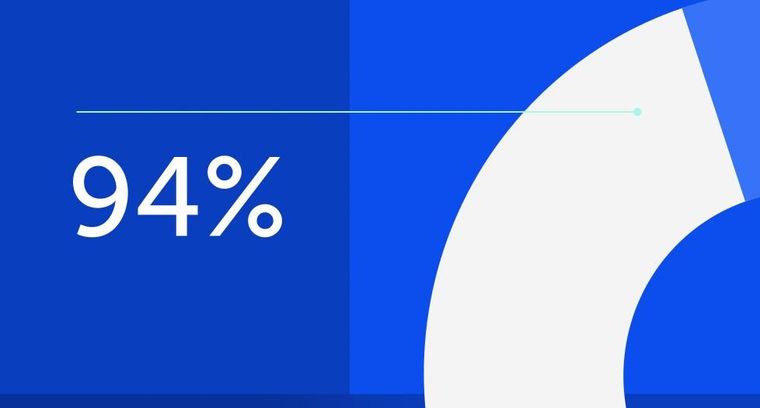
94% of researchers rate our articles as excellent or good
Learn more about the work of our research integrity team to safeguard the quality of each article we publish.
Find out more
ORIGINAL RESEARCH article
Front. Psychiatry, 11 August 2022
Sec. Computational Psychiatry
Volume 13 - 2022 | https://doi.org/10.3389/fpsyt.2022.811766
This article is part of the Research TopicWomen in Psychiatry 2021: Computational PsychiatryView all 7 articles
Impaired cognitive function related to intrusive memories of traumatic experiences is the most noticeable characteristic of post-traumatic stress disorder (PTSD); nevertheless, the brain mechanism involved in the cognitive processing is still elusive. To improve the understanding of the neuropathology in PTSD patients, we investigated functional cortical networks that are based on graph theory, by using electroencephalogram (EEG). EEG signals, elicited by an auditory oddball paradigm, were recorded from 53 PTSD patients and 39 healthy controls (HCs). Source signals in 68 regions of interests were estimated using EEG data for each subject using minimum-norm estimation. Then, using source signals of each subject, time-frequency analysis was conducted, and a functional connectivity matrix was constructed using the imaginary part of coherence, which was used to evaluate three global-level (strength, clustering coefficient, and path length) and two nodal-level (strength and clustering coefficients) network indices in four frequency bands (theta, alpha, low-beta, and high-beta). The relationships between the network indices and symptoms were evaluated using Pearson’s correlation. Compared with HCs, PTSD patients showed significantly reduced spectral powers around P300 periods and significantly altered network indices (diminished strength and clustering coefficient, and prolonged path length) in theta frequency band. In addition, the nodal strengths and nodal clustering coefficients in theta band of PTSD patients were significantly reduced, compared with those of HCs, and the reduced nodal clustering coefficients in parieto-temporo-occipital regions had negative correlations with the symptom scores (Impact of Event Scale-Revises, Beck Depression Inventory, and Beck Anxiety Inventory). The characterization of this disrupted pattern improves the understanding of the neuropathophysiology underlying the impaired cognitive function in PTSD patients.
Patients with post-traumatic stress disorder (PTSD) generally suffer from recurrent recollection of distressing memories related to terrifying events (intrusive memory) and spontaneously tend to concentrate on negative thinking (rumination) biased toward traumatic events (1–5). Both these symptoms are distinctly presented only in PTSD patients. Due to the distinct symptoms of intrusive memory and rumination, PTSD patients show attention bias toward the traumatic events. Consequently, these patients develop cognitive impairments (4); they show lower performance in cognitive tasks, such as working memory, than healthy controls (HCs) (1, 5). Moreover, the impaired cognitive function in PTSD patients has been observed in objective physiological traits investigated using various functional neuroimaging modalities (2, 3, 6, 7).
Functional magnetic resonance imaging (fMRI) studies have investigated the altered cognitive function in PTSD patients, especially focusing on two regions of interests (ROIs), namely emotion-related areas (e.g., amygdala, insula, ventral anterior cingulate (ACC), and rostral ACC) and cognition-related areas (e.g., hippocampus and prefrontal regions, including dorsal ACC) (6, 8–10). In a previous study, when performing cognitive tasks via neutral stimuli excluding emotional information, the HCs showed significantly enhanced brain activation in cognition-related areas (11), whereas emotion-related areas were not significantly activated (12), meaning that the HCs appropriately allocated neuronal resources solely to cognition-related areas to process the cognitive tasks. However, PTSD patients display significantly enhanced activation in both emotion- and cognition-related areas, unlike healthy individuals (8), and the increased brain activation in emotion-related areas has a positive correlation with the hyper-arousal symptom of PTSD patients (8). These results indicate that PTSD patients allocate neuronal resources to not only cognition-related areas but also emotion-related areas even if emotional information is not involved in the cognitive tasks. In other words, in PTSD patients, neuronal resources that should be solely allocated to cognition-related areas are partially distributed to emotion-related areas when processing cognition-related information, leading to cognitive impairments.
Although previous fMRI studies have discovered the distinct regional information related to the impaired cognitive function in PTSD patients, it is not sufficient to fully understand the neuropathological characteristics, which has a resolution of several seconds, using fMRI because the cognitive function is processed very fast, within milliseconds (13, 14). To investigate the fast cognition-related neuronal processing, electroencephalogram (EEG) has been widely used due to its high temporal resolution (15), and the altered cognitive function in PTSD patients was revealed via EEG-based neurophysiological markers in terms of functional aspects (16). In particular, PTSD patients show a reduced amplitude of P300 event-related potential (ERP) and prolonged P300 latency than healthy individuals when performing cognitive oddball tasks, indicating that PTSD patients cannot allocate the appropriate amount of brain resources when processing cognition-related tasks (17, 18). Moreover, disrupted P300 characteristics have negative relationships with the psychiatric symptoms, such as avoidance and numbing, in PTSD patients (17). Thus, the distinct psychiatric symptoms of PTSD presumably lead to the cognitive decline (17). However, since various brain regions interact with each other to process cognitive information, simple ERP-based traits, such as peak amplitudes and latencies, are insufficient to comprehend the complex brain processing associated with cognitive function in PTSD patients (19). Therefore, brain network indices based on functional connectomes can be an appropriate alternative to improve the understanding of the neuronal mechanisms underlying complicated cognitive functions.
Indeed, several previous studies have shown the possibility of using brain network analysis based on graph theory to investigate the altered cognitive functions in other mental disorders (20–22). For example, when utilizing cognitive functions, schizophrenia patient’s show altered functional networks relative to those of healthy individuals, and the disrupted brain network traits in these patients are significantly correlated with the psychotic symptoms (20). Despite the promising results derived from the EEG-based network analyses, to the best of our knowledge, network analysis has not yet been applied to the EEG data from PTSD patients to investigate the dynamic disease-specific cognitive neural information.
In this study, we investigated the cortical network characteristics of PTSD patients by using EEG data measured during a simple cognitive task based on neutral auditory stimulation, to extend the understanding of PTSD neurophysiopathology in terms of the brain network. To this end, we computed weighted network indices (strength, clustering coefficient, and path length) by using cortical-level time series. Moreover, we explored the relationships between the network indices and clinical symptoms, thereby contributing to the understanding of the cognition-related neuropathology of PTSD.
Fifty-three PTSD patients and 39 HCs were recruited for this study from the Psychiatry Department of Inje University Ilsan Paik Hospital. The patients had been diagnosed by a board-certified psychiatrist according to the Diagnostic and Statistical Manual of Mental Disorders, 4th edition (DSM-IV) Axis I Psychiatric Disorders. Patients were excluded if they had any of the followings: (1) abnormality of the central nervous system, (2) medical history of alcohol or drug abuse, (3) intellectual disability, (4) history of a head injury that resulted in loss of consciousness and experience of electrical therapy (e.g., electroconvulsive therapy), and (5) history of a psychotic symptom lasting for ≥24 h. For HCs, individuals without any psychiatric medical history were recruited from the local community through advertisements in local newspapers and posters. If the HCs had taken any psychotropic medication and, they were excluded from the study. This study protocol was approved by the Institutional Review Board of Inje University Ilsan Paik Hospital (2015-07-025) and conducted in accordance with The Code of Ethics of the World Medical Association (Declaration of Helsinki), and all the participants provided written informed consent.
Two Psychometric tests were performed to check the psychiatric severity of the PTSD patients and HCs as well as to investigate the relationship between the psychiatric severity and cortical network indices. Beck Anxiety Inventory (BAI) (23) was used to evaluate the anxiety symptom (mild, 8–15; moderate, 16–25; and severe, 26–63) (24). Beck Depression Inventory (BDI) (25) was used to evaluate the depressive symptom (mild, 14–19; moderate: 20–28; and severe: 29–63) (26). These two psychiatric symptoms were evaluated for both PTSD patients and HCs. Additionally, Impact of Event Scale-Revises (IES-R) (27) was used to evaluate the responses to the traumatic events experienced by the PTSD patients (mild, 0–24; moderate, 25–39; severe, 40–59; and very severe, ≥ 60) (28, 29). The PTSD patients experienced the following traumatic events: severe motor-vehicle accidents (n = 46, 86.02%), physical and sexual abuse (n = 2, 3.77%), and other accidents, such as being inside a collapsing building and being trapped in an elevator (n = 5, 9.43%). The demographic data and psychiatric severity scores (BAI, BDI, and IES-R) of each group are reported in Table 1.
Table 1. Demographic data of the post-traumatic stress disorder (PTSD) patients and healthy controls (HCs).
The stimuli used for the auditory oddball paradigm were composed of target and standard tones with the tone frequencies of 1,500 and 1,000 Hz, respectively. The duration of each stimulus was set to 100 ms, and the rising and falling times were equally set to 10 ms. Four-hundred pure tone stimuli consisting of 15% target tones and 85% standard tones were presented in a random order with an inter-stimulus interval of 1,500 ms. The participants were required to press a response button whenever target tones were presented to check their attention to the target auditory stimuli.
Electroencephalogram data were recorded with a 1–100 Hz band-pass filter at a sampling rate of 1,000 Hz from 64 Ag/AgCl scalp electrodes evenly mounted according to the extended international 10–20 system [NeuroScan SynAmps2 (Compumedics USA, El Paso, TX, United States); references, both mastoids]. Since mastoid-based references may cause distorted and spurious connections due to unwanted artifacts introduced outside of the brain when computing functional connectivity, re-referencing was conducted using common average reference (CAR). Eye-movement artifacts were removed using established mathematical procedures (30) based on the regression approach implemented in NeuroScan preprocessing software, and other gross artifacts were eliminated via visual inspection. Artifact-free EEG data were bandpass filtered using third Butterworth IIF filter with a forward-backward zero phase filtering process between 1 and 55 Hz and epoched between −1 and 1 s, based on the stimuli onset, and only target epochs were used for further analysis. The epochs were rejected if they contained significant physiological artifacts (± 100 μV) at any electrode (31). After performing the preprocessing steps, the number of remaining epochs were 59 ± 2.08 for PTSD patients and 59.05 ± 2.70 for HCs (p = 0.918), respectively, among all 60 target epochs. All other preprocessing steps, except EOG removal, were conducted using Matlab 2019b (MathWorks).
A lead-field matrix was computed using a three-layer (inner skull, outer skull, and scalp) boundary element method (BEM) model which was constructed from the standard head model (Colin 27) by using the Open MEEG toolbox (32). Note that to estimate accurate source signals, brain areas on cerebral cortex were only used to construct BEM model, excluding deep brain areas such as hippocampus and amygdala. An inverse operator was created using a weighted minimum-norm estimation (wMNE) algorithm implemented in the Brainstorm toolbox (33). A time series of source activities at 15,000 cortical vertices was estimated for each epoch. After computing the source-level time-series at each vertex, the representative source signals at 68 ROIs based on Desikan-Kiliany atlas were estimated using principal component analysis (34). The name of 68 ROIs is summarized in Supplementary Table 1. After computing source signals, time frequency analysis were performed to investigate whether accurate source signals were estimated, for which Fast Fourier Transform (FFT) with a single Hanning taper (three cycles long with respect to frequency bins) was performed for each trial (−200 to 1,000 ms based on the stimuli onset) to compute spectral powers. The power spectrum of each trial was then baseline normalized by subtracting the average power of the baseline period (−200 to 0 ms) from spectral powers of the task period (0 to 1,000 ms) and then the differences were divided by the average power of the baseline period to probe for changes in spectral powers before and after stimulus onset. The baseline normalized power spectra were then averaged over all trials, which resulted in baseline-normalized total power. Since the data length (1 s for task period) is too short to sufficiently cover the oscillatory activities in delta band, this band was excluded from further analysis (35, 36). Therefore, both total power and network analysis were conducted for four frequency bands [theta (4–8 Hz), alpha (8–12 Hz), low-beta (12–22 Hz), and high-beta (22–30 Hz)], respectively.
Functional connectivity analysis was performed for network analysis; the imaginary part of coherence (iCoh), which implemented in FieldTrip toolbox, was computed using a time-series of the cortical activities at each ROI. iCoh was quantified based on spectral powers obtained using FFT with a single Hanning taper (three cycles long with respect to frequency bins). An iCoh matrix was evaluated between all possible pairs of 68 ROIs and quantified for four frequency bands. An iCoh matrix was independently computed for two time periods, as follows: baseline (−1 to 0 s) and task period (0 to 1 s), and the task-based iCohs were normalized by subtracting the baseline iCohs to extract the network indices directly related to the cognitive task. After the normalization processing, two different adjacency matrices were quantified as task-specific enhanced and diminished connectomes, indicating increased (positive) and decreased (negative) connectivity’s when performing the cognitive task, respectively, compared with the baseline period. Because use of both positive and negative connectomes can improve understanding of neural mechanisms related to cognitive processing for a complementary way, both positive and negative connectomes were independently analyzed, respectively. In this study, an original adjacency matrix was used to quantify network indices without any threshold to minimize information loss between all possible ROI pairs.
Two different types of weighted brain network indices were calculated based on graph theory for each of the positive and negative adjacency matrices. The first type consisted of the following three global-level network indices that take into account the whole-brain cortex: (1) strength (the degree of connection strength in the network); (2) clustering coefficient (the degree of functional segregation in the network, meaning the clustering degree between neighboring nodes); and (3) path length (PL, the degree of functional integration in the network, representing the overall connectedness of the whole network) (37). The second type consisted of two nodal (ROI)-level network indices that focus on the characteristics of individual brain regions—(1) strength and (2) clustering coefficient. All the network indices were computed using Brain connectivity Toolbox (BCT1) based on Matlab (38).
Statistical tests were performed to investigate the significance of the difference between two groups for the total power and network analysis results independently. For multidimensional data (e.g., spectral power–4 ROIs × 1,201 time points × 27 frequency bins; nodal-level network indices–68 ROIs × 4 frequency bands), a cluster-based permutation test, implemented in Fieldtrip, was performed for correction of multiple comparisons (39). To this end, an independent t-test was conducted for each data point (e.g., spectral powers–for each ROI and each time and each frequency point), and clusters were identified based on two or more adjacent data points corresponding to t-values with a p-value less than 0.05. Then, a maximum t-value of each cluster was calculated by summing of all t-values within each cluster, and significant clusters were estimated by Monte Carlo method with 1,000 random permutations (p < 0.025, two-tailed). For the single-dimensional data, global-level network indices, a critical alpha level of 0.01 was set to correct the multiple comparison issue caused by four frequency bands, which is less than Bonferroni corrected alpha value (0.05/4 frequency bands = 0.0125). In addition, to investigate the relationships between the network indices of both global-and nodal-level network values and psychiatric-symptom scores of the PTSD patients and HCs, respectively, the Pearson’s correlation method was used with a permutation of 10,000 iterations (40, 41).
Figure 1 represents total power pattern maps quantified for four different brain lobes (frontal, parietal, temporal, and occipital) by averaging all subjects of each group and ROIs included in each lobe for each frequency band. PTSD patients showed the significantly reduced cortical spectral powers in theta frequency band around 300 ms for all four lobes as compared to those of HCs (cluster-p = 0.020).
Figure 1. Averaged total power patterns of four lobes [frontal (A), parietal (B), temporal (C), and occipital lobes (D)] across all subjects and region of interests (ROIs) included in each lobe for post-traumatic stress disorder (PTSD) patients and healthy controls (HCs), respectively, and the total power differences between PTSD patients and HCs.
For global-level network indices, the significant differences between the PTSD patients and HCs were observed when using only the task-specific positive network indices, but there was no significant difference in the task-specific negative network indices between the two groups. Figure 2 represents all statistical results of global-level network indices for both positive and negative networks, regardless of their significances. For the global-level network, the PTSD patients showed reduced strength and clustering coefficient, but increased path length in theta frequency band (p < 0.01), compared with those of the HCs. Tables 2, 3 summarize all statistical results (e.g., mean and standard deviation, p-value, t-value, degree of freedom, and effect size) for the task-specific both positive and negative networks at global-level, regardless of their statistical significances, respectively.
Figure 2. Comparison of the global-level network indices (strength, clustering coefficient, and path length) in all frequency bands for each positive and negative network between the post-traumatic stress disorder (PTSD) patients and healthy controls (HCs). PTSD, post-traumatic stress disorder; HCs, healthy controls. *p < 0.01.
Table 2. All statistic results of global-level network indices for positive network between two groups.
Table 3. All statistic results of global-level network indices for negative network between two groups.
Significant differences of nodal-level network indices between the PTSD patients and HCs were revealed only in the task-specific positive network. For the positive network, PTSD patients showed the significantly reduced nodal strengths (cluster-p = 0.018) and nodal clustering coefficients (cluster-p = 0.005), predominantly located in parieto-temporo-occipital regions, in theta frequency band compared to those of HCs. On the other hand, there was no significant difference between PTSD patients and HCs in task-specific negative network. Figure 3 depicts the statistical results of nodal-level network indices based on t-values. Supplementary Table 2 reports all statistical results (e.g., mean and standard deviation, t-value, and effect size) of nodal-level network indices between the two groups.
Figure 3. Comparison of the nodal network indices (strength and clustering coefficient) between the post-traumatic stress disorder (PTSD) patients and healthy controls (HCs) in all frequency bands for each positive and negative network. Spatial distribution is described using normalized t-values (range: –1 to 1) between two groups for each nodal network index and each frequency band. Blue color means the significantly reduced nodal network values in PTSD patients compared to HCs.
Correlation analysis was conducted for all network indices of global-and nodal-level for both positive and negative networks. Note that the correlation analysis was performed for both PTSD patients and HCs independently, but significant relationships were observed only in PTSD patients. In the case of the global-level, there was no significant relationship between the symptom scores and network indices. For the nodal-level, significant relationships were revealed only in the positive network. In the theta frequency band, significantly negative correlations were observed between the nodal clustering coefficients of five brain regions (left superior temporal, left supari marginal, left and right lingual, and right isthmus cingulate) and IES-R. Moreover, the clustering coefficients of left superior temporal were correlated with depression (BDI) and anxiety symptom scores (BAI), respectively (Figure 4).
Figure 4. Relationships between the symptom scores [Impact Event Scale-Revised (IES-R), Back’s Depression Inventory (BDI), and Back’s Anxiety Inventory (BAI)] and nodal clustering coefficient in theta frequency band in the post-traumatic stress disorder (PTSD) patients. The clustering coefficient of each patient has significantly negative correlations with symptom scores.
In this study, we investigated the altered cortical network characteristics related to the declined cognitive function of PTSD patients by using EEG recorded during performing a simple auditory cognitive task. The PTSD patients showed diminished spectral powers around P300 periods and disrupted network characteristics (reduced strength and clustering coefficient, and prolonged path length) in theta frequency band, compared with those of the HCs. Moreover, the PTSD patients displayed disrupted network indices in specific brain regions for task-specific positive networks. The nodal strengths and nodal clustering coefficients of PTSD patients were significantly reduced in theta frequency band compared with those of the HCs. We also observed the significant relationships between the nodal clustering coefficients of the task-specific positive network and psychiatric symptom scores (IES-R, BDI, and BAI) in theta band. The distinct brain network characteristics associated with the psychiatric symptoms of PTSD patients can provide neurophysiologically meaningful information to improve the understanding of the neuropathology related to PTSD.
Interestingly, in this study, distinct disease-specific network patterns were revealed when using only task-specific enhanced connectomes. It is obvious that, compared with the baseline, significantly enhanced functional connections were observed during cognitive processing, but no diminished connection was detected (42, 43). Therefore, most previous studies, which have investigated the neuronal characteristics of psychiatric disorders, have focused on investigating the enhanced connectomes during cognitive processing compared with the baseline rather than investigating the diminished connectomes (44, 45). For example, schizophrenia patients showed significantly fewer enhanced connections than healthy individuals when processing cognitive tasks (44). Additionally, these patients are inefficient in creating the enhanced connections needed to appropriately process cognitive tasks, leading to the impaired cognitive function. Therefore, our study is in line with the results from previous studies in that the significant results were only observed when using the enhanced (positive) connectomes. Moreover, since there has been no study investigating the disrupted enhanced connections in PTSD patients during processing cognitive tasks, our study provides a better understanding of the pathology of the impaired cognitive function in PTSD patients by investigating the functional network indices based on task-specific increased connectomes.
To date, most studies have investigated the impaired cognitive function in PTSD patients by using simple ERP characteristics, such as reduced amplitudes and prolonged latencies, which can provide neurophysiologically meaningful information (e.g., how fewer brain resources are allocated to cognitive processing or how slow processing speed is in PTSD patients) (46). However, such fragmentary information obtained from simple time-series ERP traits would be insufficient to fully comprehend the neuronal mechanisms involved in the cognitive function occurring during complex systematic interactions based on the network structure (19). Moreover, simple ERP studies tend to overlook the frequency-domain information that can represent the impaired cognition-related psychopathology of PTSD. For example, it has been well documented that PTSD patients show altered theta patterns during the resting-state even though neurophysiological findings are still controversial [enhanced (47) vs. diminished (48, 49) theta power]. Nevertheless, a reliable consensus has been reached that the theta oscillation is closely related to cognitive functions, such as attention and memory processing (50–53). Therefore, insightful information can be obtained by investigating the unique network characteristics along with frequency-domain information to have a better understanding of the impaired cognitive mechanism in PTSD patients. Indeed, in this study, the PTSD patients showed abnormal theta frequency information in terms of both total power (reduced spectral power) and network indices (weaker strength, lower clustering coefficient, and prolonged path length) covering the whole-brain during simple attention processing, as compared with those of the HCs. In line with previous studies, PTSD patients cannot allocate enough brain resource to boost spectral power of theta frequency band during task as compared to HCs. Moreover, the weaker strength and lower clustering coefficient indicate that the connections between specific brain regions are loose, and the prolonged path length indicates that the processing speed is slower in PTSD patients than in HCs. Consequently, this impairment in brain networks hinder the appropriate cognitive processing, leading to the impaired cognitive function in PTSD patients.
In terms of brain regional view, the PTSD patients showed significantly reduced nodal clustering coefficients of task-specific positive network in theta frequency band, dominantly located in parieto-temporo-occipital regions such as superior temporal, parahippocampal gyrus, lingual gyrus, etc., compared with those of the HCs. Particularly, temporal regions are generally involved in cognition-related information processing, especially memory processing (54). In addition, the parahippocampal gyrus is known to be a bridge region in the information-processing pathway from the temporal areas to the hippocampus regarding the memory process (encoding and retrieval) (51). Accordingly, the diminished theta clustering coefficients of the PTSD patients in those of brain regions implied that both the bridge of the memory pathway and the direct memory related regions do not properly work in PTSD patients, finally resulting in the impaired cognitive function. Furthermore, negative correlations were revealed between the nodal clustering coefficients in several regions (isthmus cingulate, lingual, superior temporal, and supra marginal) in theta frequency band and the symptom scores (IES-R for the degrees of stress from the trauma-related events, BDI for depression symptoms, and BAI for anxiety), respectively. The correlation results imply that nodal clustering coefficients of the brain regions get lower as the degree of psychiatric symptoms increases. These results indicate that parieto-temporo-occipital network is more altered in severe PTSD patients as compared to those of relatively intact patients, leading to poorer attention processing of severe PTSD patients.
There are three main limitations to the present study. First, since all recruited PTSD patients were on medication, we could not control the compounding effects from medications. Second, even though we controlled for other psychiatric illness, we did not control for comorbid depression. Third, although demographic data were statistically matched between two groups, the number of participants was unbalanced between two participant groups.
Although the impaired cognitive function is accounted as an important symptom in PTSD patients, the related brain networks have not been investigated before. We first attempted to explore the brain networks related to impaired cognitive function in PTSD patients by using a simple auditory oddball task and finally identified the modified brain-network characteristics in PTSD patients compared with HCs. PTSD patients showed disrupted whole-brain network indices (lower strength and clustering coefficient and prolonged path length) in theta frequency band, compared with those of the HCs. Moreover, diminished nodal clustering coefficients in theta band were discovered in parieto-temporo-occipital regions, and decreased nodal clustering coefficients of the parieto-temporo-occipital regions were found to have significant relationships with the psychiatric symptom scores. The presented results enhance the understanding of the impaired cognitive pathology in PTSD patients by revealing the altered brain-networks characteristics and thereby present the network indices as promising biomarkers of PTSD.
The raw data supporting the conclusions of this article will be made available by the authors, without undue reservation.
The study involving human participants was reviewed and approved by the Institutional Review Board of Inje University Ilsan Paik Hospital (2015-07-025). The patients/participants provided their written informed consent to participate in this study.
MS, H-JH, and S-HL: conception/design of the work, interpretation of data, and writing (original draft preparation and editing). MS and S-HL: data acquisition and analysis. All authors read and contributed to the final version of the manuscript.
This work was supported by a Korea University Grant, a grant from the Institute for Information & Communications Technology Planning & Evaluation (IITP) funded by the Korean government (No. 2017-0-00451; Development of BCI based Brain and Cognitive Computing Technology for Recognizing User’s Intentions using Deep Learning), Basic Science Research Program through the National Research Foundation of Korea (NRF) funded by the Ministry of Education (NRF-2020R1A4A1017775 and NRF-2019R1I1A1A01063313), a grant from the Korea Medical Device Development Fund funded by the Korea government (the Ministry of Science and ICT; the Ministry of Trade, Industry, and Energy; the Ministry of Health and Welfare; and the Ministry of Food and Drug Safety) (1711138348, KMDF_PR_20200901_0169), and the Brain Research Program through the National Research Foundation of Korea (NRF) funded by the Ministry of Science, ICT, and Future Planning (NRF-2015M3C7A1028252).
The authors declare that the research was conducted in the absence of any commercial or financial relationships that could be construed as a potential conflict of interest.
All claims expressed in this article are solely those of the authors and do not necessarily represent those of their affiliated organizations, or those of the publisher, the editors and the reviewers. Any product that may be evaluated in this article, or claim that may be made by its manufacturer, is not guaranteed or endorsed by the publisher.
The Supplementary Material for this article can be found online at: https://www.frontiersin.org/articles/10.3389/fpsyt.2022.811766/full#supplementary-material
1. Cassiday KL, Mcnally RJ, Zeitlin SB. Cognitive processing of trauma cues in rape victims with post-traumatic stress disorder. Cognit Ther Res. (1992) 16:283–95. doi: 10.1037//0022-006x.67.5.705
2. Francati V, Vermetten E, Bremner J. Functional neuroimaging studies in posttraumatic stress disorder: Review of current methods and findings. Depress Anxiety. (2007) 24:202–18.
3. Hughes KC, Shin LM. Functional neuroimaging studies of post-traumatic stress disorder. Expert Rev Neurother. (2011) 11:275–85.
4. Bomyea J, Johnson A, Lang AJ. Information processing in PTSD: Evidence for biased attentional, interpretation, and memory processes. Psychopathol Rev. (2017) 4:218–43. doi: 10.3389/fnint.2012.00089
5. Nejati V, Salehinejad MA, Sabayee A. Impaired working memory updating affects memory for emotional and non-emotional materials the same way: Evidence from post-traumatic stress disorder (PTSD). Cogn Process. (2018) 19:53–62. doi: 10.1007/s10339-017-0837-2
6. Shin LM, Rauch SL, Pitman RK. Amygdala, medial prefrontal cortex, and hippocampal function in PTSD. Ann N Y Acad Sci. (2006) 1071:67–79.
7. Yun J-Y, Jin MJ, Kim S, Lee S-H. Stress-related cognitive style is related to volumetric change of the hippocampus and FK506 binding protein 5 polymorphism in post-traumatic stress disorder. Psychol Med. (2020) 52:1243–54. doi: 10.1017/S0033291720002949
8. Bryant RA, Felmingham KL, Kemp AH, Barton M, Peduto AS, Rennie C, et al. Neural networks of information processing in posttraumatic stress disorder: A functional magnetic resonance imaging study. Biol Psychiatry. (2005) 58:111–8.
9. Morey RA, Dolcos F, Petty CM, Cooper DA, Hayes JP, Labar KS, et al. The role of trauma-related distractors on neural systems for working memory and emotion processing in posttraumatic stress disorder. J Psychiatr Res. (2009) 43:809–17. doi: 10.1016/j.jpsychires.2008.10.014
10. Aupperle RL, Melrose AJ, Stein MB, Paulus MP. Executive function and PTSD: Disengaging from trauma. Neuropharmacology. (2012) 62:686–94.
11. D’Esposito M, Postle BR, Rypma B. Prefrontal cortical contributions to working memory: Evidence from event-related fMRI studies. Executive control and the frontal lobe. Curr Issues. (2000) 133:3–11. doi: 10.1006/nimg.2001.0936
12. West HV, Burgess GC, Dust J, Kandala S, Barch DM. Amygdala activation in cognitive task fMRI varies with individual differences in cognitive traits. Cogn Affect Behav Neurosci. (2021) 21:254–64. doi: 10.3758/s13415-021-00863-3
13. Polich J. Updating P300: An integrative theory of P3a and P3b. Clin. Neurophysiol. (2007) 118:2128–48. doi: 10.1016/j.clinph.2007.04.019
14. Srinivasan N. Cognitive neuroscience of creativity: EEG based approaches. Methods. (2007) 42:109–16.
15. Beres AM. Time is of the essence: A review of electroencephalography (EEG) and event-related brain potentials (ERPs) in language research. Appl Psychophysiol Biofeedback. (2017) 42:247–55. doi: 10.1007/s10484-017-9371-3
16. Min D, Kwon A, Kim Y, Jin MJ, Kim Y-W, Jeon H, et al. Clinical implication of altered inhibitory response in patients with post-traumatic stress disorder: Electrophysiological evidence from a Go/Nogo task. Brain Topogr. (2020) 33:208–20. doi: 10.1007/s10548-020-00754-9
17. Felmingham KL, Bryant RA, Kendall C, Gordon E. Event-related potential dysfunction in posttraumatic stress disorder: The role of numbing. Psychiatry Res. (2002) 109:171–9. doi: 10.1016/s0165-1781(02)00003-3
18. Araki T, Kasai K, Yamasue H, Kato N, Kudo N, Ohtani T, et al. Association between lower P300 amplitude and smaller anterior cingulate cortex volume in patients with posttraumatic stress disorder: A study of victims of Tokyo subway sarin attack. Neuroimage. (2005) 25:43–50. doi: 10.1016/j.neuroimage.2004.11.039
19. Braun U, Schäfer A, Walter H, Erk S, Romanczuk-Seiferth N, Haddad L, et al. Dynamic reconfiguration of frontal brain networks during executive cognition in humans. Proc Natl Acad Sci USA. (2015) 112:11678–83.
20. Shim M, Kim D-W, Lee S-H, Im C-H. Disruptions in small-world cortical functional connectivity network during an auditory oddball paradigm task in patients with schizophrenia. Schizophr Res. (2014) 156:197–203. doi: 10.1016/j.schres.2014.04.012
21. Rommel A-S, Kitsune GL, Michelini G, Hosang GM, Asherson P, Mcloughlin G, et al. Commonalities in EEG spectral power abnormalities between women with ADHD and women with bipolar disorder during rest and cognitive performance. Brain Topogr. (2016) 29:856–66. doi: 10.1007/s10548-016-0508-0
22. Kazemi R, Rostami R, Khomami S, Baghdadi G, Rezaei M, Hata M, et al. Bilateral transcranial magnetic stimulation on DLPFC changes resting state networks and cognitive function in patients with bipolar depression. Front Hum Neurosci. (2018) 12:356. doi: 10.3389/fnhum.2018.00356
23. Beck AT, Steer RA. Manual for the Beck anxiety inventory. San Antonio, TX: Psychological Corporation (1990).
24. Yook S, Kim Z. A clinical study on the Korean version of Beck anxiety inventory: Comparative study of patient and non-patient. Korean J Clin Psychol. (1997) 16:185–97.
25. Beck AT, Steer RA, Brown GK. Beck depression inventory-II. San Antonio, TX: Psychological Corporation (1996). p. 78204–72498.
26. Cha JM, Kim JE, Kim MA, Shim B, Cha MJ, Lee JJ, et al. Five months follow-up study of school-based crisis intervention for Korean high school students who experienced a peer suicide. J Korean Med Sci. (2018) 33:e192. doi: 10.3346/jkms.2018.33.e192
27. Weiss DS. The impact of event scale: Revised. In: Wilson JP, Tang CS editors. Cross-cultural assessment of psychological trauma and PTSD. (Boston, MA: Springer) (2007). p. 219–38. doi: 10.3390/jpm12050681
28. Lim H-K, Woo J-M, Kim T-S, Kim T-H, Choi K-S, Chung S-K, et al. Reliability and validity of the Korean version of the impact of event scale-revised. Compr Psychiatry. (2009) 50:385–90.
29. Sohn JH, Lim J, Lee JS, Kim K, Lim S, Byeon N, et al. Prevalence of possible depression and post-traumatic stress disorder among community dwelling adult refugees and refugee applicants in South Korea. J Korean Med Sci. (2019) 34:e97. doi: 10.3346/jkms.2019.34.e97
30. Semlitsch HV, Anderer P, Schuster P, Presslich O. A solution for reliable and valid reduction of ocular artifacts, applied to the P300 ERP. Psychophysiology. (1986) 23:695–703. doi: 10.1111/j.1469-8986.1986.tb00696.x
31. Kim J-Y, Son J-B, Leem H-S, Lee S-H. Psychophysiological alteration after virtual reality experiences using smartphone-assisted head mount displays: An EEG-based source localization study. Appl Sci. (2019) 9:2501.
32. Gramfort A, Papadopoulo T, Olivi E, Clerc M. OpenMEEG: Opensource software for quasistatic bioelectromagnetics. Biomed Eng Online. (2010) 9:45. doi: 10.1186/1475-925X-9-45
33. Tadel F, Baillet S, Mosher JC, Pantazis D, Leahy RM. Brainstorm: A user-friendly application for MEG/EEG analysis. Comput Intell Neurosci. (2011) 2011:879716. doi: 10.1155/2011/879716
34. Dimitriadis SI, López ME, Bruña R, Cuesta P, Marcos A, Maestú F, et al. How to build a functional connectomic biomarker for mild cognitive impairment from source reconstructed MEG resting-state activity: The combination of ROI representation and connectivity estimator matters. Front Neurosci. (2018) 12:306. doi: 10.3389/fnins.2018.00306
35. Fraschini M, Demuru M, Crobe A, Marrosu F, Stam CJ, Hillebrand A. The effect of epoch length on estimated EEG functional connectivity and brain network organisation. J Neural Eng. (2016) 13:036015. doi: 10.1088/1741-2560/13/3/036015
36. Tóth B, Urbán G, Haden GP, Márk M, Török M, Stam CJ, et al. Large-scale network organization of EEG functional connectivity in newborn infants. Hum Brain Mapp. (2017) 38:4019–33.
37. Shim M, Im C-H, Lee S-H. Disrupted cortical brain network in post-traumatic stress disorder patients: A resting-state electroencephalographic study. Transl Psychiatry. (2017) 7:e1231. doi: 10.1038/tp.2017.200
38. Rubinov M, Sporns O. Complex network measures of brain connectivity: Uses and interpretations. Neuroimage. (2010) 52:1059–69.
39. Maris E, Oostenveld R. Nonparametric statistical testing of EEG-and MEG-data. J Neurosci Methods. (2007) 164:177–90.
40. Groppe DM, Urbach TP, Kutas M. Mass univariate analysis of event-related brain potentials/fields I: A critical tutorial review. Psychophysiology. (2011) 48:1711–25. doi: 10.1111/j.1469-8986.2011.01273.x
41. Rajkumar R, Farrher E, Mauler J, Sripad P, Régio Brambilla C, Rota Kops E, et al. Comparison of EEG microstates with resting state fMRI and FDG-PET measures in the default mode network via simultaneously recorded trimodal (PET/MR/EEG) data. Hum Brain Mapp. (2021) 42:4122–33. doi: 10.1002/hbm.24429
42. Choi JW, Jung K-Y, Kim CH, Kim KH. Changes in gamma-and theta-band phase synchronization patterns due to the difficulty of auditory oddball task. Neurosci Lett. (2010) 468:156–60. doi: 10.1016/j.neulet.2009.10.088
43. Guo M, Xu G, Wang L, Fu L. Functional brain network analysis during auditory oddball task. Proceedings of the 2016 Asia-Pacific International Symposium on Electromagnetic Compatibility (APEMC). (Manhattan, NY: IEEE) (2016). p. 1098–100.
44. Bachiller A, Poza J, Gómez C, Molina V, Suazo V, Hornero R. A comparative study of event-related coupling patterns during an auditory oddball task in schizophrenia. J Neural Eng. (2014) 12:016007. doi: 10.1088/1741-2560/12/1/016007
45. Choi JW, Jang K-M, Jung K-Y, Kim M-S, Kim KH. Reduced theta-band power and phase synchrony during explicit verbal memory tasks in female, non-clinical individuals with schizotypal traits. PLoS One. (2016) 11:e0148272. doi: 10.1371/journal.pone.0148272
46. Whelan R, Lonergan R, Kiiski H, Nolan H, Kinsella K, Hutchinson M, et al. Impaired information processing speed and attention allocation in multiple sclerosis patients versus controls: A high-density EEG study. J Neurol Sci. (2010) 293:45–50. doi: 10.1016/j.jns.2010.03.010
47. Imperatori C, Farina B, Quintiliani MI, Onofri A, Gattinara PC, Lepore M, et al. Aberrant EEG functional connectivity and EEG power spectra in resting state post-traumatic stress disorder: A sLORETA study. Biol Psychol. (2014) 102:10–7. doi: 10.1016/j.biopsycho.2014.07.011
48. Franke LM, Walker WC, Hoke KW, Wares JR. Distinction in EEG slow oscillations between chronic mild traumatic brain injury and PTSD. Int J Psychophysiol. (2016) 106:21–9. doi: 10.1016/j.ijpsycho.2016.05.010
49. Meyers J, Mccutcheon VV, Pandey AK, Kamarajan C, Subbie S, Chorlian D, et al. Early sexual trauma exposure and neural response inhibition in adolescence and young adults: Trajectories of frontal theta oscillations during a go/no-go task. J Am Acad Child Adolesc Psychiatry. (2019) 58:242.–255. doi: 10.1016/j.jaac.2018.07.905
51. Hasselmo ME. What is the function of hippocampal theta rhythm?–Linking behavioral data to phasic properties of field potential and unit recording data. Hippocampus. (2005) 15:936–49. doi: 10.1002/hipo.20116
52. Shin J. The interrelationship between movement and cognition: Theta rhythm and the P300 event-related potential. Hippocampus. (2011) 21:744–52. doi: 10.1002/hipo.20792
53. Wang X, Ding M. Relation between P300 and event-related theta-band synchronization: A single-trial analysis. Clin Neurophysiol. (2011) 122:916–24. doi: 10.1016/j.clinph.2010.09.011
Keywords: post-traumatic stress disorder (PSTD), electroencephalogram, cognitive function, functional cortical networks, theta frequency band
Citation: Shim M, Hwang H-J and Lee S-H (2022) Impaired functional cortical networks in the theta frequency band of patients with post-traumatic stress disorder during auditory-cognitive processing. Front. Psychiatry 13:811766. doi: 10.3389/fpsyt.2022.811766
Received: 09 November 2021; Accepted: 19 July 2022;
Published: 11 August 2022.
Edited by:
Marta I. Garrido, University of Melbourne, AustraliaReviewed by:
Julian Keil, University of Kiel, GermanyCopyright © 2022 Shim, Hwang and Lee. This is an open-access article distributed under the terms of the Creative Commons Attribution License (CC BY). The use, distribution or reproduction in other forums is permitted, provided the original author(s) and the copyright owner(s) are credited and that the original publication in this journal is cited, in accordance with accepted academic practice. No use, distribution or reproduction is permitted which does not comply with these terms.
*Correspondence: Han-Jeong Hwang, aHdhbmdoakBrb3JlYS5hYy5rcg==; Seung-Hwan Lee, bHNocHNzQHBhaWsuYWMua3I=
Disclaimer: All claims expressed in this article are solely those of the authors and do not necessarily represent those of their affiliated organizations, or those of the publisher, the editors and the reviewers. Any product that may be evaluated in this article or claim that may be made by its manufacturer is not guaranteed or endorsed by the publisher.
Research integrity at Frontiers
Learn more about the work of our research integrity team to safeguard the quality of each article we publish.