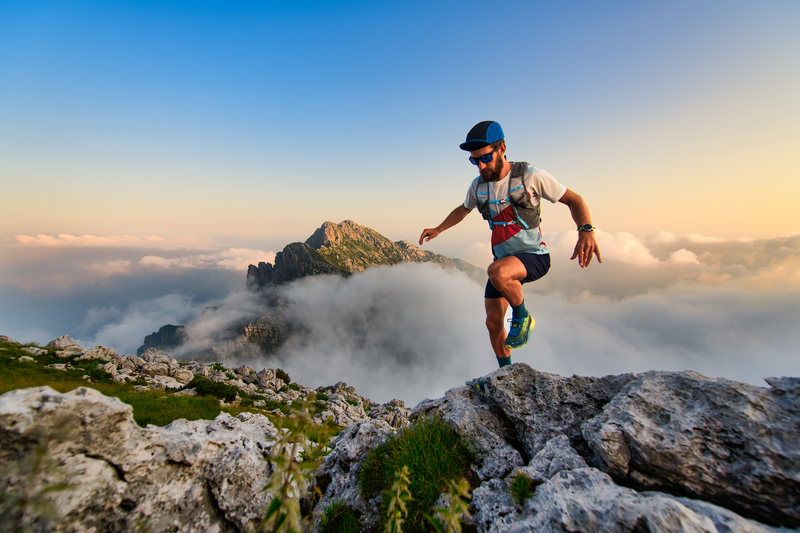
94% of researchers rate our articles as excellent or good
Learn more about the work of our research integrity team to safeguard the quality of each article we publish.
Find out more
ORIGINAL RESEARCH article
Front. Psychiatry , 24 March 2022
Sec. Addictive Disorders
Volume 13 - 2022 | https://doi.org/10.3389/fpsyt.2022.848157
This article is part of the Research Topic Community Series in Neurobiological Biomarkers for Developing Novel Treatments of Substance and Non-Substance Addiction, volume II View all 16 articles
Background: Gaming disorder (GD) has been recognized as an official diagnostic entity in the latest revision of the International Classification of Diseases (ICD-11). However, the majority of previous studies used different instruments, which are not fully consistent with the concept of GD in ICD-11. The development of a screening assessment instrument based on ICD-11 for this new disease entity is very urgent and important.
Methods: The ICD-11 Gaming Disorder Symptom Questionnaire (GDSQ), based on the ICD-11 diagnostic guidelines for GD, was developed by a team of GD experts. A total of 7,790 adolescents were included in this study. Criterion validity was assessed by GDSQ, Video Gaming Dependency Scale (VGDS), weekly game playing time, weekly game video viewing time, and monthly money spent on games. Item structure was measured by factorial analysis. Discrimination between GD and non-GD was examined based on the receiver characteristic curve (ROC).
Results: The GDSQ was very well described by three symptoms of GD (i.e., impaired control, increasing priority to gaming, and continued use despite the occurrence of negative consequences). The internal consistency was excellent (Cronbach's α = 0.964) with good criterion validity and good discriminatory power. The optimal cutoff point for determining the profile of gamers was found to be ≥62 points. The GDSQ revealed that the prevalence of GD was 2.27% in this adolescent sample.
Conclusion: The ICD-11–based GDSQ is a successfully validated measurement scale for GD among adolescents. This study provides a new tool (GDSQ) for us to effectively identify individuals with risk of GD in medical and non-medical settings.
Gaming disorder (GD) has become a significant public health concern. According to market analysis, there were 2.8 billion online game players worldwide and the global gaming market generated $175.8 billion in revenue in 2021 (1). There were 509 million online game players in China until June 2021 (2). With the increasing population of game users, the psychological and physical harm due to excessive gaming behaviors has caused concerns in psychiatry, public health, education, and administration (3, 4). Until now, the mainstream view considers excessive and uncontrollable gaming behavior as an addictive disorder (namely, GD). Adolescents are particularly vulnerable to GD (5, 6) and often experience a series of negative consequences, including low self-esteem, intense negative mood states (e.g., sadness, irritability, and boredom) (7), relationship conflicts, and problems at work or school (8–11).
Plenty of studies have been conducted to assess the prevalence of GD and gaming related problems. However, the lack of a unified instrument among these studies resulted in widespread inconsistency in the estimation of the prevalence rates. For example, studies revealed the prevalence of GD ranged from 3.5 to 17% in China (12) and from 0.3 to 4.9% in the United States (13). Therefore, it is indispensable to establish a set of more effective diagnostic criteria and screening tools for GD.
In 2013, the Diagnostic and Statistical Manual of Mental Disorders (DSM-5) included Internet Gaming Disorder (IGD) in the “Conditions for Further Study” section and proposed a set of tentative diagnostic criteria for IGD (14): indicated by five or more of the nine items (preoccupation, withdrawal, tolerance, unsuccessful attempts to control, loss of life interests, continuation despite problems, deception, escape, and jeopardizing important life aspects) for 12 months (15). It encouraged researchers to utilize the same standard to recognize people with IGD and develop screening instruments.
Nonetheless, the proposed diagnostic criteria for IGD in DSM-5 have some limitations. First, it is questionable whether certain criteria (e.g., preoccupation, tolerance, withdrawal response, and deception) have sufficient sensitivity and specificity or not (16–20). Second, all diagnostic criteria for IGD are equally weighted when counting how many of them are met, but this approach is flawed because it fails to distinguish well between core and non-core symptoms (21). Besides, the consensus about the diagnostic cutoff value for IGD (five of nine items) seems not established. By diagnosing IGD with the Nine-Item Internet Gaming Disorder Scale-Short Form (IGDS9-SF) test, the cutoff point score of 36 in Pontes' study is higher than the optimal cutoff point of 32 in Qin's study (with a sample of 3,742 from Chinese universities and 131 from Chinese clinical settings) (22, 23).
The 11th revision of the International Classification of Diseases (ICD-11) included GD as a disease entity in 2019. The WHO Working Group has proposed the diagnostic guidelines for GD (24, 25), which define the core symptoms of GD as (1) impaired control over gaming behavior (e.g., onset, frequency, intensity, duration, termination, and context); (2) increasing priority to gaming over other life interests and daily activities; and (3) continuation or escalation of gaming activities despite the occurrence of negative consequences. Because of the short history of ICD-11, until now, few research studies have been conducted based on these criteria. Some findings suggest that the ICD criteria appeared to be more stringent than the DSM criteria in diagnosing GD (26, 27). For instance, Ko et al. analyzed the diagnostic validity and utility of IGD (DSM-5) and GD (ICD-11) through the empirical data, psychiatrists conducted diagnostic interviews with 69 subjects with IGD based on the DSM-5 IGD criteria, and only 44 participants with IGD (63.8%) fulfilled the criteria for GD (26).
Up until now, only a few studies have been involved in the development of standard questionnaires for the assessment of GD based on ICD-11 criteria (28, 29). Therefore, the current study aimed to develop a screening self-assessment instrument named the Gaming Disorder Symptom Questionnaire (GDSQ) to assess GD among adolescents based on ICD-11 criteria. First, we developed the GDSQ with the GD symptoms listed in ICD-11, and we borrowed the setting style of response options from the classic screening tool Alcohol Use Disorders Identification Test (30) to promote the understandability and usability of GDSQ. Then, we validated GDSQ with a large sample of Chinese adolescents aged 12–18 years. Given that all items were based on ICD-11 criteria and developed by psychiatrists and clinical psychologists with expertise in behavioral addiction, we hypothesized that the GDSQ would be a valid and reliable screening tool to assess GD.
A stratified cluster random sampling method was used to select three urban areas in Xinjiang Uygur Autonomous Region, China. These cities included Urumqi, Kashi, and Bole. In each area, two junior middle school and two senior middle school and, in each school, four classes were selected from each grade (grade 1–3 of junior middle school and grades 1–3 of senior middle school). The classes were randomly selected in each school. The students involved in the survey were aged 12–18 years old. The survey was carried out in the classrooms of the recruited classes. After the explanation of the purpose and requirements of the study, the researchers emphasized the voluntariness of the survey and then delivered the informed consent form to the parents of each adolescent. Electronic informed consent was obtained from each student after parental informed consent had been obtained. After that, the questionnaires were distributed to the students who participated in the survey.
The data in the current survey are only a part of a big set of studies that contained multiple questionnaires that need about 30 min to complete. During the filling process, the researchers answered promptly subjects' questions about the survey. The period of the data collection spanned from October 2020 to November 2021. Next, two psychiatrists assessed whether the subjects were in a high-risk GD group based on factors such as impaired control over gaming behavior, the priority of gaming over other interests and daily activities, and the continuation or escalation of gaming activities despite negative consequences.
Informed consent was obtained from all target participants and their parents or legal guardians. The ethical approval for this study was obtained from the Ethics Committee of the Second Xiangya Hospital of Center South University (ID: 2019-S454).
Socio-demographics information included age, gender, race, and family structure (being an only child or having siblings). Additional questions about gaming habits included starting age for gameplay, devices used to play games, preferred games, hours of game-related per week (online games, stand-alone games, and game video watching), and the amount of money spent on games per month over the past 12 months.
In the current study, the VGDS was used to assist in the development and psychometric validation process of the GDSQ as a concurrent measure of GD. IGD was assessed using the Chinese version of the VGDS (see Supplementary Table 1), which is abbreviated as CSAS in the German version “Computerspielabhängigkeitsskala” and was adapted by Rehbein et al. (31) from a previous instrument (KFN-CSAS-II). This instrument is the 18–descriptive item scale, with every two items representing one of the nine DSM-5 IGD criteria. Each item was rated on a four-point Likert scale (1 = strongly disagree, 2 = somewhat disagree, 3 = somewhat agree, and 4 = strongly agree) to evaluate the symptom severity of the subject's gaming behavior within the last 12 months. According to the DSM-5 recommendations for IGD, a criterion was endorsed if at least one of the two items was answered with “strongly agree”. The subjects who endorsed five or more of the nine symptom criteria were considered for IGD. The VGDS was validated in Chinese adolescents and young adults (32). In this study, the VGDS presented an excellent internal consistency of Cronbach's α with 0.968.
The GDSQ was developed based on the diagnostic guidelines of ICD-11 GD. Initially, we had 24 items (see Table 1) after consulting with an expert panel to ensure content validity. The panel meeting was composed of psychiatrists and clinical psychologists with expertise in behavioral addiction. Every eight of the 24 items embodied one of the three symptoms of GD (i.e., impaired control, increasing priority to gaming, and continuing playing games despite the negative consequences). The subjects were asked to respond about the frequency of the event or situation described in the items within the last 12 months on a five-point Likert scale (0 = never, 1 = less than monthly, 2 = monthly, 3 = weekly, and 4 = almost daily). For example, “Once I start playing the game, it is hard to stop” was one item in the dimension of impaired control. The Chinese version of GDSQ is shown in Supplementary Table 2.
It should be noted that each of the items reflecting “continuing playing games despite the negative consequences” had two-round responses. The first question was asked about the frequency of game playing. If the subjects respond with “0” (i.e., never), then there is no need to enter the second question. However, if the response is “1” (i.e., less than monthly) or more frequent, then the subjects need to respond to the second question about whether they will continue playing games or not. The options for the second question are dichotomous (0 = no, 1 = yes). The score of the item was calculated by multiplying the point of the first question with the point of the second question. For example, the first question of one item for the dimension of “continued use despite the occurrence of negative consequences” is “Because of playing games, I don't have enough time and energy to get the right things done”, and the second question is “After the aforementioned situation, I continue to play games whenever there is a chance”.
Among all 7,901 participants, 7,790 (98.60%) adolescents answered all items of the questionnaires and included in this study. A total of 111 (1.40%) adolescents were excluded from the analysis due to missing information in GDSQ.
As the sample size for this study was sufficiently large, SPSS 25.0 was used to divide the sample into two separate data files. The first data file contained 3,871 samples and was used for exploratory factor analysis (EFA). The second data file contained 3,919 samples and was used for the confirmatory factor analysis (CFA). The full sample was used for descriptive statistical analysis and reliability estimation. Retest sample: 554 students from the first sample were retested 8 weeks apart. It was analyzed using MPLUS 8.3 for the CFA and SPSS 25.0 for Windows for the remaining analysis. A significance level of 0.05 was adopted for all statistical tests.
The chi-square (χ2) values were applied to detect the differences between the model's implied variance–covariance matrix and the observed variance–covariance matrix. The comparative fit index (CFI) was used to compare the hypothesized model with the null hypothesis (33–35). The CFI is also one of the most robust indicators (36). The Tucker–Lewis Index (TLI) is a relative goodness of fit indices. In addition to evaluating the model from the perspective of model fitting, the fitting degree of the model can also be evaluated from the size of the residual error, and then, the fitting situation of the model can be evaluated. The standardized root mean square residual (SRMR) is one of the indicators for the direct evaluation of residual error. The model fit was also assessed by the root mean square error of approximation (RMSEA). McDonald and Ho recommended that the model with a RMSEA < 0.08 as an acceptable one and < 0.05 as a good lone. Model goodness of fit was assumed according to the following criteria: RMSEA < 0.05 (35), SRMR < 0.08, TLI > 0.95, and CFI > 0.95 (36).
The GDSQ's ability to distinguish between non-disordered and disordered gamers was evaluated using a receiver characteristic curve (ROC) analysis. To achieve this goal, the GDSQ scores were compared against the standard according to ICD-11 related VGDS items. In this study, we also applied to defined 95% confidence intervals (CI). The Youden Index was calculated by sensitivity and specificity. The diagnostic efficacy of GDSQ was measured by the area under the ROC curve (AUC).
The demographic characteristics are shown in Table 2. The mean age of first gameplay was 9.48 years (SD: 2.68). With regard to the gaming platforms preference, the majority of the participants (n = 3,803, 48.8%) reported playing on smartphones. The most genre played were multiplayer online battle arena (MOBA) games (n = 2,266, 29.1%), followed by first-person shooters (FPS) and counter-strike: global offensive (CS: GO) (n = 1,231, 15.8%). The average amount of money spent on gaming was 44.92 CNY (Chinese Yuan) per month (SD: 386.51). In addition, a large amount of gamers was reported spending more than 16 h per week on playing online games (n = 441, 5.6%), stand-alone games (n = 211, 2.7%), and game video watching (n = 224, 2.8%), respectively.
An EFA using the Principal Axis Factoring extraction method with principal oblique rotation on the 24 items of the GDSQ was performed on the whole sample (n = 7,790) to examine its factorial structure and construct validity. The three principal components to be extracted were determined by the convergence of the scree plot in combination with the tendency for Kaiser's criterion. The scree plot of the GDSQ-21 by 21-factor analysis (n = 3,871) is shown in Supplementary Figure 1.
Items with factor loadings >0.45 and/or parallel loadings <0.20 were retained. After the first rotation, three items [“Even at inappropriate occasions or times, I still turn on the game and play for a while, ” “I experience some discomfort (e.g., decreased vision, dizziness, muscle stiffness, or wrist pain) after high-intensity game playing, ” and “If my time is scheduled by myself, I play games first and put other things on the back burner”] were removed due to these three items appeared to be a cross-load problem. The appropriateness for conducting the EFA was confirmed by the Kaiser–Meyer–Olkin criterion value (KMO = 0.970) used for the suitability of the date and the Bartlett's test result of sphericity (χ2 = 80,321.74, p <0.001). As shown in Table 3, component loadings for each item ranged between 0.689 (item 18) and 0.846 (item 11). The three factors that were extracted after six iterations explained 76.04% of the total variance. Moreover, the 21 items of the GDSQ were retained in the model for subsequent analyses (i.e., CFA) to ensure optimal construct validity.
Table 3. Summary of the results from the EFA on the GDSQ-21 items obtained from the sample (n = 3,871).
The goodness of fit of the unidimensional model of GDSQ in EFA was assessed using the conventional fit indices. The results showed an overall good fit to the data. The χ2 was 1,219.11 (p < 0.001) with CFI of 0.958, TLI of 0.951, RMSEA of 0.038, and SRMR of 0.039, indicating an acceptable fit. As shown in Figure 1, the CFA results showed statistically significant factors (p < 0.05) for the 21 items of the GDSQ (GDSQ-21).
Figure 1. Graphical summary of CFA results obtained from the 21 items of the GDSQ-21 (N = 3,919). GDSQ-21, Gaming Disorder Symptom Questionnaire-21; CFA, confirmatory factor analysis.
The results of the eight multiplications are summed. By summing up the responses to the 21 items, the total score was calculated to obtain a possible maximum score of 84. To further assess the validity and reliability of the GDSQ-21, the total scores obtained by participants on the VGDS and GDSQ-21 were associated (r = 0.781, p < 0.001). The GDSQ-21 was associated with self-reported weekly gaming time (online, stand-alone, and game video watching) (r = 0.619, 0.514, and 0.504, respectively, ps < 0.001). The GDSQ-21 was also associated with the monthly amount of money spent on games (Spearman's ρ = 0.338, p < 0.001). As shown in Table 4, the results obtained suggest that the GDSQ-21 is strongly positively associated with VGDS, and moderately correlated with the self-reported weekly gaming time and the monthly amount of money spent. The Spearman–Brown split-half reliability of GDSQ-21 was 0.98, and the test–retest reliability was 0.71. Cronbach's α of the GDSQ-21 was 0.964 in this analysis, which showed the scale's good internal consistency. The Cronbach's α for the three factors were 0.929, 0.950, and 0.948, respectively.
As shown in Figure 2, by the Youden Index, the optimal cutoff for the overall score was 61.5 with the curve (AUC) of 89.6% (95% CI = 86.6–92.7), sensitivity of 83.1%, and specificity of 88.8%. Factor 1 had a cutoff value of 13.5 with the curve (AUC) of 86.6% (95% CI = 83.5–90.0), specificity of 78.3%, and sensitivity of 86.7%. Factor 2 had a cutoff value of 10.5 with the curve (AUC) of 89.2% (95% CI = 86.3–92.1), specificity of 78.7%, and sensitivity of 89.3%. Factor 3 had a cutoff value of 3.5 with the curve (AUC) of 86.0% (95% CI = 82.6–89.4), specificity of 77.3%, and sensitivity of 89.4%. Finally, the cutoff ≥ 14 is applied for factor 1, ≥11 for factor 2, ≥4 for factor 3, and ≥62 for the whole scale. Participants who met each dimension and total score were classified with GD. The prevalence of GD was estimated at 2.27% in the period of the past 12 months. As shown in Table 5, there was a significant difference between GD and No-GD adolescents. For instance, the GD adolescents reported more weekly gaming time than the No-GD adolescents.
Figure 2. Area under the ROC curve of the GDSQ-21 for diagnosis (AUC, 0.896). ROC, receiver operating characteristic; AUC, area under the ROC curve.
To the best of our knowledge, this study is the first study to introduce a screening tool for assessing the ICD-11 diagnostic guidelines for GD in adolescents in China. GDSQ-21 was successfully validated in a sample of adolescents as an assessment tool with excellent internal consistency and criterion validity. The instrument covers the three symptoms for IGD/GD, and the concrete manifestations of these symptoms are referred to in detail (i.e., impaired control, increasing priority to gaming, and continued use despite the occurrence of negative consequences) and functional impairment. Thus, in addition to exhibiting psychometrically robust properties, it is an easy-to-use screening instrument that can be used by medical and non-medical institutions to distinguish non-disordered and disordered gamers.
In respect of the test reliability and validity, the GDSQ-21 appears to be a reliable and valid measure for assessing GD. The statistically significant positive associations were found among the GDSQ-21, weekly gaming hours (online, stand-alone, and video watching), average monthly gaming charge, and the VGDS test, providing empirical evidence for the validity of the test. Moreover, results from the EFA and CFA support the population cross validity of the GDSQ-21 as it was shown that the three-factor solution found in the EFA (i.e., sample 1) was also replicated and confirmed in the CFA (i.e., sample 2). Furthermore, the instrument was highly reliable in all samples as the Cronbach's α values were very high, suggesting that the GDSQ-21 measurement is reliable and accurate in detecting changes in GD levels.
Game duration and the game genres preference were significantly correlated with GD. GDSQ-21 sum scores were also associated with weekly playtime and average monthly spending on games. Previous studies have reported that spending a lot of time and money is a predictor of IGD (37). As seen in the game devices, more and more players are turning to smartphone games and table games. The current study found that smartphones may be more addictive than other devices for pathological gamers, and this finding is supported by Christian Montag's study (38, 39). In terms of game genres, the characteristics of different types of games attract different gamers, whereas the occurrence, symptoms, and negative consequences of GD are related to the genres of the game (6, 13). In general, complex, endless, and socially driven game types are more likely to have GD (40). It is worth noting that future studies should focus on different risky situations, as different situations require the adaptation of (early) intervention methods for optimal recovery of GD.
In the ROC analysis, the AUC was 89.6%, indicating strong discriminatory power. With adequate sensitivity and specificity, the GD was effectively distinguished from no GD. However, it is important to further examine whether the cutoff point of 62 (of a total score of 84) can distinguish disordered gamers from non-disordered among different populations, such as adults and individuals from other regions or countries.
Compared to the DSM-5 diagnostic criteria for IGD, the ICD-11 definition of GD may be more concise and research-based, highlighting the most central symptom presentation. Studies have found different rates of GD in screening between the DSM-5–based IGD criteria and the ICD-11 GD guidelines. For instance, the 12-month prevalence of IGD in Chinese adolescents was 2.9% according to DSM-5 criteria (22) and 2.27% in currently study.
Pontes and Mark (41) have also reported various inconsistencies and psychometric weaknesses in IGD instrumentation, but the GDSQ-21 instrument is considerable and has sufficiently sufficient reliability and validity. Its three factors take into account the size and intensity of the range of problematic game performance, and their items are not independent but interrelated. All items were good construct indicators due to all factor loadings being statistically significant and relatively high.
Although the available IGD instruments are still applicable measures based on the DSM-5 framework, the GDSQ-21 is a psychometric instrument developed under the new ICD-11 framework that will yield fundamental clinical and diagnostic differences between GD-based psychometric assessment instruments. The GDSQ-21 scale has excellent reliability and validity, and the theoretical concepts and connotations based on it are consistent with international standards.
Although the present study provides unique information about the ICD-11 criteria for GD, the limitations should be considered. First, given that adolescents are the most vulnerable group of GD, all participants in this study were adolescent students (aged 12–18 years). Thus, the current samples was not fully representative, and findings from current study should be cautiously interpreted in terms of its generalizability. Future studies should include adults and children to further confirm the robustness of the GDSQ-21. Second, the GD was assessed by the self-report questionnaires but not by professional clinical interviews and diagnoses. Future research in the field should compare clinically diagnosed sample with actual GDSQ-21 test scores.
In conclusion, the value of the GDSQ-21 as a GD screener for adolescents is evidenced by the current findings. The GDSQ-21 has excellent internal consistency reliability and criterion validity in a representative sample of adolescent game players. Furthermore, its three-factor structure supports the ICD-11 new diagnostic concept of GD, regarding persistent gaming behavior, impaired control over gaming, and functional impairment due to gaming for at least 12 months in most instances. Findings from this study recommend the use of the GDSQ-21 as a screening tool to assess GD, which can assist non-medical providers to screen people with GD.
The original contributions presented in the study are included in the article/Supplementary Material, further inquiries can be directed to the corresponding authors.
The studies involving human participants were reviewed and approved by the Ethics Committee of the Second Xiangya Hospital of Center South University (ID: 2019-S454). Written informed consent to participate in this study was provided by the participants' legal guardian/next of kin.
WH, MY, and LZ designed the assessment tools and study protocol. LZ and YC collected the data. LZ, YL, and TL performed the literature review, statistical analysis, and wrote the first draft. YL and TL commented on the manuscript. All authors interpreted the data, critically reviewed the content, and approved the submitted version.
This study was supported by the Non-profit Central Research Institute Fund of the Chinese Academy of Medical Sciences (No. 2019HY320001).
The authors declare that the research was conducted in the absence of any commercial or financial relationships that could be construed as a potential conflict of interest.
All claims expressed in this article are solely those of the authors and do not necessarily represent those of their affiliated organizations, or those of the publisher, the editors and the reviewers. Any product that may be evaluated in this article, or claim that may be made by its manufacturer, is not guaranteed or endorsed by the publisher.
The authors would like to thank all the participants.
The Supplementary Material for this article can be found online at: https://www.frontiersin.org/articles/10.3389/fpsyt.2022.848157/full#supplementary-material
1. Newzoo. An overview of 2021's key trends revenues, and projections. In: 2021 Global GamesMarket Report. Newzoo (2021). Available online at: https://newzoo.com/insights/trend-reports/newzoo-global-games-market-report-2021-free-version (accessed January 01, 2021).
2. China Internet Network Information Center. Development on recreational on-line applications. In: The 47st China Statistical Report on Internet Development. CINIC (2021). Available online at: http://www.cnnic.net.cn/hlwfzyj/hlwxzbg/hlwtjbg/202102/P020210203334633480104.pdf (accessed February 3, 2021).
3. Kuss DJ, Griffiths MD. Internet gaming addiction: a systematic review of empirical research. Int J Ment Health Addict. (2011) 10:278–96. doi: 10.1007/s11469-011-9318-5
4. Salguero R, Morán RM. Measuring problem video game playing in adolescents. Addiction. (2015) 97:1601–6. doi: 10.1046/j.1360-0443.2002.00218.x
5. Higuchi S, Nakayama H, Mihara S, Maezono M, Kitayuguchi T, Hashimoto T. Inclusion of gaming disorder criteria in ICD-11: a clinical perspective in favor. J Behav Addict. (2017) 6:293–5. doi: 10.1556/2006.6.2017.049
6. King DL, Delfabbro PH, Perales JC, Deleuze J, Kiraly O, Krossbakken E, et al. Maladaptive player-game relationships in problematic gaming and gaming disorder: a systematic review. Clin Psychol Rev. (2019) 73:101777. doi: 10.1016/j.cpr.2019.101777
7. Dong G, Wang L, Du X, Potenza MN. Gaming increases craving to gaming-related stimuli in individuals with internet gaming disorder. Biol Psychiatry Cogn Neurosci Neuroimaging. (2017) 2:404–12. doi: 10.1016/j.bpsc.2017.01.002
8. von der Heiden JM, Braun B, Muller KW, Egloff B. The association between video gaming and psychological functioning. Front Psychol. (2019) 10:1731. doi: 10.3389/fpsyg.2019.01731
9. Stevens MWR, Delfabbro PH, King DL. Prevention strategies to address problematic gaming: an evaluation of strategy support among habitual and problem gamers. J Prim Prev. (2021) 42:183–201. doi: 10.1007/s10935-021-00629-0
10. Stevens MWR, King DL, Dorstyn D, Delfabbro PH. Cognitive-behavioral therapy for Internet gaming disorder: a systematic review and meta-analysis. Clin Psychol Psychother. (2019) 26:191–203. doi: 10.1002/cpp.2341
11. Stevens MWR, Delfabbro PH, King DL. Prevention approaches to problem gaming: a large-scale qualitative investigation. Comput Human Behav. (2021) 115:6611. doi: 10.1016/j.chb.2020.106611
12. Long J, Liu T, Liu Y, Hao W, Maurage P, Billieux J. Prevalence and correlates of problematic online gaming: a systematic review of the evidence published in Chinese. Curr Addict Rep. (2018) 5:359–71. doi: 10.1007/s40429-018-0219-6
13. Petry NM, Zajac K, Ginley MK. Behavioral addictions as mental disorders: to be or not to be? Annu Rev Clin Psychol. (2018) 14:399–423. doi: 10.1146/annurev-clinpsy-032816-045120
14. American Psychiatric Publishing. Diagnostic and Statistical Manual of Mental Disorders (DSM-5®). Arlington, VA: American Psychiatric Publishing (2013).
15. Petry NM, Rehbein F, Gentile DA, Lemmens JS, Rumpf HJ, Mößle T, et al. An international consensus for assessing internet gaming disorder using the new DSM-5 approach. Addiction. (2014) 109:1399–406. doi: 10.1111/add.12457
16. Kuss DJ, Griffiths MD, Pontes HM. Chaos and confusion in DSM-5 diagnosis of Internet Gaming Disorder: issues, concerns, and recommendations for clarity in the field. J Behav Addict. (2017) 6:103–9. doi: 10.1556/2006.5.2016.062
17. Griffiths MD, van Rooij AJ, Kardefelt-Winther D, Starcevic V, Kiraly O, Pallesen S, et al. Working towards an international consensus on criteria for assessing internet gaming disorder: a critical commentary on Petry et al. 2014. Addiction. (2016) 111:167–75. doi: 10.1111/add.13057
18. Kaptsis D, King DL, Delfabbro PH, Gradisar M. Withdrawal symptoms in internet gaming disorder: a systematic review. Clin Psychol Rev. (2016) 43:58–66. doi: 10.1016/j.cpr.2015.11.006
19. Kardefelt-Winther D. A critical account of DSM-5 criteria for internet gaming disorder. Addict Res Theory. (2015) 23:93–8. doi: 10.3109/16066359.2014.935350
20. Kardefelt-Winther D. Excessive internet use - fascination or compulsion? (Ph.D. Thesis). London: The London School of Economics and Political Science (2014).
21. Starcevic V. Internet gaming disorder: inadequate diagnostic criteria wrapped in a constraining conceptual model. J Behav Addict. (2017) 6:110–3. doi: 10.1556/2006.6.2017.012
22. Qin L, Cheng L, Hu M, Liu Q, Tong J, Hao W, et al. Clarification of the cut-off score for nine-item internet gaming disorder scale-short form (IGDS9-SF) in a Chinese context. Front Psychiatry. (2020) 11:470. doi: 10.3389/fpsyt.2020.00470
23. Pontes HM, Griffiths MD. Measuring DSM-5 internet gaming disorder: development and validation of a short psychometric scale. Comput Human Behav. (2015) 45:137–43. doi: 10.1016/j.chb.2014.12.006
24. The World Heath Organization. 6C51 Gaming Disorder. The World Heath Organization (2019). Available online at: https://www.who.int/zh/news/item/18-06-2018-who-releases-new-international-classification-of-diseases-(icd-11) (accessed June 18, 2021).
25. Saunders JB, Hao W, Long J, King DL, Mann K, Fauth-Bühler M, et al. Gaming disorder: its delineation as an important condition for diagnosis, management, and prevention. J Behav Addict. (2017) 6:271–9. doi: 10.1556/2006.6.2017.039
26. Ko C-H, Lin H-C, Lin P-C, Yen J-Y. Validity, functional impairment and complications related to Internet gaming disorder in the DSM-5 and gaming disorder in the ICD-11. Austr N Zeal J Psychiatry. (2019) 54:707–18. doi: 10.1177/0004867419881499
27. Darvesh N, Radhakrishnan A, Lachance CC, Nincic V, Sharpe JP, Ghassemi M, et al. Exploring the prevalence of gaming disorder and Internet gaming disorder: a rapid scoping review. Syst Rev. (2020) 9:68. doi: 10.1186/s13643-020-01329-2
28. Paschke K, Austermann MI, Thomasius R. Assessing ICD-11 gaming disorder in adolescent gamers: development and validation of the gaming disorder scale for adolescents (GADIS-A). J Clin Med. (2020) 9:93. doi: 10.3390/jcm9040993
29. Balhara YPS, Singh S, Saini R, Kattula D, Chukkali S, Bhargava R. Development and validation of gaming disorder and hazardous gaming scale (GDHGS) based on the WHO framework (ICD-11 criteria) of disordered gaming. Asian J Psychiatry. (2020) 54:102348. doi: 10.1016/j.ajp.2020.102348
30. Saunders JB, Aasland OG, Babor TF, de la Fuente JR, Grant M. Development of the alcohol use disorders identification test (AUDIT): WHO collaborative project on early detection of persons with harmful alcohol consumption–II. Addiction. (1993) 88:791–804. doi: 10.1111/j.1360-0443.1993.tb02093.x
31. Rehbein F, Kliem S, Baier D, Mossle T, Petry NM. Prevalence of internet gaming disorder in German adolescents: diagnostic contribution of the nine DSM-5 criteria in a state-wide representative sample. Addiction. (2015) 110:842–51. doi: 10.1111/add.12849
32. Liao ZJ, Huang QP, Huang SC, Tan LX, Shao TL, Fang T, et al. Prevalence of internet gaming disorder and its association with personality traits and gaming characteristics among Chinese adolescent gamers. Front Psychiatry. (2020) 11:598585. doi: 10.3389/fpsyt.2020.598585
33. Bentler PM. Comparative fit indexes in structural models. Psychol Bull. (1999) 10712:238–46. doi: 10.1037/0033-2909.107.2.238
34. Jackson DL, Gillaspy JA, Purc-Stephenson R. Reporting practices in confirmatory factor analysis: an overview and some recommendations. Psychol Methods. (2009) 14:6–23. doi: 10.1037/a0014694
35. McDonald RP, Ho M-HR. Principles and practice in reporting structural equation analyses. Psychol Methods. (2002) 7:64–82. doi: 10.1037/1082-989X.7.1.64
36. Hu Lt, Bentler PM. Cutoff criteria for fit indexes in covariance structure analysis: conventional criteria versus new alternatives. Struct Eq Model Multidiscip J. (1999) 6:1–55. doi: 10.1080/10705519909540118
37. Rho MJ, Lee H, Lee TH, Cho H, Jung DJ, Kim DJ, et al. Risk factors for internet gaming disorder: psychological factors and internet gaming characteristics. Int J Environ Res Public Health. (2017) 15:40. doi: 10.3390/ijerph15010040
38. Montag C, Wegmann E, Sariyska R, Demetrovics Z, Brand M. How to overcome taxonomical problems in the study of Internet use disorders and what to do with “smartphone addiction”? J Behav Addict. (2021) 9:908–14. doi: 10.1556/2006.8.2019.59
39. Montag C, Lachmann B, Herrlich M, Zweig K. Addictive features of social media/messenger platforms and freemium games against the background of psychological and economic theories. Int J Environ Res Public Health. (2019) 16:2612. doi: 10.3390/ijerph16142612
40. Brand M, Young KS, Laier C, Wolfling K, Potenza MN. Integrating psychological and neurobiological considerations regarding the development and maintenance of specific Internet-use disorders: an interaction of person-affect-cognition-execution (I-PACE) model. Neurosci Biobehav Rev. (2016) 71:252–66. doi: 10.1016/j.neubiorev.2016.08.033
Keywords: gaming disorder, the Gaming Disorder Symptom Questionnaire-21, scale development, validation, ICD-11
Citation: Zhang L, Luo T, Hao W, Cao Y, Yuan M and Liao Y (2022) Gaming Disorder Symptom Questionnaire: The Development and Validation of a Screening Tool for ICD-11 Gaming Disorder in Adolescents. Front. Psychiatry 13:848157. doi: 10.3389/fpsyt.2022.848157
Received: 04 January 2022; Accepted: 31 January 2022;
Published: 24 March 2022.
Edited by:
Xiaochu Zhang, University of Science and Technology of China, ChinaReviewed by:
Ni Fan, Guangzhou Medical University, ChinaCopyright © 2022 Zhang, Luo, Hao, Cao, Yuan and Liao. This is an open-access article distributed under the terms of the Creative Commons Attribution License (CC BY). The use, distribution or reproduction in other forums is permitted, provided the original author(s) and the copyright owner(s) are credited and that the original publication in this journal is cited, in accordance with accepted academic practice. No use, distribution or reproduction is permitted which does not comply with these terms.
*Correspondence: Ming Yuan, 178202076@csu.edu.cn; Yanhui Liao, liaoyanhui@zju.edu.cn
Disclaimer: All claims expressed in this article are solely those of the authors and do not necessarily represent those of their affiliated organizations, or those of the publisher, the editors and the reviewers. Any product that may be evaluated in this article or claim that may be made by its manufacturer is not guaranteed or endorsed by the publisher.
Research integrity at Frontiers
Learn more about the work of our research integrity team to safeguard the quality of each article we publish.