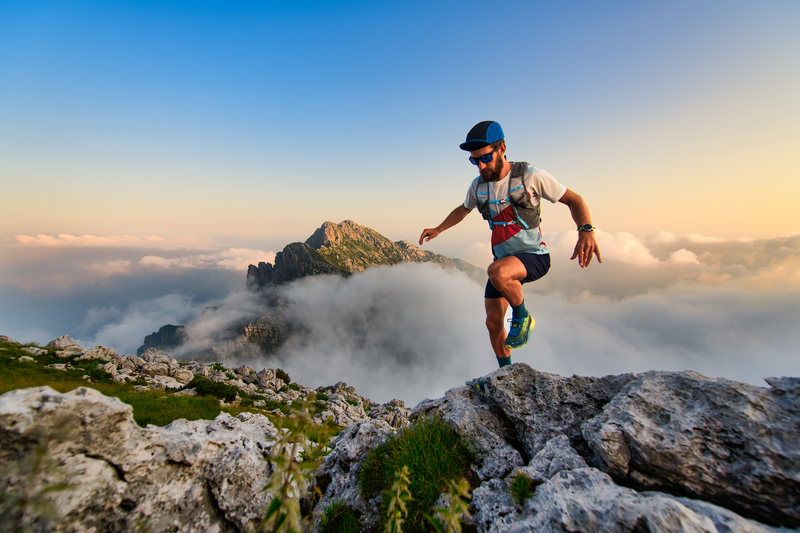
94% of researchers rate our articles as excellent or good
Learn more about the work of our research integrity team to safeguard the quality of each article we publish.
Find out more
ORIGINAL RESEARCH article
Front. Psychiatry , 06 May 2022
Sec. Neuroimaging
Volume 13 - 2022 | https://doi.org/10.3389/fpsyt.2022.852479
This article is part of the Research Topic Advances in Brain Dynamics in the Healthy and Psychiatric Disorders View all 6 articles
Obsessive-compulsive disorder (OCD) may be accompanied by an accelerated structural decline of the brain with age compared to healthy controls (HCs); however, this has yet to be proven. To answer this question, we built a brain age prediction model using mean gray matter volumes of each brain region as features, which were obtained by voxel-based morphometry derived from T1-weighted MRI scans. The prediction model was built using two Chinese Han datasets (dataset 1, N = 106 for HCs and N = 90 for patients with OCD; dataset 2, N = 270 for HCs) to evaluate its performance. Then, a new prediction model was trained using data for HCs in dataset 1 and applied to patients with OCD to investigate the brain aging trajectory. The brain-predicted age difference (brain-PAD) scores, defined as the difference between predicted brain age and chronological age, were calculated for all participants and compared between patients with matched HCs in dataset 1. It was demonstrated that the prediction model performs consistently across different datasets. Patients with OCD presented higher brain-PAD scores than matched HCs, suggesting that patients with OCD presented accelerated brain aging. In addition, brain-PAD scores were negatively correlated with the duration of illness, suggesting that brain-PAD scores might capture progressive structural brain changes. These results identified accelerated brain aging in patients with OCD for the first time and deepened our understanding of the pathogenesis of OCD.
Obsessive-compulsive disorder (OCD), a debilitating psychiatric disorder with an estimated lifetime prevalence of 1–3% (1) is characterized by uncontrollable obsessions and compulsions (2). OCD affects approximately 3% of the total population worldwide, and its symptoms impose a great burden on society (3). Despite significant advances in the study of OCD, the underlying pathogenesis remains unknown.
In clinical, OCD is highly comorbid with psychiatric diseases such as schizophrenia and bipolar disorder (4). For example, obsessive–compulsive symptoms are frequently observed in patients with schizophrenia (5). Up to 30% of patients with schizophrenia show obsessive-compulsive symptoms, and 12% of patients with schizophrenia satisfy the obsessive-compulsive diagnostic criteria for OCD (6, 7). Comorbidity is also found between OCD and depression, as well as bipolar disorder (8–10). These studies suggest that the pathogenesis of OCD overlaps with these diseases to some extent. Mental disorders are accompanied by an accelerated aging trajectory, supported by abundant evidence from functional and biological states (11–17). New evidence from recent studies combining neuroimaging and machine learning methods to calculate a so-called brain age while handling the variation in brain structures between individuals confirms this notion (18–20). Moreover, exploring abnormal brain development trajectories by means of brain age helps identify clinically indistinguishable diseases. For example, the brain age method outperforms other state-of-the-art biomarkers with an accuracy rate of 81% when identifying mild cognitive impairment (21), and the method aids early differential diagnosis between schizophrenia and bipolar disorder (18). The accelerated brain aging is also found in other brain disorders such as depression (22), epilepsy (23) autism (24) and Alzheimer's disease (25). In fact, accelerated white matter decline with age is observed in patients with OCD, suggesting that brain aging might also be accelerated in patients with OCD (26). However, whether brain aging is accelerated in OCD remains unclear.
In the current study, we explored whether the brain development trajectory was abnormal in OCD using brain gray matter volumes combined with a machine learning method for the first time. We first built a brain age prediction model where mean gray matter volumes of the brain region were treated as features using Gaussian process regression (GPR) (27). The model was trained using healthy subjects in two independent datasets (N = 130 for healthy controls (HCs) in dataset 1; N = 270 in dataset 2) and then applied to infer the brain ages of patients with OCD. The brain-predicted age difference (brain-PAD) scores, defined as the difference between predicted brain age and chronological age, were calculated in patients with OCD and then compared with age- and sex-matched HCs in dataset 1. A positive brain-PAD score reflects accelerated predicted brain aging, while a negative score represents that a predicted brain age younger than the chronological age norm. There were two assumptions in this study. First, considering the accelerated white matter decline with age observed in OCD, which suggests that brain aging is accelerated in patients with OCD (26), we expected to find higher brain-PAD scores in patients with OCD than in matched HCs. Second, we hypothesized that the brain-PAD scores in OCD were lower than those reported in schizophrenia (18, 20), due to the greater neurological abnormalities in schizophrenia compared with OCD (28–30).
Patients with OCD were recruited from the outpatient services of the Department of Psychiatry of the First Affiliated Hospital of Zhengzhou University, Zhengzhou, China. Patients were diagnosed according to the Diagnostic and Statistical Manual of Mental Disorders, Fifth Edition (DSM-V) for OCD, which was performed by two psychiatrists. The inclusion criteria were as follows: (1) first episode; (2) never having taken any antidepressants, antipsychotics, or other treatment; (3) Han Chinese ethnicity and right-handedness; (4) 18 to 50 years of age; and (5) primary school education or above. The exclusion criteria were as follows: (1) comorbidity with other mental disorders; (2) history of nervous system diseases such as brain tumor, epilepsy, or brain trauma; and (3) first-degree relatives suffering from mental illness or neurological disease. The Yale–Brown Obsessive Compulsive Scale (Y-BOCS) was used to evaluate symptom severity (31).
HCs were recruited from the community through poster advertisements. All HCs were Han Chinese and were right-handed.
The exclusion criteria for all participants (both HCs and OCD patients) were as follows: (1) use of drugs, such as anesthesia, sleeping, and analgesia, in the past month; (2) history of substance abuse; (3) history of brain tumor, trauma, surgery, or other organic body diseases; (4) history of cardiovascular disease, diabetes, or hypertension; (5) contraindications to MRI scanning, including fixed dentures, metal braces, artificial heart valves, and other metal foreign bodies in the body; and (6) other structural brain abnormalities revealed by MRI scans.
Written informed consent was obtained from all participants prior to the experiment. The study was approved by the research ethics committee of the First Affiliated Hospital of Zhengzhou University.
The second dataset comes from the Southwest University Adult Lifespan Dataset available for research purposes [(http://fcon_1000.projects.nitrc.org/indi/retro/sald.html). Dataset 2 included 492 healthy participants [306 female, 186 male; age range 19–90 years]. The exclusion criteria included MRI-related exclusion criteria, current psychiatric or neurological disorders, use of psychiatric drugs in the past 3 months prior to scanning, in addition to other criteria. A detailed description of the participant information and data acquisition parameters is provided in a previous study (32). Due to the age range for dataset 1 of 18–50 years, the current study only included participants under the age of 50 years (N = 270) in dataset 2.
T1-weighted anatomical images of participants in dataset 1 were acquired using on 3-Tsela GE Discovery MR750 scanner (General Electric, Fairfield Connecticut, USA). Using an 8-channel prototype quadrature birdcage head coil, we acquired structural T1-weighted images with 3D spoiled gradient echo scan sequence: Repetition time = 8,164 ms, echo time = 3.18 ms, inversion time = 900 ms, flip angle = 7 degrees, resolution matrix = 256 × 256, slices = 188, thickness = 1.0 mm, and voxel size = 1 × 1 × 1 mm3).
High-resolution T1-weighted anatomical images of participants in dataset 2 were acquired using a magnetization-prepared rapid gradient echo (MPRAGE) sequence (repetition time = 1,900 ms, echo time = 2.52 ms, inversion time = 900 ms, flip angle = 90 degrees, resolution matrix = 256 × 256, slices = 176, thickness = 1.0 mm, and voxel size = 1 × 1 × 1 mm3) (32).
To obtain the structural volumes of gray matter, voxel-based morphometry was adopted following the standard pipeline of the CAT12 toolbox (http://dbm.neuro.uni-jena.de/cat12/). The main steps included bias-field correction, segmentation (gray and white matter and cerebrospinal fluid), adjustment for partial volume effects, normalization into the Montreal Neurological Institute space, resampling to 1.5 mm × 1.5 mm × 1.5 mm, and non-linear modulation (33). Finally, the gray matter maps were smoothed with a 6 mm full width at half maximum Gaussian kernel. The total intracranial volume (TIV) of each participant was also calculated for comparative analysis, and its effect on brain-PAD score was investigated.
As done in previous study (27), GPR was employed to infer the brain age of each participant, where the mean gray matter volumes of brain regions were defined in 246 regions of interest of the Brainnetome atlas (34). The GPR used in the current study was implemented in the Gaussian processes for machine learning (GPML) toolbox (www.gaussianprocess.org/gpml/code/). The model parameters were optimized using a conjugate gradient optimizer (also included in the GPML toolbox), as previously described (35, 36). To exclude the influence of atlas selection on our results, an atlas with 200 regions of interest was chosen to validate the performance of the prediction model (37).
Ten-fold cross-validation (CV) was used to evaluate the performance of the prediction model (23, 38). To evaluate the performance of the prediction model, we calculated the (1) mean absolute error (MAE) between the estimated brain age and chronological age, and (2) the correlation between the chronological age and estimated brain age across 10-fold CV. To eliminate the effect of randomization on our results, this procedure was repeated 100 times. In this procedure, two datasets were used to evaluate the performance of the prediction model.
After confirming the performance of the brain age prediction model, a new prediction model was built with HCs in dataset 1 and applied to patients with OCD. The brain-PAD scores were compared between patients with OCD and HCs in dataset 1 using a two-tailed two-sample t-test. We also investigated whether there was a sex-related difference in the brain-PAD scores of patients with OCD by comparing the brain-PAD scores of female patients with those of male patients. Sex (not in sex difference), age, age2 (maybe also include TIV) and educational level were used as covariates in statistical models (19).
To explore the association between abnormal brain development and clinical symptoms in patients with OCD, Pearson's correlation coefficient was calculated for brain-PAD scores and symptoms, including duration of the disease and Y-BOCS scores.
To exclude the effect of atlas selection on our results, we validated all results (unless otherwise stated) with an atlas of 200 regions of interest (37).
The demographic information for the dataset used in this study is included in Table 1. There was no significant difference between patients with OCD and HCs in terms of sociodemographic characteristics, such as age and sex. While, patients exhibited lower education level than HCs.
The built prediction model performed consistently with different atlas selections (Figure 1). The correlation between the chronological age and estimated brain age was 0.760 (MAE = 5.049) for the 246 atlas (R = 0.715; MAE = 5.360 for 200 atlas). Then, we observed significant correlation between brain-PAD scores and age (r = −0.655, p <0.001) and age2(r = −0.653, p <0.001). There was no significant correlation between TIV and brain-PAD score (r = −0.066, p = 0.200) (Figure 2).
To explore whether the brain development trajectory was abnormal in patients with OCD, we compared brain-PAD scores between patients with OCD and HCs. According to results above, we included age, age2 and educational level as covariates in this procedure. Patients with OCD had higher brain-PAD scores than HCs (+ 0.826 years, t = 2.266, p = 0.025, Cohen's d = 0.325), suggestive of accelerated maturation of the brain in patients with OCD (Figure 3). To eliminate the effect of atlas selection, we validated this result by using a different atlas (+ 0.847 years, t = 2.037, p = 0.043, Cohen's d = 0.292 for 200 atlas). In addition, brain-PAD score did not differ between male and female patients with OCD.
We further divided patients into two subgroups (subgroup 1 with age <=25 and subgroup 2 with age > 25) considering that the human brains reached a full maturation at the age of 25 (39). The positive brain-PAD scores in subgroup 1 suggested accelerated brain maturation while that in subgroup 2 suggested accelerated brain ageing. As a result, subgroup 1 exhibited significant larger brain-PAD scores than HCs (t = 2.800, p = 0.006, Cohen's d = 0.4411). However, there was no significant difference in terms of brain-PAD scores between subgroup 2 and HCs (t = 0.346, p = 0.730, Cohen's d = 0.077).
There was a significant negative correlation between brain-PAD scores and the duration of illness in patients with OCD. This result was validated using a different brain atlas (Figure 4). We did not observe significant correlation between Y-BOCS scores and brain-PAD scores (r = 0.045, p = 0.673)
Figure 4. The correlation between brain-PAD scores and illness duration (month) in patients with OCD.
In this study, we investigated whether brain development trajectory was abnormal in patients with OCD by adopting a brain age prediction model where the gray matter volumes of brain regions were used as features for the first time. We found that patients with OCD presented with higher brain-PAD scores than matched HCs, which suggests that there is accelerated maturation of the brain in these patients. In addition, the brain-PAD scores were negatively correlated with the duration of illness in patients with OCD but not with Y-BOCS scores. Combined with the finding that there was no significant difference between the sexes, these results suggest that accelerated maturation of the brain occurs at the beginning of the disease process and might be a trait-related aberrance of OCD. Overall, we observed an accelerated brain development in patients with OCD, a finding that could help us better understand the pathogenesis of OCD.
As we hypothesized, the brain-PAD scores in patients with OCD were higher than those in HCs (+ 0.826 years, t = 2.266, p = 0.025, Cohen's d = 0.325 for the 246 atlas), suggestive of accelerated predicted brain aging in patients with OCD. Major psychiatric diseases have been found to be associated with accelerated brain aging, including schizophrenia, depression, borderline personality disorder, and bipolar disorder (18–20). OCD was found to be highly comorbid with diseases such as schizophrenia, bipolar disorder, and depression (4, 5, 7–10, 40) suggestive of overlap in the pathogenesis of OCD with the pathogenesis of these diseases. Mental disorders are accompanied by accelerated aging trajectories, as supported by a large body of evidence (11–17) and confirmed by recent studies using the brain age method (18–20). However, whether OCD presents with a similar abnormal brain development trajectory had yet to be established. Our results show that OCD is associated with accelerated brain aging for the first time. In addition, although different mental disorders are associated with accelerated brain aging, the extent of acceleration has been shown to vary across different mental diseases [e.g., + 2.64 years for early-stage schizophrenia (18), + 1.08 years for depression (19)] and across stages of illness [e.g., + 1.7 years for participants at clinical risk for schizophrenia (20), + 3.36 (41) or 5.5 years for established illness (20)]. Considering the fact that the human brains reached a full maturation at the age of 25 (39), the meaning of positive brain-PAD scores was not different in older patients with age larger than 25. We further divided patients into subgroups according to age (subgroup1 with age <=25 and subgroup 2 with age >25). Subgroup 1 exhibited larger brain-PAD scores than HCs while not subgroup 2. Overall, the finding that OCD is associated with abnormal brain development deepens our understanding of the pathogenesis of OCD.
The duration of illness was found to be negatively correlated with brain-PAD scores in patients with OCD, which suggests that brain-PAD scores might capture progressive structural brain changes. Mental disorders, such as schizophrenia (42, 43), generalized anxiety disorder (44) and depression, (45) were found to be neuroprogressive illnesses accompanied by progressive structural brain changes throughout the disease course. The negative correlation hinted that higher brain age was a stage-dependent phenomenon in OCD. This phenomenon could mirror the transition from a clinically unstable period, with large variability in functioning, to a relatively stable period, when patients have reached a plateau in functioning (46, 47). The same phenomenon was also found in depression (27). Another possible explanation was the compensation mechanism considering that increased gray matter volume was also reported in OCD (27, 48). However, this correlation should be considered carefully, as there was limited number of patients with illness duration larger than 100 months. Further studies could test this hypothesis. In addition, the fact that there was no significant correlation between brain-PAD scores and Y-BOCS scores, as well as no significant difference between female and male patients, hinted that accelerated brain aging was independent of the severity of symptoms in OCD.
The findings of this study should be considered in light of some limitations. First, nicotine and alcohol use were not controlled for in the current study and they might also affect the brain-PAD scores (49). Future studies should take into account these factors. Second, our results were based on a single dataset; an external validation dataset was needed to confirm these results. Third, the negative correlation between duration of illness and brain-PAD scores suggested that the accelerated brain age might be more pronounced since the beginning of illness, and future studies could investigate this hypothesis. Forth, the educational level of patients and controls was not matched for two groups, and future studies could examine the association between education level and brain age. Fifth, as the first attempt to investigate abnormal brain age in OCD, we only used gray matter volume as features for its interpretability when constructing prediction model. However, future studies should take into account additional features including white matter volume, structural/functional connectome. Finally, adopting deep learning method would boost the performance of the prediction model (25, 50), future studies should use large dataset combined deep learning method if possible.
To our knowledge, this is the first study to explore abnormal brain age in patients with OCD. We found that patients with OCD had higher brain-PAD scores than HCs, suggestive of accelerated brain development in patients with OCD. Moreover, an atypical brain development trajectory might be related to the progression of OCD and is independent of the severity of symptoms. These results suggest for the first time that there is accelerated brain aging in patients with OCD.
The raw data supporting the conclusions of this article will be made available by the authors, without undue reservation.
The studies involving human participants were reviewed and approved by the Ethics Committee of the First Affiliated Hospital of Zhengzhou University. The patients/participants provided their written informed consent to participate in this study.
LL, SH, and JC designed the study. LY, BW, and XZ analyzed the data. LL, JC, and YZ drafted the work. All authors contributed to the article and approved the submitted version.
This research study was supported by the Natural Science Foundation of China (81601467, 81871327, and 62106229), Medical science and technology research project of Henan province (201701011, SBGJ202102103, and SBGJ202101013) and The First Affiliated Hospital of Zhengzhou University (Grant No: YNQN2017160).
The authors declare that the research was conducted in the absence of any commercial or financial relationships that could be construed as a potential conflict of interest.
All claims expressed in this article are solely those of the authors and do not necessarily represent those of their affiliated organizations, or those of the publisher, the editors and the reviewers. Any product that may be evaluated in this article, or claim that may be made by its manufacturer, is not guaranteed or endorsed by the publisher.
The authors thank all subjects who participate in this study.
1. Ruscio AM, Stein DJ, Chiu WT, Kessler RC. The epidemiology of obsessive-compulsive disorder in the national comorbidity survey replication. Mol Psychiatry. (2010) 15:53–63. doi: 10.1038/mp.2008.94
2. Cyr M, Pagliaccio D, Yanes-Lukin P, Fontaine M, Rynn MA, Marsh R. Altered network connectivity predicts response to cognitive-behavioral therapy in pediatric obsessive-compulsive disorder. Neuropsychopharmacology. (2020) 45:1232–40. doi: 10.1038/s41386-020-0613-3
3. Hou JM, Zhao M, Zhang W, Song LH, Wu WJ, Wang J, et al. Resting-state functional connectivity abnormalities in patients with obsessive-compulsive disorder and their healthy first-degree relatives. J Psychiatry Neurosci. (2014) 39:304–11. doi: 10.1503/jpn.130220
4. Sharma LP, Reddy YCJ. Obsessive-compulsive disorder comorbid with schizophrenia and bipolar disorder. Indian J Psychiatry. (2019) 61(Suppl. 1):S140–8. doi: 10.4103/psychiatry.IndianJPsychiatry_527_18
5. Sterk B, Lankreijer K, Linszen DH, de Haan L. Obsessive-compulsive symptoms in first episode psychosis and in subjects at ultra high risk for developing psychosis; onset and relationship to psychotic symptoms. Aust N Z J Psychiatry. (2011) 45:400–6. doi: 10.3109/00048674.2010.533363
6. de Haan L, Sterk B, Wouters L, Linszen DH. The 5-year course of obsessive-compulsive symptoms and obsessive-compulsive disorder in first-episode schizophrenia and related disorders. Schizophr Bull. (2013) 39:151–60. doi: 10.1093/schbul/sbr077
7. Swets M, Dekker J, van Emmerik-van Oortmerssen K, Smid GE, Smit F, de Haan L, et al. The obsessive compulsive spectrum in schizophrenia, a meta-analysis and meta-regression exploring prevalence rates. Schizophr Res. (2014) 152:458–68. doi: 10.1016/j.schres.2013.10.033
8. Mucci F, Toni C, Favaretto E, Vannucchi G, Marazziti D, Perugi G. Obsessive-compulsive disorder with comorbid bipolar disorders: clinical features and treatment implications. Curr Med Chem. (2018) 25:5722–30. doi: 10.2174/0929867324666171108145127
9. Ferentinos P, A Preti, AA Veroniki, KG Pitsalidis, AT Theofilidis, A Antoniou, et al. Comorbidity of obsessive-compulsive disorder in bipolar spectrum disorders: systematic review and meta-analysis of its prevalence. J Affect Disord. (2020) 263:193–208. doi: 10.1016/j.jad.2019.11.136
10. McNally RJ, Mair P, Mugno BL, Riemann BC. Co-morbid obsessive-compulsive disorder and depression: a Bayesian network approach. Psychol Med. (2017) 47:1204–14. doi: 10.1017/S0033291716003287
11. Lever-van Milligen BA, Lamers F, Smit JH, Penninx BW. Six-year trajectory of objective physical function in persons with depressive and anxiety disorders. Depress Anxiety. (2017) 34:188–97. doi: 10.1002/da.22557
12. Han LKM, Aghajani M, Clark SL, Chan RF, Hattab MW, Shabalin AA, et al. Epigenetic aging in major depressive disorder. Am J Psychiatry. (2018) 175:774–82. doi: 10.1176/appi.ajp.2018.17060595
13. Darrow SM, Verhoeven JE, Révész D, Lindqvist D, Penninx BW, Delucchi KL, et al. The association between psychiatric disorders and telomere length: a meta-analysis involving 14,827 persons. Psychosom Med. (2016) 78:776–87. doi: 10.1097/PSY.0000000000000356
14. Lindqvist D, Wolkowitz OM, Picard M, Ohlsson L, Bersani FS, Fernström J, et al. Circulating cell-free mitochondrial DNA, but not leukocyte mitochondrial DNA copy number, is elevated in major depressive disorder. Neuropsychopharmacology. (2018) 43:1557–64. doi: 10.1038/s41386-017-0001-9
15. Nguyen TT, Eyler LT, Jeste DV. Systemic biomarkers of accelerated aging in schizophrenia: a critical review and future directions. Schizophr Bull. (2018) 44:398–408. doi: 10.1093/schbul/sbx069
16. Islam F, Mulsant BH, Voineskos AN, Rajji TK. Brain-Derived neurotrophic factor expression in individuals with schizophrenia and healthy aging: testing the accelerated aging hypothesis of schizophrenia. Curr Psychiatry Rep. (2017) 19:36. doi: 10.1007/s11920-017-0794-6
17. Okusaga OO. Accelerated aging in schizophrenia patients: the potential role of oxidative stress. Aging Dis. (2014) 5:256–62. doi: 10.14336/AD.2014.0500256
18. Hajek T, Franke K, Kolenic M, Capkova J, Matejka M, Propper L. Brain age in early stages of bipolar disorders or schizophrenia. Schizophr Bull. (2019) 45:190–8. doi: 10.1093/schbul/sbx172
19. Han LKM, Dinga R, Hahn T, Ching CRK, Eyler LT. Brain aging in major depressive disorder: results from the ENIGMA major depressive disorder working group (2020).
20. Koutsouleris N, Davatzikos C, Borgwardt S, Gaser C, Bottlender R, Frodl T. Accelerated brain aging in schizophrenia and beyond: a neuroanatomical marker of psychiatric disorders. Schizophr Bull. (2014) 40:1140–53. doi: 10.1093/schbul/sbt142
21. Gaser C, Franke K, Klöppel S, Koutsouleris N, Sauer H. BrainAGE in mild cognitive impaired patients: predicting the conversion to Alzheimer's disease. PLoS ONE. (2013) 8:e67346. doi: 10.1371/journal.pone.0067346
22. Han S, Chen Y, Zheng R, Li S, Jiang Y, Wang C, et al. The stage-specifically accelerated brain aging in never-treated first-episode patients with depression. Human Brain Map. (2021) 42:3656–66.
23. Sone D, Beheshti I, Maikusa N, Ota M, Kimura Y, Sato N, et al. Neuroimaging-based brain-age prediction in diverse forms of epilepsy: a signature of psychosis and beyond. Mol Psychiatry. (2021) 26:825–34. doi: 10.1038/s41380-019-0446-9
24. He C, Chen H, Uddin LQ, Erramuzpe A, Bonifazi P, Guo X, et al. Structure-Function connectomics reveals aberrant developmental trajectory occurring at preadolescence in the autistic brain. Cereb Cortex. (2020) 30:5028–37. doi: 10.1093/cercor/bhaa098
25. Qiu S, Joshi PS, Miller MI, Xue C, Zhou X, Karjadi C, et al. Development and validation of an interpretable deep learning framework for Alzheimer's disease classification. Brain. (2020) 143:1920–33. doi: 10.1093/brain/awaa137
26. Hawco C, Voineskos AN, Radhu N, Rotenberg D, Ameis S, Backhouse FA, et al. Age and gender interactions in white matter of schizophrenia and obsessive compulsive disorder compared to non-psychiatric controls: commonalities across disorders. Brain Imaging Behav. (2017) 11:1836–48. doi: 10.1007/s11682-016-9657-8
27. Han S, Chen Y, Zheng R, Li S, Jiang Y, Wang C, et al. The stage-specifically accelerated brain aging in never-treated first-episode patients with depression. Hum Brain Mapp. (2021) 42:3656–66. doi: 10.1002/hbm.25460
28. Riffkin J, Yücel M, Maruff P, Wood SJ, Soulsby B, Olver J, et al. A manual and automated MRI study of anterior cingulate and orbito-frontal cortices, and caudate nucleus in obsessive-compulsive disorder: comparison with healthy controls and patients with schizophrenia. Psychiatry Res. (2005) 138:99–113. doi: 10.1016/j.pscychresns.2004.11.007
29. Ha TH, Yoon U, Lee KJ, Shin YW, Lee JM, Kim IY, et al. Fractal dimension of cerebral cortical surface in schizophrenia and obsessive-compulsive disorder. Neurosci Lett. (2005) 384:172–6. doi: 10.1016/j.neulet.2005.04.078
30. Kim JJ, Youn T, Lee JM, Kim IY, Kim SI, Kwon JS. Morphometric abnormality of the insula in schizophrenia: a comparison with obsessive-compulsive disorder and normal control using MRI. Schizophr Res. (2003) 60:191–8. doi: 10.1016/S0920-9964(02)00306-7
31. Goodman WK, Price LH, Rasmussen SA, Mazure C, Fleischmann RL, Hill CL, et al. The yale-brown obsessive compulsive scale. I. development, use, and reliability. Arch Gen Psychiatry. (1989) 46:1006–11. doi: 10.1001/archpsyc.1989.01810110048007
32. Wei D, Zhuang K, Ai L, Chen Q, Yang W, Liu W, et al. Structural and functional brain scans from the cross-sectional Southwest university adult lifespan dataset. Sci Data. (2018) 5:180134. doi: 10.1038/sdata.2018.134
33. Ashburner J. Computational anatomy with the SPM software. Magn Reson Imaging. (2009) 27:1163–74. doi: 10.1016/j.mri.2009.01.006
34. Fan L, Li H, Zhuo J, Zhang Y, Wang J, Chen L, et al. The human brainnetome atlas: a new brain atlas based on connectional architecture. Cereb Cortex. (2016) 26:3508–26. doi: 10.1093/cercor/bhw157
36. Marquand AF, Rezek I, Buitelaar J, Beckmann CF. Understanding heterogeneity in clinical cohorts using normative models: beyond case-control studies. Biol Psychiatry. (2016) 80:552–61. doi: 10.1016/j.biopsych.2015.12.023
37. Craddock RC, James GA, Holtzheimer PE 3rd, Hu XP, Mayberg HS. A whole brain fMRI atlas generated via spatially constrained spectral clustering. Hum Brain Mapp. (2012) 33:1914–28. doi: 10.1002/hbm.21333
38. Ziegel, Eric R. The elements of statistical learning. Technometrics. (2010) 45:267–8. doi: 10.1198/tech.2003.s770
39. Arain M, Haque M, Johal L, Mathur P, Nel W, Rais A, et al. Maturation of the adolescent brain. Neuropsychiatr Dis Treat. (2013) 9:449–61. doi: 10.2147/NDT.S39776
40. Achim AM, Maziade M, Raymond E, Olivier D, Mérette C, Roy MA. How prevalent are anxiety disorders in schizophrenia? a meta-analysis and critical review on a significant association. Schizophr Bull. (2011) 37:811–21. doi: 10.1093/schbul/sbp148
41. Schnack HG, van Haren NE, Nieuwenhuis M, Hulshoff Pol HE, Cahn W, Kahn RS. Accelerated brain aging in schizophrenia: a longitudinal pattern recognition study. Am J Psychiatry. (2016) 173:607–16. doi: 10.1176/appi.ajp.2015.15070922
42. Olabi B, Ellison-Wright I, McIntosh AM, Wood SJ, Bullmore E, Lawrie SM. Are there progressive brain changes in schizophrenia? a meta-analysis of structural magnetic resonance imaging studies. Biol Psychiatry. (2011) 70:88–96. doi: 10.1016/j.biopsych.2011.01.032
43. Jiang Y, Luo C, Li X, Duan M, He H, Chen X, et al. Progressive reduction in gray matter in patients with schizophrenia assessed with MR imaging by using causal network analysis. Radiology. (2018) 287:633–42. doi: 10.1148/radiol.2017171832
44. Chen Y, Cui Q, Fan YS, Guo X, Tang Q, Sheng W, et al. Progressive brain structural alterations assessed via causal analysis in patients with generalized anxiety disorder. Neuropsychopharmacology. (2020) 45:1689–97. doi: 10.1038/s41386-020-0704-1
45. Moylan S, M Maes, Wray NR, Berk M. The neuroprogressive nature of major depressive disorder: pathways to disease evolution and resistance, and therapeutic implications. Mol Psychiatry. (2013) 18:595–606. doi: 10.1038/mp.2012.33
46. Davidson L, McGlashan TH. The varied outcomes of schizophrenia. Can J Psychiatry. (1997) 42:34–43. doi: 10.1177/070674379704200105
47. van Haren NE, Cahn W, Hulshoff Pol HE, Schnack HG, Caspers E, Lemstra A, et al. Brain volumes as predictor of outcome in recent-onset schizophrenia: a multi-center MRI study. Schizophr Res. (2003) 64:41–52. doi: 10.1016/S0920-9964(03)00018-5
48. Piras F, Piras F, Abe Y. White matter microstructure and its relation to clinical features of obsessive-compulsive disorder: findings from the ENIGMA OCD working group. Transl Psychiatry. (2021) 11:173. doi: 10.1038/s41398-021-01276-z
49. Franke K, Gaser C, Manor B, Novak V. Advanced brainAGE in older adults with type 2 diabetes mellitus. Front Aging Neurosci. (2013) 5:90. doi: 10.3389/fnagi.2013.00090
Keywords: obsessive-compulsive disorder, brain age, structural brain imaging, machine learning, gray matter volume (GMV)
Citation: Liu L, Liu J, Yang L, Wen B, Zhang X, Cheng J, Han S, Zhang Y and Cheng J (2022) Accelerated Brain Aging in Patients With Obsessive-Compulsive Disorder. Front. Psychiatry 13:852479. doi: 10.3389/fpsyt.2022.852479
Received: 13 January 2022; Accepted: 28 March 2022;
Published: 06 May 2022.
Edited by:
Bartosz Zurowski, University Medical Center Schleswig-Holstein, GermanyReviewed by:
Sean Hatton, University of California, San Diego, United StatesCopyright © 2022 Liu, Liu, Yang, Wen, Zhang, Cheng, Han, Zhang and Cheng. This is an open-access article distributed under the terms of the Creative Commons Attribution License (CC BY). The use, distribution or reproduction in other forums is permitted, provided the original author(s) and the copyright owner(s) are credited and that the original publication in this journal is cited, in accordance with accepted academic practice. No use, distribution or reproduction is permitted which does not comply with these terms.
*Correspondence: Shaoqiang Han, U2hhb3FpYW5nSGFuQDE2My5jb20=; Yong Zhang, enp1emhhbmd5b25nMjAxM0AxNjMuY29t; Jingliang Cheng, ZmNjY2hlbmdqbEB6enUuZWR1LmNu; Baohong Wen, d2VuYmFvaG9uZzIwMTJAMTYzLmNvbQ==
†These authors have contributed equally to this work
Disclaimer: All claims expressed in this article are solely those of the authors and do not necessarily represent those of their affiliated organizations, or those of the publisher, the editors and the reviewers. Any product that may be evaluated in this article or claim that may be made by its manufacturer is not guaranteed or endorsed by the publisher.
Research integrity at Frontiers
Learn more about the work of our research integrity team to safeguard the quality of each article we publish.