- 1Department of Magnetic Resonance Imaging, The First Affiliated Hospital of Zhengzhou University, Zhengzhou, China
- 2Henan Key Laboratory of Magnetic Resonance and Brain Function, Zhengzhou, China
- 3Henan Engineering Technology Research Center for Detection and Application of Brain Function, Zhengzhou, China
- 4Key Laboratory for Functional Magnetic Resonance Imaging and Molecular Imaging of Henan Province, Zhengzhou, China
- 5Henan Engineering Research Center of Medical Imaging Intelligent Diagnosis and Treatment, Zhengzhou, China
- 6Zhengzhou Key Laboratory of Brain Function and Cognitive Magnetic Resonance Imaging, Zhengzhou, China
- 7Henan Key Laboratory of Imaging Intelligence Research, Zhengzhou, China
- 8Henan Engineering Research Center of Brain Function Development and Application, Zhengzhou, China
Background: Many neuroimaging studies have reported abnormalities in brain structure and function in internet gaming disorder (IGD). However, the findings were divergent. We aimed to provide evidence-based evidence of structural and functional changes in IGD by conducting a meta-analysis integrating these studies quantitatively.
Method: A systematic search was conducted in PubMed, ScienceDirect, Web of Science, and Scopus from January 1, 2010 to October 31, 2021, to identify eligible voxel-based morphometry (VBM) and functional magnetic resonance imaging (fMRI) studies. Brain alternations between IGD subjects and healthy controls (HCs) were compared using the anisotropic seed-based d mapping (AES-SDM) meta-analytic method. Meta-regression analysis was used to investigate the relationship between gray matter volume (GMV) alterations and addiction-related clinical features.
Results: The meta-analysis contained 15 VBM studies (422 IGD patients and 354 HCs) and 30 task-state fMRI studies (617 IGD patients and 550 HCs). Compared with HCs, IGD subjects showed: (1) reduced GMV in the bilateral anterior/median cingulate cortex, superior/inferior frontal gyrus and supplementary motor area; (2) hyperactivation in the bilateral inferior frontal gyrus, precentral gyrus, left precuneus, right inferior temporal gyrus and right fusiform; (3) hypoactivation in the bilateral lingual and the left middle frontal gyrus; and (4) both decreased GMV and increased activation in the left anterior cingulate. Furthermore, Meta-regression revealed that GMV reduction in left anterior cingulate were positively correlated with BIS-11 score [r = 0.725, p = 0.012(uncorrected)] and IAT score [r = 0.761, p = 0.017(uncorrected)].
Conclusion: This meta-analysis showed structural and functional impairments in brain regions related to executive control, cognitive function and reward-based decision making in IGD. Furthermore, multi-domain assessments captured different aspects of neuronal changes in IGD, which may help develop effective interventions as potential therapeutic targets.
Introduction
Internet addiction has aroused widespread concern around the world and led to many related mental diseases (1). Internet gaming disorder (IGD) accounted for 57.5% of all types of Internet addiction among college students (2) defined as the inability of an individual to control his/her use of online game behaviors, which has many similarities with pathological gambling (3–5). Many players exhibit symptoms such as decreased executive function (6), excessive impulsivity (7, 8), impaired risky decision-making ability (9) and craving (10, 11). Although unlike substance addiction, IGD does not consume addictive substances, it can lead to dependence and mental and physical health problems similar to other addictions due to excessive gaming (12, 13). Given its growing prevalence and negative effects, IGD has been listed in Section “Results” of the fifth edition of the Diagnostic and Statistical Manual for Mental Disorders (DSM-5) as a condition worthy of further research. Therefore, more evidence is needed to understand the neuropathic factors behind IGD, which will facilitate future research and shed light on the success of its treatment.
As a non-invasive imaging technique, magnetic resonance (MR) imaging has shown great potential value in elucidating the neuropathogenesis of psychiatric disorders. Functional magnetic resonance imaging (fMRI) has been widely used to reveal neural changes in addictive disorders (14–17). Previous studies in functional neuroimaging (12, 18–20) have revealed that during performing the impulse control-related tasks, IGD subjects had aberrant activations in the frontal, insular, temporal and parietal cortex compared with the HCs. In addition to functional activity changes, gray matter volume (GMV) as a structural marker of the brain may be relatively stable over time and can be used as a basis for functional neural activity (21). GMV has been widely used in many studies of mental disorders (22–24). VBM is a voxel-based gray matter volume measurement method that can detect subtle changes in GMV. Previous VBM studies have demonstrated that IGD patients have reduced GMV in brain regions associated with executive control such as the anterior cingulate cortex (ACC) and dorsolateral prefrontal cortex involved in processing goal-directed behaviors (5, 11, 25, 26). Therefore, a quantitative synthesis of VBM studies in IGD can provide additional information to complement the results of fMRI studies. The combination of different modalities can more comprehensively identify the common and specific neural changes in IGD. The severity of IGD was measured by Young’s Internet addiction test (IAT). IAT was a reliable and valid instrument for classifying Internet addiction disorder (27). The clinical relevance of impulsivity was frequently highlighted because it affected many mental and behavioral disorders. Cao and Lee have found that IGD subjects were more impulsive than healthy controls (8, 28), which may lead to serious impairments in psychological and social functions, such as suicide attempts and crime. The severity of Internet addiction disorder (IAD) was positively correlated with both behavioral impulsivity and self-reported impulsivity (28). Barratt Impulsiveness Scale-11 (BIS-11) score could well assess the core impulsivity characteristics of addiction (29).
Although many neuroimaging studies have shown changes in brain structure and function in patients with IGD, these findings are inconsistent or even contrary. Consequently, it is of great significance to perform a pooled meta-analysis to provide evidence-based evidence of structural and functional changes in IGD by pooling “observations” with controls for random effect. A preliminary meta-analysis of 10 VBM studies and 27 fMRI studies has found brain hyperactivation in the anterior and posterior cingulate cortices, caudate and posterior inferior frontal gyrus (IFG), hypoactivation in the anterior IFG, the posterior insula, and reduced gray-matter volume in the anterior cingulate, orbitofrontal and dorsolateral prefrontal (30). However, in recent years, there have been substantial novel and high-quality studies on this subject. Compared with previous meta-analysis, this study not only included more new studies to explore the neural changes of IGD, but also focused on exploring the relationship between brain GMV and impulsivity in IGD patients. It is high time that we performed an updated meta-analysis to confirm, supplement, and/or modify the results of previous meta-analysis.
The purpose of our study was to conduct two meta-analyses separately including numerous proven VBM and fMRI studies and a conjunction analysis between two meta-analyses to explore: (a) brain GMV and functional abnormalities; (b) the association between some common addiction-related clinical features and GMV alterations. Based on previous empirical studies, we hypothesized that IGD subjects compared to HCs would show GMV and functional alterations in brain regions involved in reward-based decision-making such as the inferior prefrontal cortex (31), cognitive control such as the cingulate gyrus and the precuneus (18), and visual cognitive functions such as the fusiform (8). As a reliable structural marker, GMV can easily identify different psychiatric disorders without potentially confounding considerations of tasks in fMRI (32). Therefore, we also hypothesized that the GMV alterations in IGD subjects would be closely associated with clinical variables such as the IAT score and BIS-11 score.
Methods
Inclusion of studies
Extensive searches were carried out in PubMed, ScienceDirect, Web of Science, and Scopus from January 1, 2010, to October 31, 2021, combined with the following keywords: (“voxel-based morphometry” or “VBM” or “gray matter” or “voxel-wise” or “functional magnetic resonance imaging” or “fMRI”) and (“online-game” or “Internet gaming disorder” or “IGD”). Studies were included if (1) they used specific tasks during the MRI scan or they used VBM to analyze gray matter; (2) they provided whole-brain pairwise voxel-based comparisons of patient groups (IGD) relative to controls; (3) the studies reported Montreal Neurological Institute (MNI) or Talairach coordinates of the whole brain; (4) there were no neurological or psychiatric comorbidities such as depression, anxiety, autism, learning disorder and epilepsy; (5) studies were peer-reviewed and published in English. Studies were excluded if (1) they only reported region of interest findings; (2) peak coordinates were still not available even if we contacted the authors by email; (3) studies used tensor-based morphometry; (4) they were unpublished studies; (5) they didn’t use the same threshold throughout the whole brain within each included study. Three authors independently searched, selected, and cross-checked the literature. Any divergence was settled through a joint revaluation of the original studies. And then we implemented the following steps.
Statistical analysis
Structural and functional brain differences between individuals with IGD and healthy controls were analyzed by using the anisotropic seed-based d mapping (AES-SDM) meta-analytic software, version 5.151. The AES-SDM method has been well validated in recent meta-analysis of psychiatric diseases (33, 34). AES-SDM is a statistical technique that can use previously reported peak coordinates and effect sizes (t-scores) to calculate signed (positive/negative) effect sizes and variance maps of brain regional differences between patient and control groups. For each meta-analysis, maps are combined across studies based on the random-effect model, considering sample size, intra-study variability and inter-study heterogeneity (35). The processing process of AES-SDM data is summarized here2. Here we briefly outline the steps: (1) we extracted peak coordinates and effect sizes (t-values) from each included study. Sometimes some studies included z-scores without t-values, then the z-scores could be converted to t statistics using an online converter3; (2) we converted peak coordinates into standardized MNI space; (3) we set the full width at half maximum (FWHM) to 20 mm as this will maintain a balance between sensitivity. Voxel p < 0.005 was used as a significant threshold. Peak height threshold > 1 and cluster extent threshold > 10 voxels were supplemented to optimally balance sensitivity and specificity (36).
First, two meta-analyses including all fMRI and VBM studies were conducted separately to identify neural changes in IGD. Second, a multimodal analysis was further performed on patients and controls to examine overlapping regions of functional and structural abnormalities. Next, a jack-knife sensitivity analysis was also conducted to test the robustness of the results by repeating the same analysis excluding one study each time and to assess the reproducibility of the results for each meta-analysis (36). If one brain region survives in most of the repeats, we consider the finding to be highly replicable. Then meta-regression analysis was conducted to explore the association between GMV alterations and clinical features including the BIS-11 score and IAT score. At last, funnel plots constructed by AES-SDM (Supplementary Figure 1) and Egger’s test were used to examine possible publication bias (37).
Results
Included studies and sample characteristics
Searching in various databases, a total of 1002 records were identified. After removing duplicates, 506 records were screened and 131 full-text articles were assessed for eligibility. Our final dataset consisted of 15 VBM studies including 422 IGDs and 354 HCs and 30 fMRI studies including 617 IGDs and 550 HCs (Figure 1 and Table 1).
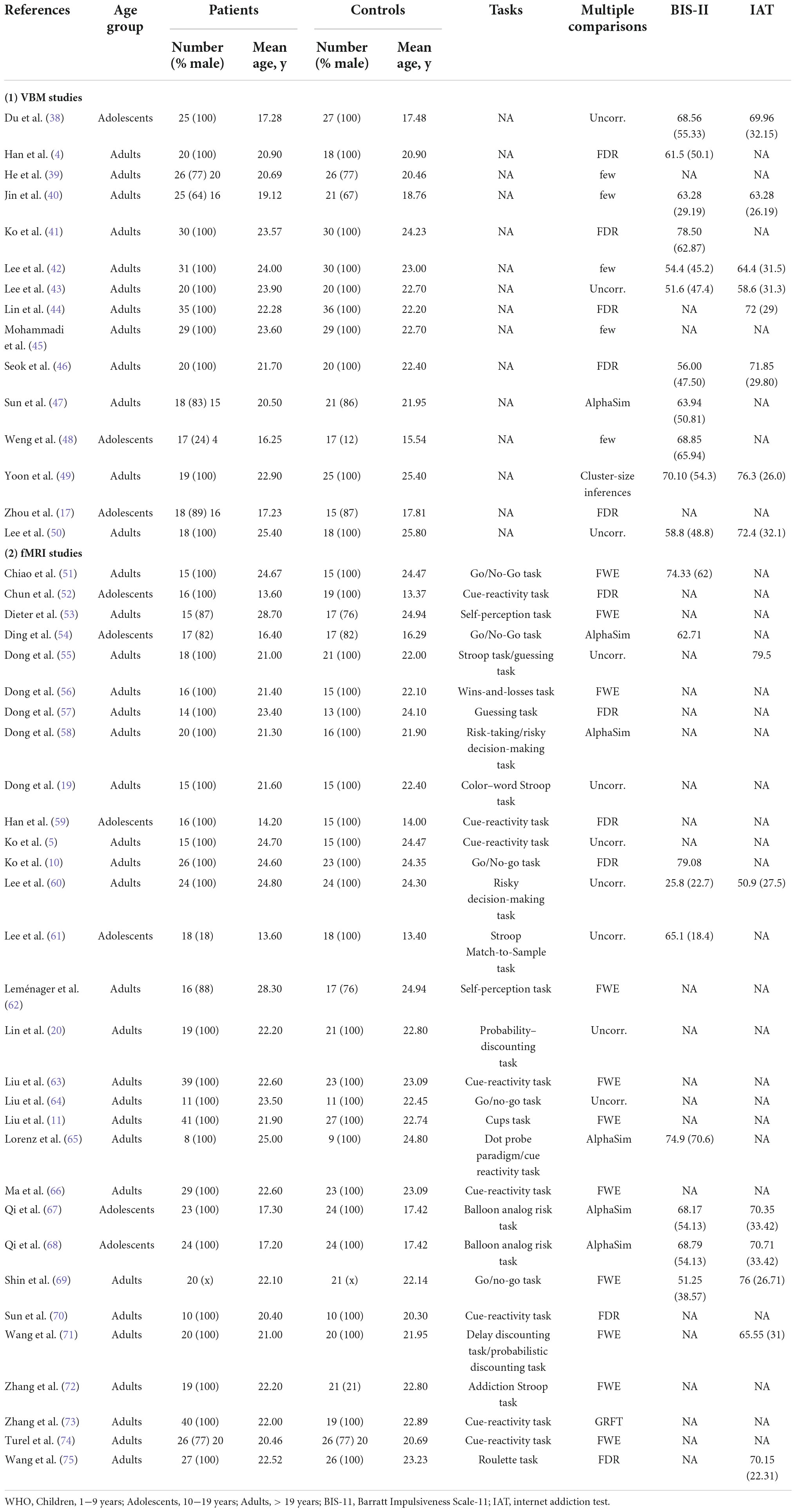
Table 1. Demographic and clinical characteristics of voxel-based morphometry and functional magnetic resonance imaging studies in internet gaming disorder.
Meta-analysis result
Voxel-based morphometry meta-analysis
As demonstrated in Figure 2 and Table 2, IGD subjects showed significantly smaller GMV in the bilateral anterior cingulate cortex (ACC), median cingulate cortex (MCC), superior frontal gyrus, medial orbital (SFG), inferior frontal gyrus (IFG) and supplementary motor area (SMA) compared with healthy controls. Compared with the control group, there was no significant increase of GMV in the IGD group.
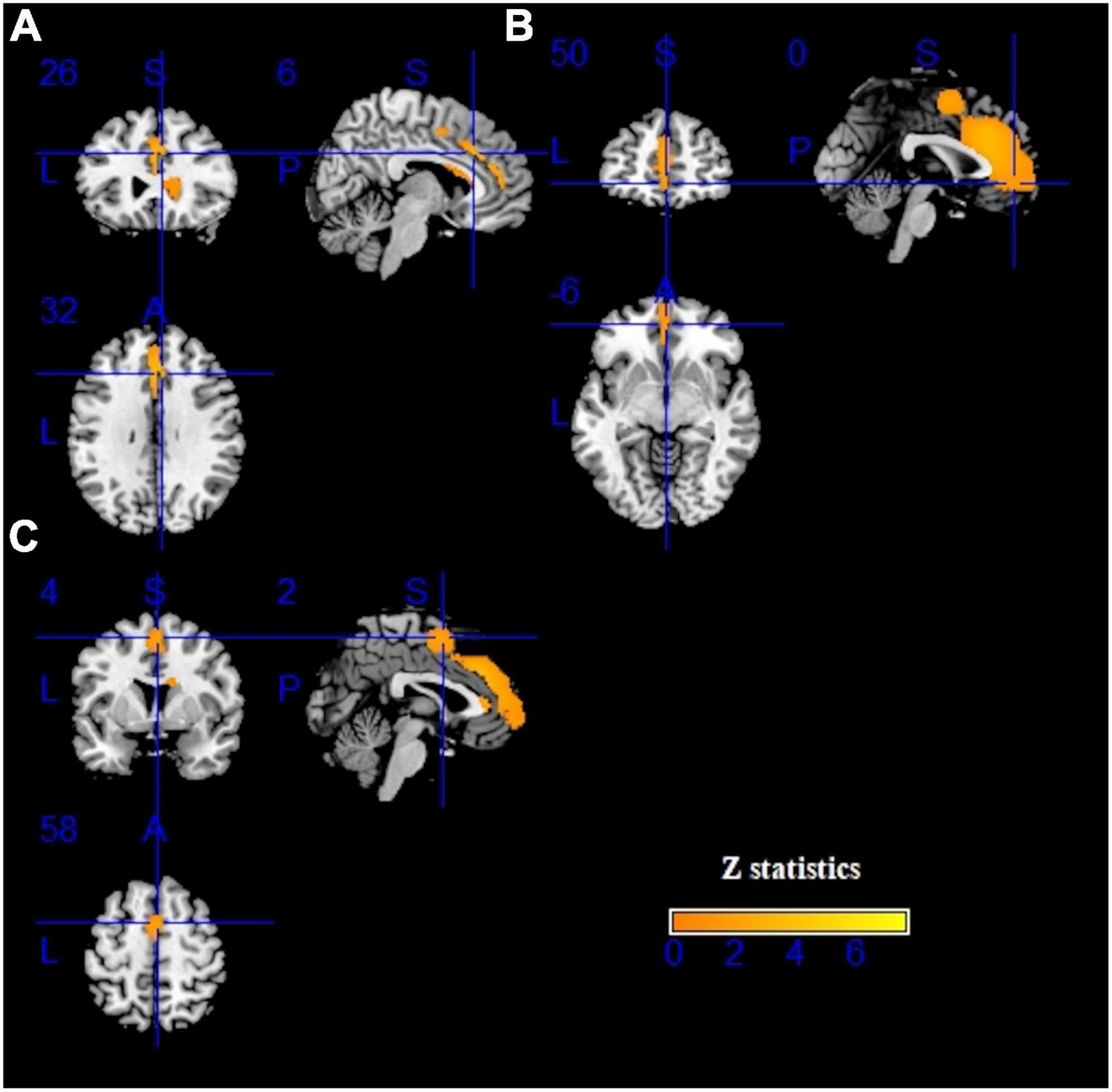
Figure 2. Results of voxel-based morphometry (VBM) for internet gaming disorder (IGD). Gray matter volume (GMV) reductions were displayed in yellow shown in the sagittal, axial, and coronal planes separately. (A–C) show three different brain regions.
Functional magnetic resonance imaging meta-analysis
Pooling across all fMRI studies, IGDs showed significantly higher activation in the bilateral inferior frontal gyrus (IFG), precentral gyrus, left precuneus, right inferior temporal gyrus (ITG), right supramarginal and right fusiform compared with HCs (Figure 3 and Table 2). Besides, lower activation was also detected in the bilateral lingual, calcarine and left middle frontal gyrus (MFG) (Figure 4 and Table 2).
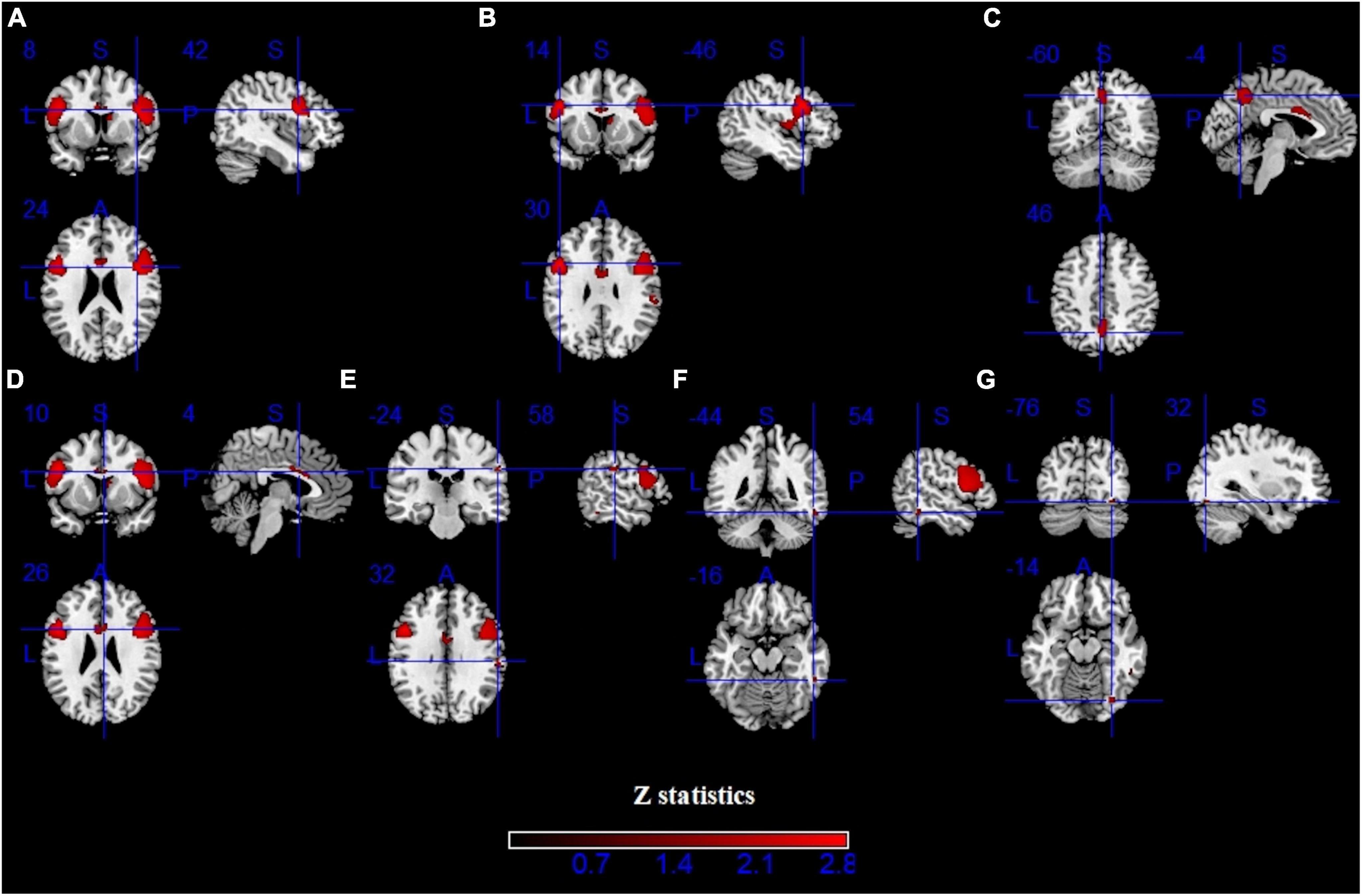
Figure 3. Results of functional magnetic resonance imaging (fMRI) for IGD. Clusters with hyperactivation were shown in red. (A–G) show seven different brain regions.
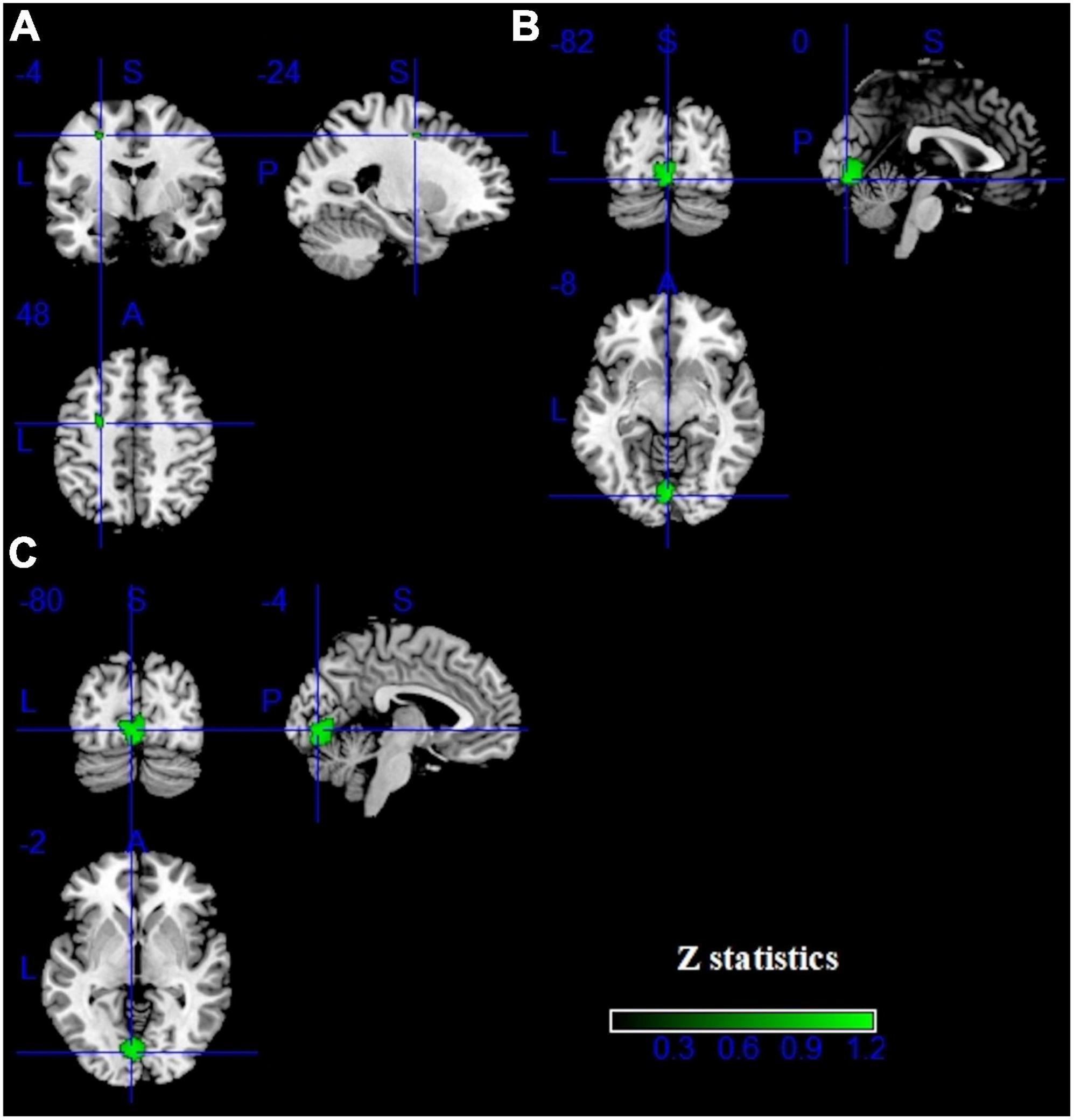
Figure 4. Results of fMRI for IGD. Clusters with hypoactivation were shown in green. (A–C) show three different brain regions.
Multimodal voxel-based morphometry and functional magnetic resonance imaging analysis
Compared with healthy controls, IGD subjects showed both decreased GMV and increased activation in the left ACC (MNI coordinates, 0, 20, 22; 15 voxels) (Figure 5).
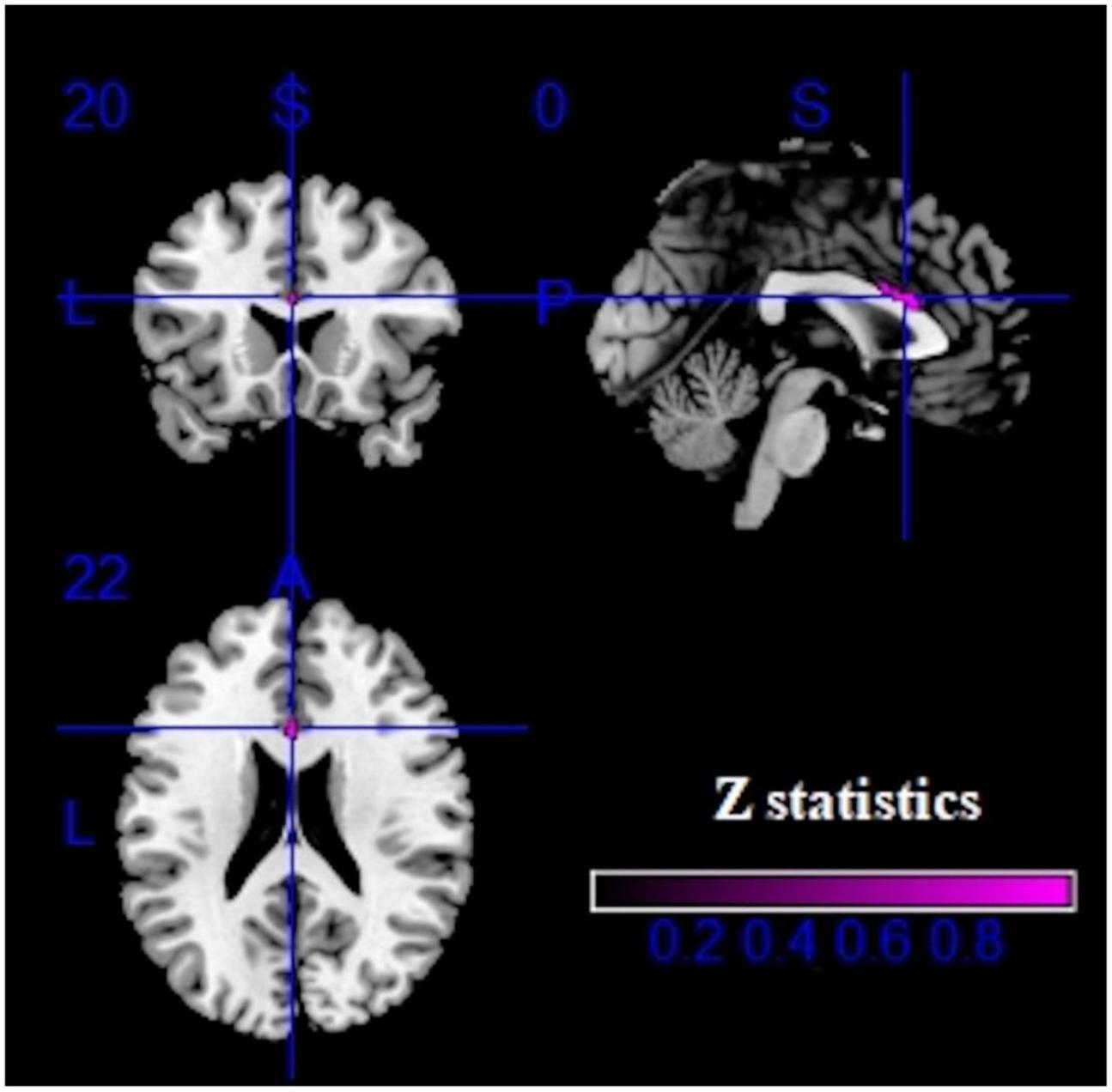
Figure 5. Multimodal analysis results in the IGD. Purple clusters represent reduced GMV and increased brain activation.
Reliability analyses
For the VBM meta-analysis, a systematic whole-brain jackknife sensitivity analysis revealed a highly robust GMV decrease in bilateral anterior cingulate cortex (ACC) and median cingulate cortex (MCC) because these results were replicable in all 15 datasets. Alterations in the supplementary motor area (SMA) and inferior frontal gyrus (IFG) remained highly replicable, as they could be identified in at least 12 and 10 of the 15 combinations respectively. For the fMRI meta-analysis, hypoactivation of bilateral lingual was highly replicable, as these findings were preserved throughout all 30 combinations of datasets. Besides, the results in the left calcarine and left middle frontal gyrus were also significant because they could be identified in 27 and 26 datasets respectively. Hyperactivation of bilateral inferior frontal gyrus, right supramarginal, right fusiform, left precuneus and right inferior temporal gyrus (ITG) remained robust as at least 25 of the 30 combinations were identifiable (Table 2).
Meta-regression
In our study, the variables explored by meta-regression analysis included the BIS-11 score and IAT score. The results showed that GMV reduction in left ACC (MNI coordinate, 0, 28, 22; SDM-Z, −3.067; p = 0.00001; 477 voxels) was positively correlated with BIS-11 score [r = 0.725, p = 0.012(uncorrected)] and IAT score [r = 0.761, p = 0.017(uncorrected)] (Figure 6).
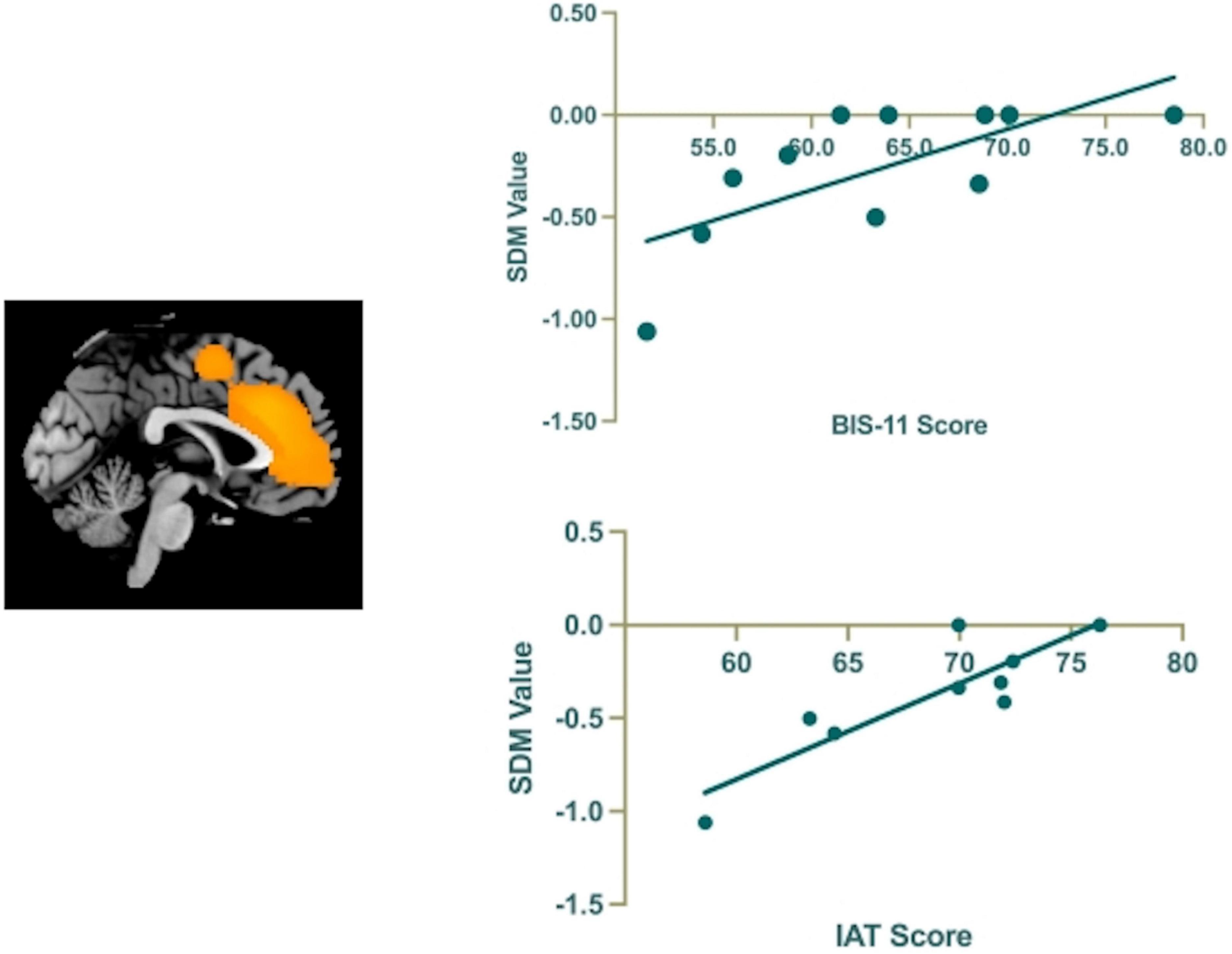
Figure 6. The results of the meta-regression analysis show that the BIS-11 and IAT scores are positively correlated to regional GMV reduction in the left anterior cingulate cortex (ACC).
Discussion
Our study integrated the results of 15 VBM studies and 30 fMRI studies to explore changes in brain GMV and functional neural activation in IGD patients compared to healthy controls, using an anisotropic seed d-Mapping (AES-SDM) method. The result demonstrated that GMV reduction was found in the anterior cingulate cortex (ACC), median cingulate cortex (MCC), and supplementary motor area (SMA). Functionally, IGDs as compared with HCs showed significantly higher activation in the left precuneus, right inferior temporal gyrus (ITG), fusiform and inferior frontal gyrus (IFG). Besides, lower activation in the left middle frontal gyrus (MFG) and the lingual was also detected, stable and replicable under jack-knife sensitivity analysis. In addition, both decreased GMV and increased activation was found in the left ACC among IGD patients. Moreover, meta-regression analysis revealed that higher BIS-11 score and IAT score were correlated with decreased GMV in the left ACC. These findings could become a preliminary implication of neural structural and functional biomarkers in IGD and may help develop effective interventions in these brain regions as potential therapeutic neuro-target for IGD.
Consistent with previous findings in IGD (26, 76), robust GMV decrease in brain regions involved in executive control, namely, the ACC and the SMA (77). The ACC plays important roles in the neural activity of several large-scale networks (78) and is critically involved in multiple processes including cognitive control (79), emotional regulation (80), reward-based decision-making (81), conflict monitoring and error processing (82).
Unsurprisingly, the results of the VBM and fMRI meta-analyses converged on the ACC, showing both functional hyperactivation and gray-matter reduction in IGDs as compared to HCs, which is consistent with previous studies suggesting that structural or functional alterations in the region lead to impaired executive control (10, 83). A study by Wang et al. (76) showed that the GMV of the ACC was negatively correlated with conflict monitoring in the Stroop task. As a functional imaging study demonstrated, IGD individuals exhibited altered brain activity in the ACC during error processing (56). Although numerous executive control problems have been implicated in IGD as mentioned above, IGD is most consistently associated with high impulsivity (28). Conflict monitoring capacity is closely associated with impulse control (84). Our results showed that higher BIS-11 scores are significantly associated with decreased GMV in the left ACC, suggesting that high impulsivity in IGD patients may be due to structural abnormalities in the executive control regions of the brain (38, 76). Although the direction of the alteration of the ACC is inconsistent across modalities (fMRI and VBM), there is evidence that an increase or decrease in GMV may not simply correspond to functional neural activation or inactivation (85). Previous studies have been shown that the ACC always activates in the Stroop task involved in conflict monitoring and cognitive control (86–88). In conclusion, fMRI and VBM may reflect the distinctive aspects of neural alterations, it is plausible to postulate that the alterations of the ACC in IGD subjects may play a vital role in a dysfunctional interaction between executive control and reward-based decision making.
We find decreased GMV of SMA in IGD people through VBM analysis. The SMA is capable of controlling internally generated movements and action sequences (89). Spending more time playing computer games and on repetitive motor actions, such as clicking the mouse or hitting the keyboard, may cause structural changes in the SMA. Just like the cingulate gyrus, the SMA also contributes significantly to cognitive control (77) such as integrating sensory information and monitoring conflict (90). A study has shown that disruptions of the SMA can provoke impairments in response inhibition (91). Besides, during a Go/No-Go Task, IGD subjects showed decreased activation of the SMA for response inhibition compared with healthy controls in a functional imaging study (51). We speculate that IGD subjects’ inability to control their urge to play games is associated with a reduction in SMA gray matter involved in response inhibition, consistent with previous evidence that appropriate response inhibition may be a key aspect of impulse control (92).
The findings of lower activation of the MFG in IGD subjects cannot be ignored. It is commonly believed that the MFG contributes to inhibitory control (57, 83, 93). A study (58) has found that the MFG activation was negatively correlated with the Stroop effect in the IGD groups, which is consistent with previous studies on IGD subjects in go/no-go task (94) and switching (95) task. Hypoactivation in the MFG in IGD subjects probably suggests that they may engage less endeavor in controlling their impulses to play games.
The precuneus, also known as the posterior region of the medial parietal cortex, plays an important role in fundamental cognitive functions such as episodic memory retrieval, visual-spatial information, spatially guided behavior, and attentional processing (96). Numerous studies have confirmed that parietal areas are activated during attention-shifting and are activated by visual addiction-related cues when attention is reflexively drawn to salient features of the stimulus (97). Higher brain activation in precuneus in IGD subjects may indicate that they experience more cognitive conflict and require more top-down attention during the addictive Stroop task (98, 99). That means, gaming-related words have attracted IGD subjects’ attention during the addiction Stroop task and the precuneus was activated to promote cue-induced cravings for online gaming.
The fusiform, located in the middle of the ventral temporal lobe, is considered to be one of the most important brain regions in the visual ventral stream and plays an important role in a range of visual cognitive functions, such as the recognition of the face, body and various object features (100–104), color information processing and emotion perception in facial stimulation (105). Furthermore, the fusiform topographically connects the striate cortex to the inferior temporal lobe, which is associated with auditory processing, comprehension and verbal memory (47). We observed significant activation of the fusiform and inferior temporal gyrus, which may indicate that IGD subjects were more focused on the visual and auditory stimuli of games and were reminded of past online gaming experiences, triggering cravings.
What’s more, we also found increased activation in the inferior frontal gyrus (IFG) involved in risk-evaluation (106, 107) and audio-visual information accumulation in decision-making tasks (108), as well as in the regulation between reward and risk levels (20, 106). A study by Dong et al. (56) demonstrated that IGD subjects needed longer time than healthy controls to make decisions and showed greater activation of the inferior frontal gyrus both in WIN and LOSS trials. Another research found that the activation of the IFG had a positive correlation with the risk aversion (106). We speculate the reason for hyperactivation in IFG is that IGD subjects need more endeavors to complete the decision-making task and may affect executive functions needed to perform other tasks such as conflict inhibition. It could also explain that the occurrence of negative outcomes can lead to further negative reinforcement and the continuation of addictive behaviors to avoid negative effects (109).
Limitation
This meta-analysis sheds new light on brain structure and functional changes of IGD, which may have implications for both clinical interventions and future research. However, there are still some limitations that need to be considered. First, it is difficult to rule out the inter-study heterogeneity of methodologies (including MRI machine, slice thickness, pretreatment protocol, and statistical threshold), which may influence our results. Future research should focus on these issues. Second, the meta-analysis was based primarily on peak coordinates rather than raw statistical brain maps, it may not be sufficient enough to detect some results with small or medium effect sizes (30, 110). Third, male participants were dominant in all samples, and we did not analyze neurological differences in IGD patients of different genders. Fourth, due to the integration of cross-sectional studies, the causal relationship between the structural and functional brain changes and the development of addiction still requires careful consideration. As a consequence, longitudinal studies should be focused on in future research.
Conclusion
In summary, our pooled meta-analysis found distinctive brain structural and functional alterations in IGD across different modalities. Evidence from brain functional abnormalities and gray-matter volume alteration converged to show that IGD was associated with brain regions involved in executive control, cognitive function, and reward-based decision-making such as the ACC, the SMA, the precuneus, fusiform and the IFG. Meta-regression analysis further explored the association between brain structural alterations in IGD and clinical information. Our findings stress the neurofunctional and neurostructural biomarkers for IGD, which may help to develop effective interventions for these brain regions as potential behavioral, pharmacological or neurotherapeutic targets. Besides, longitudinal studies should be performed to complement and validate our findings in the future.
Data availability statement
The original contributions presented in this study are included in the article/Supplementary material, further inquiries can be directed to the corresponding author/s.
Author contributions
XN, MZ, XG, ZY, MY, and YZ conceived and designed the study. XN analyzed the data, performed the statistical study, and drafted the manuscript. MZ, YZ, JC, SH, and XG revised the manuscript. All authors contributed to the article and approved the submitted version.
Conflict of interest
The authors declare that the research was conducted in the absence of any commercial or financial relationships that could be construed as a potential conflict of interest.
Publisher’s note
All claims expressed in this article are solely those of the authors and do not necessarily represent those of their affiliated organizations, or those of the publisher, the editors and the reviewers. Any product that may be evaluated in this article, or claim that may be made by its manufacturer, is not guaranteed or endorsed by the publisher.
Supplementary material
The Supplementary Material for this article can be found online at: https://www.frontiersin.org/articles/10.3389/fpsyt.2022.1029344/full#supplementary-material
Supplementary Figure 1 | Funnel plots for significant results.
Footnotes
- ^ https://www.sdmproject.com/
- ^ http://www.sdmproject.com/software/tutorial.pdf
- ^ http://www.sdmproject.com/utilities/?show=Statistics
References
1. Ko CH, Yen JY, Yen CF, Chen CS, Chen CC. The association between internet addiction and psychiatric disorder: a review of the literature. Eur Psychiatry. (2012) 27:1–8. doi: 10.1016/j.eurpsy.2010.04.011
2. Ko C-H, Yen J-Y, Chen S-H, Yang M-J, Lin H-C, Yen C-F. Proposed diagnostic criteria and the screening and diagnosing tool of internet addiction in college students. Compr Psychiatry. (2009) 50:378–84. doi: 10.1016/j.comppsych.2007.05.019
3. Griffiths M. Relationship between gambling and video-game playing: a response to Johansson and Gotestam. Psychol Rep. (2005) 96(3 Pt 1):644–6. doi: 10.2466/pr0.96.3.644-646
4. Han DH, Lyoo IK, Renshaw PF. Differential regional gray matter volumes in patients with on-line game addiction and professional gamers. J Psychiatr Res. (2012) 46:507–15. doi: 10.1016/j.jpsychires.2012.01.004
5. Ko C-H, Liu G-C, Yen J-Y, Chen C-Y, Yen C-F, Chen C-S. Brain correlates of craving for online gaming under cue exposure in subjects with internet gaming addiction and in remitted subjects. Addict Biol. (2013) 18:559–69. doi: 10.1111/j.1369-1600.2011.00405.x
6. Yao Y-W, Wang L-J, Yip SW, Chen P-R, Li S, Xu J, et al. Impaired decision-making under risk is associated with gaming-specific inhibition deficits among college students with internet gaming disorder. Psychiatry Res. (2015) 229:302–9. doi: 10.1016/j.psychres.2015.07.004
7. Choi S-W, Kim HS, Kim G-Y, Jeon Y, Park SM, Lee J-Y, et al. Similarities and differences among internet gaming disorder, gambling disorder and alcohol use disorder: a focus on impulsivity and compulsivity. J Behav Addict. (2014) 3:246–53. doi: 10.1556/JBA.3.2014.4.6
8. Lee HW, Choi J-S, Shin Y-C, Lee J-Y, Jung HY, Kwon JS. Impulsivity in internet addiction: a comparison with pathological gambling. Cyberpsychol Behav Soc Netw. (2012) 15:373–7. doi: 10.1089/cyber.2012.0063
9. Pawlikowski M, Brand M. Excessive internet gaming and decision making: do excessive World of Warcraft players have problems in decision making under risky conditions? Psychiatry Res. (2011) 188:428–33. doi: 10.1016/j.psychres.2011.05.017
10. Ko C-H, Hsieh T-J, Chen C-Y, Yen C-F, Chen C-S, Yen J-Y, et al. Altered brain activation during response inhibition and error processing in subjects with Internet gaming disorder: a functional magnetic imaging study. Eur Arch Psychiatry Clin Neurosci. (2014) 264:661–72. doi: 10.1007/s00406-013-0483-3
11. Liu L, Xue G, Potenza MN, Zhang J-T, Yao Y-W, Xia C-C, et al. Dissociable neural processes during risky decision-making in individuals with internet-gaming disorder. Neuroimage Clin. (2017) 14:741–9. doi: 10.1016/j.nicl.2017.03.010
12. Dong G, Lin X, Hu Y, Lu Q. Brain activity in advantageous and disadvantageous situations: implications for reward/punishment sensitivity in different situations. PLoS One. (2013) 8:e80232. doi: 10.1371/journal.pone.0080232
13. Petry NM, Rehbein F, Gentile DA, Lemmens JS, Rumpf H-J, Mößle T, et al. An international consensus for assessing internet gaming disorder using the new DSM-5 approach. Addiction. (2014) 109:1399–406. doi: 10.1111/add.12457
14. Feng Q, Chen X, Sun J, Zhou Y, Sun Y, Ding W, et al. Voxel-level comparison of arterial spin-labeled perfusion magnetic resonance imaging in adolescents with internet gaming addiction. Behav Brain Funct. (2013) 9:33. doi: 10.1186/1744-9081-9-33
15. Ding W-N, Sun J-H, Sun Y-W, Zhou Y, Li L, Xu J-R, et al. Altered default network resting-state functional connectivity in adolescents with Internet gaming addiction. PLoS One. (2013) 8:e59902. doi: 10.1371/journal.pone.0059902
16. Lin F, Zhou Y, Du Y, Qin L, Zhao Z, Xu J, et al. Abnormal white matter integrity in adolescents with internet addiction disorder: a tract-based spatial statistics study. PLoS One. (2012) 7:e30253. doi: 10.1371/journal.pone.0030253
17. Zhou Y, Lin F-C, Du Y-S, Qin L-D, Zhao Z-M, Xu J-R, et al. Gray matter abnormalities in Internet addiction: a voxel-based morphometry study. Eur J Radiol. (2011) 79:92–5. doi: 10.1016/j.ejrad.2009.10.025
18. Dong G, Devito EE, Du X, Cui Z. Impaired inhibitory control in ‘internet addiction disorder’: a functional magnetic resonance imaging study. Psychiatry Res. (2012) 203:153–8. doi: 10.1016/j.pscychresns.2012.02.001
19. Dong G, Potenza MN. A cognitive-behavioral model of internet gaming disorder: theoretical underpinnings and clinical implications. J Psychiatr Res. (2014) 58:7–11. doi: 10.1016/j.jpsychires.2014.07.005
20. Lin X, Zhou H, Dong G, Du X. Impaired risk evaluation in people with internet gaming disorder: fMRI evidence from a probability discounting task. Prog Neuropsychopharmacol Biol Psychiatry. (2015) 56:142–8. doi: 10.1016/j.pnpbp.2014.08.016
21. Honey CJ, Kötter R, Breakspear M, Sporns O. Network structure of cerebral cortex shapes functional connectivity on multiple time scales. Proc Natl Acad Sci USA. (2007) 104:10240–5. doi: 10.1073/pnas.0701519104
22. Han S, Xu Y, Guo H-R, Fang K, Wei Y, Liu L, et al. Two distinct subtypes of obsessive compulsive disorder revealed by a framework integrating multimodal neuroimaging information. Hum Brain Mapp. (2022) 43:4254–65. doi: 10.1002/hbm.25951
23. Han S, Zheng R, Li S, Liu L, Wang C, Jiang Y, et al. Progressive brain structural abnormality in depression assessed with MR imaging by using causal network analysis. Psychol Med. (2021):1–10. doi: 10.1017/S0033291721003986
24. Wang C, Zhang Z, Che L, Wu Y, Qian H, Guo X. The gray matter volume in superior frontal gyrus mediates the impact of reflection on emotion in internet gaming addicts. Psychiatry Res Neuroimaging. (2021) 310:111269. doi: 10.1016/j.pscychresns.2021.111269
25. Ko C-H, Liu G-C, Hsiao S, Yen J-Y, Yang M-J, Lin W-C, et al. Brain activities associated with gaming urge of online gaming addiction. J Psychiatr Res. (2009) 43:739–47. doi: 10.1016/j.jpsychires.2008.09.012
26. Yuan K, Qin W, Wang G, Zeng F, Zhao L, Yang X, et al. Microstructure abnormalities in adolescents with internet addiction disorder. PLoS One. (2011) 6:e20708. doi: 10.1371/journal.pone.0020708
27. Widyanto L, Griffiths MD, Brunsden V. A psychometric comparison of the internet addiction test, the internet-related problem scale, and self-diagnosis. Cyberpsychol Behav Soc Netw. (2011) 14:141–9. doi: 10.1089/cyber.2010.0151
28. Cao F, Su L, Liu T, Gao X. The relationship between impulsivity and internet addiction in a sample of Chinese adolescents. Eur Psychiatry. (2007) 22:466–71. doi: 10.1016/j.eurpsy.2007.05.004
29. Fineberg NA, Chamberlain SR, Goudriaan AE, Stein DJ, Vanderschuren LJMJ, Gillan CM, et al. New developments in human neurocognition: clinical, genetic, and brain imaging correlates of impulsivity and compulsivity. CNS Spectr. (2014) 19:69–89. doi: 10.1017/S1092852913000801
30. Yao Y-W, Liu L, Ma S-S, Shi X-H, Zhou N, Zhang J-T, et al. Functional and structural neural alterations in internet gaming disorder: a systematic review and meta-analysis. Neurosci Biobehav Rev. (2017) 83:313–24. doi: 10.1016/j.neubiorev.2017.10.029
31. Remijnse PL, van den Heuvel OA, Nielen MMA, Vriend C, Hendriks G-J, Hoogendijk WJG, et al. Cognitive inflexibility in obsessive-compulsive disorder and major depression is associated with distinct neural correlates. PLoS One. (2013) 8:e59600. doi: 10.1371/journal.pone.0059600
32. Goodkind M, Eickhoff SB, Oathes DJ, Jiang Y, Chang A, Jones-Hagata LB, et al. Identification of a common neurobiological substrate for mental illness. JAMA Psychiatry. (2015) 72:305–15. doi: 10.1001/jamapsychiatry.2014.2206
33. Norman LJ, Carlisi C, Lukito S, Hart H, Mataix-Cols D, Radua J, et al. Structural and functional brain abnormalities in attention-deficit/hyperactivity disorder and obsessive-compulsive disorder: a comparative meta-analysis. JAMA Psychiatry. (2016) 73:815–25. doi: 10.1001/jamapsychiatry.2016.0700
34. Yang Z, Zhang Y, Cheng J, Zheng R. Meta-analysis of brain gray matter changes in chronic smokers. Eur J Radiol. (2020) 132:109300. doi: 10.1016/j.ejrad.2020.109300
35. Zhang M, Gao X, Yang Z, Wen M, Huang H, Zheng R, et al. Shared gray matter alterations in subtypes of addiction: a voxel-wise meta-analysis. Psychopharmacology (Berl). (2021) 238:2365–79. doi: 10.1007/s00213-021-05920-w
36. Radua J, Mataix-Cols D. Voxel-wise meta-analysis of grey matter changes in obsessive-compulsive disorder. Br J Psychiatry. (2009) 195:393–402. doi: 10.1192/bjp.bp.108.055046
37. Egger M, Smith GD, Phillips AN. Meta-analysis: principles and procedures. BMJ (Clin Res ed). (1997) 315:1533–7. doi: 10.1136/bmj.315.7121.1533
38. Du X, Qi X, Yang Y, Du G, Gao P, Zhang Y, et al. Altered structural correlates of impulsivity in adolescents with internet gaming disorder. Front Hum Neurosci. (2016) 10:4. doi: 10.3389/fnhum.2016.00004
39. He Q, Turel O, Wei L, Bechara A. Structural brain differences associated with extensive massively-multiplayer video gaming. Brain Imaging Behav. (2021) 15:364–74. doi: 10.1007/s11682-020-00263-0
40. Jin C, Zhang T, Cai C, Bi Y, Li Y, Yu D, et al. Abnormal prefrontal cortex resting state functional connectivity and severity of internet gaming disorder. Brain Imaging Behav. (2016) 10:719–29. doi: 10.1007/s11682-015-9439-8
41. Ko C-H, Hsieh T-J, Wang P-W, Lin W-C, Yen C-F, Chen C-S, et al. Altered gray matter density and disrupted functional connectivity of the amygdala in adults with internet gaming disorder. Prog Neuropsychopharmacol Biol Psychiatry. (2015) 57:185–92. doi: 10.1016/j.pnpbp.2014.11.003
42. Lee D, Namkoong K, Lee J, Jung Y-C. Abnormal gray matter volume and impulsivity in young adults with internet gaming disorder. Addict Biol. (2018) 23:1160–7. doi: 10.1111/adb.12552
43. Lee D, Namkoong K, Lee J, Jung Y-C. Preliminary evidence of altered gray matter volume in subjects with internet gaming disorder: associations with history of childhood attention-deficit/hyperactivity disorder symptoms. Brain Imaging Behav. (2019) 13:660–8. doi: 10.1007/s11682-018-9872-6
44. Lin X, Dong G, Wang Q, Du X. Abnormal gray matter and white matter volume in ‘internet gaming addicts’. Addict Behav. (2015) 40:137–43. doi: 10.1016/j.addbeh.2014.09.010
45. Mohammadi B, Szycik GR, Te Wildt B, Heldmann M, Samii A, Münte TF. Structural brain changes in young males addicted to video-gaming. Brain Cogn. (2020) 139:105518. doi: 10.1016/j.bandc.2020.105518
46. Seok J-W, Sohn J-H. Altered gray matter volume and resting-state connectivity in individuals with internet gaming disorder: a voxel-based morphometry and resting-state functional magnetic resonance imaging study. Front Psychiatry. (2018) 9:77. doi: 10.3389/fpsyt.2018.00077
47. Sun Y, Sun J, Zhou Y, Ding W, Chen X, Zhuang Z, et al. Assessment of in vivo microstructure alterations in gray matter using DKI in internet gaming addiction. Behav Brain Funct. (2014) 10:37. doi: 10.1186/1744-9081-10-37
48. Weng C-B, Qian R-B, Fu X-M, Lin B, Han X-P, Niu C-S, et al. Gray matter and white matter abnormalities in online game addiction. Eur J Radiol. (2013) 82:1308–12. doi: 10.1016/j.ejrad.2013.01.031
49. Yoon EJ, Choi J-S, Kim H, Sohn BK, Jung HY, Lee J-Y, et al. Altered hippocampal volume and functional connectivity in males with internet gaming disorder comparing to those with alcohol use disorder. Sci Rep. (2017) 7:5744. doi: 10.1038/s41598-017-06057-7
50. Lee D, Namkoong K, Lee J, Jung Y-C. Dorsal striatal functional connectivity changes in internet gaming disorder: a longitudinal magnetic resonance imaging study. Addict Biol. (2021) 26:e12868. doi: 10.1111/adb.12868
51. Chen C-Y, Huang M-F, Yen J-Y, Chen C-S, Liu G-C, Yen C-F, et al. Brain correlates of response inhibition in internet gaming disorder. Psychiatry Clin Neurosci. (2015) 69:201–9. doi: 10.1111/pcn.12224
52. Chun JW, Choi J, Cho H, Lee SK, Kim DJ. Dysfunction of the frontolimbic region during swear word processing in young adolescents with internet gaming disorder. Transl Psychiatry. (2015) 5:e624. doi: 10.1038/tp.2015.106
53. Dieter J, Hill H, Sell M, Reinhard I, Vollstädt-Klein S, Kiefer F, et al. Avatar’s neurobiological traces in the self-concept of massively multiplayer online role-playing game (MMORPG) addicts. Behav Neurosci. (2015) 129:8–17. doi: 10.1037/bne0000025
54. Ding W-N, Sun J-H, Sun Y-W, Chen X, Zhou Y, Zhuang Z-G, et al. Trait impulsivity and impaired prefrontal impulse inhibition function in adolescents with internet gaming addiction revealed by a Go/No-Go fMRI study. Behav Brain Funct. (2014) 10:20. doi: 10.1186/1744-9081-10-20
55. Dong G, Potenza MN. Risk-taking and risky decision-making in internet gaming disorder: implications regarding online gaming in the setting of negative consequences. J Psychiatr Res. (2016) 73:1–8. doi: 10.1016/j.jpsychires.2015.11.011
56. Dong G, Hu Y, Lin X, Lu Q. What makes internet addicts continue playing online even when faced by severe negative consequences? Possible explanations from an fMRI study. Biol Psychol. (2013) 94:282–9. doi: 10.1016/j.biopsycho.2013.07.009
57. Dong G, Huang J, Du X. Enhanced reward sensitivity and decreased loss sensitivity in internet addicts: an fMRI study during a guessing task. J Psychiatr Res. (2011) 45:1525–9. doi: 10.1016/j.jpsychires.2011.06.017
58. Dong G, Li H, Wang L, Potenza MN. Cognitive control and reward/loss processing in Internet gaming disorder: results from a comparison with recreational internet game-users. Eur Psychiatry. (2017) 44:30–8. doi: 10.1016/j.eurpsy.2017.03.004
59. Han DH, Kim SM, Lee YS, Renshaw PF. The effect of family therapy on the changes in the severity of on-line game play and brain activity in adolescents with on-line game addiction. Psychiatry Res. (2012) 202:126–31. doi: 10.1016/j.pscychresns.2012.02.011
60. Lee D, Lee J, Yoon KJ, Kee N, Jung Y-C. Impaired anterior insular activation during risky decision making in young adults with internet gaming disorder. Neuroreport. (2016) 27:605–9. doi: 10.1097/WNR.0000000000000584
61. Lee J, Lee S, Chun JW, Cho H, Kim D-J, Jung Y-C. Compromised prefrontal cognitive control over emotional interference in adolescents with internet gaming disorder. Cyberpsychol Behav Soc Netw. (2015) 18:661–8. doi: 10.1089/cyber.2015.0231
62. Leménager T, Dieter J, Hill H, Koopmann A, Reinhard I, Sell M, et al. Neurobiological correlates of physical self-concept and self-identification with avatars in addicted players of Massively Multiplayer Online Role-Playing Games (MMORPGs). Addict Behav. (2014) 39:1789–97. doi: 10.1016/j.addbeh.2014.07.017
63. Liu L, Yip SW, Zhang J-T, Wang L-J, Shen Z-J, Liu B, et al. Activation of the ventral and dorsal striatum during cue reactivity in internet gaming disorder. Addict Biol. (2017) 22:791–801. doi: 10.1111/adb.12338
64. Liu G-C, Yen J-Y, Chen C-Y, Yen C-F, Chen C-S, Lin W-C, et al. Brain activation for response inhibition under gaming cue distraction in internet gaming disorder. Kaohsiung J Med Sci. (2014) 30:43–51. doi: 10.1016/j.kjms.2013.08.005
65. Lorenz RC, Krüger J-K, Neumann B, Schott BH, Kaufmann C, Heinz A, et al. Cue reactivity and its inhibition in pathological computer game players. Addict Biol. (2013) 18:134–46. doi: 10.1111/j.1369-1600.2012.00491.x
66. Ma S-S, Worhunsky PD, Xu J-S, Yip SW, Zhou N, Zhang J-T, et al. Alterations in functional networks during cue-reactivity in internet gaming disorder. J Behav Addict. (2019) 8:277–87. doi: 10.1556/2006.8.2019.25
67. Qi X, Du X, Yang Y, Du G, Gao P, Zhang Y, et al. Decreased modulation by the risk level on the brain activation during decision making in adolescents with internet gaming disorder. Front Behav Neurosci. (2015) 9:296. doi: 10.3389/fnbeh.2015.00296
68. Qi X, Yang Y, Dai S, Gao P, Du X, Zhang Y, et al. Effects of outcome on the covariance between risk level and brain activity in adolescents with internet gaming disorder. Neuroimage Clin. (2016) 12:845–51. doi: 10.1016/j.nicl.2016.10.024
69. Shin Y-B, Kim H, Kim S-J, Kim J-J. A neural mechanism of the relationship between impulsivity and emotion dysregulation in patients with internet gaming disorder. Addict Biol. (2021) 26:e12916. doi: 10.1111/adb.12916
70. Sun Y, Ying H, Seetohul RM, Xuemei W, Ya Z, Qian L, et al. Brain fMRI study of crave induced by cue pictures in online game addicts (male adolescents). Behav Brain Res. (2012) 233:563–76. doi: 10.1016/j.bbr.2012.05.005
71. Wang Y, Wu L, Wang L, Zhang Y, Du X, Dong G. Impaired decision-making and impulse control in internet gaming addicts: evidence from the comparison with recreational internet game users. Addict Biol. (2017) 22:1610–21. doi: 10.1111/adb.12458
72. Zhang Y, Lin X, Zhou H, Xu J, Du X, Dong G. Brain activity toward gaming-related cues in internet gaming disorder during an addiction stroop task. Front Psychol. (2016) 7:714. doi: 10.3389/fpsyg.2016.00714
73. Zhang J-T, Yao Y-W, Potenza MN, Xia C-C, Lan J, Liu L, et al. Effects of craving behavioral intervention on neural substrates of cue-induced craving in internet gaming disorder. Neuroimage Clin. (2016) 12:591–9. doi: 10.1016/j.nicl.2016.09.004
74. Turel O, He Q, Wei L, Bechara A. The role of the insula in internet gaming disorder. Addict Biol. (2021) 26:e12894. doi: 10.1111/adb.12894
75. Wang L, Yang G, Zheng Y, Li Z, Qi Y, Li Q, et al. Enhanced neural responses in specific phases of reward processing in individuals with internet gaming disorder. J Behav Addict. (2021) 10:99–111. doi: 10.1556/2006.2021.00003
76. Wang H, Jin C, Yuan K, Shakir TM, Mao C, Niu X, et al. The alteration of gray matter volume and cognitive control in adolescents with internet gaming disorder. Front Behav Neurosci. (2016) 9:64. doi: 10.3389/fnbeh.2015.00064
77. Nachev P, Kennard C, Husain M. Functional role of the supplementary and pre-supplementary motor areas. Nat Rev Neurosci. (2008) 9:856–69. doi: 10.1038/nrn2478
78. Sutherland MT, McHugh MJ, Pariyadath V, Stein EA. Resting state functional connectivity in addiction: lessons learned and a road ahead. Neuroimage. (2012) 62:2281–95. doi: 10.1016/j.neuroimage.2012.01.117
79. Meng Y, Deng W, Wang H, Guo W, Li T. The prefrontal dysfunction in individuals with internet gaming disorder: a meta-analysis of functional magnetic resonance imaging studies. Addict Biol. (2015) 20:799–808. doi: 10.1111/adb.12154
80. Etkin A, Egner T, Kalisch R. Emotional processing in anterior cingulate and medial prefrontal cortex. Trends Cogn Sci. (2011) 15:85–93. doi: 10.1016/j.tics.2010.11.004
81. Kennerley SW, Walton ME, Behrens TEJ, Buckley MJ, Rushworth MFS. Optimal decision making and the anterior cingulate cortex. Nat Neurosci. (2006) 9:940–7. doi: 10.1038/nn1724
82. Dong G, Shen Y, Huang J, Du X. Impaired error-monitoring function in people with internet addiction disorder: an event-related fMRI study. Eur Addict Res. (2013) 19:269–75. doi: 10.1159/000346783
83. Wang L, Wu L, Lin X, Zhang Y, Zhou H, Du X, et al. Dysfunctional default mode network and executive control network in people with internet gaming disorder: independent component analysis under a probability discounting task. Eur Psychiatry. (2016) 34:36–42. doi: 10.1016/j.eurpsy.2016.01.2424
84. Checa P, Castellanos MC, Abundis-Gutiérrez A, Rosario Rueda M. Development of neural mechanisms of conflict and error processing during childhood: implications for self-regulation. Front Psychol. (2014) 5:326. doi: 10.3389/fpsyg.2014.00326
85. Calhoun VD, Adali T, Giuliani NR, Pekar JJ, Kiehl KA, Pearlson GD. Method for multimodal analysis of independent source differences in schizophrenia: combining gray matter structural and auditory oddball functional data. Hum Brain Mapp. (2006) 27:47–62. doi: 10.1002/hbm.20166
86. Aarts E, Roelofs A. Attentional control in anterior cingulate cortex based on probabilistic cueing. J Cogn Neurosci. (2011) 23:716–27. doi: 10.1162/jocn.2010.21435
87. Botvinick MM, Cohen JD, Carter CS. Conflict monitoring and anterior cingulate cortex: an update. Trends Cogn Sci. (2004) 8:539–46. doi: 10.1016/j.tics.2004.10.003
88. Floden D, Vallesi A, Stuss DT. Task context and frontal lobe activation in the stroop task. J Cogn Neurosci. (2011) 23:867–79. doi: 10.1162/jocn.2010.21492
89. Zhang S, Ide JS, Li CS. Resting-state functional connectivity of the medial superior frontal cortex. Cereb Cortex. (2012) 22:99–111. doi: 10.1093/cercor/bhr088
90. Liu J, Gao X-P, Osunde I, Li X, Zhou S-K, Zheng H-R, et al. Increased regional homogeneity in internet addiction disorder: a resting state functional magnetic resonance imaging study. Chin Med J (Engl). (2010) 123:1904–8.
91. Picton TW, Stuss DT, Alexander MP, Shallice T, Binns MA, Gillingham S. Effects of focal frontal lesions on response inhibition. Cereb Cortex. (2007) 17:826–38. doi: 10.1093/cercor/bhk031
92. Bari A, Robbins TW. Inhibition and impulsivity: behavioral and neural basis of response control. Prog Neurobiol. (2013) 108:44–79. doi: 10.1016/j.pneurobio.2013.06.005
93. Dong G, Lin X, Zhou H, Du X. Decision-making after continuous wins or losses in a randomized guessing task: implications for how the prior selection results affect subsequent decision-making. Behav Brain Funct. (2014) 10:11. doi: 10.1186/1744-9081-10-11
94. Dong G, Zhou H, Zhao X. Impulse inhibition in people with internet addiction disorder: electrophysiological evidence from a Go/NoGo study. Neurosci Lett. (2010) 485:138–42. doi: 10.1016/j.neulet.2010.09.002
95. Dong G, Lin X, Zhou H, Lu Q. Cognitive flexibility in internet addicts: fMRI evidence from difficult-to-easy and easy-to-difficult switching situations. Addict Behav. (2014) 39:677–83. doi: 10.1016/j.addbeh.2013.11.028
96. Cavanna AE, Trimble MR. The precuneus: a review of its functional anatomy and behavioural correlates. Brain. (2006) 129(Pt 3):564–83. doi: 10.1093/brain/awl004
97. Hassabis D, Kumaran D, Vann SD, Maguire EA. Patients with hippocampal amnesia cannot imagine new experiences. Proc Natl Acad Sci USA. (2007) 104:1726–31. doi: 10.1073/pnas.0610561104
98. Peterson BS, Skudlarski P, Gatenby JC, Zhang H, Anderson AW, Gore JC. An fMRI study of Stroop word-color interference: evidence for cingulate subregions subserving multiple distributed attentional systems. Biol Psychiatry. (1999) 45:1237–58. doi: 10.1016/S0006-3223(99)00056-6
99. Mitchell RLC. The BOLD response during Stroop task-like inhibition paradigms: effects of task difficulty and task-relevant modality. Brain Cogn. (2005) 59:23–37. doi: 10.1016/j.bandc.2005.04.001
100. Kanwisher N, McDermott J, Chun MM. The fusiform face area: a module in human extrastriate cortex specialized for face perception. J Neurosci. (1997) 17:4302–11. doi: 10.1523/JNEUROSCI.17-11-04302.1997
101. Sergent J, Ohta S, MacDonald B. Functional neuroanatomy of face and object processing. A positron emission tomography study. Brain. (1992) 115(Pt 1):15–36. doi: 10.1093/brain/115.1.15
102. Taylor JC, Wiggett AJ, Downing PE. Functional MRI analysis of body and body part representations in the extrastriate and fusiform body areas. J Neurophysiol. (2007) 98:1626–33. doi: 10.1152/jn.00012.2007
103. Schwarzlose RF, Swisher JD, Dang S, Kanwisher N. The distribution of category and location information across object-selective regions in human visual cortex. Proc Natl Acad Sci USA. (2008) 105:4447–52. doi: 10.1073/pnas.0800431105
104. Wang X, Han Z, He Y, Caramazza A, Song L, Bi Y. Where color rests: spontaneous brain activity of bilateral fusiform and lingual regions predicts object color knowledge performance. Neuroimage. (2013) 76:252–63. doi: 10.1016/j.neuroimage.2013.03.010
105. Weiner KS, Golarai G, Caspers J, Chuapoco MR, Mohlberg H, Zilles K, et al. The mid-fusiform sulcus: a landmark identifying both cytoarchitectonic and functional divisions of human ventral temporal cortex. Neuroimage. (2014) 84:453–65. doi: 10.1016/j.neuroimage.2013.08.068
106. Christopoulos GI, Tobler PN, Bossaerts P, Dolan RJ, Schultz W. Neural correlates of value, risk, and risk aversion contributing to decision making under risk. J Neurosci. (2009) 29:12574–83. doi: 10.1523/JNEUROSCI.2614-09.2009
107. Rushworth MFS, Noonan MP, Boorman ED, Walton ME, Behrens TE. Frontal cortex and reward-guided learning and decision-making. Neuron. (2011) 70:1054–69. doi: 10.1016/j.neuron.2011.05.014
108. Noppeney U, Ostwald D, Werner S. Perceptual decisions formed by accumulation of audiovisual evidence in prefrontal cortex. J Neurosci. (2010) 30:7434–46. doi: 10.1523/JNEUROSCI.0455-10.2010
109. Koob GF, Volkow ND. Neurocircuitry of addiction. Neuropsychopharmacology. (2010) 35:217–38. doi: 10.1038/npp.2009.110
Keywords: voxel-based morphometry, functional magnetic resonance imaging, meta-analysis, internet gaming disorder, gray matter volume
Citation: Niu X, Gao X, Zhang M, Yang Z, Yu M, Wang W, Wei Y, Cheng J, Han S and Zhang Y (2022) Meta-analysis of structural and functional brain alterations in internet gaming disorder. Front. Psychiatry 13:1029344. doi: 10.3389/fpsyt.2022.1029344
Received: 27 August 2022; Accepted: 03 October 2022;
Published: 25 October 2022.
Edited by:
Xiaochu Zhang, University of Science and Technology of China, ChinaReviewed by:
Zhiyi Chen, Army Medical University, ChinaRuibin Zhang, Southern Medical University, China
Copyright © 2022 Niu, Gao, Zhang, Yang, Yu, Wang, Wei, Cheng, Han and Zhang. This is an open-access article distributed under the terms of the Creative Commons Attribution License (CC BY). The use, distribution or reproduction in other forums is permitted, provided the original author(s) and the copyright owner(s) are credited and that the original publication in this journal is cited, in accordance with accepted academic practice. No use, distribution or reproduction is permitted which does not comply with these terms.
*Correspondence: Yong Zhang, zzuzhangyong2013@163.com; Jingliang Cheng, cjr.chjl@vip.163.com; Shaoqiang Han, ShaoqiangHan@163.com