- 1Department of Neurosurgery, The Yangzhou School of Clinical Medicine of Dalian Medical University, Dalian, China
- 2Department of Neurosurgery, Clinical Medical College, Yangzhou University, Yangzhou, China
Background: Psychiatric traits have been associated with intracerebral hemorrhage (ICH) in observational studies, although their causal relationships remain uncertain. We used Mendelian randomization analyses to infer causality between psychiatric traits and ICH.
Methods: We collected data from genome-wide association studies of ICH (n = 361,194) and eight psychiatric traits among Europeans, including mood swings (n = 451,619), major depressive disorder (n = 480,359), attention-deficit/hyperactivity disorder (n = 53,293), anxiety (n = 459,560), insomnia (n = 462,341), schizophrenia (n = 77,096), neuroticism (n = 374,323), and bipolar disorder (n = 51,710). We performed a series of bidirectional two-sample Mendelian randomization and related sensitivity analyses. A Bonferroni corrected threshold of p < 0.00625 (0.05/8) was considered to be significant, and p < 0.05 was considered suggestive of evidence for a potential association.
Results: Mendelian randomization analyses revealed suggestive positive causality of mood swings on ICH (odds ratio = 1.006, 95% confidence interval = 1.001–1.012, p = 0.046), and the result was consistent after sensitivity analysis. However, major depressive disorder (p = 0.415), attention-deficit/hyperactivity disorder (p = 0.456), anxiety (p = 0.664), insomnia (p = 0.699), schizophrenia (p = 0.799), neuroticism (p = 0.140), and bipolar disorder (p = 0.443) are not significantly associated with the incidence of ICH. In the reverse Mendelian randomization analyses, no causal effects of ICH on mood swings (p = 0.565), major depressive disorder (p = 0.630), attention-deficit/hyperactivity disorder (p = 0.346), anxiety (p = 0.266), insomnia (p = 0.102), schizophrenia (p = 0.463), neuroticism (p = 0.261), or bipolar disorder (p = 0.985) were found.
Conclusion: Our study revealed that mood swings are suggestively causal of ICH and increase the risk of ICH. These results suggest the clinical significance of controlling mood swings for ICH prevention.
1. Introduction
Intracerebral hemorrhage (ICH) is the most lethal form of stroke and is commonly caused by hypertension, vascular malformation, tumor (1), trauma (2), cerebral amyloid angiopathy (3), intracranial aneurysms (4), and oral anticoagulants (5). ICH accounts for approximately 10–15% of all stroke types among Europeans (6) with high disability and fatality rates (7), thereby increasing the burden on patients, medical staff, and society. Psychiatric traits usually coexist in patients with ICH. Several observational studies have revealed that ICH is associated with psychiatric traits, including mood swings (8), major depressive disorder (MDD) (9–11), attention-deficit/hyperactivity disorder (ADHD) (12), anxiety (13), insomnia (14), schizophrenia (15, 16), neuroticism (17), and bipolar disorder (BPD) (18). Depression, mood swings, anxiety, and cognitive function disorders are more common in patients with ICH compared to those with acute ischemic stroke (10, 11, 13). In addition, previous research reported that approximately 20% of ICH survivors suffered from depressive mood which adversely affected their quality of life (9). Previous studies provided strong evidence for an association between psychiatric traits and ICH, and our study aimed to explore the causality between psychiatric traits and ICH. Potential residual confounding or reverse causality bias in observational studies has limited the ability to identify causal relationships (19). Therefore, better evaluation methods are needed to prove causality between psychiatric traits and ICH.
With the development of Mendelian randomization (MR) and genome-wide association studies (GWASs), causality between exposure factors and disease outcomes can be identified (20). MR is a method used to infer the relationships between exposure factors and outcomes based on the principle of independent assortment of alleles during meiosis, as they are independent of behavioral and environmental factors that confound epidemiological studies (21). MR assesses the effect of exposure factors on outcomes using genetic variations as instrumental variables (IVs), thereby avoiding potential biases in observational studies (22). A recent MR study indicated that MDD increased the risk of ischemic stroke (23), but the relationships between ICH and psychiatric traits are still unclear.
Here, we collected GWAS data to infer and genetically investigate the causality between ICH and the eight psychiatric traits by bidirectional two-sample MR analyses. Our research may provide evidence to prevent and treat psychiatric traits in patients with ICH.
2. Materials and methods
2.1. Hypotheses of the MR study
The three hypotheses of our MR study are that (i) genetic IVs are strongly associated with the eight psychiatric traits such as anxiety and depression, (ii) they are not associated with any potential confounders, and (iii) they do not affect ICH independently of the eight psychiatric traits. A causal directed acyclic graph is shown in Figure 1. And similar hypotheses are applicable for the reverse MR analysis.
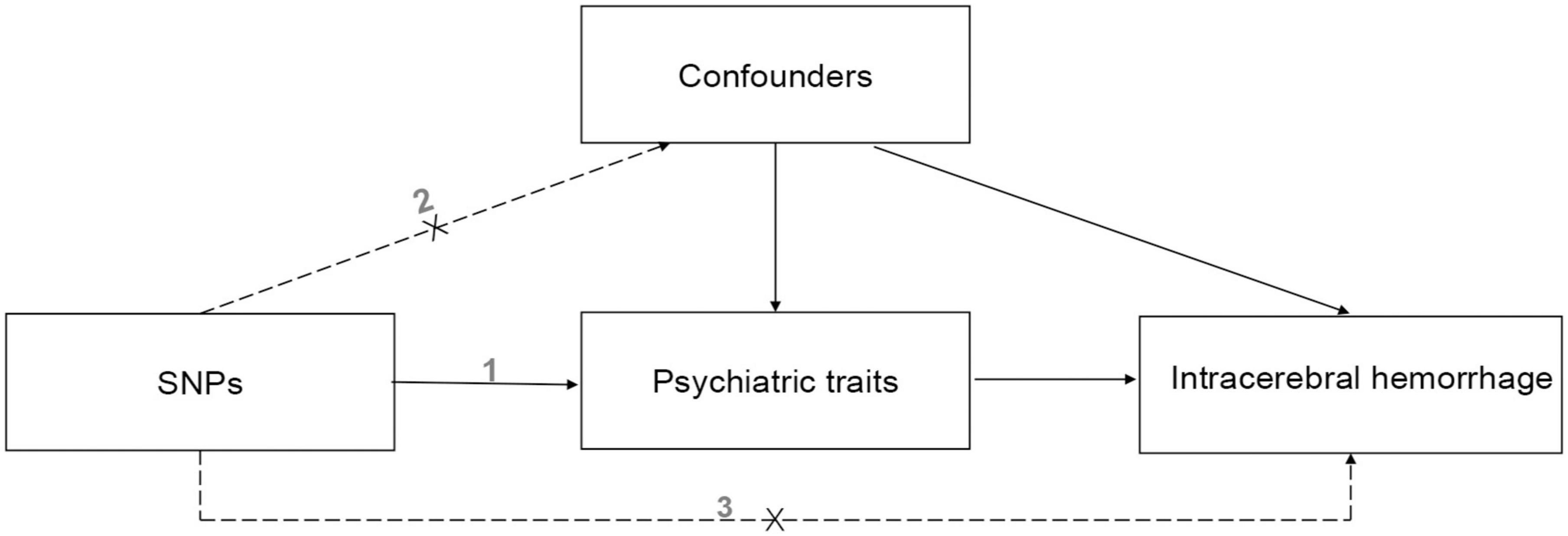
Figure 1. The causal directed acyclic graph of the Mendelian randomization study. The design hypotheses are that the genetic variants are associated with psychiatric traits, but not with confounders, and the genetic variants are associated with the risk of ICH only through psychiatric traits. And similar hypotheses are applicable for the reverse MR analysis. SNP, single nucleotide polymorphism.
2.2. Data extraction
We collected summary data for ICH and the eight psychiatric traits from published studies with European population samples (Table 1). The definitions of ICH and the 8 psychiatric traits are displayed in Supplementary Table 1. We selected a large GWAS (1,253 cases and 359,941 controls) of ICH and download data from.1 We were able to download data of mood swings (204,412 cases and 247,207 controls), anxiety (158,565 cases and 300,995 controls), insomnia (total sample size = 462,341), and neuroticism (total sample size = 374,323) from the MRC Integrative Epidemiology Unit at the University of Bristol.2 There were 44 independent significant loci for MDD (24) from 135,458 cases to 344,901 controls. The sample size of the ADHD study (25) was 55,374 and included 96% European data; there were 12 independent loci and we selected only the European population sample including 19,099 cases and 34,194 controls. The schizophrenia study (26) included 33,640 cases and 43,456 controls with 128 independent associations. The sample size for the BPD study (27) was 51,710 including 20,352 cases and 31,358 controls with 30 loci, including 20 new loci.3
2.3. IV selection and bidirectional MR analyses
Figure 2 illustrates the research workflow. Based on the required MR, valid IVs were selected from the collected data. First, we selected single-nucleotide polymorphisms (SNPs) associated with exposure factors (p < 5 × 10–8) as candidate IVs. Second, linkage disequilibrium was removed for the screened SNPs, leaving independent IVs without linkage disequilibrium (r2 = 0.001, kb = 10,000) (28). Third, the potentially related phenotypes were detected using the online database “PhenoScanner4” by filtration of r2 > 0.8 and p < 5 × 10–8. Fourth, we extracted information regarding SNPs and outcomes; in this step, no proxy SNPs were used. Fifth, we used the harmonization effect to align the effect alleles of the IVs and ensured that the SNP effects on exposures and outcomes were consistent with the effect alleles. Valid IVs of the SNPs associated with ICH as outcomes and eight psychiatric traits as exposure factors (group A) were obtained using the above process and then used for two-sample MR and sensitivity analyses. In the reverse MR analysis, we repeated the above four steps and obtained valid IVs associated with the eight psychiatric traits as outcomes and ICH as exposure factors (group B). Then, we calculated F statistics for each valid IV in both groups to exclude the weak IV bias by using the following formula (29):
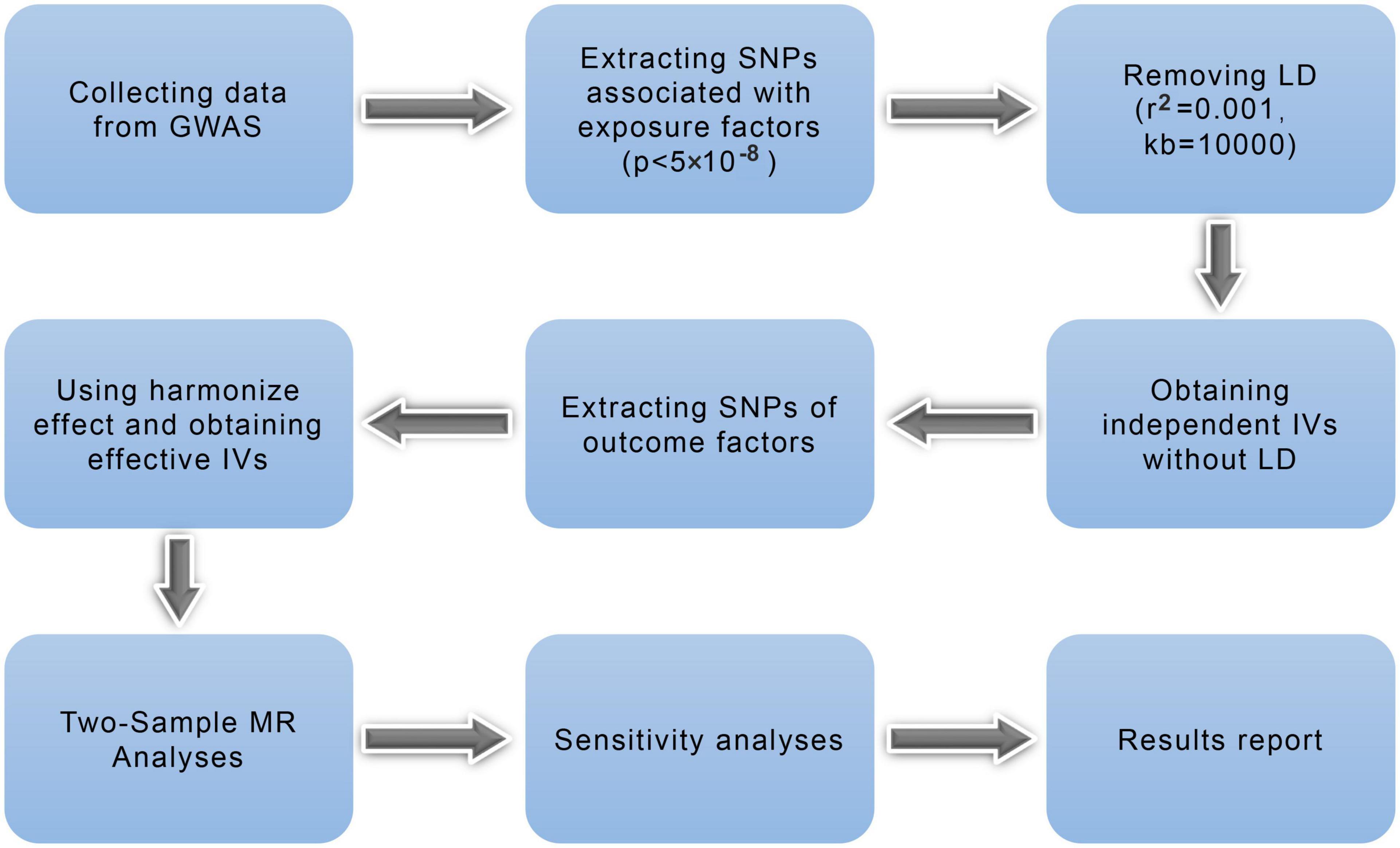
Figure 2. Mendelian randomization study flowchart. The blue boxes represent research steps while gray arrows indicate the overall direction. GWAS, genome-wide association study; IV, instrumental variable; LD, linkage disequilibrium; MR, Mendelian randomization; SNP, single-nucleotide polymorphism.
where n, k, and R2, are sample size, number of IVs, and the variance explained by IVs, respectively. A previous study suggested that a weak IV is considered when the F statistic is less than 10, and an F-value greater than 11 may ensure that relative bias will be <10% at least 95% of the time, regardless of the number of IVs used in the analysis (29–31). Then, we set the p-value < 0.00625 (0.05/8) was considered to be significant according to the Bonferroni multiple correction criterion. A p-value < 0.05 but above the Bonferroni-corrected threshold is considered suggestive evidence of a potential causal relationship.
2.4. Statistical and sensitivity analyses
All statistical analyses were performed using the “Two Sample MR,” “Mendelian Randomization,” and “MR-PRESSO” (Mendelian Randomization Pleiotropy RESidual Sum and Outlier) packages in R (version 4.2.1; The R Foundation for Statistical Computing, Vienna, Austria). Valid IVs were used to perform two-sample MR analyses. The inverse variance weighted method was used in the primary analyses; the weighted median, simple mode, weight mode, and MR-Egger methods were used in the secondary analyses. The sensitivity analysis included heterogeneity, pleiotropy, and leave-one-out sensitivity tests.
We tested for heterogeneity using the inverse variance weighted method and MR-Egger regression, with results revealed by the p-value of Cochran’s Q-test. The intercept term of the MR-Egger method indicated horizontal pleiotropy (32). MR-PRESSO was used to detect and remove horizontal pleiotropy (33) by removing the outlier-corrected horizontal pleiotropy (p < 0.05 for detecting outliers) (34) and testing the difference in estimates before and after outlier correction (35). In addition, we performed a leave-one-out sensitivity test (36) to assess the robustness of the MR results. A p-value less than 0.05 was considered statistically significant in the pleiotropy and heterogeneity tests.
3. Results
3.1. MR and sensitivity analyses results in group A
We performed two-sample MR analyses on the eight groups of data in group A. After obtained for significant SNPs (p < 5 × 10–8), the linkage disequilibrium was removed, and then we found 17 SNPs of psychiatric traits associated with phenotypes such as smoking, hypertension and alcohol consumption. The information on effective IVs are shown in Supplementary Tables 2–9 after removed the above 17 SNPs and the removed SNPs are shown in Supplementary Table 10. These valid IVs were used to complete the MR study and obtain the results. The scatter plots are shown in Figure 3. Each point in the scatter plot represents an IV and the line on each point reflects the 95% confidence interval. The horizontal coordinate is the effect of SNPs on exposure factors (psychiatric traits), the vertical coordinate is the effect of SNPs on outcomes (ICH), and the colored lines indicate the MR fit results indicating that mood swings exhibit a suggestive risk effect on ICH, with an odds ratio of 1.006 (95% confidence interval = 1.001–1.012, p = 0.046), meaning that the risk of ICH increases by 1.006 per unit increase in log-odds of mood swings. However, no evidence demonstrated that MDD (p = 0.415), ADHD (p = 0.456), anxiety (p = 0.664), insomnia (p = 0.699), schizophrenia (p = 0.799), neuroticism (p = 0.140), or BPD (p = 0.443) are causally associated with ICH. The F statistics for each IV in Group A are shown in Supplementary Tables 2–9. No evidence suggested weak IV bias in group A (each F statistics >29). We then summarized the results of group A into a forest plot (Figure 4).
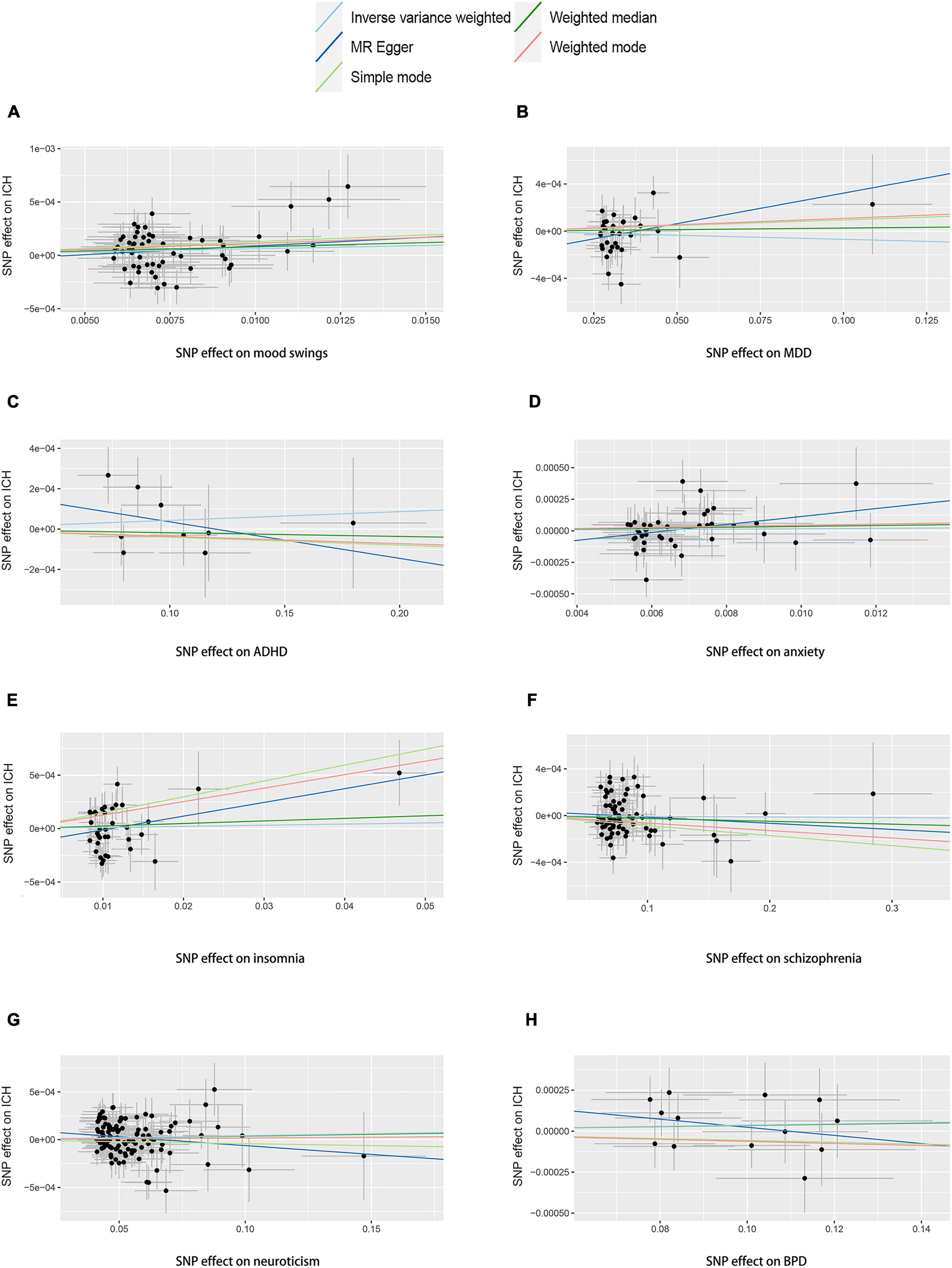
Figure 3. Scatter plots of group A. Scatter plots of the eight MR results from the eight psychiatric traits related to ICH. Each point in the scatter plot represents an IV. The line on each point reflects the 95% CI, and the horizontal coordinate is the effect of SNPs on (A) mood swings, (B) MDD, (C) ADHD, (D) anxiety, (E) insomnia, (F) schizophrenia, (G) neuroticism, and (H) BPD. The vertical coordinate is the effect of SNPs on ICH. SNP effects were plotted into lines for the inverse-variance weighted test (light blue line), MR-Egger regression (dark blue line), simple mode (light green line), weighted median (dark green line), and weighted mode (pink line). The slope of the line corresponds to the causal estimation. A positive slope indicates that mood swings had a positive effect on ICH. ADHD, attention deficit/hyperactivity disorder; BPD, bipolar disorder; CI, confidence interval; ICH, intracerebral hemorrhage; IV, instrumental variable; MDD, major depressive disorder; MR, Mendelian randomization; SNP, single-nucleotide polymorphism.
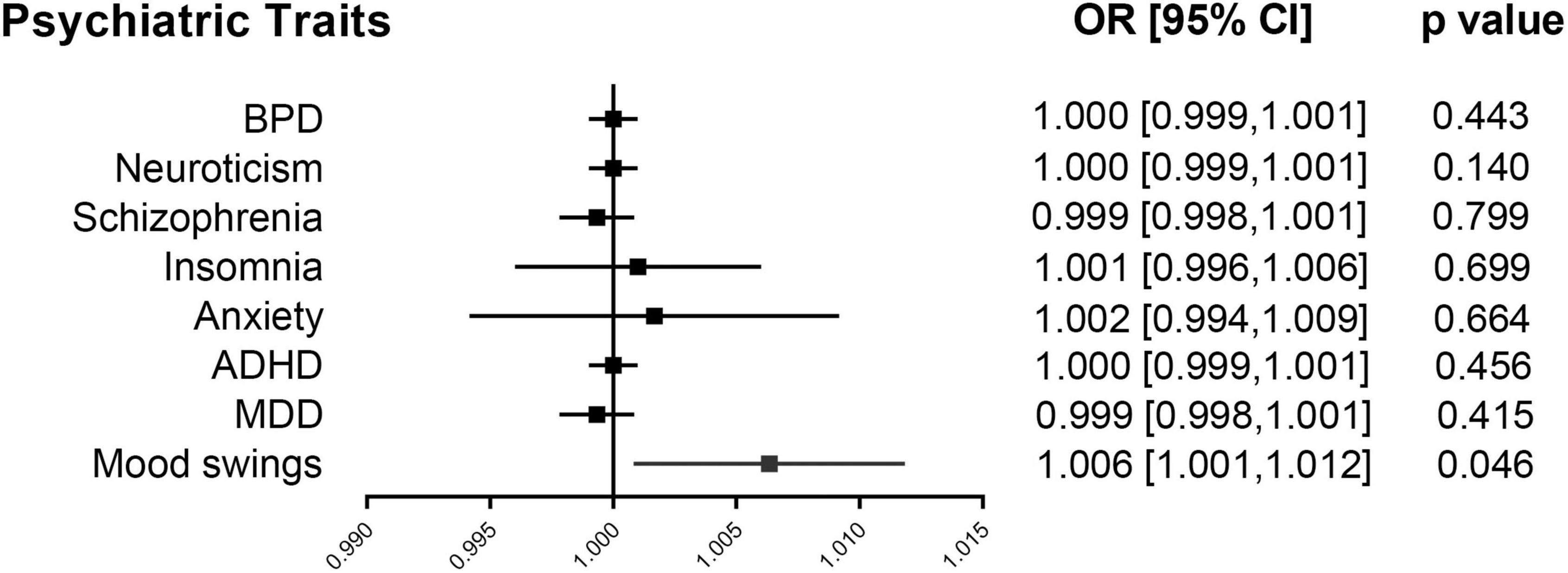
Figure 4. Forest plot of group A. Mendelian randomization associations for eight psychiatric traits on ICH. Results derived from IVW analyses. ADHD, attention deficit/hyperactivity disorder; BPD, bipolar disorder; CI, confidence interval; ICH, intracerebral hemorrhage; IVW, inverse variance weighted; MDD, major depressive disorder; OR, odds ratio.
In the sensitivity analyses, Cochran’s Q-test revealed no heterogeneity (p = 0.131) in the mood swings-ICH subgroup, and the funnel plot (Figure 5A) of the causality of mood swings and ICH was symmetrically distributed overall. No horizontal pleiotropy (p = 0.550) was observed in the mood swings-ICH subgroup. In the leave-one-out graph (Figure 5B), all lines are located to the right of the “0 line,” indicating that the removal of any SNPs had no fundamental effect on the results, suggesting that the MR results were robust and reliable in this group. Furthermore, Cochran’s Q-test revealed no heterogeneity (p > 0.05) or horizontal pleiotropy (p > 0.05) in the other seven subgroups of group A. The leave-one-out analysis showed that no specific SNP affected the overall estimate (Supplementary Figures 1–7).
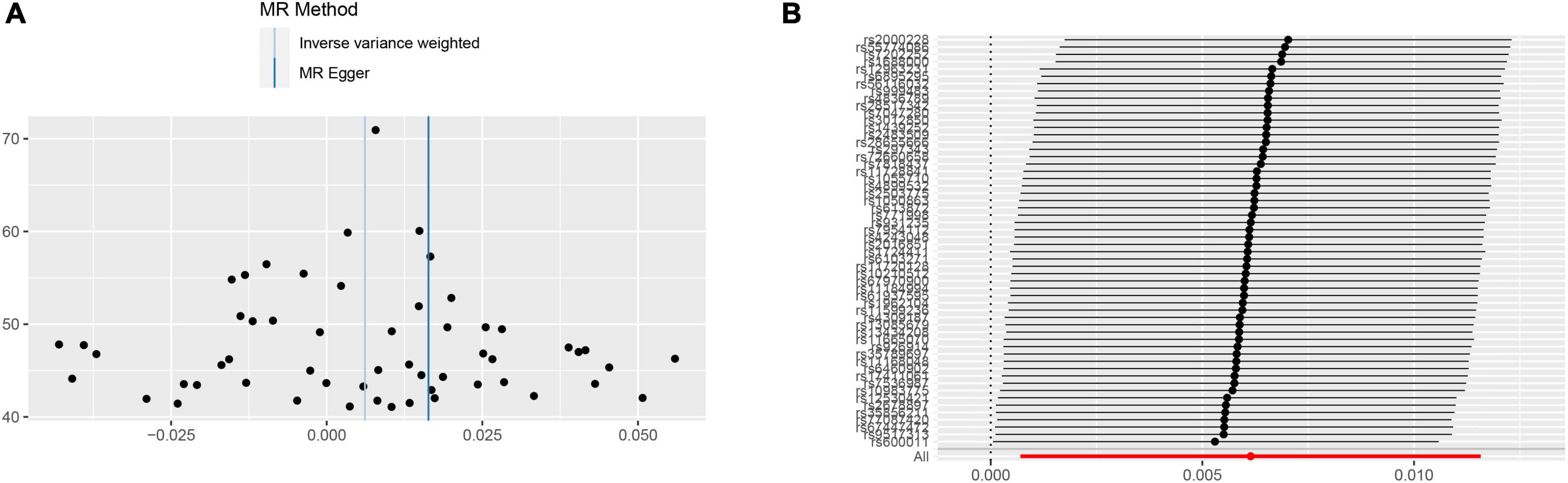
Figure 5. Sensitivity analyses for mood swings on ICH group. (A) The funnel plot of the causality of mood swings on ICH was symmetrically distributed overall, suggesting that no significant heterogeneity exists. (B) The leave-one-out graph indicated that all lines were located to the right of the “0 line,” indicating that the removal of any SNPs had no fundamental effect on the results, suggesting that the MR results were reliable. ICH, intracerebral hemorrhage; MR, Mendelian randomization; SNP, single nucleotide polymorphism.
3.2. MR and sensitivity analyses results in group B
In the reverse MR analyses, the number of relevant SNPs (Supplementary Tables 11–18) obtained for ICH as exposure factors was lower in group B. We decreased the criteria for selecting IVs (p < 5 × 10–6), and no evidence suggested weak IV bias existed in group B (each F statistics >21). The results indicate that mood swings (p = 0.565), MDD (p = 0.630), ADHD (p = 0.346), anxiety (p = 0.266), insomnia (p = 0.102), schizophrenia (p = 0.463), neuroticism (p = 0.261), and BPD (p = 0.985) were not significantly associated with the incidence of ICH and no causal effects of ICH on the eight psychiatric traits were revealed. Scatter plots of group B are shown in Supplementary Figures 8–15. In addition, Cochran’s Q-test revealed no heterogeneity (p > 0.05) or horizontal pleiotropy (p > 0.05) in group B. No specific SNPs affected the MR estimate in the leave-one-out analysis (Supplementary Figures 16–23).
4. Discussion
In this study, we first explored the causality of ICH and eight psychiatric traits via bidirectional two-sample MR analyses by leveraging GWAS summary data. Our study provides suggestive evidence that genetically increasing odds of mood swings are causally associated with the risk of ICH, whereas the other seven psychiatric traits had no significant genetic effect on ICH. In the reverse MR analyses, no evidence supported the presence of a causal relationship between the genetically determined risk of ICH and psychiatric traits.
Our MR results provide genetic evidence of a causal relationship between mood swings and ICH risk. By using data from GWASs, we showed that genetically associated mood swings are associated with a higher risk of ICH, suggesting that preventive strategies focusing on mood stability will reduce the risk of ICH. A meta-analysis suggested a higher incidence and mortality from cardiocerebral vascular disease in people with severe mental illness than in those without (37). Some mental illnesses are associated with insulin resistance (38, 39); patients with severe mental illness are more likely to develop diabetes with more severe and frequent microvascular and macrovascular complications, and insulin resistance may further contribute to hypertension, thereby increasing the risk of cardiocerebral vascular disease (40). In addition, a recent MR study suggested that mood swings are related to cardiocerebral vascular disease risk (41). Evidence from prospective studies implies that mood swings are correlated with blood pressure, which may increase the incidence of ICH (42). Our findings on the effect of mood swings on ICH are compatible with known clinical research.
However, the pathophysiological mechanisms linking mood swings to ICH development are unclear, and several current studies have attempted to explain their mechanisms. Observational studies have shown that oxidative stress is associated with psychiatric traits (43). Total bilirubin is an important antioxidant that exhibits antioxidant, anti-inflammatory, and cytoprotective properties during oxidative stress (44). In terms of oxidative stress, mood swings may decrease total bilirubin levels (45), and lower total bilirubin levels are generally associated with higher blood pressure (44) which increases the risk of ICH from ruptured blood vessels. Inflammatory factors also play an important role in psychiatric traits (46): mood swings may increase the levels of interleukin-6 (47) and cyclooxygenase-2 (48) which may increase the ICH risk and severity, such as larger ICH volumes and perihematomal edema volumes. In addition, alterations in microglia (49, 50), white matter degeneration (51, 52), and aberrant monoaminergic neuron development (53, 54) may also play a role in the relationship between mood swings and ICH. Therefore, further studies are still needed to elucidate these complex mechanisms between mood swings and ICH.
Several clinical studies indicated that ICH was associated with psychiatric traits, including insomnia (14, 55) and schizophrenia (15). ADHD in a small proportion of children may be associated with grade 3 or 4 intraventricular hemorrhage (12). In addition, MDD was reported to share genetic risk factors with cerebrovascular disease and may share common pathophysiological mechanisms, thus explaining the relationship between MDD and ICH (56). On the other hand, whether there is reverse causality between psychiatric traits and ICH has been previously debated. Clinical research demonstrated that greater disability and worse quality of life may increase the risk of depression (10). MDD after ICH may reflect acute mechanical disruption of neural pathways and regions responsible for cognitive and affective function, which is thought to be associated with larger hematoma size or intraventricular hemorrhage (57). ICH patients also often exhibit anxiety that persists for many years after onset (58). Furthermore, cerebral amyloid angiopathy-related ICH displayed prolonged depression and higher resistance to antidepressant treatment (59). Although previous clinical research suggested that psychiatric traits were associated with ICH, in our MR research, there were no genetic causal relationships for the other seven psychiatric traits on the development of ICH, including insomnia, anxiety, MDD, BPD, ADHD, neuroticism, and schizophrenia, and no genetic evidence proved that ICH causes any of these traits. This may be due to several possible reasons. The first is that the discrepancy may be related to other residual confounding bias in observational studies, including blood pressure, diabetes, and microvascular complications (37), which limit the ability to identify causal relationships. The second possible reason is that the number of valid IVs for each trait is small in the reverse MR study, and weak IV bias should be considered, which needs more relevant data to improve further statistics. The third possible reason is that the psychiatric traits after ICH may be associated with physical disability, reducing quality of life, and decreasing social identity in patients with ICH. Therefore, we should not ignore the psychiatric traits associated with ICH, and early intervention and therapy for these traits and adequate social support are beneficial to improve prognosis in patients with ICH.
This study had some limitations. First, our sample was based on a European population. This restriction of our analyses to the European population minimized bias by demographics, but also limits the generalizability of our results for non-European populations. Follow-up studies are required to confirm our results in different ethnicities. Second, psychiatric traits vary widely (60); we only selected eight psychiatric traits in this study, and other psychiatric traits should be included in further studies. Third, the present study did not separately analyze the causality between psychiatric traits and ICH subtypes, such as ventricular, parenchymal, and mixed hemorrhage, which should be considered in follow-up studies. Fourth, psychiatric traits may have some interaction; further research is needed after adjusting for other traits. Our results in our two-sample MR study can only explain to a limited extent the causality between psychiatric traits and ICH. Fifth, as a limitation of the two-sample MR method, sample overlap may exist in our study that may increase weak IV bias. We are temporarily unable to verify or improve this limitation due to data limitations. However, large F statistics of IVs may minimize this dilemma (61).
5. Conclusion
In conclusion, we performed bidirectional two-sample MR analyses after collecting GWAS data and concluded that there is suggestive evidence that mood swings potentially cause ICH. Severe mood swings increase the incidence of ICH. The other seven psychiatric traits (MDD, ADHD, insomnia, schizophrenia neuroticism, anxiety, and BPD) had no causality with ICH. In the reverse MR analyses, no genetic evidence implicated ICH as the cause of mood swings, MDD, schizophrenia, ADHD, BPD, insomnia, neuroticism, or anxiety. These results suggest the clinical significance of controlling mood swings for ICH prevention.
Data availability statement
The original contributions presented in this study are included in the article/Supplementary material, further inquiries can be directed to the corresponding author.
Author contributions
QW and YQ: concept and design and drafting of the manuscript. QW, XW, and ZY: acquisition, analysis, and interpretation of data. YL and HZ: critical revision of the manuscript for important intellectual content. QW, CT, and QM: statistical analysis. YQ, QW, and XL: charting. HZ: obtained funding and supervision. MW: administrative and technical support. All authors contributed to the article and approved the submitted version.
Funding
This study was supported by the Scientific Research Project of Jiangsu Provincial Health Commission (H2018064), Cross Cooperation Project of Northern Jiangsu People’s Hospital (SBIC21009), and Six One Project Top Talent Scientific Research Project of Jiangsu Provincial (LGY2017026).
Acknowledgments
We are grateful for all of the previous studies and databases that facilitated our use of genome-wide association study summary data.
Conflict of interest
The authors declare that the research was conducted in the absence of any commercial or financial relationships that could be construed as a potential conflict of interest.
Publisher’s note
All claims expressed in this article are solely those of the authors and do not necessarily represent those of their affiliated organizations, or those of the publisher, the editors and the reviewers. Any product that may be evaluated in this article, or claim that may be made by its manufacturer, is not guaranteed or endorsed by the publisher.
Supplementary material
The Supplementary Material for this article can be found online at: https://www.frontiersin.org/articles/10.3389/fpsyt.2022.1049432/full#supplementary-material
Footnotes
- ^ http://www.nealelab.is/uk-biobank/
- ^ https://gwas.mrcieu.ac.uk/
- ^ http://www.med.unc.edu/pgc/
- ^ www.phenoscanner.medschl.cam.ac.uk/phenoscanner
References
1. Ikram M, Wieberdink R, Koudstaal P. International epidemiology of intracerebral hemorrhage. Curr Atheroscler Rep. (2012). 14:300–6. doi: 10.1007/s11883-012-0252-1
2. Cepeda S, Gómez P, Castaño-Leon A, Martínez-Pérez R, Munarriz P, Lagares A. Flow induced inflammation mediated artery wall remodeling in the formation and progression of intracranial aneurysms. J Neurotrauma. (2015) 32:1246–53. doi: 10.1089/neu.2014.3808
3. Charidimou A, Boulouis G, Gurol M, Ayata C, Bacskai B, Frosch M, et al. Emerging concepts in sporadic cerebral amyloid angiopathy. Brain. (2017) 140:1829–50. doi: 10.1093/brain/awx047
4. Frösen J, Cebral J, Robertson A, Aoki T. Flow induced inflammation mediated artery wall remodeling in the formation and progression of intracranial aneurysms. Neurosurg Focus. (2019) 47:E21. doi: 10.3171/2019.5.FOCUS19234
5. Cavallini A, Fanucchi S, Persico A. Warfarin-associated intracerebral hemorrhage. Neurol Sci. (2008) 29(Suppl. 2):S266–8. doi: 10.1007/s10072-008-0959-5
6. Hostettler I, Seiffge D, Werring D. Intracerebral hemorrhage: an update on diagnosis and treatment. Expert Rev Neurother. (2019) 19:679–94. doi: 10.1080/14737175.2019.1623671
7. Poon M, Fonville A, Al-Shahi Salman R. Long-term prognosis after intracerebral haemorrhage: systematic review and meta-analysis. J Neurol Neurosurg Psychiatry. (2014) 85:660–7. doi: 10.1136/jnnp-2013-306476
8. Fenger-Grøn M, Paulsen Møller I, Schou Pedersen H, Frost L, Sandbæk A, Davydow D, et al. Death of a partner and risks of ischemic stroke and intracerebral hemorrhage: a nationwide danish matched cohort study. J Am Heart Assoc. (2020) 9:e018763. doi: 10.1161/JAHA.120.018763
9. Christensen M, Mayer S, Ferran J, Kissela B. Depressed mood after intracerebral hemorrhage: the FAST trial. Cerebrovasc Dis. (2009) 27:353–60. doi: 10.1159/000202012
10. Zeng Y, Cheng H, Cheng L, Huang G, Chen Y, Tang W. Comparison of poststroke depression between acute ischemic and hemorrhagic stroke patients. Int J Geriatr Psychiatry. (2021) 36:493–9. doi: 10.1002/gps.5444
11. de Man-van Ginkel J, Hafsteinsdóttir T, Lindeman E, Geerlings M, Grobbee D, Schuurmans M. Clinical manifestation of depression after stroke: is it different from depression in other patient populations? PLoS One. (2015) 10:e0144450. doi: 10.1371/journal.pone.0144450
12. O’Callaghan M, Harvey J. Biological predictors and co-morbidity of attention deficit and hyperactivity disorder in extremely low birthweight infants at school. J Paediatr Child Health. (1997) 33:491–6. doi: 10.1111/j.1440-1754.1997.tb01657.x
13. Koivunen R, Harno H, Tatlisumak T, Putaala J. Depression, anxiety, and cognitive functioning after intracerebral hemorrhage. Acta Neurol Scand. (2015) 132:179–84. doi: 10.1111/ane.12367
14. Wu M, Lin H, Weng S, Ho C, Wang J, Hsu Y. Insomnia subtypes and the subsequent risks of stroke: report from a nationally representative cohort. Stroke. (2014) 45:1349–54. doi: 10.1161/STROKEAHA.113.003675
15. Liang Y, Huang J, Tian J, Cao Y, Zhang G, Wang C, et al. The prevalence and risk factors of stroke in patients with chronic schizophrenia. Neuropsychiatr Dis Treat. (2016) 12:1131–4. doi: 10.2147/NDT.S106663
16. Hüfner K, Frajo-Apor B, Hofer A. Neurology issues in schizophrenia. Curr Psychiatry Rep. (2015) 17:32. doi: 10.1007/s11920-015-0570-4
17. Siragusa M, Rufin T, Courtois R, Belzung C, Andersson F, Brizard B, et al. Left amygdala volume and brain tissue pulsatility are associated with neuroticism: an MRI and ultrasound study. Brain Imaging Behav. (2021) 15:1499–507. doi: 10.1007/s11682-020-00348-w
18. Yuan M, Xiao Z, Zhou H, Rao W, Huang G, Nie H, et al. Bipolar disorder and the risk for stroke incidence and mortality: a meta-analysis. Neurol Sci. (2022) 43:467–76. doi: 10.1007/s10072-021-05348-2
19. Lawlor D, Harbord R, Sterne J, Timpson N, Davey Smith G. Mendelian randomization: using genes as instruments for making causal inferences in epidemiology. Stat Med. (2008) 27:1133–63. doi: 10.1002/sim.3034
20. Davey Smith G, Hemani G. Mendelian randomization: genetic anchors for causal inference in epidemiological studies. Hum Mol Genet. (2014) 23:R89–98. doi: 10.1093/hmg/ddu328
21. Sleiman P, Grant S. Mendelian randomization in the era of genomewide association studies. Clin Chem. (2010) 56:723–8. doi: 10.1373/clinchem.2009.141564
22. Davey Smith G, Ebrahim S. What can mendelian randomisation tell us about modifiable behavioural and environmental exposures? BMJ. (2005) 330:1076–9. doi: 10.1136/bmj.330.7499.1076
23. Cai H, Cai B, Zhang H, Sun W, Wang Y, Zhou S, et al. Major depression and small vessel stroke: a mendelian randomization analysis. J Neurol. (2019) 266:2859–66. doi: 10.1007/s00415-019-09511-w
24. Wray N, Ripke S, Mattheisen M, Trzaskowski M, Byrne E, Abdellaoui A, et al. Genome-wide association analyses identify 44 risk variants and refine the genetic architecture of major depression. Nat Genet. (2018) 50:668–81. doi: 10.1038/s41588-018-0090-3
25. Demontis D, Walters R, Martin J, Mattheisen M, Als T, Agerbo E, et al. Discovery of the first genome-wide significant risk loci for attention-deficit/hyperactivity disorder. Nat Genet. (2019) 51:63–75. doi: 10.1038/s41588-018-0269-7
26. Ripke S, Neale B, Corvin A, Walters J, Farh K, Holmans P, et al. Biological insights from 108 schizophrenia-associated genetic loci. Nature. (2014) 511:421–7. doi: 10.1038/nature13595
27. Stahl E, Breen G, Forstner A, McQuillin A, Ripke S, Trubetskoy V, et al. Genome-wide association study identifies 30 loci associated with bipolar disorder. Nat Genet. (2019) 51:793–803. doi: 10.1038/s41588-019-0397-8
28. Chang C, Chow C, Tellier L, Vattikuti S, Purcell S, Lee J. Second-generation PLINK: rising to the challenge of larger and richer datasets. Gigascience. (2015) 4:7. doi: 10.1186/s13742-015-0047-8
29. Pierce B, Ahsan H, Vanderweele T. Power and instrument strength requirements for mendelian randomization studies using multiple genetic variants. Int J Epidemiol. (2011) 40:740–52. doi: 10.1093/ije/dyq151
30. Staiger D, Stock JH. Instrumental variables regression with weak instruments. Econometrica. (1994) 65:557–86. doi: 10.3386/t0151
31. Stock JH, Yogo M. “Testing for weak instruments in linear IV regression,” In: Andrews DWK, Stock JH editors. Identification and Inference for Econometric Models: essays in Honor of Thomas Rothenberg. Cambridge: Cambridge University Press (2005). p. 80–108. doi: 10.1017/CBO9780511614491.006
32. Bowden J, Holmes M. Meta-analysis and mendelian randomization: a review. Res Synth Methods. (2019) 10:486–96. doi: 10.1002/jrsm.1346
33. Verbanck M, Chen C, Neale B, Do R. Detection of widespread horizontal pleiotropy in causal relationships inferred from Mendelian randomization between complex traits and diseases. Nat Genet. (2018) 50:693–8. doi: 10.1038/s41588-018-0099-7
34. Burgess S, Bowden J, Fall T, Ingelsson E, Thompson S. Sensitivity analyses for robust causal inference from mendelian randomization analyses with multiple genetic variants. Epidemiology. (2017) 28:30–42. doi: 10.1097/EDE.0000000000000559
35. Ong J, MacGregor S. Implementing MR-PRESSO and GCTA-GSMR for pleiotropy assessment in Mendelian randomization studies from a practitioner’s perspective. Genet Epidemiol. (2019) 43:609–16. doi: 10.1002/gepi.22207
36. Wang R, Yang Y, Li H, Shen X, Chen S, Cui M, et al. Genetically determined low income modifies Alzheimer’s disease risk. Ann Transl Med. (2021) 9:1222. doi: 10.21037/atm-21-344
37. Lambert A, Parretti H, Pearce E, Price M, Riley M, Ryan R, et al. Temporal trends in associations between severe mental illness and risk of cardiovascular disease: a systematic review and meta-analysis. PLoS Med. (2022) 19:e1003960. doi: 10.1371/journal.pmed.1003960
38. Fanelli G, Franke B, De Witte W, Ruisch I, Haavik J, van Gils V, et al. Insulinopathies of the brain? Genetic overlap between somatic insulin-related and neuropsychiatric disorders. Transl Psychiatry. (2022) 12:59. doi: 10.1038/s41398-022-01817-0
39. Wimberley T, Horsdal H, Brikell I, Laursen T, Astrup A, Fanelli G, et al. Temporally ordered associations between type 2 diabetes and brain disorders – a danish register-based cohort study. BMC Psychiatry. (2022) 22:573. doi: 10.1186/s12888-022-04163-z
40. Holt R, Mitchell A. Diabetes mellitus and severe mental illness: mechanisms and clinical implications. Nat Rev Endocrinol. (2015) 11:79–89. doi: 10.1038/nrendo.2014.203
41. Peng P, Chen Z, Zhang X, Guo Z, Dong F, Xu Y, et al. Investigating causal relationships between psychiatric traits and intracranial aneurysms: a bi-directional two-sample mendelian randomization study. Front Genet. (2021) 12:741429. doi: 10.3389/fgene.2021.741429
42. McGowan N, Nichols M, Bilderbeck A, Goodwin G, Saunders K. Blood pressure in bipolar disorder: evidence of elevated pulse pressure and associations between mean pressure and mood instability. Int J Bipolar Disord. (2021) 9:5. doi: 10.1186/s40345-020-00209-x
43. Kim Y, Vadodaria K, Lenkei Z, Kato T, Gage F, Marchetto M, et al. Mitochondria, metabolism, and redox mechanisms in psychiatric disorders. Antioxid Redox Signal. (2019) 31:275–317. doi: 10.1089/ars.2018.7606
44. Fu K, Garvan C, Heaton S, Nagaraja N, Doré S. Association of serum bilirubin with the severity and outcomes of intracerebral hemorrhages. Antioxidants. (2021) 10:1346. doi: 10.3390/antiox10091346
45. Lu Z, Pu C, Zhang Y, Sun Y, Liao Y, Kang Z, et al. Oxidative stress and psychiatric disorders: evidence from the bidirectional mendelian randomization study. Antioxidants. (2022) 11:1386. doi: 10.3390/antiox11071386
46. Bavaresco D, da Rosa M, Uggioni M, Ferraz S, Pacheco T, Toé H, et al. Increased inflammatory biomarkers and changes in biological rhythms in bipolar disorder: a case-control study. J Affect Disord. (2020) 271:115–22. doi: 10.1016/j.jad.2020.03.073
47. Leasure A, Kuohn L, Vanent K, Bevers M, Kimberly W, Steiner T, et al. Association of serum IL-6 (Interleukin 6) with functional outcome after intracerebral hemorrhage. Stroke. (2021) 52:1733–40. doi: 10.1161/STROKEAHA.120.032888
48. Wu T, Wu H, Wang J, Wang J. Expression and cellular localization of cyclooxygenases and prostaglandin E synthases in the hemorrhagic brain. J Neuroinflammation. (2011) 8:22. doi: 10.1186/1742-2094-8-22
49. Mattei D, Notter T. Basic concept of microglia biology and neuroinflammation in relation to psychiatry. Curr Top Behav Neurosci. (2020) 44:9–34. doi: 10.1007/7854_2018_83
50. Wang J. Preclinical and clinical research on inflammation after intracerebral hemorrhage. Prog Neurobiol. (2010) 92:463–77. doi: 10.1016/j.pneurobio.2010.08.001
51. Song W, Qian W, Wang W, Yu S, Lin G. Mendelian randomization studies of brain MRI yield insights into the pathogenesis of neuropsychiatric disorders. BMC Genomics. (2021) 22:342. doi: 10.1186/s12864-021-07661-8
52. Oide T, Takahashi H, Yutani C, Ishihara T, Ikeda S. Relationship between lobar intracerebral hemorrhage and leukoencephalopathy associated with cerebral amyloid angiopathy: clinicopathological study of 64 Japanese patients. Amyloid. (2003) 10:136–43. doi: 10.3109/13506120308998994
53. Jukic M, Carrillo-Roa T, Bar M, Becker G, Jovanovic V, Zega K, et al. Abnormal development of monoaminergic neurons is implicated in mood fluctuations and bipolar disorder. Neuropsychopharmacology. (2015) 40:839–48. doi: 10.1038/npp.2014.244
54. Dun N, Dun S, Chiaia N. Hemorrhage induces Fos immunoreactivity in rat medullary catecholaminergic neurons. Brain Res. (1993) 608:223–32. doi: 10.1016/0006-8993(93)91462-2
55. Zhou L, Yu K, Yang L, Wang H, Xiao Y, Qiu G, et al. Sleep duration, midday napping, and sleep quality and incident stroke: the Dongfeng-Tongji cohort. Neurology. (2020) 94:e345–56. doi: 10.1212/WNL.0000000000008739
56. Zhao F, Yue Y, Jiang H, Yuan Y. Shared genetic risk factors for depression and stroke. Prog Neuropsychopharmacol Biol Psychiatry. (2019) 93:55–70. doi: 10.1016/j.pnpbp.2019.03.003
57. Keins S, Abramson J, Castello J, Pasi M, Charidimou A, Kourkoulis C, et al. Latent profile analysis of cognitive decline and depressive symptoms after intracerebral hemorrhage. BMC Neurol. (2021) 21:481. doi: 10.1186/s12883-021-02508-x
58. Scopelliti G, Casolla B, Boulouis G, Kuchcinski G, Moulin S, Leys D, et al. Long-term anxiety in spontaneous intracerebral hemorrhage survivors. Int J Stroke. (2022) 17:1093–9. doi: 10.1177/17474930221085443
59. Castello J, Pasi M, Kubiszewski P, Abramson J, Charidimou A, Kourkoulis C, et al. Cerebral small vessel disease and depression among intracerebral hemorrhage survivors. Stroke. (2022) 53:523–31. doi: 10.1161/STROKEAHA.121.035488
60. Hübel C, Gaspar H, Coleman J, Hanscombe K, Purves K, Prokopenko I, et al. Genetic correlations of psychiatric traits with body composition and glycemic traits are sex- and age-dependent. Nat Commun. (2019) 10:5765. doi: 10.1038/s41467-019-13544-0
Keywords: Mendelian randomization, intracerebral hemorrhage, psychiatric traits, genome-wide association study, causality
Citation: Wang Q, Qi Y, Li Y, Yan Z, Wang X, Ma Q, Tang C, Liu X, Wei M and Zhang H (2023) Psychiatric traits and intracerebral hemorrhage: A Mendelian randomization study. Front. Psychiatry 13:1049432. doi: 10.3389/fpsyt.2022.1049432
Received: 20 September 2022; Accepted: 12 December 2022;
Published: 04 January 2023.
Edited by:
Panagiotis Ferentinos, National and Kapodistrian University of Athens, GreeceReviewed by:
Zhe Lu, Peking University Sixth Hospital, ChinaGiuseppe Fanelli, University of Bologna, Italy
Copyright © 2023 Wang, Qi, Li, Yan, Wang, Ma, Tang, Liu, Wei and Zhang. This is an open-access article distributed under the terms of the Creative Commons Attribution License (CC BY). The use, distribution or reproduction in other forums is permitted, provided the original author(s) and the copyright owner(s) are credited and that the original publication in this journal is cited, in accordance with accepted academic practice. No use, distribution or reproduction is permitted which does not comply with these terms.
*Correspondence: Hengzhu Zhang, emhhbmdoZW5nemh1QHNpbmEuY29t
†These authors have contributed equally to this work and share first authorship