- 1Department of Psychiatry, The Affiliated Brain Hospital of Nanjing Medical University, Nanjing, China
- 2Nanjing Brain Hospital, Medical School of Nanjing University, Nanjing, China
- 3School of Biological Sciences and Medical Engineering, Southeast University, Nanjing, China
- 4Key Laboratory of Child Development and Learning Science of Ministry of Education, Nanjing, China
Background: Previous research studies have demonstrated that impaired interoception is involved in emotional information processing in major depressive disorder (MDD). Heartbeat-evoked potential (HEP) amplitudes, an index for interoception, could be manipulated by emotional faces in healthy people. Considering negative emotional bias is the core characteristic in MDD, we hypothesized that interoception dysfunction was associated with the negative emotional bias in MDD.
Methods: An electroencephalogram (EEG) study under an emotional faces task was applied to explore the relationship between interoception and emotional bias. HEPs before emotional faces stimuli were used to predict the late positive potential (LPP) amplitudes and it worked as an index of emotional bias. Twenty-seven patients with MDD and 27 healthy controls (HCs) participated in this study. Source analysis gave an auxiliary description for results in sensory level.
Results: Major depressive disorders (MDDs) had poor performance in the heartbeat count task (HCT) and attenuate HEP average amplitudes (455–550 ms). Compared with HCs, cluster-based permutation t-tests revealed that MDDs had attenuated LPP amplitudes (300–1,000 ms) over centroparietal regions and enhanced LPP amplitudes over frontocentral regions. Furthermore, abnormal attenuated HEPs could predict aberrant LPPs under sad face stimuli in MDDs, which could be associated with the dysfunction of the anterior cingulate cortex (ACC) and right insula.
Conclusion: Mediated by ACC and insula, interoception dysfunction contributes to the negative emotional bias of MDD, highlighting the importance of interoception in the disorder.
Introduction
Major depressive disorder (MDD) is a common affective psychiatric disorder and has been the leading cause of burden worldwide (1). Emotion dysregulation is the core characteristic of MDD and efforts to elaborate potential mechanisms of emotion are particularly pressing for the development of effective prevention and therapy in MDD (2). Previous works have reported that depressive patients are characterized by the negative emotional bias (3), concretely showing in abnormal negative faces processing (4). It was reported that depressive patients showed abnormal event-related potential (ERP) amplitude resulting from aberrant neuron discharge evoked by emotional faces stimuli (5). Compared with healthy controls (HCs), negative emotion stimuli evoked more aberrant ERPs than positive emotion stimuli and neutral emotion stimuli tended to evoke negative similar ERPs (6, 7) in depressive patients, which was mediated by the abnormal activity patterns within common networks of brain regions that include the amygdala, basal ganglia, insula, anterior cingulate cortex (ACC), and several regions of the prefrontal cortex (8–10). Late positive potential (LPP), which occurs between 300 and 1,000 ms after stimuli and maximum over centroparietal regions, is a late emotion-related ERP component specially related to negative emotional bias (11, 12). LPPs were thought to reflect emotion arousal and motivated attention for their synchronism with the arousal of the autonomic nervous system (13, 14). The attenuated mean amplitude of LPPs in MDD was associated with low dopamine levels (15).
The theory of embodied cognition indicates that not only cognitive processes are influenced by the body but also cognition exists in the service of action (16). Interoception is the core of the theory of embodied cognition, and embodied cognition supports the extension to other brain regions of the principles of organization of cerebral cortical connections (17, 18). Further investigating the relationship between interoception and depression would be helpful in understanding the neural mechanism of MDD. In psychology, predictive coding is applied to study the brain function, especially in electrophysiological investigations (19). Unlike the traditional experimental model, which recognized the brain as a “stimulus-response” organ, in the predictive coding model, the brain actively applies learned predictions to infer the causes of incoming sensory information, namely, brain could predict the following state by previous experiences in a probability-driven way (20). By integrating the neuroanatomical model, Barrett provided the Embodied Predictive Interoception Coding (EPIC) model to explain the potential pathological mechanism for MDD (21). The model speculates that the imbalance of allostasis detected by interoception dysfunction leads to diverse clinical features (such as emotion dysregulation) that result from the disabled internal-and-external information processing. ACC, insula, primary interoceptive cortex, and somatosensory regions could be the neural basis mediated by dopamine and acetylcholine (21). The neural network in this model had been proved with a large healthy sample of MRI study and macaque monkeys study, which put a new perspective to understand the emotion dysfunction in MDD (22, 23).
Up to now, many studies had revealed the correlation between peripheral system dysfunction and MDD (24–26), highlighting the important role of allostasis in MDD. It is conceived that the interoception dysfunction leads to an imbalance of allostasis in MDDs by the abnormal integration of internal and external information (27), which would affect emotion information processing ulteriorly. ERPs, with the advantages of the high temporal resolution, are beneficial to studying high informative power on neural alterations in MDD (28). Cardiac interoception working as a robust internal signal (29) is a majority way to investigate interoception because of heartbeats’ large effect and easy availability (30). By time-locking to the electrocardiogram (ECG) R-peak of the heartbeat, cardiac interoception is quantified with the heartbeat-evoked potential (HEP) (31, 32). It was reported that depressive individuals had decreased heartbeat perception accuracy and reduced HEP amplitudes (33, 34). Further evidence of an impaired association between HEP amplitudes and heartbeat perception accuracy in depressive patients suggested the imbalance of allostasis in MDD (34). In addition, many researchers had reported the relationship between emotional information processing and interoception in psychiatric disorders, such as anxiety (35), alcohol use disorder (36), and functional neurological symptom disorder (37). However, previous research studies on interoception mainly focused on somatic symptoms in MDD (27, 38). Recently, some studies have shown that HEP amplitude could be manipulated by negative emotional face stimuli in healthy people (39), which provided the clue to explore the relationship between interoception and the negative emotional bias in MDD.
The current study was aimed to study the negative emotional bias in MDD from the aspect of internal-external information integration. We supposed that there is an association between impaired interoception and negative emotional bias in MDD. We further speculate that aberrant HEPs before emotional faces stimuli could predict abnormal LPPs in MDD.
Materials and Methods
Ethics Statement
Procedures were approved by the ethics committee of the Affiliated Nanjing Brain Hospital of Nanjing Medical University in accordance with the Declaration of Helsinki, and all participants provided written informed consent. The data were collected from 01 March 2021 to 30 October 2021.
Participants and Procedures
Participants included 30 MDDs and 30 healthy volunteers who matched for age, sex, and body mass index (BMI). The education of parents was also matched in that this information contained intelligence and economic level at the same time. MDDs fulfilled the Diagnostic and Statistical Manual of Mental Disorders Fourth Edition (DSM-IV) diagnosis of MDD as verified with the Chinese version of the Mini-International Neuropsychiatric Interview (MINI) interview to further study inclusion. Beck Depression Inventory-II (BDI-II) (40) and the State-Trait Anxiety Inventory (STAI) (41) were applied to assess the depressive level and anxiety state. General exclusion criteria comprised (a) neurological disorders; (b) alcohol dependence, nicotine dependence, or other psychoactive substance abuse; (c) comorbidity or diagnosis of other psychiatric disorders; (d) severe medical illness, such as cardiac dysregulations; (e) received medicine therapy in 2 weeks; and (f) received Electroconvulsive Therapy (ECT) or modified ECT within last 6 months. After data processing, a total of 54 participants remained (shown in Figure 1B).
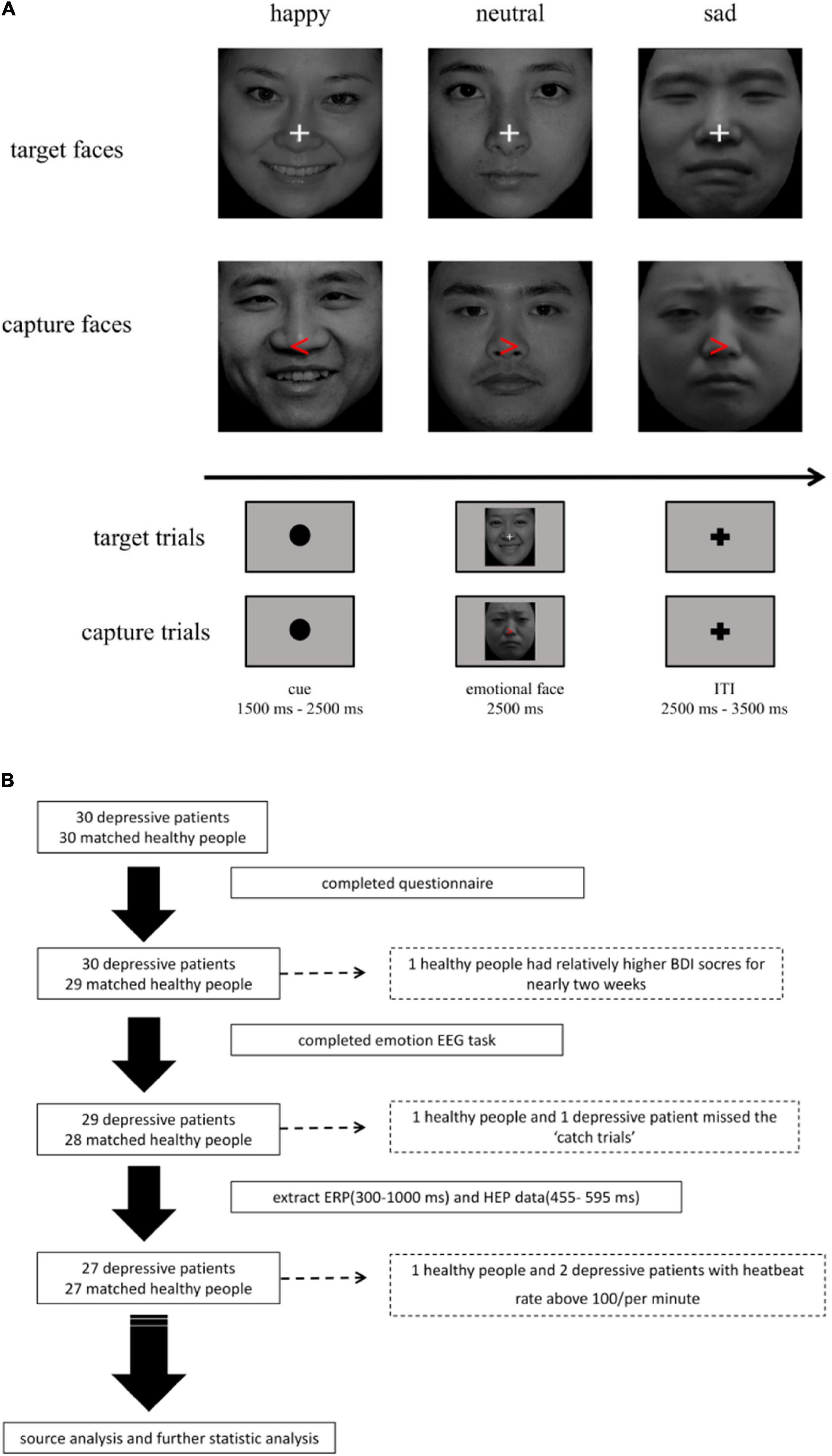
Figure 1. (A) Emotional facial stimuli task. The task was divided into two parts (target trials: 80%; capture trials: 20%). (B) The flowchart for the study. After processing of data, 27 major depressive disorders (MDDs) and 27 healthy controls (HCs) were involved in the task.
After evaluating the depressive level and anxiety state, participants have a 10-min rest and completed a standard HCT (42). They were instructed to report their heartbeats numbers in a calm state during five time periods (25, 35, 45, 55, and 60 s), which were presented in random order. Guessing the number of heartbeats was forbidden. The task was performed twice and participants with large differences in reports were asked to attend the experiment another day. Heartbeat tracking score was calculated using the following formula:
Then, participants finished a revised emotional face visual paradigm.
Produces and Task Design
An emotional face visual paradigm was used to produce emotional stimuli edited by E-prime 3.0 (Psychology Software Tools Inc.). In total, 300 gray-scale photographs of different Chinese individuals without hair posing one of three different facial expressions (100 sad faces, 100 happy faces, and 100 neutral faces, half male and half female faces in each condition) were selected from the revised version of the Chinese Facial Affective Picture System (43). All faces with white fixation crosses at the point of the nose were resized to present centrally on a computer screen at a viewing distance of 80 cm.
In the task, we set a cue stimulus with a black fixation dot ranged from 1,500 to 2,500 ms randomly in order to capture HEP before an emotional stimulus. Then, 2,500 ms emotional faces would be followed. A cue stimulus and an emotional face stimulus constituted a trial, and trials were separated by a 2,500–3,500 ms interval to avoid residual emotion effect that affected the next pre-stimulate HEP. To avoid potential confusion, we set 20% capture trials to assess participants’ attention. The capture trials contained a red arrow replacing white fixation cross randomly in the emotional faces, which need participants to make a response by pressing “A” (right) or “L” (left) as soon as possible. The participants who failed to press the button were excluded from later analyses (as shown in Figure 1A). The task was divided into three blocks. The training session contained 10 trials and the duration of each block was about 15–20 min.
Electroencephalogram Data Acquisition
Electroencephalogram (EEG) signal was acquired from a 64-channel active electrode system with Compumedics Neuvo at a sampling rate of 1,000 Hertz (Hz), referenced to a common average reference. The electrode cap was Quik-Cap HydroCell Array made in Australia. Two additional ECG electrodes were put on the participants’ wrist and ankle on the same side. The continuous EEG signal was filtered with a 30 Hz low filter and a 0.3 Hz high filter. Considering the volume-conduction effects, the electrodes, which neared the orbital cavity, were excluded. Finally, 47 EEG channels were included in the following analysis. Independent component analysis (ICA) as implemented in EEGLAB (EEGLAB 9.0.3, University of San Diego, San Diego, CA, United States) was conducted to remove eye movements, blinks, and the cardiac field artifact (CFA) (44). The removing ICA components in every data were no more than 4. LPPs and HEPs were calculated by averaging across trials in different conditions.
Late Positive Potential and Heartbeat-Evoked Potential Analyses
For the LPP, the segment set was put from − 200 to 1,000 ms relative to the presentation of the segment set the facial stimulus. Every participant completed 100 trials per condition and at least 70 trials (70–93 trials, average 90.47) leaving after artifact correction. The LPP in this study was defined as the mean voltage from 300 to 1,000 ms.
For the HEP, the R-peak was marked by HEPLAB [HEPLAB: a MATLAB graphical interface for the preprocessing of the HEP (Version v1.0.0)]. EEG data were segmented into 1,000–2,500 ms periods relative to the ERP stimulus’ markers to reduce the overlap from vision-evoked potential. Although in a recent study, authors were asked to segment heartbeat period with −100 to 700 ms based on the R-peak marker in the healthy population, the heartbeats of the most MDDs in our study were above average 86/per min during the task, which was in line with the authenticated phenomenon that MDDs had faster heartbeats than healthy human (45–47). It would fail to show disease attributes with segmentation standard by −100 to 700 ms. Therefore, epochs were further segmented into periods ranging from −100 to 600 ms according to the R-peak marker in our study (48–50). In HEP analysis, CFA was considered as the most important confounding factor for its same event time-locked characteristics with HEP (42). The strong electrical signal of CFA would influence results by spatio-temporal overlap with the HEP. ICA has been accepted as one of the most effective methods to excluded CFA; however, it is hard to completely remove the CFA with ICA. In our study, we had chosen the mean voltage from 455 to 595 ms after the ECG R wave as HEP index in that it was reported that CFA in this time window is less than 1% (51–54). Furthermore, the ECG mean amplitude in 455–595 ms was also compared between the two groups to avoid confounding cardiac effects (39).
Heartbeat-evoked potentials before emotion stimuli were used to predict LPPs that were induced by emotional faces.
Source Localization of the Scalp-Domain Electrophysiological Activity
In this study, the different scalp-domain electrophysiological activity was further explored by source localization analysis, which was conducted by free standardized low-resolution brain electromagnetic tomography (sLORETA) software.1 The sLORETA estimates brain neural activity by solving the EEG inverse problem, which has been widely applied in the EEG source localization analysis. The time windows were set according to significant scalp-domain electrophysiological activity, and mean amplitude analysis was used to figure out the different source activation between HCs and MDDs for HEPs (455–595 ms) and LPPs (300–1,000 ms).
Statistical Analyses
Independent t-tests and chi-square test were performed to compare the differences between the MDD group and HC group in demography characteristics (age, BMI, parents’ education, disease course, ECG data, and gender) and questionnaires (BDI-II, STAI) with IBM Statistical Product and Service Solution (SPSS) Statistics for Windows, version 17 (IBM Corp., Armonk, NY, United States). Non-parametric cluster-based permutation tests were performed in both sensor and source levels with a value of p was set for < 0.05. Partial correlation analysis was applied to examine the relationship between the significant electrodes of HEPs and LPPs, with age, BMI, gender, education of parents, disease course, BDI-II, SAI, and TAI as control variables. To eliminate the effect of dimension, data were normalized and a stepwise regression analysis was used to establish a predictive mode with the significant clusters of LPPs as the dependent variables and the significant clusters of HEPs as the independent variables. The value of p was set for < 0.05. The best model was selected to explain the relationship between LPP and HEP.
Results
Demographic and Clinical Characteristics
As shown in Table 1, there are no differences in age, BMI, gender, and the education of parents between the MDD group and HC group. However, the differences were found in heartbeat interoception, the scores of BDI-II, SAI, and TAI between the two groups.
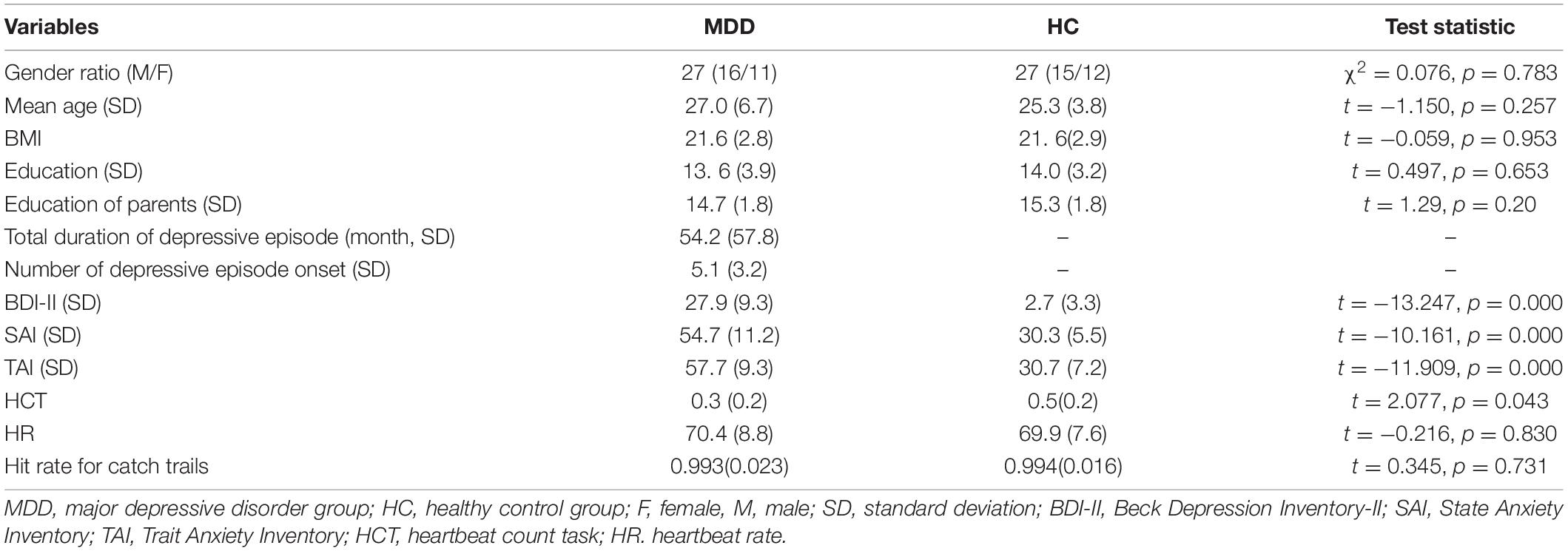
Table 1. Demographic information for the major depressive disorder (MDD) group and healthy control (HC) group.
Heartbeat-Evoked Potentials Component
As shown in Figure 2, HEPs during the prediction stage (predict-HEPs) are significantly attenuated in MDDs vs. HCs (p < 0.005, marked by the red pot in Figure 2). While there were no significant differences in ECG during the same stage, which indicated that the significant differences of HEP amplitudes were not evoked by ECG (ECG during prediction stage: t = − 1.291, p = 0.203).
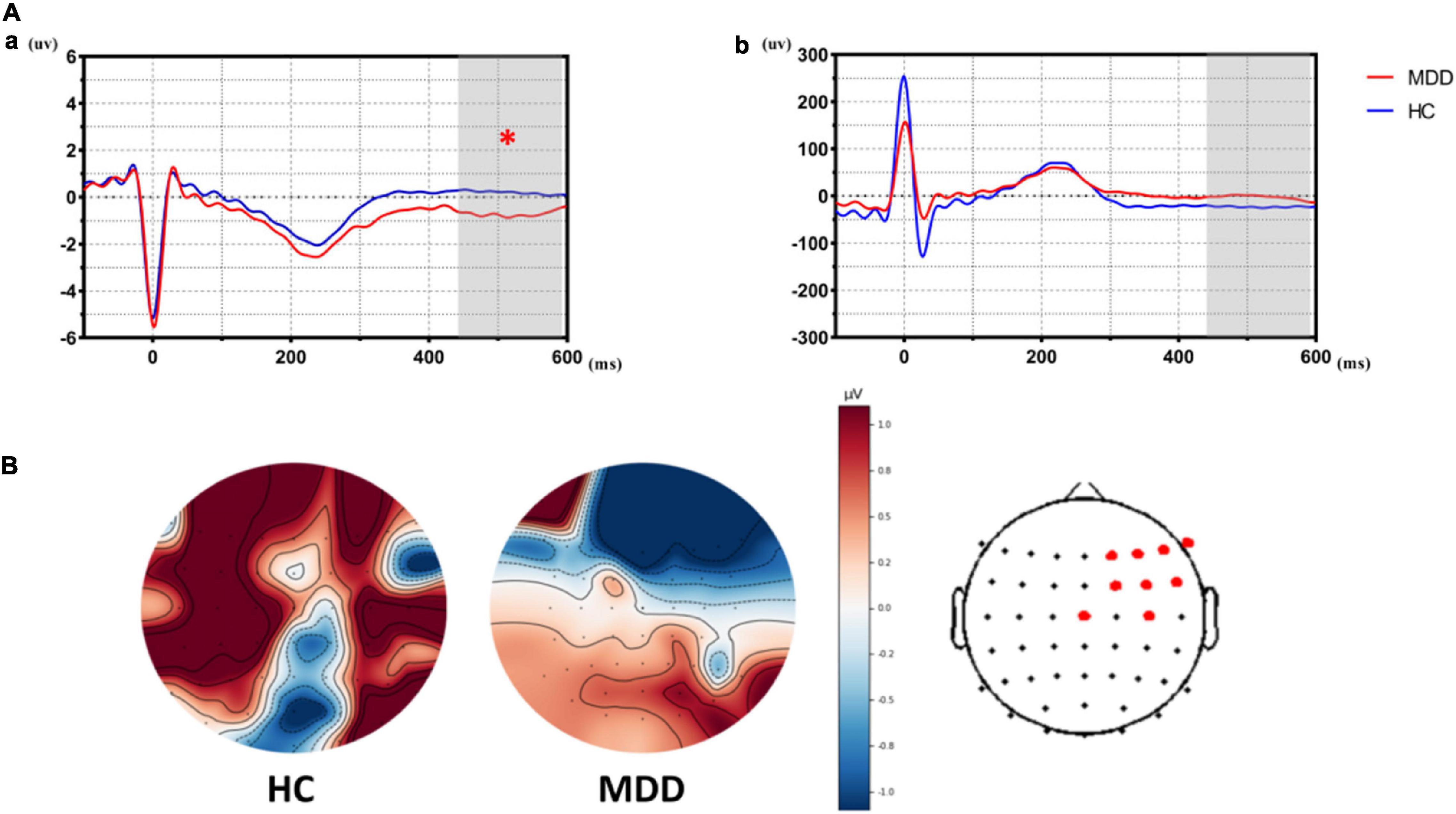
Figure 2. The differences of heartbeat-evoked potentials (HEPs) in the stage of affective prediction between the major depressive disorder (MDD) group and the healthy control (HC) group. (Aa) Showed significant differences in HEPs during the prediction stage between the MDD group and the HC group. (Ab) Suggested that significant differences did not exist in ECG during the prediction stage between the MDD group and the HC group, which indicated significant differences in HEPs did not result from the effect of cardiac field artifact (CFA). (B) Topological graph of HEP in both groups. Electrodes marked with red color indicate a significant group-difference.
Late Positive Potentials Component
As shown in Figure 3, LPPs in MDDs over right centroparietal sites are significantly attenuated in all emotional face trials while LPPs over left frontocentral sites are significantly increased in sad and neutral faces than HCs (p < 0.005).
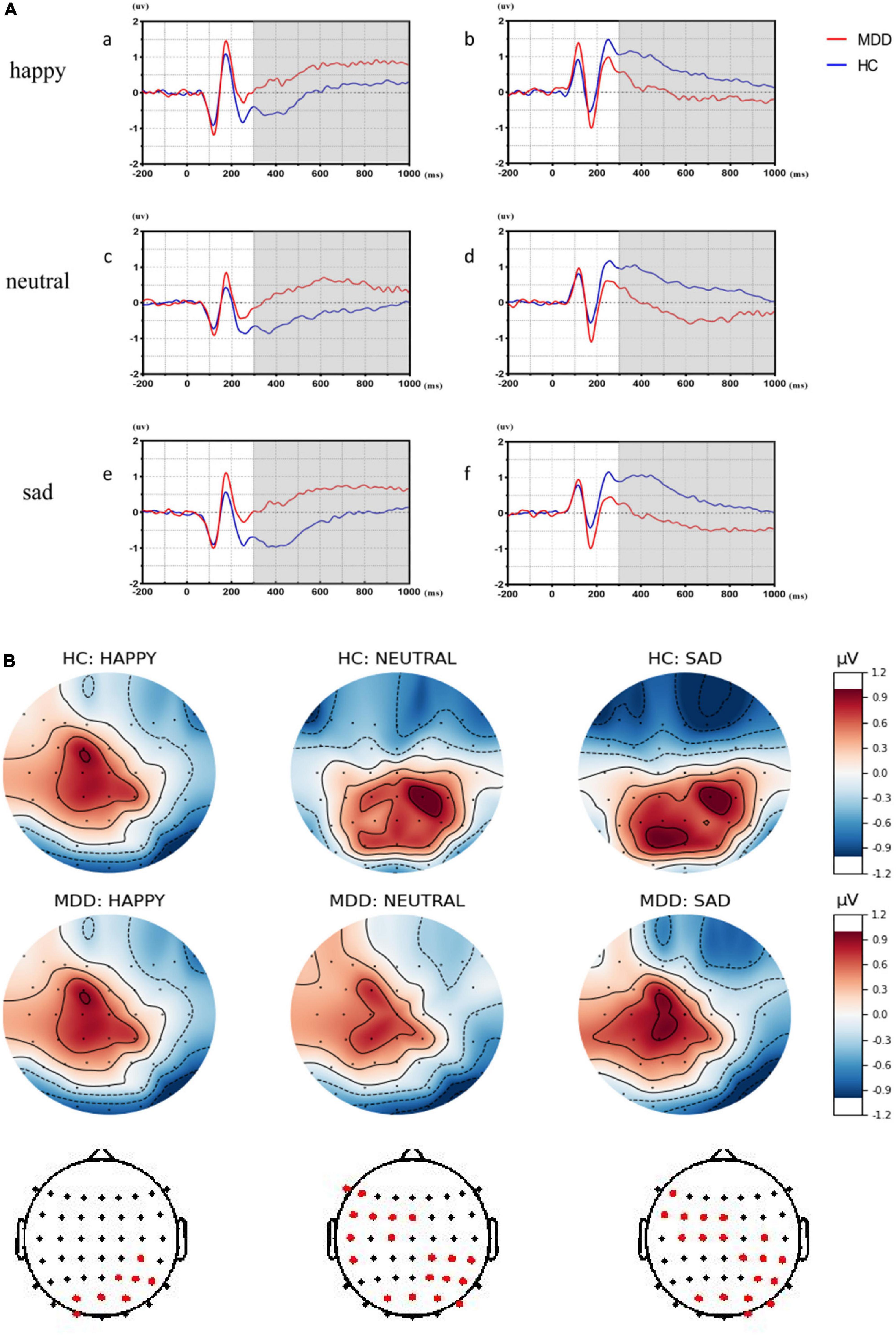
Figure 3. The significant differences of late positive potentials (LPPs) in different affective faces trials between major depressive disorder (MDD) group and healthy control (HC) group. (Aa,c,e) Showed the significant differences over left frontocentral sites between the MDD group and the HC group by averaging significant differences in electrodes in different affective face trials. (Ab,d,f) Showed the significant differences over right centroparietal sites between MDD group and HC group by averaging significant differences in electrodes in different affective face trials. (B) Was the top plots with significant differences for LPPs.
Source Reconstruction of Event-Related Potential Components
Source localization analysis was applied to find the underlying responsible generators of HEPs during the prediction stage and LPPs in sad face trials. Non-parametric voxel-wise tests with p < 0.01 were used to compare sources between HCs and MDDs, separately for HEPs and LPPs.
The Different Source Localization of Heartbeat-Evoked Potentials in Major Depressive Disorder Group vs. Healthy Control Group
As shown in Figure 4, the sLORETA analysis suggests that there are 963 voxels different in two groups, mainly located in the frontal lobe [middle frontal gyrus (BA6) (maximal activity at montreal neurological institute (MNI) coordinates 30, 15, and 60), superior frontal gyrus (BA8) (maximal activity at MNI coordinates 25, 20, and 55)], and limbic lobe [cingulate gyrus (BA32) (maximal activity at MNI coordinates − 5, 20, and 45)]. These significant voxels showed a higher source activation in the HC group than the MDD group.
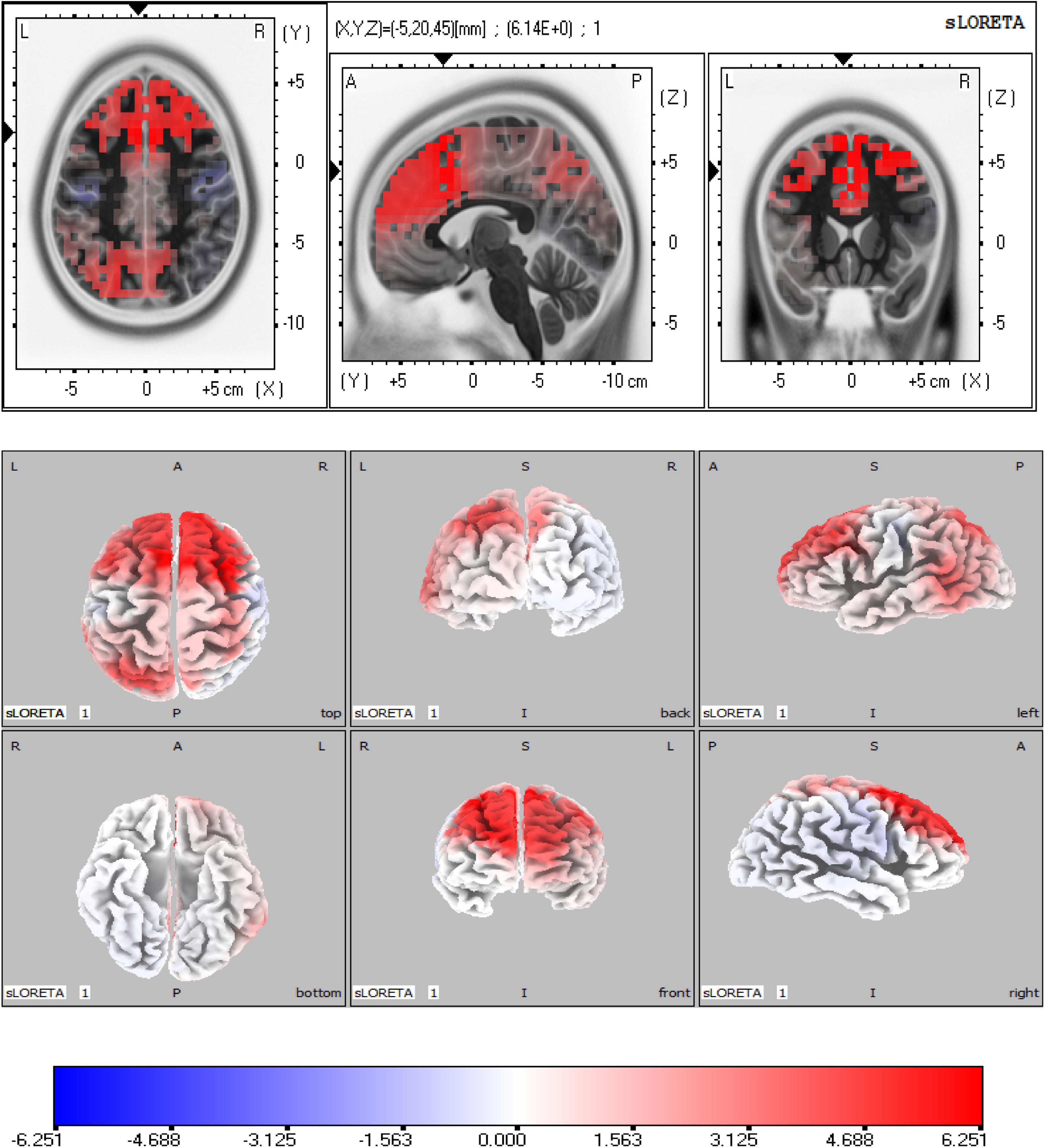
Figure 4. Source analysis showed the attenuated Heartbeat-evoked potentials (HEPs) in major depressive disorder (MDD) mainly resulted from the frontal lobe (BA6, BA8) and limbic lobe (BA32). The marked site was anterior cingulate cortex (ACC) (BA32).
The Different Source Localization of Sad-Late Positive Potentials in Major Depressive Disorder vs. Healthy Control
As shown in Figure 5, the significant differences of sad-LPPs are mainly located in the right hemisphere. The sLORETA analysis showed that the source activation of the HC group was significantly higher in limbic lobe than the MDD group, especially in the anterior cingulate (BA32) (maximal activity at MNI coordinates 5, 30, and 25). While in the parietal lobe, such as the postcentral gyrus (BA2, BA40) (maximal activity at MNI coordinates 60, −30, and 20), inferior parietal lobe (BA40) (maximal activity at MNI coordinates 65, −45, 25), the source activation is higher in MDDs than HCs. In addition, the right insula (BA13) (maximal activity at MNI coordinates 55, −35, and 20) is also salient in MDDs.
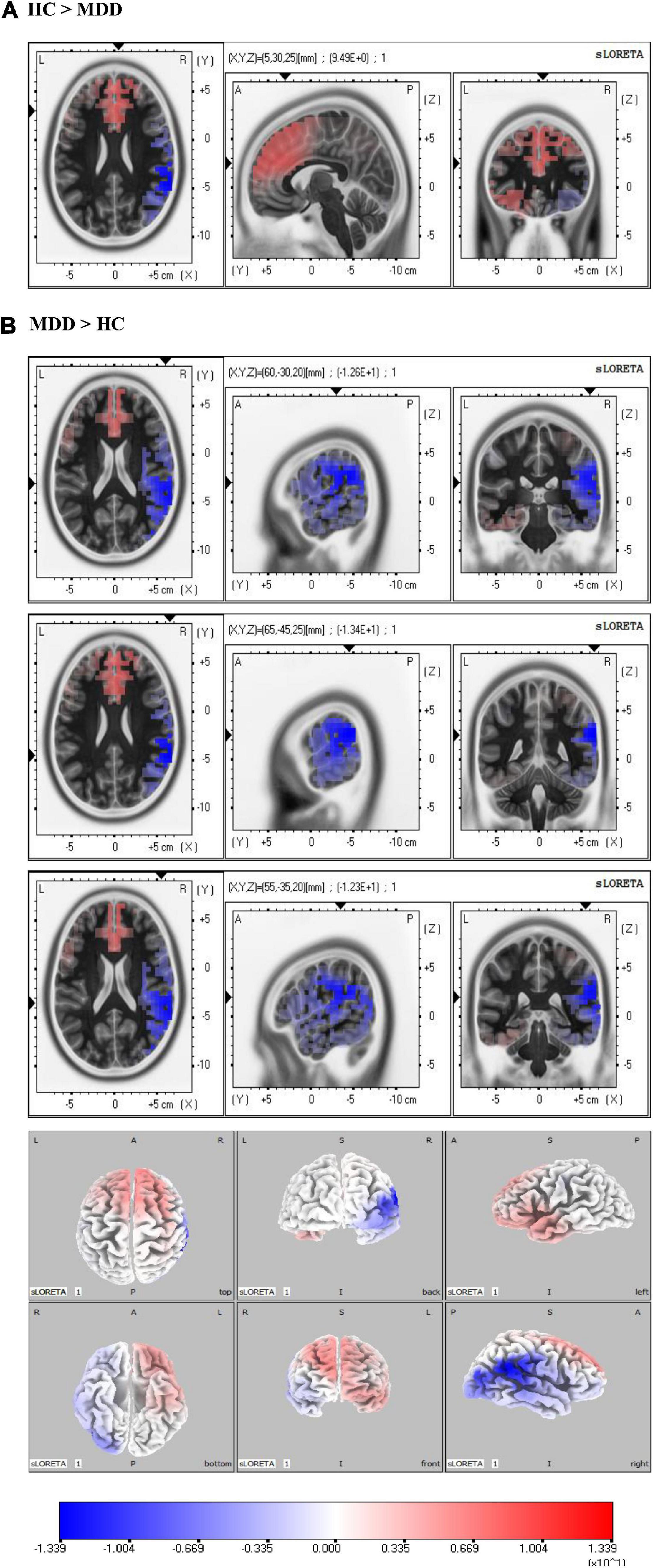
Figure 5. Source analysis in the time window of sad-late positive potentials (LPPs). (A) Revealed the activity of anterior cingulate cortex (ACC) in the healthy control (HC) group was higher than major depressive disorder (MDD) group. In (B), the MDD group showed higher activity in the right parietal lobe. Especially, the right insula showed higher activity in the MDD group than the HC group.
Regression Model Analysis
As shown in Figure 6, only under sad face stimuli, significant differences in HEPs are correlated with LPPs cluster in the MDD group. In the MDD group, HEP electrode sites have a significantly positive correlation with LPP electrode sites in three clusters as shown in Figure 4. These results showed that the all significant HEP electrode sites could positively predict LPPs in left frontocentral sites (F5, F3, FC5, FC3, and FC1) (r = 0.586, p = 0.008). HEPs in right frontocentral sites (FC2, FC4, and FC6) were negatively correlated with LPPs in centroparietal sites (C4, CP4, and CP6) (r = − 0.545, p = 0.016), while HEPs in right frontal sites (F2, F4, and F6) were negatively correlated with LPPs in parieto-occipital sites (PO4, PO8, and O2) (r = − 0.470, p = 0.042). However, the correlation did not appear in the HC group.
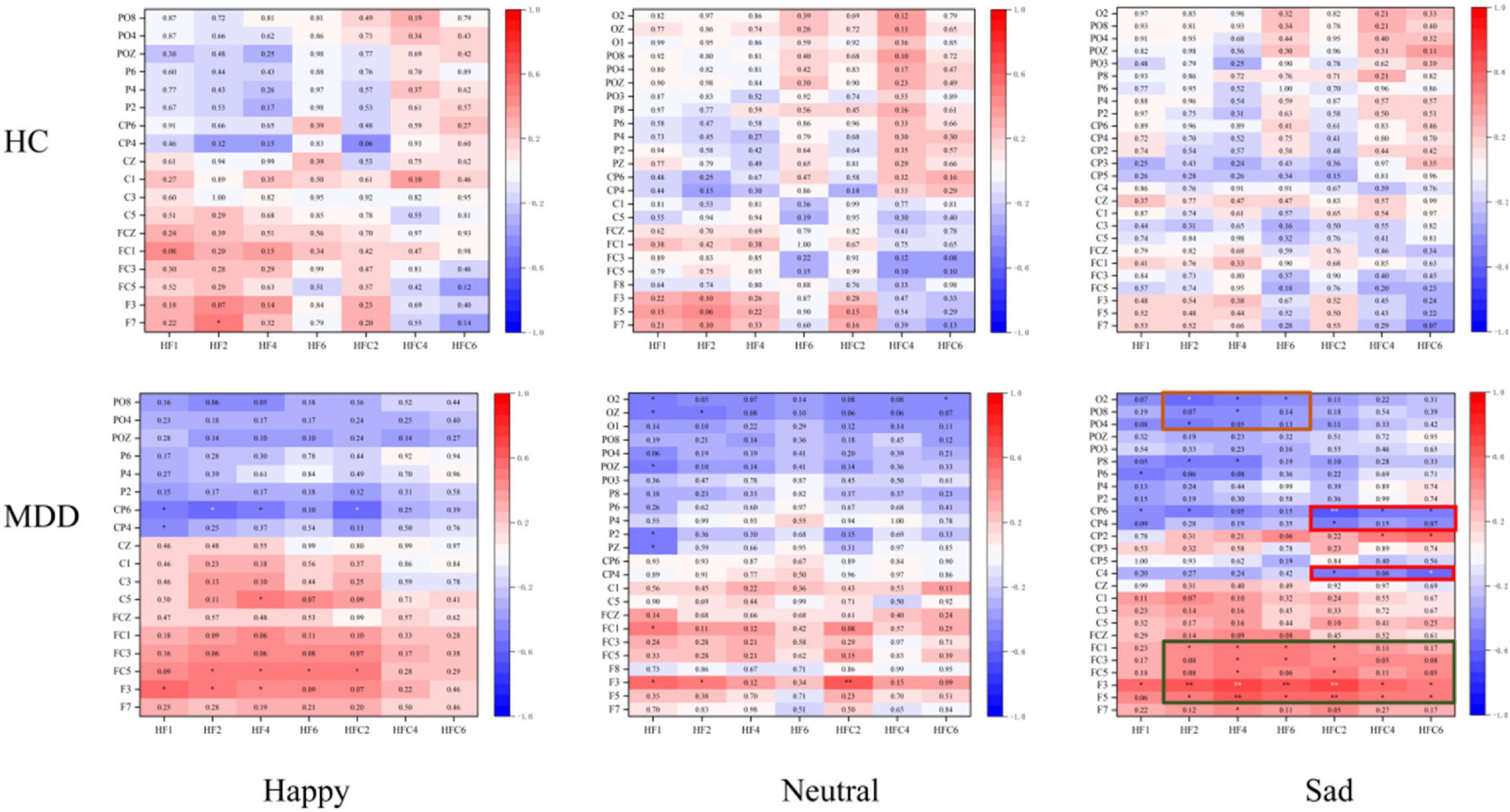
Figure 6. The correlation between predict-heartbeat-evoked potentials (HEPs) and sad-late positive potentials (LPPs) in major depressive disorder (MDD) and healthy control (HC) groups. The significantly correlated clusters were only found in the MDD group.
In addition, the item-20 in BDI-II (“evaluation of own health”) correlated with HEPs in right frontocentral sites positively in MDDs (r = 0.538, p = 0.010), while the scores of HCT were negative correlated with the scores of TAI (r = − 0.468, p = 0.028) in HCs. LPPs in right centroparietal sites (C4, CP4, and CP6) were negatively correlated with a cognitive factor of BDI-II (r = − 0.464, p = 0.034) in MDD. With stepwise regression analysis, two optimally predictive models were created. The equation is shown below:
Where the adjusted R2 statistic was 0.360 and the p of the model was 0.002. The values of p for included variables in (1) are shown in Table 2.
Where the adjusted R2 statistic was 0.470 and the p of the model was 0.000. The values of p for included variables in (2) are shown in Table 3.
Discussion
To our knowledge, this study was the first to investigate the negative emotional bias in MDD from the aspect of internal-external information integration by interoception. With converging evidence reporting the intimate connections between the peripheral nervous system and emotion in MDD (55, 56), the role of allostasis in depression pathological mechanism could not be ignored anymore. In the current study, we found that abnormal HEPs could predict aberrant LPP clusters in sad face trials only in the MDD group, indicating the possibility that aberrant interoception dysfunction has led to abnormal processing of sad faces in MDDs. Furthermore, by combing with source location analysis and previous research studies (29, 57), we cautiously provided the initial evidence that low activity of ACC might be the primary source of attenuated HEPs and predicted the overactivity of the right insula in sad face processing in MDD. These results revealed that an abnormal internal-external interactive way could be the reason for impairment of emotion processing in MDD and provide valuable clew to extend the understanding of pathological mechanism of depression.
Many studies had reported that depressive patients had abnormal interoception that is reflected by poor performance in HCT and attenuated average HEP amplitudes (27, 58). Our study had similar findings. HEP, which is generated from the cardiac signal and processed by the brain, has been proved to be a valid index for interoception (59). Previous studies focused on the relationship between HEPs and early visual evoked potentials have suggested that HEPs could predict the threshold of the visual sad faces in healthy people (60). Here, considering the relatively slow conduction of interoceptive information by weakly myelinated neurons (61), we focused on the relationship between HEPs and LPPs (62, 63). We found blunt HEPs over frontocentral regions before emotion stimulation could predict abnormal LPPs over right centroparietal sites and left frontocentral regions under emotion stimulation. More importantly, aberrant HEPs contribute to abnormal LPPs under sad emotion processing merely existed in MDD. Although limited studies in this field have been conducted in MDD, recently research studies that focused on the association between HEPs and psychiatric disorders have dramatically increased, such as social anxiety, nightmare disorder, and borderline personality disorder (57, 64–66), and revealed that interoception fluctuation contributed to perception dysfunction. Negative emotional bias seems to indicate that patients with MDD had affective perception dysfunction. Our results validated this point and further elucidated the potential neuroimaging mechanism that negative emotional bias in MDD would have resulted from the dysfunction of interoception. In our study, attenuated HEPs predicted more health-like sad-LPPs in MDD, suggesting that the HEP worked as a self-compensation to recruit more cognition resources for negative emotion processing (67–69).
Combined with source analysis, the present results showed that abnormal interoception contributed to aberrant emotion processing in two different ways in MDD. One of them was controlled by frontal electrodes. In this way, predict-HEPs could predict LPP amplitude over left frontocentral sites under sad face context, which was implicated in low activity ACC. ACC was one of the major sources of HEPs and was famous for its autonomic function (39). Generally, ACC received wide nerve projections from the frontal lobe and took part in negative emotion-related information processing (70–73). In major depression, it was reported that the connectivity between ACC and orbitofrontal cortex (OFC) was decreased with increasing depressive symptoms (29, 74) and electrical stimulation in ACC could relieve depression (75). In our study, hypoactive ACC performed its limited ability in a time frame of both predict-HEPs and sad-LPPs. Considering the crucial role of ACC in viscera signal processing and emotion processing, it was easy to understand that the low activity ACC contributed to abnormal HEPs and emotion-related LPPs, which was in consonance with the fact that HEP was correlated with LPPs over left frontocentral sites positively. The other of them was a cognition-related way that is mainly controlled by right centroparietal sites under sad content. Sad-LPPs over centroparietal sites were reported to have intimate connections with emotional motivation and were correlated with the cognition scores in BDI-II in our work. The hyperactivity of the right insula (BA40) might be the source of abnormal sad-LPPs. The right insula was famous for its high response in negative emotion processing (70, 76) and was provided to have a connection with depression’s negative emotional bias (77). Notably, the hyperactivity right insula in sadness could be predicted by hypoactive ACC, which validated the insight that interoception constitutes the emotion context by the interaction between limbic sensory (right insula) and limbic motor (ACC).
In our study, we suggested that the self-compensation of cognition source function is mediated by the information flow from ACC to the right insula. When processing sad face information, hypoactive ACC led to low emotional motivation which led to the potential negative effects, and to protect oneself, the right insula had to over-activated trying to arousing activation of ACC. This also could explain the result that blunt average amplitudes of predict-HEPs would arouse better sad-LPPs over right centroparietal sites.
In conclusion, the abnormal HEPs predict aberrant LPP amplitudes in MDD, which may result from the ACC and right insula. This study highlighted the importance of interoception for depressive patients and indicated the underlying mechanism of interoception dysfunction in emotional information processing. The study highlights interoception in MDD not only contributes to somatic symptoms but also affects emotion processing. The role of interoception in MDD is seriously undervalued. What is more, the therapy for MDD without considering homeostasis, especially at the cost of disturbing autonomic function, should be seriously reconsidered.
Limitations
The major limitation in this study is a relatively small sample size, therefore, the results must be considered as preliminary. The cardiac rates of depressive patients were easy to increase during the task. To keep the accuracy of HEPs, we had to choose the segmentation from − 100 to 600 ms and excluded people whose heartbeats were above 86/min. In addition, considering the heartbeat is constantly changing, it is difficult and inappropriate to study the direction of information flow in our study by effective connectivity and other methods. We could only infer the process by time sequence. Future studies with larger sample sizes and optimized parameters are warranted to further verify these findings.
Data Availability Statement
The raw data supporting the conclusions of this article will be made available by the authors, without undue reservation.
Ethics Statement
The studies involving human participants were reviewed and approved by the Affiliated Nanjing Brain Hospital of Nanjing Medical University. The patients/participants provided their written informed consent to participate in this study.
Author Contributions
HLZ: data curation, formal analysis, methodology, writing-original draft, and writing-review and editing. HWZ: data curation. ZPD, SZ, LLH, YX, YLH, RY, HT, YHH, YSD, and XQW: methodology. QL and ZJY: data curation, formal analysis, methodology, and writing-review and editing. All authors contributed to the article and approved the submitted version.
Funding
This work was supported by the National Natural Science Foundations of China (grant no. 81871066), the Jiangsu Provincial Medical Innovation Team of the Project of Invigorating Health Care through Science, Technology and Education (grant no. CXTDC2016004), and the Jiangsu Provincial Key Research and Development Program (grant no. BE2018609).
Conflict of Interest
The authors declare that the research was conducted in the absence of any commercial or financial relationships that could be construed as a potential conflict of interest.
Publisher’s Note
All claims expressed in this article are solely those of the authors and do not necessarily represent those of their affiliated organizations, or those of the publisher, the editors and the reviewers. Any product that may be evaluated in this article, or claim that may be made by its manufacturer, is not guaranteed or endorsed by the publisher.
Acknowledgments
We thank the editors and reviewers of this manuscript for many helpful suggestions.
Footnotes
References
1. Thornicroft G, Chatterji S, Evans-Lacko S, Gruber M, Sampson N, Aguilar-Gaxiola S, et al. Undertreatment of people with major depressive disorder in 21 countries. Br J Psychiatry. (2017) 210:119–24. doi: 10.1192/bjp.bp.116.188078
2. Joormann J, Stanton CH. Examining emotion regulation in depression: a review and future directions. Behav Res Ther. (2016) 86:35–49. doi: 10.1016/j.brat.2016.07.007
3. Roiser JP, Elliott R, Sahakian BJ. Cognitive mechanisms of treatment in depression. Neuropsychopharmacology. (2012) 37:117–36. doi: 10.1038/npp.2011.183
4. Gorka SM, Young CB, Klumpp H, Kennedy AE, Francis J, Ajilore O, et al. Emotion-based brain mechanisms and predictors for SSRI and CBT treatment of anxiety and depression: a randomized trial. Neuropsychopharmacology. (2019) 44:1639–48. doi: 10.1038/s41386-019-0407-7
5. Buchheim A, Labek K, Taubner S, Kessler H, Pokorny D, Kächele H, et al. Modulation of gamma band activity and late positive potential in patients with chronic depression after psychodynamic psychotherapy. Psychother Psychosom. (2018) 87:252–4. doi: 10.1159/000488090
6. Auerbach RP, Stewart JG, Stanton CH, Mueller EM, Pizzagalli DA. Emotion-processing biases and resting EEG activity in depressed adolescents. Depress Anxiety. (2015) 32:693–701. doi: 10.1002/da.22381
7. Delle-Vigne D, Wang W, Kornreich C, Verbanck P, Campanella S. Emotional facial expression processing in depression: data from behavioral and event-related potential studies. Neurophysiol Clin. (2014) 44:169–87. doi: 10.1016/j.neucli.2014.03.003
8. Chechko N, Augustin M, Zvyagintsev M, Schneider F, Habel U, Kellermann T. Brain circuitries involved in emotional interference task in major depression disorder. J Affect Disord. (2013) 149:136–45. doi: 10.1016/j.jad.2013.01.013
9. MacNamara A, Klumpp H, Kennedy AE, Langenecker SA, Phan KL. Transdiagnostic neural correlates of affective face processing in anxiety and depression. Depress Anxiety. (2017) 34:621–31. doi: 10.1002/da.22631
10. Mertens LJ, Wall MB, Roseman L, Demetriou L, Nutt DJ, Carhart-Harris RL. Therapeutic mechanisms of psilocybin: changes in amygdala and prefrontal functional connectivity during emotional processing after psilocybin for treatment-resistant depression. J Psychopharmacol. (2020) 34:167–80. doi: 10.1177/0269881119895520
11. Dennis TA, Hajcak G. The late positive potential: a neurophysiological marker for emotion regulation in children. J Child Psychol Psychiatry. (2009) 50:1373–83. doi: 10.1111/j.1469-7610.2009.02168.x
12. McGhie SF, Holbrook A, Arienzo D, Amir N. Psychometric properties of the late positive potential in adult females. Biol Psychol. (2021) 163:108145. doi: 10.1016/j.biopsycho.2021.108145
13. Hajcak G, Foti D. Significance?… Significance! Empirical, methodological, and theoretical connections between the late positive potential and P300 as neural responses to stimulus significance: an integrative review. Psychophysiology. (2020) 57:e13570. doi: 10.1111/psyp.13570
14. Kim BM, Lee J, Choi AR, Chung SJ, Park M, Koo JW, et al. Event-related brain response to visual cues in individuals with internet gaming disorder: relevance to attentional bias and decision-making. Transl Psychiatry. (2021) 11:258. doi: 10.1038/s41398-021-01375-x
15. Mardaga S, Iakimova G. Neurocognitive processing of emotion facial expressions in individuals with self-reported depressive symptoms: the role of personality and anxiety. Neurophysiol Clin. (2014) 44:447–55. doi: 10.1016/j.neucli.2014.08.007
16. Stephen DG, Megan HP, Anthony SB, Whitney AH, Michael C. The poverty of embodied cognition. Psychon Bull Rev. (2016) 23:959–78. doi: 10.3758/s13423-015-0860-1
17. Guell X, Gabrieli J, Schmahmann JD. Embodied cognition and the cerebellum: perspectives from the dysmetria of thought and the universal cerebellar transform theories. Cortex. (2017) 100:140–8. doi: 10.1016/j.cortex.2017.07.005
18. Giovanni S, Massimo B, Anthony V, John C, Milena M. Abnormal body phenomena in persons with major depressive disorder. Psychopathology. (2021) 54:203–13. doi: 10.1159/000514642
19. Rao RP, Ballard DH. Predictive coding in the visual cortex: a functional interpretation of some extra-classical receptive-field effects. Nat Neurosci. (1999) 2:79–87. doi: 10.1038/4580
20. Friston K. A theory of cortical responses. Philos Trans R Soc Lond B Biol Sci. (2005) 360:815–36. doi: 10.1098/rstb.2005.1622
21. Barrett LF, Quigley KS, Hamilton P. An active inference theory of allostasis and interoception in depression. Philos Trans R Soc Lond B Biol Sci. (2016) 371:20160011. doi: 10.1098/rstb.2016.0011
22. Hultman R, Ulrich K, Sachs BD, Blount C, Carlson DE, Ndubuizu N, et al. Brain-wide electrical spatiotemporal dynamics encode depression vulnerability. Cell. (2018) 173:166–80.e14. doi: 10.1016/j.cell.2018.02.012
23. Kleckner IR, Zhang J, Touroutoglou A, Chanes L, Xia C, Simmons WK, et al. Evidence for a large-scale brain system supporting allostasis and interoception in humans. Nat Hum Behav. (2017) 1:0069. doi: 10.1038/s41562-017-0069
24. Bauer ME, Teixeira AL. Inflammation in psychiatric disorders: what comes first? Ann N Y Acad Sci. (2019) 1437:57–67. doi: 10.1111/nyas.13712
25. Chevalier G, Siopi E, Guenin-Macé L, Pascal M, Laval T, Rifflet A, et al. Effect of gut microbiota on depressive-like behaviors in mice is mediated by the endocannabinoid system. Nat Commun. (2020) 11:6363. doi: 10.1038/s41467-020-19931-2
26. Ogłodek E, Szota A, Just M, Moś D, Araszkiewicz A. The role of the neuroendocrine and immune systems in the pathogenesis of depression. Pharmacol Rep. (2014) 66:776–81. doi: 10.1016/j.pharep.2014.04.009
27. Harshaw C. Interoceptive dysfunction: toward an integrated framework for understanding somatic and affective disturbance in depression. Psychol Bull. (2015) 141:311–63. doi: 10.1037/a0038101
28. Ruohonen EM, Alhainen V, Astikainen P. Event-related potentials to task-irrelevant sad faces as a state marker of depression. Biol Psychol. (2019) 149:107806. doi: 10.1016/j.biopsycho.2019.107806
29. Park C, Rosenblat JD, Lee Y, Pan Z, Cao B, Iacobucci M, et al. The neural systems of emotion regulation and abnormalities in major depressive disorder. Behav Brain Res. (2019) 367:181–8. doi: 10.1016/j.bbr.2019.04.002
30. Garfinkel SN, Manassei MF, Hamilton-Fletcher G, In den Bosch Y, Critchley HD, Engels M. Interoceptive dimensions across cardiac and respiratory axes. Philos Trans R Soc Lond B Biol Sci. (2016) 371:20160014. doi: 10.1098/rstb.2016.0014
31. Montoya P, Schandry R, Müller A. Heartbeat evoked potentials (HEP): topography and influence of cardiac awareness and focus of attention. Electroencephalogr Clin Neurophysiol. (1993) 88:163–72. doi: 10.1016/0168-5597(93)90001-6
32. Pollatos O, Schandry R. Accuracy of heartbeat perception is reflected in the amplitude of the heartbeat-evoked brain potential. Psychophysiology. (2004) 41:476–82. doi: 10.1111/1469-8986.2004.00170.x
33. Ito Y, Shibata M, Tanaka Y, Terasawa Y, Umeda S. Affective and temporal orientation of thoughts: electrophysiological evidence. Brain Res. (2019) 1719:148–56. doi: 10.1016/j.brainres.2019.05.041
34. Terhaar J, Viola FC, Bär KJ, Debener S. Heartbeat evoked potentials mirror altered body perception in depressed patients. Clin Neurophysiol. (2012) 123:1950–7. doi: 10.1016/j.clinph.2012.02.086
35. Lackner RJ, Fresco DM. Interaction effect of brooding rumination and interoceptive awareness on depression and anxiety symptoms. Behav Res Ther. (2016) 85:43–52. doi: 10.1016/j.brat.2016.08.007
36. Jakubczyk A, Trucco EM, Klimkiewicz A, Skrzeszewski J, Suszek H, Zaorska J, et al. Association between interoception and emotion regulation in individuals with alcohol use disorder. Front Psychiatry. (2019) 10:1028. doi: 10.3389/fpsyt.2019.01028
37. Williams IA, Reuber M, Levita L. Interoception and stress in patients with functional neurological symptom disorder. Cogn Neuropsychiatry. (2021) 26:75–94. doi: 10.1080/13546805.2020.1865895
38. Eggart M, Todd J, Valdés-Stauber J. Validation of the Multidimensional Assessment of Interoceptive Awareness (MAIA-2) questionnaire in hospitalized patients with major depressive disorder. PLoS One. (2021) 16:e0253913. doi: 10.1371/journal.pone.0253913
39. Park HD, Blanke O. Heartbeat-evoked cortical responses: underlying mechanisms, functional roles, and methodological considerations. Neuroimage. (2019) 197:502–11. doi: 10.1016/j.neuroimage.2019.04.081
41. Spielberger CD. Manual for the State-Trait Anxiety Inventory (Form Y1-Y2). Palo Alto, CA: Consulting Psychologists Press (1970).
42. Schandry R, Montoya P. Event-related brain potentials and the processing of cardiac activity. Biol Psychol. (1996) 42:75–85. doi: 10.1016/0301-0511(95)05147-3
43. Wang Y. Standardization and assessment of college students’ facial expression of emotion. Chin J Clin Psychol. (2005) 13:396–8. doi: 10.1016/j.nepr.2019.02.017
44. Makeig S, Debener S, Onton J, Delorme A. Mining event-related brain dynamics. Trends Cogn Sci. (2004) 8:204–10. doi: 10.1016/j.tics.2004.03.008
45. Bair A, Marksteiner J, Falch R, Ettinger U, Reyes Del Paso GA, Duschek S. Features of autonomic cardiovascular control during cognition in major depressive disorder. Psychophysiology. (2021) 58:e13628. doi: 10.1111/psyp.13628
46. Chesnut M, Harati S, Paredes P, Khan Y, Foudeh A, Kim J, et al. Stress markers for mental states and biotypes of depression and anxiety: a scoping review and preliminary illustrative analysis. Chronic Stress (Thousand Oaks). (2021) 5:24705470211000338. doi: 10.1177/24705470211000338
47. Sgoifo A, Carnevali L, Alfonso Mde L, Amore M. Autonomic dysfunction and heart rate variability in depression. Stress. (2015) 18:343–52. doi: 10.3109/10253890.2015.1045868
48. Gentsch A, Sel A, Marshall AC, Schütz-Bosbach S. Affective interoceptive inference: evidence from heart-beat evoked brain potentials. Hum Brain Mapp. (2019) 40:20–33. doi: 10.1002/hbm.24352
49. Marshall AC, Gentsch A, Schütz-Bosbach S. Interoceptive cardiac expectations to emotional stimuli predict visual perception. Emotion. (2020) 20:1113–26. doi: 10.1037/emo0000631
50. Raimondo F, Rohaut B, Demertzi A, Valente M, Engemann DA, Salti M, et al. Brain-heart interactions reveal consciousness in noncommunicating patients. Ann Neurol. (2017) 82:578–91. doi: 10.1002/ana.25045
51. Gray MA, Taggart P, Sutton PM, Groves D, Holdright DR, Bradbury D, et al. A cortical potential reflecting cardiac function. Proc Natl Acad Sci U S A. (2007) 104:6818–23. doi: 10.1073/pnas.0609509104
52. Schulz A, Ferreira de Sá DS, Dierolf AM, Lutz A, van Dyck Z, Vögele C, et al. Short-term food deprivation increases amplitudes of heartbeat-evoked potentials. Psychophysiology. (2015) 52:695–703. doi: 10.1111/psyp.12388
53. Schulz A, Köster S, Beutel ME, Schächinger H, Vögele C, Rost S, et al. Altered patterns of heartbeat-evoked potentials in depersonalization/derealization disorder: neurophysiological evidence for impaired cortical representation of bodily signals. Psychosom Med. (2015) 77:506–16. doi: 10.1097/psy.0000000000000195
54. Shao S, Shen K, Wilder-Smith EP, Li X. Effect of pain perception on the heartbeat evoked potential. Clin Neurophysiol. (2011) 122:1838–45. doi: 10.1016/j.clinph.2011.02.014
55. Stone LB, McCormack CC, Bylsma LM. Cross system autonomic balance and regulation: associations with depression and anxiety symptoms. Psychophysiology. (2020) 57:e13636. doi: 10.1111/psyp.13636
56. Wenzler S, Hagen M, Tarvainen MP, Hilke M, Ghirmai N, Huthmacher AC, et al. Intensified emotion perception in depression: differences in physiological arousal and subjective perceptions. Psychiatry Res. (2017) 253:303–10. doi: 10.1016/j.psychres.2017.03.040
57. Perogamvros L, Park HD, Bayer L, Perrault AA, Blanke O, Schwartz S. Increased heartbeat-evoked potential during REM sleep in nightmare disorder. Neuroimage Clin. (2019) 22:101701. doi: 10.1016/j.nicl.2019.101701
58. Quadt L, Critchley HD, Garfinkel SN. The neurobiology of interoception in health and disease. Ann N Y Acad Sci. (2018) 1428:112–28. doi: 10.1111/nyas.13915
59. Coll MP, Hobson H, Bird G, Murphy J. Systematic review and meta-analysis of the relationship between the heartbeat-evoked potential and interoception. Neurosci Biobehav Rev. (2021) 122:190–200. doi: 10.1016/j.neubiorev.2020.12.012
60. Marshall AC, Gentsch A, Schröder L, Schütz-Bosbach S. Cardiac interoceptive learning is modulated by emotional valence perceived from facial expressions. Soc Cogn Affect Neurosci. (2018) 13:677–86. doi: 10.1093/scan/nsy042
61. Pace-Schott EF, Amole MC, Aue T, Balconi M, Bylsma LM, Critchley H, et al. Physiological feelings. Neurosci Biobehav Rev. (2019) 103:267–304. doi: 10.1016/j.neubiorev.2019.05.002
62. Weinberg A, Perlman G, Kotov R, Hajcak G. Depression and reduced neural response to emotional images: distinction from anxiety, and importance of symptom dimensions and age of onset. J Abnorm Psychol. (2016) 125:26–39. doi: 10.1037/abn0000118
63. Disner SG, Beevers CG, Haigh EA, Beck AT. Neural mechanisms of the cognitive model of depression. Nat Rev Neurosci. (2011) 12:467–77. doi: 10.1038/nrn3027
64. Pang J, Tang X, Li H, Hu Q, Cui H, Zhang L, et al. Altered interoceptive processing in generalized anxiety disorder-a heartbeat-evoked potential research. Front Psychiatry. (2019) 10:616. doi: 10.3389/fpsyt.2019.00616
65. Müller LE, Schulz A, Andermann M, Gäbel A, Gescher DM, Spohn A, et al. Cortical representation of afferent bodily signals in borderline personality disorder: neural correlates and relationship to emotional dysregulation. JAMA Psychiatry. (2015) 72:1077–86. doi: 10.1001/jamapsychiatry.2015.1252
66. Kim J, Park HD, Kim KW, Shin DW, Lim S, Kwon H, et al. Sad faces increase the heartbeat-associated interoceptive information flow within the salience network: a MEG study. Sci Rep. (2019) 9:430. doi: 10.1038/s41598-018-36498-7
67. Raoelison M, Thompson VA, De Neys W. The smart intuitor: cognitive capacity predicts intuitive rather than deliberate thinking. Cognition. (2020) 204:104381. doi: 10.1016/j.cognition.2020.104381
68. Kopala-Sibley DC, Zuroff DC. The self and depression: four psychological theories and their potential neural correlates. J Pers. (2020) 88:14–30. doi: 10.1111/jopy.12456
70. Barthas F, Sellmeijer J, Hugel S, Waltisperger E, Barrot M, Yalcin I. The anterior cingulate cortex is a critical hub for pain-induced depression. Biol Psychiatry. (2015) 77:236–45. doi: 10.1016/j.biopsych.2014.08.004
71. He Z, Lu F, Sheng W, Han S, Pang Y, Chen Y, et al. Abnormal functional connectivity as neural biological substrate of trait and state characteristics in major depressive disorder. Prog Neuropsychopharmacol Biol Psychiatry. (2020) 102:109949. doi: 10.1016/j.pnpbp.2020.109949
72. Lichenstein SD, Verstynen T, Forbes EE. Adolescent brain development and depression: a case for the importance of connectivity of the anterior cingulate cortex. Neurosci Biobehav Rev. (2016) 70:271–87. doi: 10.1016/j.neubiorev.2016.07.024
73. Philippi CL, Cornejo MD, Frost CP, Walsh EC, Hoks RM, Birn R, et al. Neural and behavioral correlates of negative self-focused thought associated with depression. Hum Brain Mapp. (2018) 39:2246–57. doi: 10.1002/hbm.24003
74. Rive MM, van Rooijen G, Veltman DJ, Phillips ML, Schene AH, Ruhé HG. Neural correlates of dysfunctional emotion regulation in major depressive disorder. A systematic review of neuroimaging studies. Neurosci Biobehav Rev. (2013) 37:2529–53. doi: 10.1016/j.neubiorev.2013.07.018
75. Paillère Martinot ML, Martinot JL, Ringuenet D, Galinowski A, Gallarda T, Bellivier F, et al. Baseline brain metabolism in resistant depression and response to transcranial magnetic stimulation. Neuropsychopharmacology. (2011) 36:2710–9. doi: 10.1038/npp.2011.161
76. Eisenberger NI, Lieberman MD, Williams KD. Does rejection hurt? An FMRI study of social exclusion. Science. (2003) 302:290–2. doi: 10.1126/science.1089134
Keywords: major depressive disorders, negative emotional bias, interoception, predictive coding, HEP (heartbeat evoked potential)
Citation: Zhou H, Zou H, Dai Z, Zhao S, Hua L, Xia Y, Han Y, Yan R, Tang H, Huang Y, Du Y, Wang X, Yao Z and Lu Q (2022) Interoception Dysfunction Contributes to the Negative Emotional Bias in Major Depressive Disorder. Front. Psychiatry 13:874859. doi: 10.3389/fpsyt.2022.874859
Received: 13 February 2022; Accepted: 08 March 2022;
Published: 11 April 2022.
Edited by:
Wenhao Jiang, Southeast University, ChinaCopyright © 2022 Zhou, Zou, Dai, Zhao, Hua, Xia, Han, Yan, Tang, Huang, Du, Wang, Yao and Lu. This is an open-access article distributed under the terms of the Creative Commons Attribution License (CC BY). The use, distribution or reproduction in other forums is permitted, provided the original author(s) and the copyright owner(s) are credited and that the original publication in this journal is cited, in accordance with accepted academic practice. No use, distribution or reproduction is permitted which does not comply with these terms.
*Correspondence: Zhijian Yao, zjyao@njmu.edu.cn; Qing Lu, luq@seu.edu.cn