- 1Key Laboratory of Psychoseomadsy, Stomatological Hospital of Chongqing Medical University, Chongqing, China
- 2College of Stomatology and Affiliated Stomatological Hospital of Chongqing Medical University, Chongqing, China
- 3Chongqing Key Laboratory for Oral Diseases and Biomedical Sciences, Chongqing, China
- 4National Health Commission Key Laboratory of Diagnosis and Treatment on Brain Functional Diseases, The First Affiliated Hospital of Chongqing Medical University, Chongqing, China
- 5Department of Neurology, Yongchuan Hospital of Chongqing Medical University, Chongqing, China
- 6Department of Pathology, Faculty of Basic Medicine, Chongqing Medical University, Chongqing, China
Accumulating evidence indicates an important role for microRNA (miRNA)–messenger RNA (mRNA) regulatory networks in human depression. However, the mechanisms by which these networks act are complex and remain poorly understood. We used data mining to identify differentially expressed miRNAs from GSE81152 and GSE152267 datasets, and differentially expressed mRNAs were identified from the Netherlands Study of Depression and Anxiety, the GlaxoSmithKline-High-Throughput Disease-specific target Identification Program, and the Janssen-Brain Resource Company study. We constructed a miRNA–mRNA regulatory network based on differentially expressed mRNAs that intersected with target genes of differentially expressed miRNAs, and then performed bioinformatics analysis of the network. The key candidate genes were assessed in the prefrontal cortex of chronic social defeat stress (CSDS) depression mice by quantitative real-time polymerase chain reaction (qRT-PCR). Three differentially expressed miRNAs were commonly identified across the two datasets, and 119 intersecting differentially expressed mRNAs were identified. A miRNA–mRNA regulatory network including these three key differentially expressed miRNAs and 119 intersecting differentially expressed mRNAs was constructed. Functional analysis of the intersecting differentially expressed mRNAs revealed that an abnormal inflammatory response characterized by disturbed T-helper cell 17 (Th17) differentiation was the primary altered biological function. qRT-PCR validated the decreased expression of Th17 cell differentiation-related genes, including interleukin (IL)17A, IL21, IL22, and IL1β, and the increased expression of retinoic acid receptor-related orphan receptor gamma-t (RORγt) in CSDS mice, which showed significant depressive- and anxiety-like behaviors. This study indicates that an abnormal inflammatory response characterized by disturbed Th17 cell differentiation is the primary altered biological process in major depressive disorder. Our findings indicate possible biomarkers and treatment targets and provide novel clues to understand the pathogenesis of major depressive disorder.
Introduction
Major depressive disorder (MDD) is a debilitating disease and contributes greatly to the global burden of disease (1). One in six adults were reported to be affected by depression, which not only can dramatically affect one's ability to work and function, but also increases the risk of developing other conditions, such as cardiovascular diseases and diabetes mellitus (2). In addition, the comorbidity of depression with other psychiatric disorders (e.g., anxiety) is increasingly prevalent, and this can lead to an increased risk for suicidality (3, 4). However, up to 40% of patients do not remit from MDD, even after several treatment attempts (5). This may be because of the biological heterogeneity of depression (6); therefore, further exploration of the biological processes underlying depression is essential for precision medicine.
Preclinical animal models have enabled the discovery of neurophysiological mechanisms underlying depression (7). We previously verified that different categories of stressor, “physical” and “psychological,” elicit distinctive molecular responses (8). However, the symptoms of human depression can only partly be mimicked in animals. Therefore, biological samples from patients are valuable for ascertaining the molecular mechanisms of depression and for identifying diagnostic biomarkers. Previously, we used a metabolomics approach to uncover molecular changes in MDD patients. We found that acyl carnitine metabolism, lipid metabolism, and tryptophan metabolism were the primary disturbed pathways in adult patients (9, 10), while polyunsaturated fatty acid metabolism and purine metabolism were prominent pathways in child and adolescent patients (11). Moreover, accumulating evidence suggest pivotal roles of inflammatory responses in MDD pathophysiology (12–14). Inflammatory mediators and cytokines regulate immune responses through anti-inflammatory and pro-inflammatory pathways (15, 16). T-cell cytokines play a central role in the initiation and regulation of cellular immune responses (17, 18). The imbalance of different T cells, such as T-helper 17 cells (Th17) and regulatory T cells is critical for the progression of MDD. However, the underlying mechanisms of depression are complex and remain poorly understood.
Non-coding RNAs are essential epigenetic regulators involved in complex biological processes and play key roles in the pathogenesis of MDD. MicroRNAs (miRNAs) are endogenous small non-coding RNAs ~22 nucleotides in length that regulate gene expression by binding to the 3′-untranslated region of target messenger RNAs (mRNAs) (19), which leads to translational repression (20). Many miRNAs are functionally dysregulated in MDD (21). For example, our previous studies indicate dysregulation of various miRNAs (e.g., miR-190a-5p, miR-539-5p, and miR-363-5p) in depression-related brain dysfunction (22). We have also shown downregulation of miR-134 in MDD patients, which may be recognized as a state-dependent biomarker for depression (23). In addition, miR-138 increased depressive-like behaviors in mice by regulating SIRT1 (24). However, the miRNA–mRNA interactions in the pathogenesis of depression need to be further explored.
We hypothesize that the disorganized immune response and the miRNA–mRNA interactions that regulate Th17 cell differentiation are potential therapeutic targets in MDD. In this study, we performed a systematic analysis to identify aberrant miRNAs based on public databases. We then constructed a miRNA–mRNA interaction network and performed bioinformatics functional analysis to predict the potential mechanisms of these miRNA–mRNA pairs in the development of MDD. Furthermore, the MDD-associated candidate genes were assessed in the prefrontal cortex of chronic social defeat stress (CSDS) depression mice by quantitative real-time polymerase chain reaction (qRT-PCR). This study identifies potential diagnostic biomarkers and provides clues for exploring the pathogenesis of MDD.
Materials and Methods
miRNA Microarray
We searched for suitable datasets that focused on miRNA changes between patients with MDD and healthy controls in the Gene Expression Omnibus (GEO) database (http://www.ncbi.nlm.nih.gov/geo/). After evaluating the datasets of interest, original miRNA profiles from two datasets (GSE81152 and GSE152267), based on the Exiqon Human miRCURY LNA microRNA Array (GPL21814) and the [miRNA-4] Affymetrix Multispecies miRNA-4 Array (GPL21572), respectively, were obtained for subsequent analysis. The GSE81152 dataset contained 30 pre-treatment MDD patients and 19 healthy controls, and the GSE152267 dataset contained 7 MDD patients and 6 healthy controls.
Identification of Differentially Expressed miRNAs
GEO2R (http://www.ncbi.nlm.nih.gov/geo/geo2r/), an interactive web tool based on the limma R package, was used to identify the differentially expressed miRNAs (DEMs) between MDD patients and health controls from the two datasets. The DEMs were identified by the thresholds of |log2FC| > 1 and p < 0.05. The common DEMs identified from both GSE81152 and GSE152267 datasets were re-defined as key miRNAs and used for further analysis. A Venn diagram was produced using the E-Venn online tool (http://www.ehbio.com/test/venn/#/).
Prediction of DEM Targets
The downstream target genes of DEMs were predicted using TargetScan (25), DIANA-TarBase v8 (26), miRDB (27), miRTarBase (28), and miRWalk (29).
Construction of a miRNA–mRNA Interaction Network
To identify MDD-related genes, differentially expressed mRNAs (DEGs) were selected from the Netherlands Study of Depression and Anxiety (NESDA) (30), the GlaxoSmithKline-High-Throughput Disease-specific target Identification Program (GSK-HiTDiP), and the Janssen-Brain Resource Company (Janssen-BRC) study (31). The identified DEGs were intersected with target genes of DEMs. The intersected DEG sets were used to construct the miRNA–mRNA network on the basis of the interactions between key DEMs and DEGs, and this regulatory network was visualized using Cytoscape (version 3.7.2) (32).
Functional Enrichment Analysis
To further assess the biological functions of the miRNA–mRNA pairs, Gene Ontology (GO) annotation, and Kyoto Encyclopedia of Genes and Genomes (KEGG) enrichment pathway analyses were performed for the intersected DEGs using the online OmicsBean system (http://www.omicsbean.cn). p-values are calculated using Fisher's Exact test with a hypergeometric algorithm. GO terms, including biological process, cellular component and molecular function, and KEGG pathways were considered to be significantly enriched with a p < 0.05.
Protein–Protein Interaction Analysis
To obtain a better understanding of the relationships among the genes of interest, a PPI network was established based on the Search Tool for the Retrieval of Interacting Genes (STRING) database (https://string-db.org/), and visualized using Cytoscape (version 3.7.2). Any interaction with a combined score ≥0.4 was included in the PPI network.
CSDS Model of Depression
Adult male C57BL/6 J mice (weighing 20–23 g) and male CD1 mice (weighing 35–40 g) were obtained from the Experimental Animal Center of Chongqing Medical University (Chongqing, China). This experiment was approved by the Ethics Committee of Chongqing Medical University. The mice were kept under standard conditions (constant temperature, 21 ± 1°C; relative humidity, 55 ± 5%; and a 12-h light cycle with lights on at 8:00) with food and water available ad libitum. The CSDS procedure was conducted as previously described (33). Briefly, CSDS was initiated when an “intruder” (C57BL/6 J mouse) was introduced into the home cage of an aggressive “resident” (CD1 mouse) for physical contact lasting 10 min. Subsequently, the intruder was immediately transferred to the opposite compartment of the defeated cages, which allowed sensory contact for 24 h. After the 12-day CSDS period, a series of behavioral tests was performed.
A social interaction test was used to detect social avoidance behaviors. The immobility time in the forced swimming test and tail suspension test was used to evaluate depression-like behaviors. Time spent in the central and perimeter areas during the open field test (34), as well as the time spent in the open and closed arms of an elevated plus-maze are recognized as measures of anxiety-like behaviors. The data are expressed as the mean ± standard error of the mean (SEM), and statistical analysis was performed with the Mann–Whitney U-test using SPSS 21.0 (IBM, New York, USA).
RNA Extraction and qRT-PCR
Total RNA was extracted from prefrontal cortex tissues using Trizol reagent (Invitrogen, USA). A total of 1 μg of RNA was reverse transcribed into cDNA using a PrimeScript RT reagent Kit (Takara, Japan). For RNA quantification, three replicate CSDS and control samples were analyzed using the SYBR gene detection system (Roche, Germany). Expression levels of target miRNAs were normalized to small nuclear RNA U6, and those of mRNAs were normalized to β-actin, and calculated using the 2−ΔΔCt method. We used non-parametric statistical methods to reduce the effect of the small sample size. The primers used are shown in Supplementary Table S1.
Results
Screening for Significant DEMs in MDD
miRNA microarray datasets in the GEO database relevant to MDD were searched for and GSE81152 and GSE152267 datasets were identified. The interactive web tool, GEO2R, was then used to analyze the DEMs from each dataset. Volcano plots showing the DEMs from GSE81152 and GSE152267 datasets are displayed in Figures 1A,B, respectively. We identified 61 DEMs in the GSE81152 dataset and 73 in the GSE152267 dataset. We then analyzed overlap of the DEMs from the two datasets, and found three overlapping DEMs (Figure 1C), hsa-miR-194-5p, hsa-miR-25-3p, and hsa-miR-125a-5p. These common DEMs were selected for further analysis.
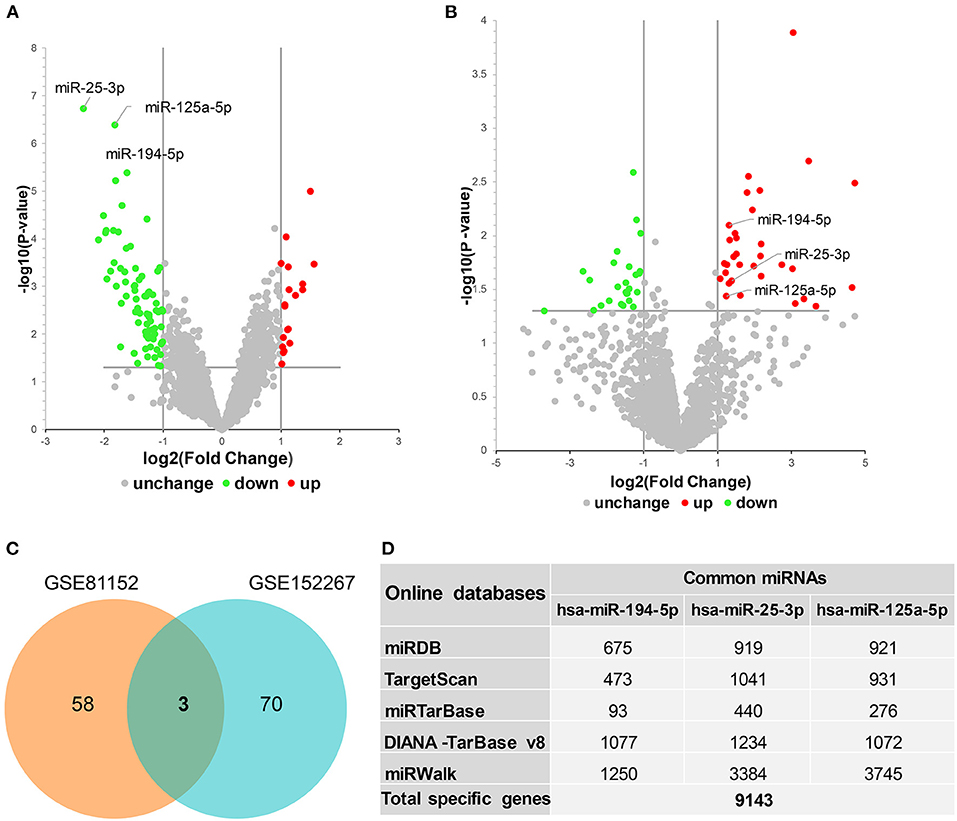
Figure 1. Identification of differentially expressed miRNAs (DEMs) in major depressive disorder. (A,B) Volcano plots showing DEMs identified from GSE81152 and GSE152267 datasets, respectively. UP, upregulated; DW, downregulated; NoDiff, no differential expression. (C) Venn diagram showing the intersected DEMs between GSE81152 and GSE152267 datasets. (D) The prediction of target genes of these common DEMs.
Construction of a miRNA–mRNA Regulatory Network in MDD
We first predicted targets of the miRNAs using several online software packages and identified 9,143 target genes after removing duplicates (Figure 1D). In addition, to analyze changes in MDD at the mRNA level, we reanalyzed case–control studies focusing on gene expression in peripheral blood of MDD patients. As a result, we selected 129 DEGs between the MDD and control groups in the NESDA study, as well as 165 DEGs in both GSK-HiTDiP and Janssen-BRC studies that had concordant direction of expression changes. Merging these identified DEGs with the 9,143 DEM target genes identified 119 intersected DEGs. Finally, we used the three key DEMs and the 119 intersected DEGs to construct a miRNA–mRNA regulatory network based on the interactions between miRNAs and mRNAs (Figure 2). As shown in this miRNA–mRNA interaction network, hsa-miR-25-3p has the most interaction relationships with 70 pairs, followed by hsa-miR-125a-5p with 55 pairs and hsa-miR-194-5p with 31 pairs. Interestingly, several genes were targeted by all three key DEMs, such as Rora, indicating the potential roles of these genes in the pathogenesis of MDD.
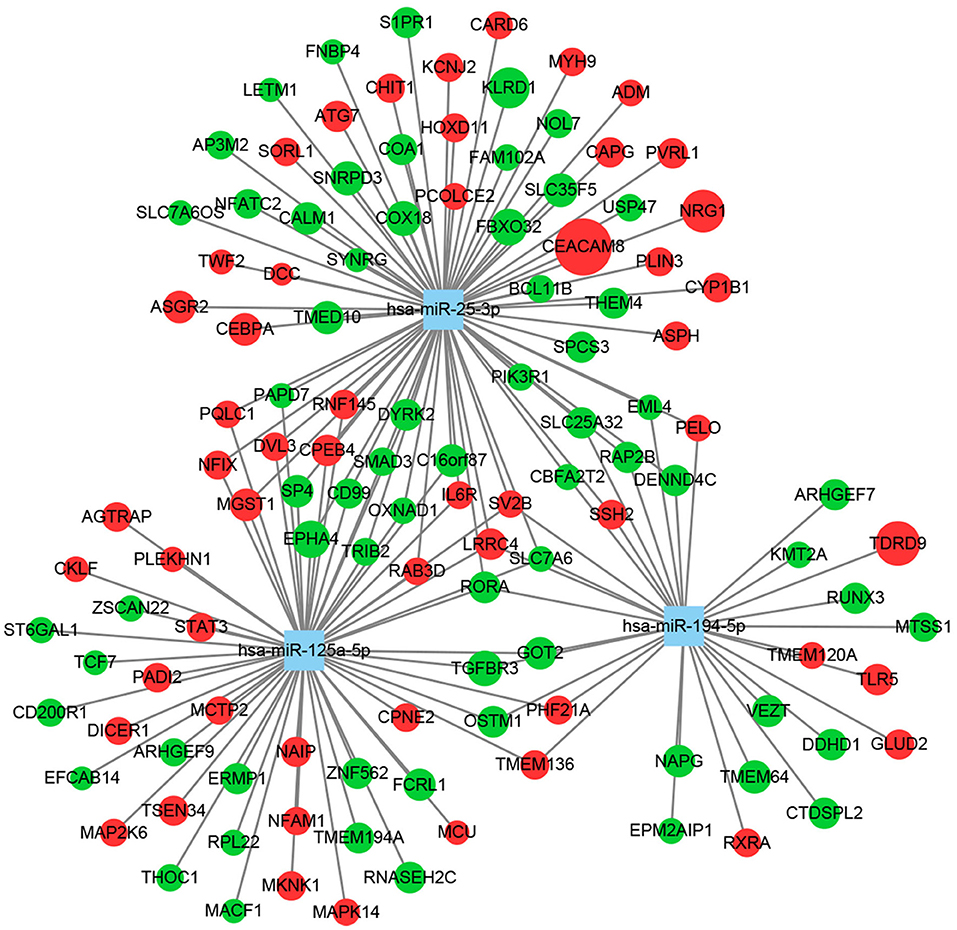
Figure 2. Construction of a miRNA–mRNA regulatory network using Cytoscape. Squares represent miRNAs, circles represent mRNAs, green indicates downregulation, red indicates upregulation, and circle size reflects the degree of change.
Functional Analysis for the Intersected DEGs
To further investigate the underlying functions of these 119 DEGs in MDD, we first performed GO annotations for the intersected genes (Figure 3). Single-multicellular organism process (p = 1.11E-26) was the most significantly enriched GO term in the biological process category (Supplementary Table S2). Intracellular membrane-bounded organelle (p = 8.37E-19) was the primary annotated term in the cellular component category at level 5 (Supplementary Table S3), and protein homodimerization activity (p = 4.85E-08) was the top term in the molecular function category (Supplementary Table S4). Moreover, KEGG enrichment pathway analysis revealed that disturbed Th17 differentiation (p = 6.73E-06) in the immune system was the primary altered function in MDD patients. Cellular senescence is a cellular process that is significantly altered in MDD patients (Figure 4A and Supplementary Table S5). In addition, based on the STRING database, a PPI network of the 119 intersected DEGs was constructed and is shown in Figure 4B. According to the interaction degree, we identified two hub genes, STAT3 and MAPK14, in the primary sub-network, which included 47 nodes and 65 edges. Interestingly, STAT3 and MAPK14 proteins play essential roles in regulating Th17 cell differentiation.
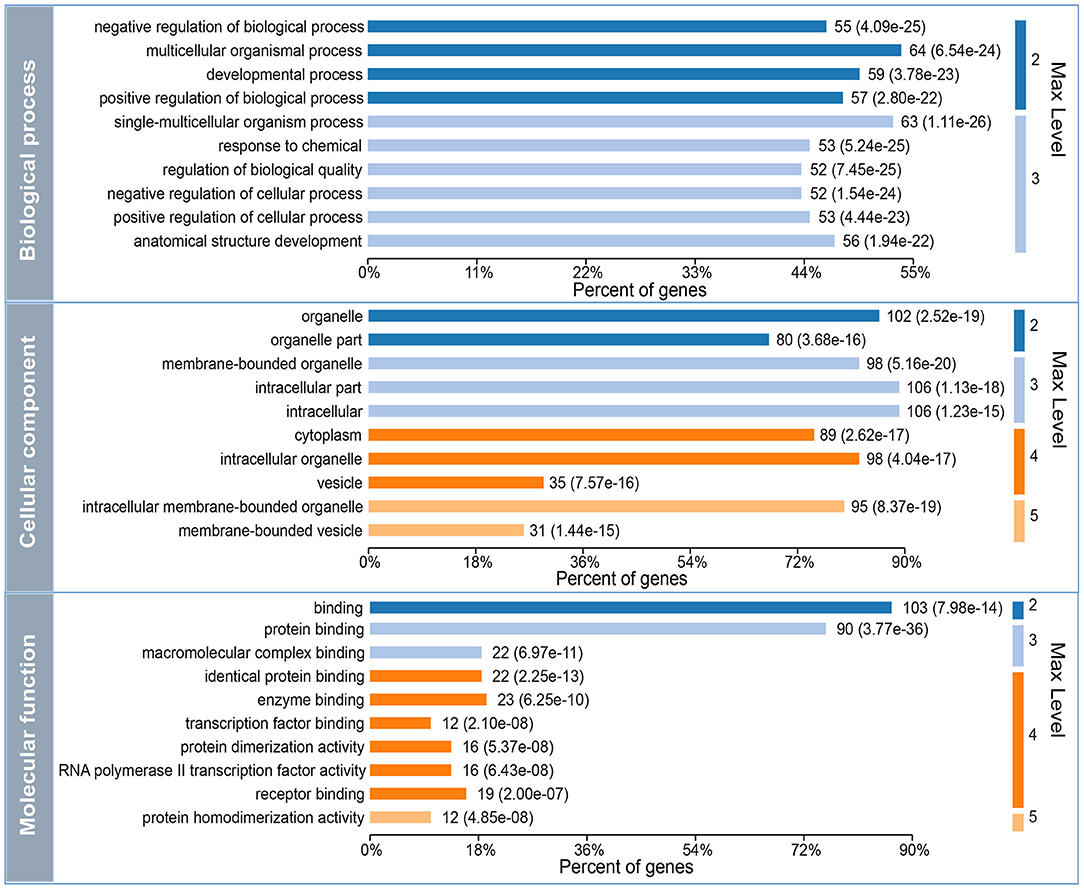
Figure 3. Gene Ontology (GO) annotation for the 119 differentially expressed mRNAs (DEGs) that intersected with the target genes of differentially expressed miRNAs (DEMs). Max level means the maximal annotated level of this term in the GO graph (tree), and the number indicates the depth of the GO term level.
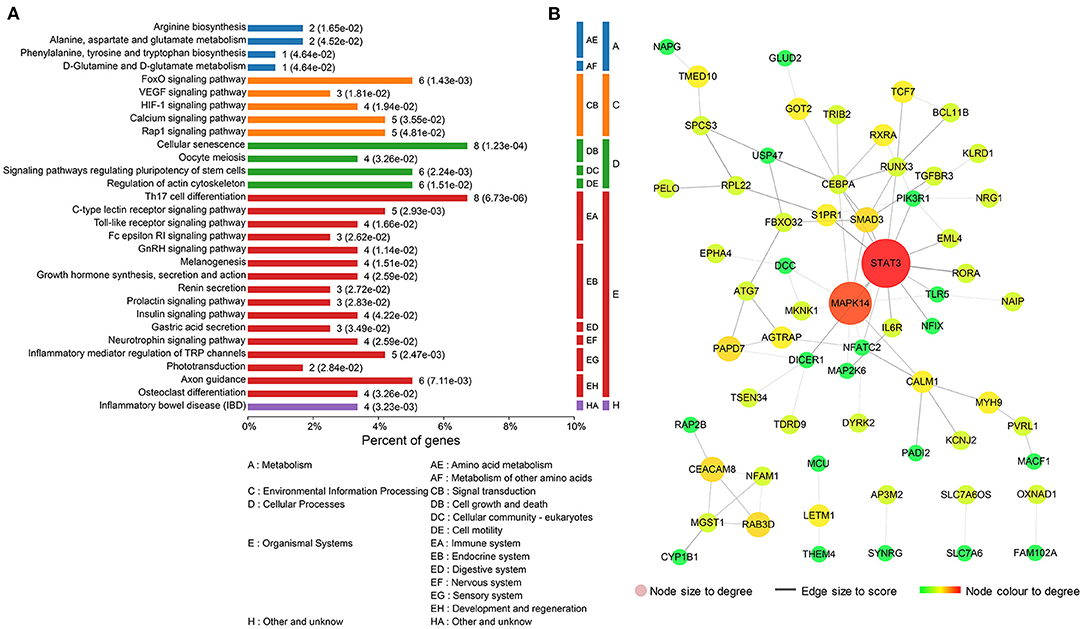
Figure 4. Functional analysis of the 119 differentially expressed mRNAs (DEGs) that intersected with the target genes of differentially expressed miRNAs (DEMs). (A) Kyoto Encyclopedia of Genes and Genomes (KEGG) enrichment pathway analysis for the 119 intersected DEGs. (B) Protein–protein interaction (PPI) network for the 119 intersected DEGs.
Generation of CSDS Depression Model Mice
According to the experimental schedule shown in Figure 5A, we generated a CSDS model mouse group (n = 14) and a control (CON) mouse group (n = 11). There was no statistical difference in body weight gain between the two groups after the 12-day CSDS period (Figure 5B); however, the CSDS group had a lower social interaction ratio than the CON group (p < 0.001, Figure 5C), indicating establishment of the CSDS model. In the open field test, CSDS group mice showed more anxiety-like behaviors compared with CON group mice, characterized by a significantly longer time spent in the perimeter area and a shorter time spent in the central area (p < 0.01, Figures 5D,E). Time spent in the open and closed arms in the elevated plus-maze test was also analyzed to investigate anxiety-like behaviors; however, no significant differences were found (Figures 5F,G). In the forced swimming test (Figure 5H, p < 0.05) and the tail suspension test (Figure 5I, p < 0.01), immobility times were significantly increased in the CSDS group compared with the CON group, indicating significant depressive-like behaviors in CSDS mice.
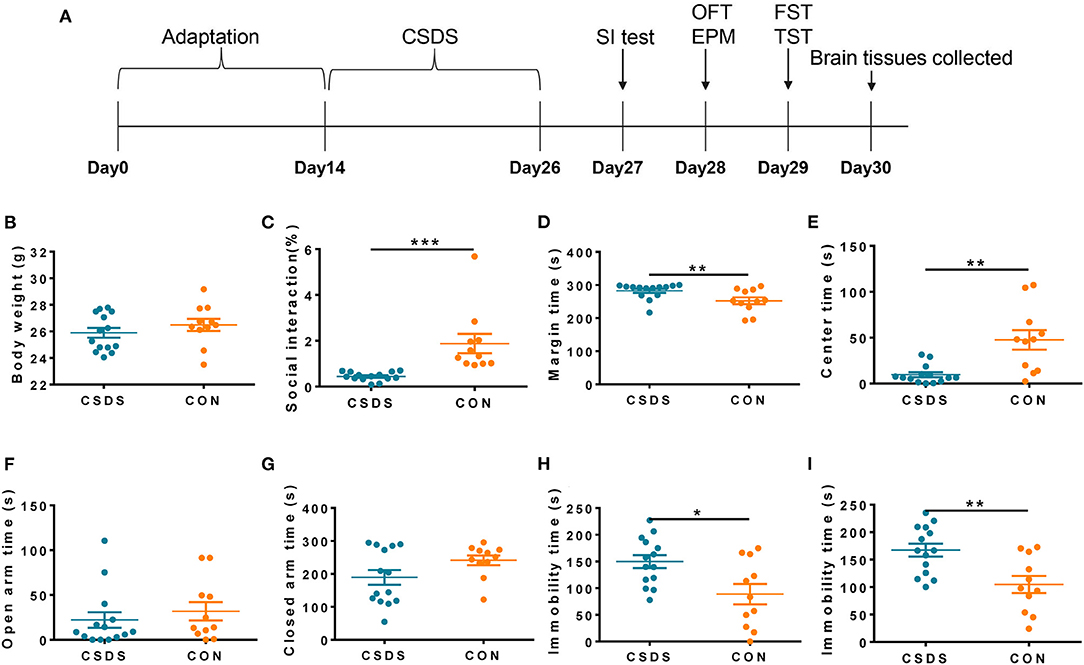
Figure 5. Generation of the chronic social defeat stress (CSDS) depression mouse model. (A) Schedule of the experimental process. (B) No significant difference was found in body weight between CSDS and control mice. (C) The social interaction (SI) ratio was significantly reduced in the SI test in CSDS mice compared with control mice. (D,E) CSDS mice spent significantly more time in the perimeter area and significantly less time in the central area in the open field test compared with control mice. (F,G) No significant differences were found in time spent in open arms or closed arms in the elevated plus-maze test between CSDS and control mice. (H,I) Immobility time was significantly increased in CSDS mice in the forced swimming test and the tail suspension test compared with control mice. Data represent the mean ± SEM. *p < 0.05, **p < 0.01, and ***p < 0.001.
Validation of Th17 Cell Differentiation by qRT-PCR
To directly assess the state of depression-related Th17 cell differentiation, we analyzed the expression levels of 26 related genes in prefrontal cortex tissues of CSDS depression model mice using qRT-PCR, and the expression levels of the three common DEMs, miR-194-5p, miR-25-3p, and miR-125a-5p. Among these genes, miR-194-5p and key factors in promoting Th17 cell differentiation, interleukin (Il)17A, Il21, Il22, and Il1β were significantly downregulated in CSDS depression mice compared with control mice, while retinoic acid receptor-related orphan receptor gamma-t (Rorγt) was upregulated (Figure 6). In addition, the expression level of IL6 receptor (Il6R) was decreased, although not significantly, in CSDS mice compared with controls (p = 0.063). The expression levels of the remaining genes were unchanged.
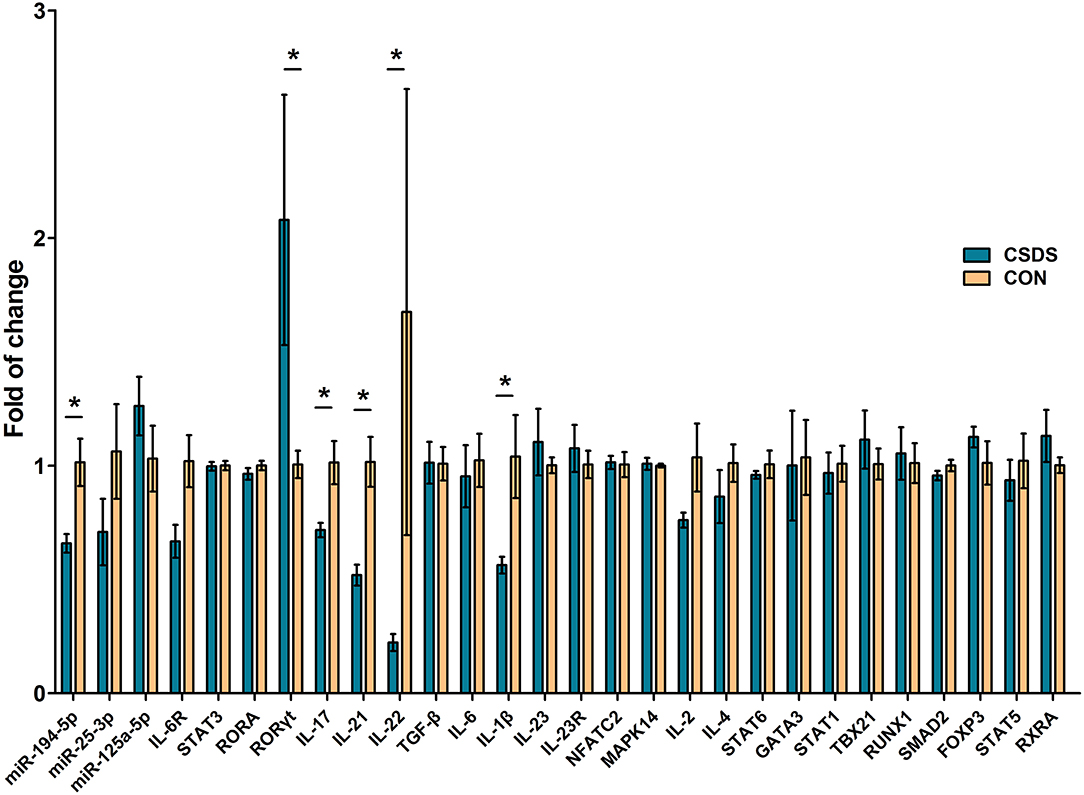
Figure 6. Assessment of Th17 cell differentiation-related gene expression in the prefrontal cortex of CSDS mice by real-time polymerase chain reaction. Data represent the mean ± SD. *p < 0.05.
Discussion
In the present study, we constructed a miRNA–mRNA regulatory network for MDD. We found that a disorganized immune response, characterized by disturbed Th17 cell differentiation, plays a pivotal role in the pathophysiology of MDD. We also assessed the expression of Th17 cell differentiation-related genes in CSDS mice. Our data indicate that a disorganized immune response and the miRNA–mRNA interactions that regulate Th17 cell differentiation might be appropriate therapeutic targets in MDD. MDD is a multifactorial disorder induced by interactions of social, psychological, and biological factors; its pathophysiological mechanisms are complex and remain elusive. Accumulating evidence indicates that neurotransmitter disturbances, hypothalamic–pituitary–adrenal axis perturbations, and disrupted neurogenesis play roles in MDD. However, no established mechanism can explain all aspects of this disorder. Our poor understanding of the pathophysiological mechanisms of depression greatly limits the promise of precision medicine for MDD. In this study, we explored the pathophysiological mechanisms of MDD based on publicly available blood miRNA and mRNA expression data, and then validated the main findings in a CSDS depression mouse model. We found that an abnormal inflammatory response characterized by disturbed Th17 cell differentiation was the primary altered biological process in MDD.
In recent decades, preclinical studies have indicated that an abnormal inflammatory response is associated with the onset of depression (35). Immune-mediated chronic inflammatory diseases, such as infectious diseases (34), rheumatoid arthritis (36), and type 1 diabetes mellitus (37), increase the risk of mood disorders, in particular depression. Neuroinflammation is a key component of various neurological diseases (38) and the levels of most central and peripheral pro-inflammatory markers, such as C-reactive protein and tumor necrosis factor α (TNFα), are increased in depressed patients (39, 40) and decrease to normal levels after recovery. Moreover, patients treated with interferon α, a pro-inflammatory factor, for somatic diseases, such as malignant melanoma, can suffer from depressive symptoms (41). Anti-inflammatory drugs, such as non-steroidal anti-inflammatory drugs and cytokine inhibitors, may provide antidepressant properties. Interestingly, beneficial effects of anti-inflammatory treatment for depression were validated in randomized clinical trials (42, 43). In addition, classic antidepressants, such as tricyclic antidepressants and selective serotonin reuptake inhibitors, significantly inhibit the secretion of pro-inflammatory markers, such as TNFα, and stimulate that of anti-inflammatory markers, such as IL10 (44, 45). Taken together, we speculate that a disturbed inflammatory response plays a pivotal role in the pathogenesis of depression.
miR-194-5p and miR-125a-5p regulate the release of some pro-inflammatory cytokines, including transforming growth factor β, IL1β, and IL6 (46, 47), and have vital functions in the pathogenesis of neuroinflammation-associated diseases. Here, we identified a decrease in the expression level of miR-194-5p in depression. miR-194-5p is also significantly related to drug addiction (48). miR-25-3p is a key regulator of the social defeat stress-induced inflammatory response and depression (49). Based on our prediction analysis, miR-194-5p, miR-25-3p, and miR-125a-5p regulate the expression of target genes related to Th17 cell differentiation, including IL6R, MAPK14, STAT3, NFATC2, RUNX1, and RORA, as well as IL17 and IL17R. Th17 cells are a subset of CD4+ T cells that play a central role in the immune responses against extracellular pathogens. They are also implicated in the pathophysiology of autoimmune diseases. miR-125a-5p overexpression causes increased FOXP3 expression and decreased RORγt expression, which contributes to a regulatory T cell (Treg)/Th17 imbalance (50). Rora expression was unaltered in the prefrontal cortex of CSDS mice compared with control mice.
In recent years, Th17 cells were confirmed to have a pivotal role in the pathogenesis of depression (51–53); however, the details of this role are uncertain. Significantly, patients with MDD have a decreased percentage of Th17 cells compared with healthy controls because of impaired maturation of Th17 cells in MDD patients (54). Treg cells are crucial for the suppression of potentially harmful excessive immune responses (55). An imbalance between Th17 and Treg cells, characterized by a decrease in the percentage of Th17 cells and an increase in the percentage of Treg cells, occurs in the development of depression-like behavior in mice induced by chronic unpredictable mild stress (56). Stress inhibits pro-inflammatory cytokines and induces anti-inflammatory cytokines (57). Some cytokines, such as IL1β, initially promote CD4+ T cells to secrete IL17, IL21, and IL22, which in turn produces Th17 cells. Interestingly, we show here that the expression level of Il1β was downregulated in CSDS depression mice compared with controls, as were cytokines specific for Th17 cells, Il17A, Il21, and Il22. Consistently, elevated levels of IL17 are associated with symptomatic reduction in depressed patients treated with a bupropion-selective serotonin reuptake inhibitor combination (58). Our findings indicate that disturbed Th17 cell differentiation may play a crucial role in the onset of depression.
Although our present findings have important clinical implications, some limitations need to be noted. First, because of the heterogeneity of depression and the paucity of available clinical information, we did not consider MDD subtypes in the datasets. We were therefore unable to observe DEM signatures that correlate with comorbidities associated with MDD or potential MDD subtypes. In addition, there are some paradoxes in the molecular changes in MDD based on the GEO database and future studies that include ethnically diverse patients from different populations are required to reveal subtype-specific molecular changes in MDD. Second, the main findings of this study need to be further investigated in large-scale samples. Third, verification of protein levels by Western blotting would be valuable.
Conclusions
In conclusion, our study shows that a disorganized immune response plays a pivotal role in the pathophysiology of MDD. Furthermore, Th17 cell differentiation was the primary altered biological process in MDD. Taken together, our findings show that the disorganized immune response, and the miRNA–mRNA interactions that regulate Th17 cell differentiation might be appropriate therapeutic targets in MDD. The present findings indicate possible biomarkers and treatment targets for MDD and provide novel clues to understand the pathogenesis of MDD.
Data Availability Statement
Publicly available datasets were analyzed in this study. This data can be found here: GSE81152 and GSE152267 datasets can be downloaded from the GEO database, and mRNA data can be found in the NESDA study (30), as well as the GSK–HiTDiP study and the Janssen—BRC study (31).
Ethics Statement
The animal study was reviewed and approved by the Ethics Committee of Chongqing Medical University.
Author Contributions
HW, PJ, and PX conceived and designed the study. HW and LL wrote the manuscript. XC, XR, WXL, and CZ revised the manuscript. WWL, PJ, YL, and LF made the figures with the help of HZ and JS. All authors contributed to the article and approved the submitted version.
Funding
This study was supported by the National Key R&D Program of China (Grant No. 2017YFA0505700), the Non-Profit Central Research Institute Fund of the Chinese Academy of Medical Sciences (Grant No. 2019PT320002), the Natural Science Foundation Project of China (Grant No. 81820108015), and the China Postdoctoral Science Foundation (Nos. 2020TQ0393, 2020M683634XB, and 2021M693926).
Conflict of Interest
The authors declare that the research was conducted in the absence of any commercial or financial relationships that could be construed as a potential conflict of interest.
Publisher's Note
All claims expressed in this article are solely those of the authors and do not necessarily represent those of their affiliated organizations, or those of the publisher, the editors and the reviewers. Any product that may be evaluated in this article, or claim that may be made by its manufacturer, is not guaranteed or endorsed by the publisher.
Supplementary Material
The Supplementary Material for this article can be found online at: https://www.frontiersin.org/articles/10.3389/fpsyt.2022.824209/full#supplementary-material
References
1. GBD 2017 Disease and Injury Incidence and Prevalence Collaborators. Global, regional, national incidence. prevalence, and years lived with disability for 354 diseases and injuries for 195 countries and territories, 1990-2017: a systematic analysis for the Global Burden of Disease Study 2017. Lancet (London, England). (2018) 392:1789–858. doi: 10.1016/S0140-6736(18)32279-7
2. Rasgon NL, McEwen BS. Insulin resistance-a missing link no more. Mol Psychiatry. (2016) 21:1648–52. doi: 10.1038/mp.2016.162
3. Chesney E, Goodwin GM, Fazel S. Risks of all-cause and suicide mortality in mental disorders: a meta-review. World Psychiatry. (2014) 13:153–60. doi: 10.1002/wps.20128
4. Quevedo L, de Mola CL, Pearson R, Murray J, Hartwig FP, Gonçalves H, et al. Mental disorders, comorbidities, and suicidality at 30 years of age in a Brazilian birth cohort. Compr Psychiatry. (2020) 102:152194. doi: 10.1016/j.comppsych.2020.152194
5. Holtzheimer PE, Mayberg HS. Stuck in a rut: rethinking depression and its treatment. Trends Neurosci. (2011) 34:1–9. doi: 10.1016/j.tins.2010.10.004
6. Keers R, Uher R. Gene-environment interaction in major depression and antidepressant treatment response. Curr Psychiatry Rep. (2012) 14:129–37. doi: 10.1007/s11920-011-0251-x
7. Fox ME, Lobo MK. The molecular and cellular mechanisms of depression: a focus on reward circuitry. Mol Psychiatry. (2019) 24:1798–815. doi: 10.1038/s41380-019-0415-3
8. Liu L, Zhou X, Zhang Y, Pu J, Yang L, Yuan S, et al. Hippocampal metabolic differences implicate distinctions between physical and psychological stress in four rat models of depression. Transl Psychiatry. (2018) 8:4. doi: 10.1038/s41398-017-0018-1
9. Liu X, Zheng P, Zhao X, Zhang Y, Hu C, Li J, et al. Discovery and validation of plasma biomarkers for major depressive disorder classification based on liquid chromatography-mass spectrometry. J Proteome Res. (2015) 14:2322–30. doi: 10.1021/acs.jproteome.5b00144
10. Zheng P, Gao HC, Li Q, Shao WH, Zhang ML, Cheng K, et al. Plasma metabonomics as a novel diagnostic approach for major depressive disorder. J Proteome Res. (2012) 11:1741–8. doi: 10.1021/pr2010082
11. Zhou X, Liu L, Lan X, Cohen D, Zhang Y, Ravindran AV, et al. Polyunsaturated fatty acids metabolism, purine metabolism and inosine as potential independent diagnostic biomarkers for major depressive disorder in children and adolescents. Mol Psychiatry. (2019) 24:1478–88. doi: 10.1038/s41380-018-0047-z
12. Gadad BS, Jha MK, Grannemann BD, Mayes TL, Trivedi MH. Proteomics profiling reveals inflammatory biomarkers of antidepressant treatment response: findings from the CO-MED trial. J Psychiatr Res. (2017) 94:1–6. doi: 10.1016/j.jpsychires.2017.05.012
13. Lee MY, Kim EY, Kim SH, Cho K-C, Ha K, Kim KP, et al. Discovery of serum protein biomarkers in drug-free patients with major depressive disorder. Prog Neuropsychopharmacol Biol Psychiatry. (2016) 69:60–8. doi: 10.1016/j.pnpbp.2016.04.009
14. Roohi E, Jaafari N, Hashemian F. On inflammatory hypothesis of depression: what is the role of IL-6 in the middle of the chaos? J Neuroinflammation. (2021) 18:45. doi: 10.1186/s12974-021-02100-7
15. Ahmad SF, Ansari MA, Nadeem A, Bakheet SA, Alzahrani MZ, Alshammari MA, et al. Resveratrol attenuates pro-inflammatory cytokines and activation of JAK1-STAT3 in BTBR T(+) Itpr3(tf)/J autistic mice. Eur J Pharmacol. (2018) 829:70–8. doi: 10.1016/j.ejphar.2018.04.008
16. Nadeem A, Ahmad SF, Al-Harbi NO, Attia SM, Alshammari MA, Alzahrani KS, et al. Increased oxidative stress in the cerebellum and peripheral immune cells leads to exaggerated autism-like repetitive behavior due to deficiency of antioxidant response in BTBR T+tf/J mice. Prog Neuropsychopharmacol Biol Psychiatry. (2019) 89:245–53. doi: 10.1016/j.pnpbp.2018.09.012
17. Ahmad SF, Zoheir KM, Ansari MA, Korashy HM, Bakheet SA, Ashour AE, et al. Stimulation of the histamine 4 receptor with 4-methylhistamine modulates the effects of chronic stress on the Th1/Th2 cytokine balance. Immunobiology. (2015) 220:341–9. doi: 10.1016/j.imbio.2014.10.014
18. Bakheet SA, Ansari MA, Nadeem A, Attia SM, Alhoshani AR, Gul G, et al. CXCR3 antagonist AMG487 suppresses rheumatoid arthritis pathogenesis and progression by shifting the Th17/Treg cell balance. Cell Signal. (2019) 64:109395. doi: 10.1016/j.cellsig.2019.109395
19. Guo H, Ingolia NT, Weissman JS, Bartel DP. Mammalian microRNAs predominantly act to decrease target mRNA levels. Nature. (2010) 466:835–40. doi: 10.1038/nature09267
20. Bartel DP. MicroRNAs: genomics, biogenesis, mechanism, and function. Cell. (2004) 116:281–97. doi: 10.1016/S0092-8674(04)00045-5
21. Allen L, Dwivedi Y. MicroRNA mediators of early life stress vulnerability to depression and suicidal behavior. Mol Psychiatry. (2020) 25:308–20. doi: 10.1038/s41380-019-0597-8
22. Liu L, Wang H, Yu Y, Zeng B, Rao X, Chen J, et al. Microbial regulation of a lincRNA-miRNA-mRNA network in the mouse hippocampus. Epigenomics. (2020) 12:1377–87. doi: 10.2217/epi-2019-0307
23. Zhang H-P, Liu X-L, Chen J-J, Cheng K, Bai S-J, Zheng P, et al. Circulating microRNA 134 sheds light on the diagnosis of major depressive disorder. Transl Psychiatry. (2020) 10:95. doi: 10.1038/s41398-020-0773-2
24. Li C, Wang F, Miao P, Yan L, Liu S, Wang X, et al. miR-138 increases depressive-like behaviors by targeting SIRT1 in hippocampus. Neuropsychiatr Dis Treat. (2020) 16:949–7. doi: 10.2147/NDT.S237558
25. Agarwal V, Bell GW, Nam J-W, Bartel DP. Predicting effective microRNA target sites in mammalian mRNAs. eLife. (2015) 4:e05005. doi: 10.7554/eLife.05005
26. Karagkouni D, Paraskevopoulou MD, Chatzopoulos S, Vlachos IS, Tastsoglou S, Kanellos I, et al. DIANA-TarBase v8: a decade-long collection of experimentally supported miRNA-gene interactions. Nucleic Acids Res. (2018) 46:D239–D45. doi: 10.1093/nar/gkx1141
27. Wong N, Wang X. miRDB: an online resource for microRNA target prediction and functional annotations. Nucleic Acids Res. (2015) 43:D146–D52. doi: 10.1093/nar/gku1104
28. Chou CH, Shrestha S, Yang CD, Chang NW, Lin YL, Liao KW, et al. miRTarBase update 2018: a resource for experimentally validated microRNA-target interactions. Nucleic Acids Res. (2018) 46:D296–D302. doi: 10.1093/nar/gkx1067
29. Sticht C, De La Torre C, Parveen A, Gretz N. miRWalk: an online resource for prediction of microRNA binding sites. PLoS One. (2018) 13:e0206239. doi: 10.1371/journal.pone.0206239
30. Jansen R, Penninx BW, Madar V, Xia K, Milaneschi Y, Hottenga JJ, et al. Gene expression in major depressive disorder. Mol Psychiatry. (2016) 21:339–47. doi: 10.1038/mp.2015.57
31. Leday GGR, Vértes PE, Richardson S, Greene JR, Regan T, Khan S, et al. Replicable and coupled changes in innate and adaptive immune gene expression in two case-control studies of blood microarrays in major depressive disorder. Biol Psychiatry. (2018) 83:70–80. doi: 10.1016/j.biopsych.2017.01.021
32. Su G, Morris JH, Demchak B, Bader GD. Biological network exploration with Cytoscape 3. Curr Protoc Bioinformatics. (2014) 47:8.13.1–24. doi: 10.1002/0471250953.bi0813s47
33. Wang W, Wang T, Bai S, Chen Z, Qi X, Xie P. Dl-3-n-butylphthalide attenuates mouse behavioral deficits to chronic social defeat stress by regulating energy metabolism via AKT/CREB signaling pathway. Transl Psychiatry. (2020) 10:49. doi: 10.1038/s41398-020-0731-z
34. Benros ME, Waltoft BL, Nordentoft M, Ostergaard SD, Eaton WW, Krogh J, et al. Autoimmune diseases and severe infections as risk factors for mood disorders: a nationwide study. JAMA Psychiatry. (2013) 70:812–20. doi: 10.1001/jamapsychiatry.2013.1111
35. Haapakoski R, Mathieu J, Ebmeier KP, Alenius H, Kivimäki M. Cumulative meta-analysis of interleukins 6 and 1β, tumour necrosis factor α and C-reactive protein in patients with major depressive disorder. Brain Behav Immun. (2015) 49:206–15. doi: 10.1016/j.bbi.2015.06.001
36. Dickens C, McGowan L, Clark-Carter D, Creed F. Depression in rheumatoid arthritis: a systematic review of the literature with meta-analysis. Psychosom Med. (2002) 64:52–60. doi: 10.1097/00006842-200201000-00008
37. Korczak DJ, Pereira S, Koulajian K, Matejcek A, Giacca A. Type 1 diabetes mellitus and major depressive disorder: evidence for a biological link. Diabetologia. (2011) 54:2483–93. doi: 10.1007/s00125-011-2240-3
38. Ahmad SF, Ansari MA, Nadeem A, Alzahrani MZ, Bakheet SA, Attia SM. Resveratrol improves neuroimmune dysregulation through the inhibition of neuronal toll-like receptors and COX-2 signaling in BTBR T(+) Itpr3(tf)/J Mice. Neuromol Med. (2018) 20:133–46. doi: 10.1007/s12017-018-8483-0
39. Dahl J, Ormstad H, Aass HCD, Malt UF, Bendz LT, Sandvik L, et al. The plasma levels of various cytokines are increased during ongoing depression and are reduced to normal levels after recovery. Psychoneuroendocrinology. (2014) 45:77–86. doi: 10.1016/j.psyneuen.2014.03.019
40. Dowlati Y, Herrmann N, Swardfager W, Liu H, Sham L, Reim EK, et al. A meta-analysis of cytokines in major depression. Biol Psychiatry. (2010) 67:446–57. doi: 10.1016/j.biopsych.2009.09.033
41. Friebe A, Horn M, Schmidt F, Janssen G, Schmid-Wendtner MH, Volkenandt M, et al. Dose-dependent development of depressive symptoms during adjuvant interferon-{alpha} treatment of patients with malignant melanoma. Psychosomatics. (2010) 51:466–73. doi: 10.1176/appi.psy.51.6.466
42. Müller N, Schwarz MJ, Dehning S, Douhe A, Cerovecki A, Goldstein-Müller B, et al. The cyclooxygenase-2 inhibitor celecoxib has therapeutic effects in major depression: results of a double-blind, randomized, placebo controlled, add-on pilot study to reboxetine. Mol Psychiatry. (2006) 11:680–4. doi: 10.1038/sj.mp.4001805
43. Tyring S, Gottlieb A, Papp K, Gordon K, Leonardi C, Wang A, et al. Etanercept and clinical outcomes, fatigue, and depression in psoriasis: double-blind placebo-controlled randomised phase III trial. Lancet (London, England). (2006) 367:29–35. doi: 10.1016/S0140-6736(05)67763-X
44. Alboni S, Poggini S, Garofalo S, Milior G, El Hajj H, Lecours C, et al. Fluoxetine treatment affects the inflammatory response and microglial function according to the quality of the living environment. Brain Behav Immun. (2016) 58:261–71. doi: 10.1016/j.bbi.2016.07.155
45. Ramirez K, Sheridan JF. Antidepressant imipramine diminishes stress-induced inflammation in the periphery and central nervous system and related anxiety- and depressive- like behaviors. Brain Behav Immun. (2016) 57:293–303. doi: 10.1016/j.bbi.2016.05.008
46. Chen T, Huang Z, Wang L, Wang Y, Wu F, Meng S, et al. MicroRNA-125a-5p partly regulates the inflammatory response, lipid uptake, and ORP9 expression in oxLDL-stimulated monocyte/macrophages. Cardiovasc Res. (2009) 83:131–9. doi: 10.1093/cvr/cvp121
47. Wang M, Li Z, Zuo Q. miR-194-5p inhibits LPS-induced astrocytes activation by directly targeting neurexophilin 1. Mol Cell Biochem. (2020) 471:203–13. doi: 10.1007/s11010-020-03780-0
48. Sim MS, Soga T, Pandy V, Wu YS, Parhar IS, Mohamed Z. MicroRNA expression signature of methamphetamine use and addiction in the rat nucleus accumbens. Metab Brain Dis. (2017) 32:1767–83. doi: 10.1007/s11011-017-0061-x
49. Pfau ML, Menard C, Cathomas F, Desland F, Kana V, Chan KL, et al. Role of monocyte-derived microRNA106b~25 in resilience to social stress. Biol Psychiatry. (2019) 86:474–82. doi: 10.1016/j.biopsych.2019.02.023
50. Li JQ, Hu SY, Wang ZY, Lin J, Jian S, Dong YC, et al. Long non-coding RNA MEG3 inhibits microRNA-125a-5p expression and induces immune imbalance of Treg/Th17 in immune thrombocytopenic purpura. Biomed Pharmacother. (2016) 83:905–11. doi: 10.1016/j.biopha.2016.07.057
51. Bettelli E, Oukka M, Kuchroo VK. T(H)-17 cells in the circle of immunity and autoimmunity. Nat Immunol. (2007) 8:345–50. doi: 10.1038/ni0407-345
52. Kowalczyk M, Szemraj J, Blizniewska K, Maes M, Berk M, Su KP, et al. An immune gate of depression - Early neuroimmune development in the formation of the underlying depressive disorder. Pharmacol Rep. (2019) 71:1299–307. doi: 10.1016/j.pharep.2019.05.022
53. Slyepchenko A, Maes M, Köhler CA, Anderson G, Quevedo J, Alves GS, et al. T helper 17 cells may drive neuroprogression in major depressive disorder: proposal of an integrative model. Neurosci Biobehav Rev. (2016) 64:83–100. doi: 10.1016/j.neubiorev.2016.02.002
54. Grosse L, Hoogenboezem T, Ambrée O, Bellingrath S, Jörgens S, de Wit HJ, et al. Deficiencies of the T and natural killer cell system in major depressive disorder: T regulatory cell defects are associated with inflammatory monocyte activation. Brain Behav Immun. (2016) 54:38–44. doi: 10.1016/j.bbi.2015.12.003
55. Abd-Allah AR, Ahmad SF, Alrashidi I, Abdel-Hamied HE, Zoheir KM, Ashour AE, et al. Involvement of histamine 4 receptor in the pathogenesis and progression of rheumatoid arthritis. Int Immunol. (2014) 26:325–40. doi: 10.1093/intimm/dxt075
56. Hong M, Zheng J, Ding ZY, Chen JH, Yu L, Niu Y, et al. Imbalance between Th17 and Treg cells may play an important role in the development of chronic unpredictable mild stress-induced depression in mice. Neuroimmunomodulation. (2013) 20:39–50. doi: 10.1159/000343100
57. Ahmad SF, Zoheir KM, Ansari MA, Nadeem A, Bakheet SA, Al-Hoshani AR, et al. Histamine 4 receptor promotes expression of costimulatory B7.1/B7.2 molecules, CD28 signaling and cytokine production in stress-induced immune responses. J Neuroimmunol. (2015) 289:30–42. doi: 10.1016/j.jneuroim.2015.10.008
Keywords: depression, miRNAs, mRNAs, Th17 cell differentiation, CSDS
Citation: Wang H, Liu L, Chen X, Zhou C, Rao X, Li W, Li W, Liu Y, Fang L, Zhang H, Song J, Ji P and Xie P (2022) MicroRNA–Messenger RNA Regulatory Network Mediates Disrupted TH17 Cell Differentiation in Depression. Front. Psychiatry 13:824209. doi: 10.3389/fpsyt.2022.824209
Received: 29 November 2021; Accepted: 21 February 2022;
Published: 05 April 2022.
Edited by:
Hsiuying Wang, National Yang Ming Chiao Tung University, TaiwanReviewed by:
Sheikh Fayaz Ahmad, King Saud University, Saudi ArabiaZulqarnain Baloch, Kunming University of Science and Technology, China
Copyright © 2022 Wang, Liu, Chen, Zhou, Rao, Li, Li, Liu, Fang, Zhang, Song, Ji and Xie. This is an open-access article distributed under the terms of the Creative Commons Attribution License (CC BY). The use, distribution or reproduction in other forums is permitted, provided the original author(s) and the copyright owner(s) are credited and that the original publication in this journal is cited, in accordance with accepted academic practice. No use, distribution or reproduction is permitted which does not comply with these terms.
*Correspondence: Peng Xie, xiepeng@cqmu.edu.cn; Ping Ji, jiping@hospital.cqmu.edu.cn
†These authors have contributed equally to this work