested that approximately two-thirds of children with ADHD continue to suffer from impairing symptoms in adulthood (22). Therefore, ADHD is a lifespan persistent disorder that brings with it a higher risk of both psychiatric and physical comorbidities (23, 24). Some recent meta-analyses have reported higher rates of different medical conditions among patients with ADHD than controls, such as migraine [OR = 1.32, (25)], asthma [OR = 1.34, (26)], atopic eczema [OR = 1.32, (26)], coeliac disease [OR = 1.39, (27)].
To the best of our knowledge, only a recent European study has investigated the relationship between ADHD and MS, also considering the impact of comorbid affective disorders. No significant differences in the MS prevalence have been detected between ADHD with anxiety/depressive disorders (23.9%), ADHD without anxiety/depressive disorders (19.9%) and controls (19.0%, χ2[2] = 2.08, p = 0.384) (28).
As stated before, the definition and the actual prevalence of MS have recently been challenged by some authors (29, 30), however, every single MS diagnostic criterion is still recognized as an independent risk factor for cardiovascular disease (31, 32).
Moreover, though it is well known that Insulin Resistance (IR) plays a key role in the MS development (33, 34), MS and IR are mutually independent predictors of cardiovascular risk (35) and IR seems to be one of the most significant determinants of endothelial dysfunction in MS (36). The most reliable procedure to assess IR is the hyperinsulinemic-euglycemic clamp that is too cumbersome in terms of time and cost. Homeostasis Model Assessment-Insulin Resistance (HOMA-IR), instead, still requires insulinemia determination, which is not commonly included in the standard blood panel. However, different surrogate indexes of IR have been proposed and recently validated (37–39). Among these Triglyceride-Waist Circumference (TG-WC) (39) and Lipid Accumulation Product (LAP) (37) have shown to be the most powerful predictors of MS (38, 40). Since TG-WC and LAP have been recently validated, no studies investigated them in ADHD patients.
ADHD is associated with a significant risk for overweight and obesity at all ages (41–43) and, beyond the actual or not ADHD diagnosis, the presence of inattentive and hyperactive/impulsive symptoms during adolescence correlates with larger waist circumference in adulthood (44). ADHD has also been found to significantly associate with type 2 diabetes, which implies IR, and hypertension (45, 46). Furthermore, a Body Mass Index (BMI) over 25 Kg/m2, a diastolic blood pressure higher than 90 mmHg, and an increased level in Low-Density Lipoprotein (LDL) are more likely to be present in adults with ADHD (47). A partial explanation for the metabolic risk could be found in unhealthy dietary habits which are known to be associated with ADHD including late-night eating (a behavior associated with either ADHD and increased BMI) (48, 49) and keeping irregular intervals between meals (50). ADHD also showed a high comorbidity rate with mood disorders (21, 51), which, as reminded before, are associated with a higher rate of MS (13, 52, 53).
The aims of the present study were (a) to investigate the prevalence of MS in an Italian naturalistic sample of adult outpatients with ADHD using the National Cholesterol Education Program—Adult Treatment Panel III (ATP III) criteria and (b) to evaluate the role of the IR surrogate indexes and MS components in determining MS.
The present cross-sectional study was conducted at the adult ADHD outpatient center of the San Luigi Gonzaga University Hospital (Orbassano, Turin, Italy), between January 2019 and January 2020, on a consecutive naturalistic sample of patients newly diagnosed with adult ADHD according to DSM-IV-TR criteria (age>18 years). The recruiting center is located within the University General Hospital and it is a tertiary referral service for the diagnosis and treatment of ADHD in adults, covering the northwestern region of Italy.
The invitation to participate in the study was accompanied by comprehensive information regarding its aims, methods, risks, and benefits. If accepted, the agreement was formalized by the patient signing a written informed consent form. A unique identification code was assigned to each patient in order to maintain data anonymity and patient confidentiality. All procedures involving human subjects/patients were approved by the Research Ethics Committee of the San Luigi Gonzaga University Hospital (Orbassano, Turin, Italy; Prot no. 16/2019) as a part of a wider observational study project. Therefore, the authors assert that all procedures contributing to this work comply with the ethical standards of the relevant national and institutional committees on human experimentation and with the Helsinki Declaration of 1975, as revised in 2008.
ADHD diagnosis was assessed by a comprehensive psychiatric clinical interview followed by the administration of Adult ADHD Self Reporting Scale (ASRS-1.1) screener for adult ADHD; it was then confirmed through the Diagnostic Interview for Adult ADHD (DIVA 2.0). The severity of ADHD symptoms was rated by using the Adult ADHD Investigation Rating Scale (AISRS).
At the moment of their enrollment, patients were not taking any medication for ADHD as they were diagnosed for the first time at the center. Conversely, any other current psychopharmacological treatments were collected according to their main drug class (i.e., mood stabilizers, antidepressants, antipsychotics).
The metabolic syndrome was diagnosed according to the ATP III criteria. Thus, a dichotomous variable (Yes/No) for each MS component was obtained, using ATP III thresholds (i.e., waist circumference >102 cm in men and >88 cm in women; triglycerides levels ≥125 mg/dL; blood pressure ≥130/85 mmHg; High-Density Lipoprotein Cholesterol levels–HDL-C <40 mg/dL in men and <50 mg/dL in women; and fasting glucose values ≥100 mg/dL) and gathering any available information about current lipid-lowering, glucose-lowering, and antihypertensive medications. All patients having at least three out of five components were considered to be affected by MS and thus they were assigned to the MS group. The remainder of the sample was considered as belonging to the no MS group. Each component was also collected as a continuous variable, thereby weight, height, waist circumference and blood pressure were measured. BMI, defined as the ratio of body weight in kilograms and height in square meters, was calculated. Waist circumference, measuring central adiposity, was taken at the point in the middle between ribs' inferior margin and the superior edge of the iliac crest, while patients were exhaling. Blood pressure was detected using a mercury sphygmomanometer before and after examination, then the mean values for both diastolic and systolic blood pressures were collected. Blood concentrations of each blood test required (i.e., triglycerides, HDL-C, and fasting glucose) were derived from the standard blood panel carried on at the first examination.
IR surrogate indexes were calculated according to the original published formulas, i.e., LAP = (WC-65)*TG for men and (WC-58)*TG for women (37); TG-WC = TG*WC (39).
All computations were performed using RStudio for MAC OS (Version 1.1.383, RStudio Inc., Boston, MA, USA).
A comparison between MS group and no MS group was performed: Pearson's χ2 test or Fisher's exact test was used for categorical variables, depending on the expected frequencies in each group, whereas the independent samples Student's t test or the Mann-Whitney's U test was adopted for continuous variables, depending on their distributions previously evaluated by the Shapiro-Wilk's test.
The relationship between MS and age was even appraised comparing the prevalence of MS of the study sample with that of a normative population [i.e., taken from the most recent report on the prevalence of MS in the European general population (9)], both stratified by age.
Multicollinearity between MS components and IR indexes was also assessed by calculating the square root of variance inflation factor (√VIF), indicating the degree to which the confidence interval for each variable regression parameter is expanded relative to a model with uncorrelated predictors. As a general rule, √VIF > 2 was considered as an indicator of a multicollinearity problem (54).
The role of MS components and IR surrogate indexes in determining MS was evaluated by logistic regression. A first model (i.e., COMP model) was built including age and all MS components (i.e., waist circumference, systolic and diastolic blood pressures, triglycerides, HDL-C, and fasting glucose). According to multicollinearity assessment, two further models were performed excluding collinear MS components: The second model (i.e., LAP model) including LAP and the third model (TG-WG model) was with TG-WC.
Finally, the fitting level of the first model was compared to that of the second and the third model by anova() function of the MASS package and through Nagelkerke's pseudo-R2 and Akaike Information Criterion (AIC) calculation.
Probability tests were considered bilateral with a type I error set at 5% (p = 0.05) but p-values resulting from multiple comparisons were adjusted using Sidak's correction to control for the family-wise error rate.
The recruited sample consisted of 158 patients, 112 of which were males (70.9% of the total) and 46 females (29.1%); the mean age was 25 (SD = 14.0) years. The majority of them were referred to the center from a psychiatrist they had consulted for different ailments (n = 53 patients, 33.5% of the sample) but few less decided to undergo an evaluation for ADHD on their own initiative (n = 50 patients, 31.6%). The remaining patients were sent to the center by their child neuropsychiatrist (n = 23, 14.6%), by any association of ADHD children's families (n = 10, 6.3%), by their general practitioner (n = 2, 1.3%) or by their addiction service (n = 2, 1.3%). Eighteen patients (10.8%) were referred to the center by other sources.
Seventeen patients (10.8%, 95%CI = 6.4/16.7%) fulfilled the ATP-III MS criteria for MS, meeting at least three out of five requirements; 37 (23.4%) had the waist circumference criterion, 59 (37.3%) had the hypertension criterion, 43 (27.2%) had the triglycerides criterion, 45 (28.5%) had the HDL criterion, and only 8 (5.1%) had the hyperglycemia criterion.
The prevalence rates of MS stratified by age were compared with those of a normative population [Vishram et al. (9); N = 69.094]. Although the overall rate of our sample is about half of that of normative population (ADHD MS = 10.8% vs. normative MS = 20%, χ2[1] = 7.881, p = 0.005), no differences were found under 60 years (19–39 years, ADHD MS = 9.8% vs. normative MS = 9%, χ2[1] = 0.035, p = 0.852; 40–49 years, ADHD MS = 12.5 vs. normative MS = 20.2, χ2[1] = 0.205, p = 0.650; 50–59 years, ADHD MS = 25% vs. normative MS = 23.9%, χ2[1] = 0.006, p = 0.940). A comparison for elderly patients (above 60 years of age) could not be performed because of their paucity in the study sample (two patients, nobody with MS vs. 8671 patients, 2561 with MS).
No significant differences were found between MS and no MS groups regarding categorical variables including psychopharmacological treatments (Table 1, Figure 1).
Figure 1. Clinical categorical variables (N = 158). ADHD-I, Predominantly inattentive presentation; ADHD-H/I, Predominantly hyperactive/impulsive presentation; ADHD-C, Combined presentation. *Statistically significant after Sidak's adjustment, p ≤ 0.004.
As regards continuous variables (Table 2), diastolic blood pressure, waist circumference, triglycerides, LAP, TG-WC as well as weight and BMI were confirmed to be higher in the MS group than in the no MS group. Notably, no significant age difference was found between study groups.
Multicollinearity was confirmed for both IR surrogate indexes (TG-WC, √VIF = 28.54; LAP, √VIF = 5.40), triglyceride (√VIF = 4.86), and waist circumference (√VIF = 23.33). Triglycerides and waist circumference were confirmed to be strongly correlated with IR surrogate indexes but not with each other. A significant correlation was detected also between IR surrogate indexes (Table 3).
The COMP model, including age and MS components, significantly explained the variation of MS (Nagelkerke's pseudo-R2 = 0.518, χ2[7] = 46.6, p < 0.001, AIC = 77.15). The main predictor was triglycerides blood level (OR = 1.02, 95%CI = 1.01/1.04, z = 3.27, p = 0.001), followed by diastolic blood pressure (OR = 1.08, 95%CI = 1.01/1.17, z = 2.24, p = 0.024), waist circumference (OR = 1.06, 95%CI = 1.01/1.13, z = 2.18, p = 0.029) and, with a weak protective effect, the HDL-C (OR = 0.93, 95%CI = 0.87/0.99, z = −2.05, p = 0.040).
Both the LAP and TG-WC model significantly explained the variation of MS (LAP model, Nagelkerke's pseudo-R2 = 0.546, χ2[6] = 49.7, p < 0.001, AIC = 72.18; TG-WC model, Nagelkerke's pseudo-R2 = 45.6, χ2[6] = 40.4, p < 0.001, AIC = 81.45). Nevertheless, the COMP model better fitted than the TG-WC model (deviance = −6.31, df = 1, p = 0.012) whereas it did not outperform the LAP model (deviance = 2.97, df = 1, p = 0.915). As triglycerides and waist circumference in the COMP model, both TG-WC and LAP confirmed to be significant predictors of MS in its relevant model (LAP, OR = 1.0006, 95%CI = 1.0003/1.0009, z = 4.14, p < 0.001; TG-WC, OR = 1.03, 95%CI = 1.01/1.04, z = 3.60, p < 0.001).
The overall prevalence of MS in our sample of adult ADHD outpatients fell into the range of the Italian general population (from 3% to 30%) (7, 8). This consistency with the general population still persisted when MS prevalence was analyzed in function of age. As already mentioned above, MS as well as any other cardiovascular risk factor tends to increase with age (55). According to the comparison between our ADHD sample and the most recent available normative data (9), no differences were found in age ranges between 18 to 60 but, above this age, the rate of MS in the general population continues to rise while in our sample it drops. This was easily explained by the young age of the study sample due to the paucity of patients above 60. As most of the patients are referred to the outpatient services from a psychiatrist or on their own, it can be assumed that older ADHD patients are less likely to be either recognized by doctors or sensitive to their own symptoms than younger adult patients.
Only over recent years, some authors have remarked the importance of underdiagnosis and untreated ADHD consequences in old adults and elderly people (56–59). A recent epidemiological study conducted on a Swedish national register (56) has shown that ADHD persists in elderly taking along psychiatric and metabolic comorbidity (over nine and two-fold prevalence than subjects without ADHD, respectively). However, according to another European study, no significant difference in lifestyle emerged when elderly ADHD patients were compared to same-age non-ADHD subjects (59). Therefore, more attention should be paid to this age group, which, by itself, seems to be at higher risk for cardiovascular complications. Since ADHD usually has a high prevalence of family history (60), its diagnosis may pose an indication to screen patients' parents for the same disorder to increase dysfunctional ADHD symptoms detection in elderly people.
The comparison with the prevalence of MS in other psychiatric disorders is hindered by the heterogeneity of different populations. Again, age plays a crucial role in determining different MS rates since older populations showed higher prevalence than our sample. In a recent systematic review and meta-analysis, the prevalence of MS was 33.4%, 31.7%, and 31.3% among patients diagnosed with schizophrenia, bipolar disorder and major depressive disorder, respectively (10), but the mean age of the overall population was 41.4 years (ranging from 22 to 73 years). An earlier review also found a bidirectional association between depression and MS but again the final sample age ranged from 20 to 91 years (14). In 2017, Moreira et colleagues carried out a controlled cross-sectional study among young patients (mean age = 25.81 ± 2.17) either with bipolar or major depressive disorder, experiencing a current depressive episode. The prevalence of MS (bipolar disorder = 46.9% and major depressive disorder = 35.1% vs. controls = 22.1%) was not significantly higher than that of healthy controls (12). Despite the similar mean age, the prevalence rate was higher than that detected in our ADHD sample, four-folds for bipolar, three-folds for depressive and even double for controls. Although this study focused on a young population, it considered only patients in the acute phase, thus limiting comparability with other studies. Moreover, differences in settings (acute stage of illness vs. outpatient assessment), regional factors, and pharmacological treatments can be held to explain this gap, especially with healthy controls.
The Italian clinical population was also investigated for the presence of MS (61, 62). Particularly, among bipolar patients, the role of age was confirmed. The prevalence of MS indeed increased from 9.1% under 30 years to 41.8% over 60 years, however, the sample mean age was 50.9 (SD = 15.5) years, way higher than that of the present study (62). Nevertheless, focusing on the prevalence of young patients only, our findings seem to be consistent with that reported by Salvi and colleagues (13) in their sample of Italian patients with bipolar disorder.
Fewer studies have focused on the relationship between OCD and metabolic complications (62, 63), but, in these patients, the main factor involved in increasing the risk of MS appeared to be the lifetime exposure to antipsychotic medications rather than other clinical or socio-demographic features (62).
Although a large proportion of our sample presented with at least one psychiatric comorbidity (82.3%), these conditions did not differ between the MS and no MS groups. This could once again be explained by the young age of the study sample which also implies a short duration of illness for comorbid conditions and thus a shorter exposition to treatments. As a matter of fact, the MS and the no MS group did not have significant differences in terms of ongoing medications. Furthermore, given the non-random allocation to treatments and the different metabolic risk profiles of each antipsychotic, it is expected that patients were receiving the best drug for their overall health status. This may lead to a confounding by indication bias.
Aside from strict metabolic criteria, BMI and weight significantly differed between groups, showing higher values in patients diagnosed with MS. These results are consistent with previous literature not investigating the presence of MS as a whole, but focusing on single metabolic risk factors (45, 47) and even on obesity (41–44). Nonetheless, the logistic regression analysis suggested that triglyceridemia was the main predictor of MS in our sample, as a rise of 1 mg/dl in blood triglycerides levels increases the MS risk of 0.2 points, which means that an increase of 50 mg/dl makes the patient positive for MS. Since blood triglycerides levels depend on dietary habits (e.g., calories intake and alcohol assumption) and weight (64), it is possible that the main driver for MS, in young adult ADHD patients, could be an unhealthy lifestyle rather than a reciprocal predisposition common to both the metabolic and the psychiatric condition (14). Because there is no evidence for more eating and drinking bad habits among elderly patients with ADHD (59), it can be assumed that triglycerides could be lower and this possibly could influence the prevalence rate of MS in this age group. Hence, further studies should be conducted including also older patients to estimate the actual rate of MS and of its main predictors.
As regards IR surrogate indexes, LAP seemed to perform better than TC-WC in predicting the risk for MS in our adult outpatient ADHD sample.
Although the relationship between ADHD and type 2 diabetes was investigated by Landau and Pinhas-Hamiel (46) this is the first study expressively reporting on OR indexes in adult ADHD patients.
To reduce atherosclerotic cardiovascular disease risk in MS, lifestyle interventions are the first-choice, regardless of age (65). Non-pharmacological approaches are recommended to all patients, including quit smoking, follow healthy diet and practice physical activity (66, 67). Pharmacological strategies such as statins and antihypertensive medications should be reserved to patients with specific risk profile based on previous history of cardiovascular disease, and Framingham-based 10-year risk score (65, 68). Fibrate could be an option if triglycerides ≥ 500 mg/dL or if non-HDL cholesterol remains elevated after maximum tolerated high intensity statin and ezetimibe (68). Recent data seem to support that also red yeast rice preparations can reduce mortality and major adverse cardiovascular events, with improvement in lipidic and glycemic serum levels, and blood pressure (69). Metformin has shown efficacy in decreasing weight/BMI and reducing progression toward diabetes mellitus (70), but data on cardiovascular risk reduction are not this straightforward. Although improvements have been reported with bariatric surgery (71), an accurate risk-benefit analysis should be performed before exposing the patient to surgical risks.
Target mechanisms for new medications include increasing insulin sensitivity, reducing appetite, decreasing pro-inflammatory cytokines release by adipose tissue, and counteracting both endothelial dysfunction and thrombogenic effects of MS (68).
Among the limitations of the present study, the lack of patients over 60 years of age is certainly the most important because it has not allowed us to properly evaluate the relationship between ADHD and MS, given the central role played by age, reducing also the possibility of confronting and generalizing our results. Secondly, in the present study, data concerning lifestyle habits (i.e., diet, physical activity, smoking, alcohol) and details on the exposition to medications (i.e. second-generation antipsychotic), which might have contributed to the development of MS, were not collected. Another limitation was the absence of a control group, which was addressed through a comparison of the age-stratified prevalence rate of MS with those of a European population. However, through this artifice we could reach reliable findings, at least for under 60 age groups. Finally, although the cross-sectional design limited the inferences on temporal relationships among variables, we could anyway perform a logistic regression to assess the contribution of each criterion in defining MS in our sample. Further, possibly perspective, studies are needed to better clarify the extent of cardiovascular risk in terms of metabolic factors among adult patients with ADHD.
In conclusion, we recommend clinicians to thoroughly screen ADHD patients for each MS component and to repeat the evaluation during the follow-up, paying particular attention to blood triglyceride concentration, inasmuch as they seem to predict the risk of a full MS.
The original contributions presented in the study are included in the article/supplementary files, further inquiries can be directed to the corresponding author.
The studies involving human participants were reviewed and approved by Research Ethics Committee of the San Luigi Gonzaga University Hospital (Orbassano, Turin, Italy; Prot no. 16/2019). The patients/participants provided their written informed consent to participate in this study.
FO and GG conceived the study and drafted the manuscript together with IB who also collected the data. FO performed the statistical analysis. AP participated in the initial design of the study. SP contributed to revising the manuscript. All authors read and approved the final manuscript.
The authors declare that the research was conducted in the absence of any commercial or financial relationships that could be construed as a potential conflict of interest.
All claims expressed in this article are solely those of the authors and do not necessarily represent those of their affiliated organizations, or those of the publisher, the editors and the reviewers. Any product that may be evaluated in this article, or claim that may be made by its manufacturer, is not guaranteed or endorsed by the publisher.
Researchers would like to thank all patients involved in the study for their valuable contribution.
1. Zimmet P, Alberti KGMM, Stern N, Bilu C, El-Osta A, Einat H, Kronfeld-Schor N. The circadian syndrome: is the metabolic syndrome and much more! J Intern Med. (2019) 286:181–91. doi: 10.1111/joim.12924
2. Birnbaum HG, Mattson ME, Kashima S, Williamson TE. Prevalence rates and costs of metabolic syndrome and associated risk factors using employees' integrated laboratory data and health care claims. J Occup Environ Med. (2011) 53:27–33. doi: 10.1097/JOM.0b013e3181ff0594
3. Boudreau DM, Malone DC, Raebel MA, Fishman PA, Nichols GA, Feldstein AC, et al. Health care utilization and costs by metabolic syndrome risk factors. Metab Syndr Relat Disord. (2009) 7:305–14. doi: 10.1089/met.2008.0070
4. Scholze J, Alegria E, Ferri C, Langham S, Stevens W, Jeffries D, et al. Epidemiological and economic burden of metabolic syndrome and its consequences in patients with hypertension in Germany, Spain and Italy; a prevalence-based model. BMC Public Health. (2010) 10:529. doi: 10.1186/1471-2458-10-529
5. Beltrán-Sánchez H, Harhay MO, Harhay MM, McElligott S. Prevalence and trends of metabolic syndrome in the adult U.S. population, 1999-2010. J Am Coll Cardiol. (2013) 62:697–703. doi: 10.1016/j.jacc.2013.05.064
6. Moore JX, Chaudhary N, Akinyemiju T. Metabolic syndrome prevalence by race/ethnicity and sex in the United States, national health and nutrition examination survey, 1988-2012. Prev Chronic Dis. (2017) 14:E24. doi: 10.5888/pcd14.160287
7. Tocci G, Ferrucci A, Bruno G, Mannarino E, Nati G, Trimarco B, et al. Prevalence of metabolic syndrome in the clinical practice of general medicine in Italy. Cardiovasc Diagn Ther. (2015) 5:271–9.
8. Miccoli R, Bianchi C, Odoguardi L, Penno G, Caricato F, Giovannitti MG, et al. Prevalence of the metabolic syndrome among Italian adults according to ATP III definition. Nutr Metab Cardiovasc Dis NMCD. (2005) 15:250–4. doi: 10.1016/j.numecd.2004.09.002
9. Vishram JKK, Borglykke A, Andreasen AH, Jeppesen J, Ibsen H, Jørgensen T, et al. Impact of age and gender on the prevalence and prognostic importance of the metabolic syndrome and its components in Europeans. The MORGAM Prospective Cohort Project PloS One. (2014) 9:e107294. doi: 10.1371/journal.pone.0107294
10. Vancampfort D, Stubbs B, Mitchell AJ, De Hert M, Wampers M, Ward PB, et al. Risk of metabolic syndrome and its components in people with schizophrenia and related psychotic disorders, bipolar disorder and major depressive disorder: a systematic review and meta-analysis. World Psychiatry Off J World Psychiatr Assoc WPA. (2015) 14:339–47. doi: 10.1002/wps.20252
11. Mitchell AJ, Vancampfort D, Sweers K, van Winkel R, Yu W, De Hert M. Prevalence of metabolic syndrome and metabolic abnormalities in schizophrenia and related disorders–a systematic review and meta-analysis. Schizophr Bull. (2013) 39:306–18. doi: 10.1093/schbul/sbr148
12. Moreira FP, Jansen K, Cardoso T de A, Mondin TC, Magalhães PV da S, Kapczinski F, et al. Metabolic syndrome in subjects with bipolar disorder and major depressive disorder in a current depressive episode: population-based study: Metabolic syndrome in current depressive episode. J Psychiatr Res. (2017) 92:119–23. doi: 10.1016/j.jpsychires.2017.03.025
13. Salvi V, Albert U, Chiarle A, Soreca I, Bogetto F, Maina G. Metabolic syndrome in Italian patients with bipolar disorder. Gen Hosp Psychiatry. (2008) 30:318–23. doi: 10.1016/j.genhosppsych.2008.04.009
14. Pan A, Keum N, Okereke OI, Sun Q, Kivimaki M, Rubin RR, et al. Bidirectional association between depression and metabolic syndrome: a systematic review and meta-analysis of epidemiological studies. Diabetes Care. (2012) 35:1171–80. doi: 10.2337/dc11-2055
15. Stubbs B, Ku P-W, Chung M-S, Chen L-J. Relationship between objectively measured sedentary behavior and cognitive performance in patients with schizophrenia vs controls. Schizophr Bull. (2017) 43:566–74. doi: 10.1093/schbul/sbw126
16. Vancampfort D, Knapen J, Probst M, Scheewe T, Remans S, De Hert M, et al. systematic review of correlates of physical activity in patients with schizophrenia. Acta Psychiatr Scand. (2012) 125:352–62. doi: 10.1111/j.1600-0447.2011.01814.x
17. Lin Y-C, Lai C-L, Chan H-Y. The association between rehabilitation programs and metabolic syndrome in chronic inpatients with schizophrenia. Psychiatry Res. (2018) 260:227–32. doi: 10.1016/j.psychres.2017.11.081
18. Nayerifard R, Bureng MA, Zahiroddin A, Namjoo M, Rajezi S. Comparison of metabolic syndrome prevalence in patients with schizophrenia and bipolar I disorder. Diabetes Metab Syndr. (2017) 11 (Suppl. 1):S411–6. doi: 10.1016/j.dsx.2017.03.027
19. Arlington: American Psychiatric. American Psychiatric Association. Diagnostic and statistical manual of mental disorders, 5th Edition: DSM-5. (2013). Available online at: https://www.psychiatry.org/psychiatrists/practice/dsm (accessed December 6, 2021).
20. Polanczyk GV, Salum GA, Sugaya LS, Caye A, Rohde LA. Annual research review: a meta-analysis of the worldwide prevalence of mental disorders in children and adolescents. J Child Psychol Psychiatry. (2015) 56:345–65. doi: 10.1111/jcpp.12381
21. Fayyad J, Sampson NA, Hwang I, Adamowski T, Aguilar-Gaxiola S, Al-Hamzawi A, et al. The descriptive epidemiology of DSM-IV Adult ADHD in the world health organization world mental health surveys. Atten Deficit Hyperact Disord. (2017) 9:47–65. doi: 10.1007/s12402-016-0208-3
22. Faraone SV, Biederman J, Mick E. The age-dependent decline of attention deficit hyperactivity disorder: a meta-analysis of follow-up studies. Psychol Med. (2006) 36:159–65. doi: 10.1017/S003329170500471X
23. Asherson P, Buitelaar J, Faraone SV, Rohde LA. Adult attention-deficit hyperactivity disorder: key conceptual issues. Lancet Psychiatry. (2016) 3:568–78. doi: 10.1016/S2215-0366(16)30032-3
24. Kooij JJS, Bijlenga D, Salerno L, Jaeschke R, Bitter I, Balázs J, et al. Updated European Consensus Statement on diagnosis and treatment of adult ADHD. Eur Psychiatry J Assoc Eur Psychiatr. (2019) 56:14–34. doi: 10.1016/j.eurpsy.2018.11.001
25. Salem H, Vivas D, Cao F, Kazimi IF, Teixeira AL, Zeni CP, et al. ADHD is associated with migraine: a systematic review and meta-analysis. Eur Child Adolesc Psychiatry. (2018) 27:267–77. doi: 10.1007/s00787-017-1045-4
26. Schans J. van der, Çiçek R, de Vries TW, Hak E, Hoekstra PJ. Association of atopic diseases and attention-deficit/hyperactivity disorder: a systematic review and meta-analyses. Neurosci Biobehav Rev. (2017) 74:139–48. doi: 10.1016/j.neubiorev.2017.01.011
27. Clappison E, Hadjivassiliou M, Zis P. Psychiatric manifestations of coeliac disease, a systematic review and meta-analysis. Nutrients. (2020) 12:E142. doi: 10.3390/nu12010142
28. Wynchank D, Bijlenga D, Lamers F, Kooij JJS, Bron TI, Beekman ATF, et al. The association between metabolic syndrome, obesity-related outcomes, and ADHD in Adults with comorbid affective disorders. J Atten Disord. (2018) 22:460–71. doi: 10.1177/1087054716659137
29. Borch-Johnsen K, Wareham N. The rise and fall of the metabolic syndrome. Diabetologia. (2010) 53:597–9. doi: 10.1007/s00125-010-1659-2
30. Magliano DJ, Cameron A, Shaw JE, Zimmet P. “Epidemiology of Metabolic Syndrome.,” The Epidemiology of Diabetes Mellitus. Hoboken, NJ: John Wiley & Sons, Ltd (2008). p. 31–55 doi: 10.1002/9780470779750.ch4
31. Cornier M-A, Dabelea D, Hernandez TL, Lindstrom RC, Steig AJ, Stob NR, et al. The metabolic syndrome. Endocr Rev. (2008) 29:777–822. doi: 10.1210/er.2008-0024
32. Reaven GM. The metabolic syndrome: time to get off the merry-go-round? J Intern Med. (2011) 269:127–36. doi: 10.1111/j.1365-2796.2010.02325.x
33. Gallagher EJ, LeRoith D, Karnieli E. The metabolic syndrome–from insulin resistance to obesity and diabetes. Endocrinol Metab Clin North Am. (2008) 37:559–579. doi: 10.1016/j.ecl.2008.05.002
34. Roberts CK, Hevener AL, Barnard RJ. Metabolic syndrome and insulin resistance: underlying causes and modification by exercise training. Compr Physiol. (2013) 3:1–58. doi: 10.1002/cphy.c110062
35. Saely CH, Aczel S, Marte T, Langer P, Hoefle G, Drexel H. The metabolic syndrome, insulin resistance, and cardiovascular risk in diabetic and nondiabetic patients. J Clin Endocrinol Metab. (2005) 90:5698–703. doi: 10.1210/jc.2005-0799
36. Lteif AA, Han K, Mather KJ. Obesity, insulin resistance, and the metabolic syndrome: determinants of endothelial dysfunction in whites and blacks. Circulation. (2005) 112:32–8. doi: 10.1161/CIRCULATIONAHA.104.520130
37. Kahn HS. The “lipid accumulation product” performs better than the body mass index for recognizing cardiovascular risk: a population-based comparison. BMC Cardiovasc Disord. (2005) 5:26. doi: 10.1186/1471-2261-5-26
38. Okosun IS, Okosun B, Lyn R, Airhihenbuwa C. Surrogate indexes of insulin resistance and risk of metabolic syndrome in non-Hispanic White, non-Hispanic Black and Mexican American. Diabetes Metab Syndr. (2020) 14:3–9. doi: 10.1016/j.dsx.2019.11.012
39. Zheng S, Shi S, Ren X, Han T, Li Y, Chen Y, et al. Triglyceride glucose-waist circumference, a novel and effective predictor of diabetes in first-degree relatives of type 2 diabetes patients: cross-sectional and prospective cohort study. J Transl Med. (2016) 14:260. doi: 10.1186/s12967-016-1020-8
40. Lee J, Kim B, Kim W, Ahn C, Choi HY, Kim JG, et al. Moon S. Lipid indices as simple and clinically useful surrogate markers for insulin resistance in the US population. Sci Rep. (2021) 11:2366. doi: 10.1038/s41598-021-82053-2
41. Cortese S. The Association between ADHD and obesity: intriguing, progressively more investigated, but still puzzling. Brain Sci. (2019) 9:E256. doi: 10.3390/brainsci9100256
42. Pagoto SL, Curtin C, Lemon SC, Bandini LG, Schneider KL, Bodenlos JS, et al. Association between adult attention deficit/hyperactivity disorder and obesity in the US population. Obes Silver Spring Md. (2009) 17:539–44. doi: 10.1038/oby.2008.587
43. Waring ME, Lapane KL. Overweight in children and adolescents in relation to attention-deficit/hyperactivity disorder: results from a national sample. Pediatrics. (2008) 122:e1–6. doi: 10.1542/peds.2007-1955
44. Fuemmeler BF, Østbye T, Yang C, McClernon FJ, Kollins SH. Association between attention-deficit/hyperactivity disorder symptoms and obesity and hypertension in early adulthood: a population-based study. Int J Obes. 2005. (2011) 35:852–862. doi: 10.1038/ijo.2010.214
45. Chen Q, Hartman CA, Haavik J, Harro J, Klungsøyr K, Hegvik T-A, et al. Common psychiatric and metabolic comorbidity of adult attention-deficit/hyperactivity disorder: a population-based cross-sectional study. PLoS ONE. (2018) 13:e0204516. doi: 10.1371/journal.pone.0204516
46. Landau Z, Pinhas-Hamiel O. Attention Deficit/Hyperactivity, the metabolic syndrome, and type 2 diabetes. Curr Diab Rep. (2019) 19:46. doi: 10.1007/s11892-019-1174-x
47. Spencer TJ, Faraone SV, Tarko L, McDermott K, Biederman J. Attention-deficit/hyperactivity disorder and adverse health outcomes in adults. J Nerv Ment Dis. (2014) 202:725–31. doi: 10.1097/NMD.0000000000000191
48. Docet MF, Larrañaga A, Pérez Méndez LF, García-Mayor RV. Attention deficit hyperactivity disorder increases the risk of having abnormal eating behaviours in obese adults. Eat Weight Disord EWD. (2012) 17:e132–136. doi: 10.1007/BF03325337
49. Lucassen EA, Zhao X, Rother KI, Mattingly MS, Courville AB, de Jonge L, et al. Sleep extension study group, Evening chronotype is associated with changes in eating behavior, more sleep apnea, and increased stress hormones in short sleeping obese individuals PloS ONE. (2013) 8:e56519. doi: 10.1371/journal.pone.0056519
50. Ptacek R, Kuzelova H, Stefano GB, Raboch J, Sadkova T, Goetz M, et al. Disruptive patterns of eating behaviors and associated lifestyles in males with ADHD. Med Sci Monit Int Med J Exp Clin Res. (2014) 20:608–13. doi: 10.12659/MSM.890495
51. Katzman MA, Bilkey TS, Chokka PR, Fallu A, Klassen LJ. Adult ADHD and comorbid disorders: clinical implications of a dimensional approach. BMC Psychiatry. (2017) 17:302. doi: 10.1186/s12888-017-1463-3
52. Alswat KA, Alnemari AK, Alghamdi I, Almalki AA, Al-Thomali B, Mahfouz T. Prevalence of Metabolic Syndrome in the Hospitalized Psychiatric Patients. Med Arch Sarajevo Bosnia Herzeg. (2017) 71:412–6. doi: 10.5455/medarh.2017.71.412-416
53. Czepielewski L, Daruy Filho L, Brietzke E, Grassi-Oliveira R. Bipolar disorder and metabolic syndrome: a systematic review. Rev Bras Psiquiatr São Paulo Braz 1999. (2013) 35:88–93. doi: 10.1016/j.rbp.2012.07.004
54. Kabacoff R. R in Action: Data Analysis and Graphics with R. Shelter Island, NY: Manning (2011). p. 447.
55. Eckel RH, Grundy SM, Zimmet PZ. The metabolic syndrome. Lancet Lond Engl. (2005) 365:1415–28. doi: 10.1016/S0140-6736(05)66378-7
56. Guldberg-Kjär T, Johansson B. ADHD symptoms across the lifespan: a comparison of symptoms captured by the Wender and Barkley Scales and DSM-IV criteria in a population-based Swedish sample aged 65 to 80. J Atten Disord. (2015) 19:390–404. doi: 10.1177/1087054713514853
57. Michielsen M, de Kruif JTCM, Comijs HC, van Mierlo S, Semeijn EJ, Beekman ATF, et al. The burden of ADHD in older adults: a qualitative study. J Atten Disord. (2018) 22:591–600. doi: 10.1177/1087054715610001
58. Oliva F, Malandrone F, Mirabella S, Ferreri P, di Girolamo G, Maina G. Diagnostic delay in ADHD: duration of untreated illness and its socio-demographic and clinical predictors in a sample of adult outpatients. Early Interv Psychiatry. (2021) 15:957–65. doi: 10.1111/eip.13041
59. Semeijn EJ, Kooij JJS, Comijs HC, Michielsen M, Deeg DJH, Beekman ATF. Attention-deficit/hyperactivity disorder, physical health, and lifestyle in older adults. J Am Geriatr Soc. (2013) 61:882–7. doi: 10.1111/jgs.12261
60. Faraone SV, Doyle AE. The nature and heritability of attention-deficit/hyperactivity disorder. Child Adolesc Psychiatr Clin N Am. (2001) 10:299–316. doi: 10.1016/S1056-4993(18)30059-2
61. Albert U, Aguglia A, Chiarle A, Bogetto F, Maina G. Metabolic syndrome and obsessive-compulsive disorder: a naturalistic Italian study. Gen Hosp Psychiatry. (2013) 35:154–9. doi: 10.1016/j.genhosppsych.2012.10.004
62. Salvi V, D'Ambrosio V, Rosso G, Bogetto F, Maina G. Age-Specific prevalence of metabolic syndrome in Italian patients with bipolar disorder. Psychiatry Clin Neurosci. (2011) 65:47–54. doi: 10.1111/j.1440-1819.2010.02160.x
63. Isomura K, Brander G, Chang Z, Kuja-Halkola R, Rück C, Hellner C, et al. la Cruz L. Metabolic and cardiovascular complications in obsessive-compulsive disorder: a total population, sibling comparison study with long-term follow-up. Biol Psychiatry. (2018) 84:324–31. doi: 10.1016/j.biopsych.2017.12.003
64. Rygiel K. Hypertriglyceridemia - common causes, prevention and treatment strategies. Curr Cardiol Rev. (2018) 14:67–76. doi: 10.2174/1573403X14666180123165542
65. Grundy SM, Stone NJ, Bailey AL, Beam C, Birtcher KK, Blumenthal RS, et al. 2018 AHA/ACC/AACVPR/AAPA/ABC/ACPM/ADA/AGS/APhA/ASPC/NLA/PCNA Guideline on the Management of Blood Cholesterol: a report of the American College of Cardiology/American Heart Association Task Force on Clinical Practice Guidelines. Circulation. (2019) 139:e1082–143. doi: 10.1161/CIR.0000000000000624
66. Peiris CL, van Namen M, O'Donoghue G. Education-based, lifestyle intervention programs with unsupervised exercise improve outcomes in adults with metabolic syndrome a systematic review and meta-analysis Rev Endocr Metab Disord. (2021) 22:877–90. doi: 10.1007/s11154-021-09644-2
67. Rahimi GRM, Yousefabadi HA, Niyazi A, Rahimi NM, Alikhajeh Y. Effects of lifestyle intervention on inflammatory markers and waist circumference in overweight/obese adults with metabolic syndrome: a systematic review and meta-analysis of randomized controlled trials. Biol Res Nurs. (2022) 24:94–105. doi: 10.1177/10998004211044754
68. Rask Larsen J, Dima L, Correll CU, Manu P. The pharmacological management of metabolic syndrome. Expert Rev Clin Pharmacol. (2018) 11:397–410. doi: 10.1080/17512433.2018.1429910
69. Yuan R, Yuan Y, Wang L, Xin Q, Wang Y, Shi W, et al. Red yeast rice preparations reduce mortality, major cardiovascular adverse events, and risk factors for metabolic syndrome: a systematic review and meta–analysis. Front Pharmacol. (2022) 13:744928. doi: 10.3389/fphar.2022.744928
70. Lentferink YE, Knibbe C A J, van der Vorst MMJ. Efficacy of metformin treatment with respect to weight reduction in children and adults with obesity: a systematic review. Drugs. (2018) 78:1887–901. doi: 10.1007/s40265-018-1025-0
Keywords: metabolic syndrome, ADHD, insulin resistance, adult, obesity, BMI, age, hypertriglyceridemia
Citation: di Girolamo G, Bracco IF, Portigliatti Pomeri A, Puglisi S and Oliva F (2022) Prevalence of Metabolic Syndrome and Insulin Resistance in a Sample of Adult ADHD Outpatients. Front. Psychiatry 13:891479. doi: 10.3389/fpsyt.2022.891479
Received: 07 March 2022; Accepted: 27 May 2022;
Published: 21 June 2022.
Edited by:
Giacomo Deste, Civil Hospital of Brescia, ItalyReviewed by:
Francesco Monaco, Azienda Sanitaria Locale Salerno, ItalyCopyright © 2022 di Girolamo, Bracco, Portigliatti Pomeri, Puglisi and Oliva. This is an open-access article distributed under the terms of the Creative Commons Attribution License (CC BY). The use, distribution or reproduction in other forums is permitted, provided the original author(s) and the copyright owner(s) are credited and that the original publication in this journal is cited, in accordance with accepted academic practice. No use, distribution or reproduction is permitted which does not comply with these terms.
*Correspondence: Francesco Oliva, ZnJhbmNlc2NvLm9saXZhQHVuaXRvLml0
Disclaimer: All claims expressed in this article are solely those of the authors and do not necessarily represent those of their affiliated organizations, or those of the publisher, the editors and the reviewers. Any product that may be evaluated in this article or claim that may be made by its manufacturer is not guaranteed or endorsed by the publisher.
Research integrity at Frontiers
Learn more about the work of our research integrity team to safeguard the quality of each article we publish.