- 1Clinical Research Development Unit of Tabriz Valiasr Hospital, Tabriz University of Medical Sciences, Tabriz, Iran
- 2Department of Medical Genetics, School of Medicine, Shahid Beheshti University of Medical Sciences, Tehran, Iran
- 3Department of Medical Genetics, Faculty of Medicine, Tabriz University of Medical Sciences, Tabriz, Iran
- 4Urology and Nephrology Research Center, Shahid Beheshti University of Medical Sciences, Tehran, Iran
- 5Institute of Human Genetics, Jena University Hospital, Jena, Germany
- 6Department of Pharmacognosy, College of Pharmacy, Hawler Medical University, Erbil, Iraq
- 7Center of Research and Strategic Studies, Lebanese French University, Erbil, Iraq
- 8Center for Affective, Stress and Sleep Disorders, Psychiatric Clinics of the University of Basel, Basel, Switzerland
- 9Department of Psychiatric, School of Medicine, Shahid Beheshti University of Medical Sciences, Tehran, Iran
Schizophrenia (SCZ) is a serious mental condition with an unknown cause. According to the reports, Brodmann Area 10 (BA10) is linked to the pathology and cortical dysfunction of SCZ, which demonstrates a number of replicated findings related to research on SCZ and the dysfunction in tasks requiring cognitive control in particular. Genetics' role in the pathophysiology of SCZ is still unclear. Therefore, it may be helpful to understand the effects of these changes on the onset and progression of SCZ to find novel mechanisms involved in the regulation of gene transcription. In order to determine the molecular regulatory mechanisms affecting the SCZ, the long non-coding RNA (lncRNA)-associated competing endogenous RNAs (ceRNAs) axes in the BA10 area were determined using a bioinformatics approach in the present work. A microarray dataset (GSE17612) consisted of brain post-mortem tissues of the BA10 area from SCZ patients and matched healthy subjects was downloaded from the Gene Expression Omnibus (GEO) database. This dataset included probes for both lncRNAs and mRNAs. Using the R software's limma package, the differentially expressed lncRNAs (DElncRNAs) and mRNAs (DEmRNAs) were found. The RNA interactions were also discovered using the DIANA-LncBase and miRTarBase databases. In the ceRNA network, positive correlations between DEmRNAs and DElncRNAs were evaluated using the Pearson correlation coefficient. Finally, lncRNA-associated ceRNA axes were built by using the co-expression and DElncRNA-miRNA-DEmRNA connections. We identified the DElncRNA-miRNA-DEmRNA axes, which included two key lncRNAs (PEG3-AS1, MIR570HG), seven key miRNAs (hsa-miR-124-3p, hsa-miR-17-5p, hsa-miR-181a-5p, hsa-miR-191-5p, hsa-miR-26a-5p, hsa-miR-29a-3p, hsa-miR-29b-3p), and eight key mRNAs (EGR1, ETV1, DUSP6, PLOD2, CD93, SERPINB9, ANGPTL4, TGFB2). Furthermore, DEmRNAs were found to be enriched in the “AGE-RAGE signaling pathway in diabetic complications”, “Amoebiasis”, “Transcriptional misregulation in cancer”, “Human T-cell leukemia virus 1 infection”, and “MAPK signaling pathway”. This study offers research targets for examining significant molecular pathways connected to the pathogenesis of SCZ, even though the function of these ceRNA axes still needs to be investigated.
Introduction
Schizophrenia (SCZ) is a mentally destructive ailment affecting young adults. Its symptoms comprise a spectrum of delusions, hallucinations, and cognitive impairment (1). About one percent of the population is affected by this condition on a worldwide scale (2). It is believed that complicated interactions between genetic and environmental factors contribute to the pathophysiology of SCZ (3, 4). Transcriptional changes in the post-mortem human brain that are correlated with SCZ have been appraised in various studies using microarray or RNA-sequencing (5). Such studies revealed alterations in the expression of genes involved in a variety of biological processes in various parts of the brain, including the anterior prefrontal cortex (Brodmann area 10; BA10) (6–8). To devise new diagnostic techniques and individualized therapies for SCZ, we need to augment our knowledge of molecular processes that are involved in the onset and exacerbation of SCZ. Recent findings about novel mechanisms of gene expression regulation can be useful in understanding how changes in these regulatory mechanisms can affect SCZ formation and progression (9). Non-coding RNAs (ncRNAs) play an important role in the regulation of gene expression. One of the proposed mechanisms for the involvement of these transcripts in the control of gene expression is the competing endogenous RNA (ceRNA) hypothesis (10). In this novel regulatory mechanism, ncRNAs, especially long non-coding RNAs (lncRNAs), regulate other RNA transcripts through sponging shared microRNAs (miRNAs) (11). Cross-talk between RNAs, including coding RNAs and ncRNAs, through miRNA complementary sequences known as miRNA response elements (MREs), produces a large-scale regulatory network throughout the transcriptome, according to this notion. If two RNA transcripts regulate each other by a ceRNA-mediated mechanism, then the expression levels of these two RNA transcripts would be negatively correlated with the levels of target miRNAs and positively correlated with each other (11).
Although multiple investigations have shown that ceRNA networks differ in SCZ (9, 12–14), the role of ceRNA networks in the pathogenesis of SCZ remains to be elucidated, and it is of great importance to evaluate the expression of downstream affected genes by these networks. With the emergence of high throughput techniques such as RNA-sequencing and microarray, assessing the expression of numerous genes simultaneously became possible, and a bulk amount of information about the expression profile of different samples has been made publicly available for the scientific community. Different computational methods can be utilized to analyze these bulk data to unravel potential regulatory mechanisms like ncRNA networks that contribute to SCZ (9, 13, 14). RNAs are more “druggable” than proteins because they can be simply targeted by corresponding complementary sequences. These unique features make it easier and economical to design and develop drugs targeting RNA molecules as novel therapeutic targets (15–17). CeRNA networks contain a variety of transcripts, which makes them useful for exploring possible treatment targets for complex diseases like SCZ, even if only one of them is targeted (i.e., an immediate fluctuation in the levels of various disease-related RNAs) (18).
The objective of this bioinformatics-based study was to identify the expression patterns and relevant lncRNA-associated ceRNA regulatory axes in the BA10 brain region of SCZ patients.
Methods
In the current study, a microarray dataset (GSE17612) consisting of brain post-mortem tissues of the BA10 area from SCZ patients and matched healthy subjects was analyzed. Differentially expressed lncRNAs (DElncRNAs) and mRNAs (DEmRNAs) were extracted from the dataset, and lncRNA-miRNA-mRNAs axes were constructed by utilizing previously published bioinformatic approaches (13).
Data collection of the gene expression profile
We obtained the GSE17612 dataset from the NCBI Gene Expression Omnibus database (GEO, https://www.ncbi.nlm.nih.gov/geo/). In this dataset, the GPL570 platform (HG-U133_Plus_2) was used as microarray chip that included probes for mRNAs and lncRNAs. The GSE17612 dataset contains brain post-mortem tissues of the BA10 area from 28 patients with SCZ and 23 matched healthy subjects (8). Demographic information is shown in Table 1. According to the original study, RNA integrity number (RIN) was employed as the major inclusion criterion to assess the quality of the RNA. The RIN was evaluated using spectrophotometry to estimate the amount of extracted RNA, and an Agilent 2100 Bioanalyzer (South Plainfield, NJ, USA) was used to evaluate the RNA's quality. The RIN was used to classify samples into three quality categories: pass (RIN > 7.0), borderline (RIN 6.0-7.0), and fail (RIN < 6.0) (8). Five samples in the fail group were excluded from the study after classification. In addition, six samples were excluded following the evaluation of hybridization quality (8). A total of 51 samples were included (8).
Data preprocessing and identification of DEmRNAs and DElncRNAs
We employed Robust Multichip Average (RMA) method for background correction and quantile normalization of all primary data records (19). In addition, to exclude insignificant probe sets with no expression, we performed an interquartile range (IQR) filter (IQR across the samples on the log2 scale larger than median IQR), that was followed by an intensity filter (a minimum of >100 expression signals in a minimum of 25% of the arrays) (20). AgiMicroRna Bioconductor package (version 2.46.0) was used for quality control. We applied removeBatchEffect() function from the limma package and added age and gender as covariates in all statistical models to adjust for possible effects. We applied linear models for microarray data (limma) package (version 3.52.2) of R (version 4.2.1) for differential gene expression analysis between patients with SCZ and control subjects (21) in Bioconductor (https://www.bioconductor.org/) (22). We used the same approach in our previous paper to recognize lncRNA probes (13). We downloaded the latest list of lncRNAs from the HUGO Gene Nomenclature Committee (HGNC) (https://www.genenames.org/) with approved symbols (23). Then after, we merged obtained lncRNAs list with the dataset based on their gene symbols to retrieve common lncRNAs between the lncRNAs list and dataset. Student t-test was applied to evaluate the statistical significance of differential expression, and P-value adjustment was carried out using Benjamini-Hochberg method. Cut-off values applied in this study were set as follows: (1) a false discovery rate (adjusted P-value) < 0.001, and (2) |log2 fold change (log2FC) | > 0.5. Finally, a volcano plot and heat map of DEGs was drawn using the Enhanced Volcano (version1.14.0) and the Pheatmap (version 1.0.12) packages.
RNA interaction pairs prediction
We utilized DIANA-LncBase v3 to identify miRNAs targeting DElncRNAs based on experimentally validated interaction (24). The DIANA-LncBase query was performed based on the “Species” of Homo Sapiens and high “miRNA Confidence Levels”. Also, we recognized miRNAs targeting mRNAs using miRTarBase (25); only the interactions with strong experimental evidence were included in the study. By comparing the mRNAs obtained from miRTarBase and the previously identified DEmRNA, the common mRNAs were used to construct the DElncRNA-miRNA-DEmRNA regulatory axes.
Analysis of correlation between DEmRNAs and DElncRNAs, protein–protein interaction (PPI) network analysis, and lncRNA-associated ceRNA axes construction
We investigated the positive correlation between DElncRNAs and DEmRNAs in ceRNA networks using Pearson correlation analysis. DELncRNAs, targeted DEmRNAs, and the interacting miRNAs were omitted upon observing opposing expression patterns between the targeted DEmRNAs and DElncRNAs. The correlations were calculated and illustrated using bcdstats (version 0.0.0.9005) and corrplot (version 0.92) R packages. Inclusion criteria was based on Pearson correlation coefficient (r) > 0.5 and false discovery rate (FDR) < 0.001. The online STRING database (https://string-db.org/) (26) was utilized to create a PPI to prognosticate the interactions among DEmRNAs encoding proteins. For PPI network construction, a combined score of 0.4 (medium confidence) was selected. Visualization of these PPI and construction of ceRNA networks was carried out using Cytoscape software (version 3.8.0) (27).
DEmRNAs pathway enrichment analysis
We performed a KEGG pathway enrichment analysis of DEmRNAs in the ceRNA network using the Enricher tool (28, 29).
Results
Identification of DEmRNAs and DElncRNAs
Before implementing differential expression analysis, background correction, normalization, and gene filtering were carried out. The quality control of the dataset was conducted using the AgiMicroRna Bioconductor package. To assess the distribution of data, we drew box plots of the gene expression data after performing normalization (Supplementary File 1). The medians of expression level were equal for each of the distinct arrays in the box plot, proving that the correction was done correctly.
According to cut-off values for identification of DEGs and DElncRNAs (|log2FC | ≥ 0.5, and adjusted P < 0.001), a total of 183 DEmRNAs and six DElncRNAs were observed between SCZ and control samples. Figures 1, 2 represent the volcano plot of the DEmRNAs and heatmap of DElncRNAs, respectively. Also, we summarized more details about DEGs in Supplementary File 2.
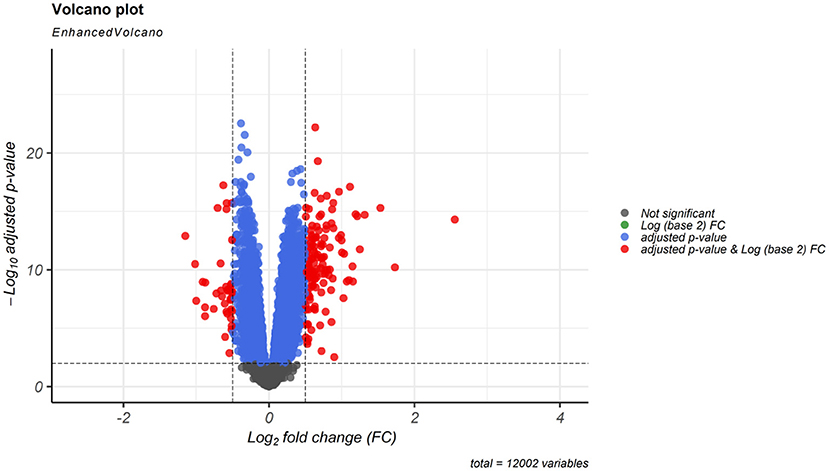
Figure 1. Differentially expressed mRNAs (DEmRNAs) volcano plot. DEmRNAs were screened using a |(log2FC)| ≥ 0.5 and an adjusted P < 0.001 threshold.
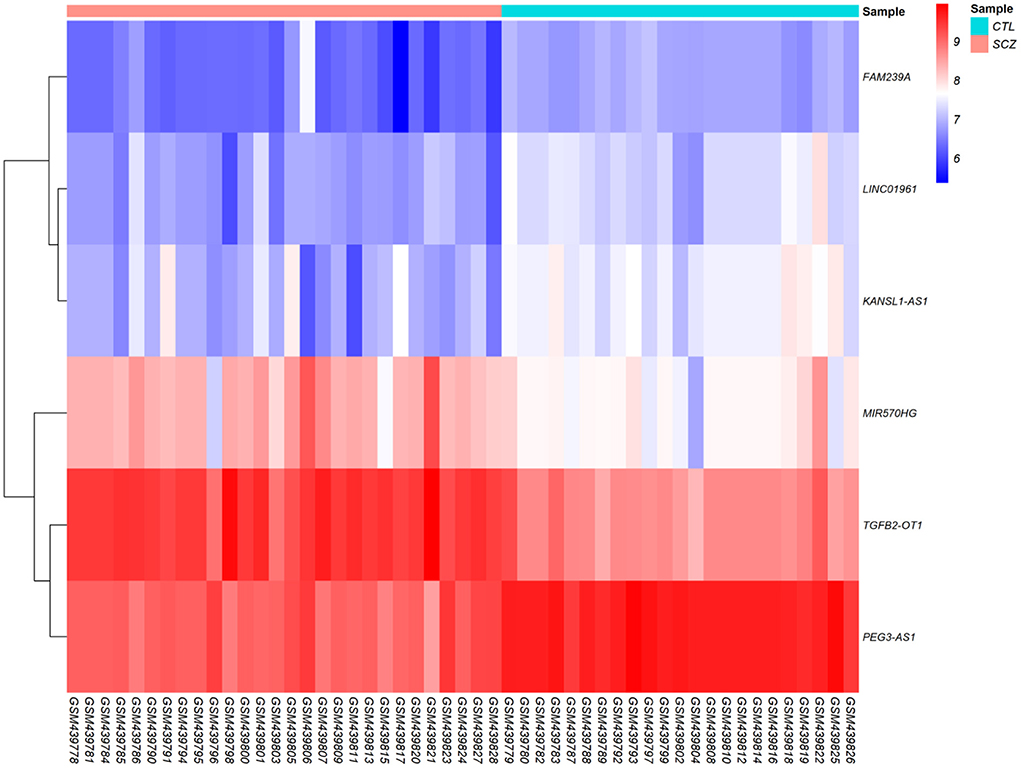
Figure 2. Differentially expressed long non-coding RNAs (DElncRNAs) heatmap. The color values correspond to normalized gene expression data. The color and intensity of the boxes are used to reflect the expression level of DElncRNAs in each sample. The color blue shows those DElncRNAs with low expression, while red represents those with high expression.
Prediction of RNA interaction pairs
We used the DIANA-LncBase ver. 3 online tool to predict miRNAs targeting DElncRNA. Results showed that the miRNAs might target three of the six DElncRNAs. Then we obtained mRNAs targeted by candidate miRNAs using miRTarBase. Lastly, we retrieved 20 overlapping genes by comparing mRNAs obtained from miRTarBase and DEmRNAs. Additional information regarding RNA interaction pairs is provided in Supplementary File 3.
Analysis of correlation between DEmRNAs and DElncRNAs, PPI network analysis, and lncRNA-associated ceRNA axes construction
In this step, we applied Pearson correlation analysis of expression levels between DEmRNAs and DElncRNAs to explore positive correlations to establish the ceRNA hypothesis (mRNAs' expression is positively modulated by lncRNAs through sponging miRNAs; Figure 3). We constructed a ceRNA network based on interactions of DElncRNA, miRNA, and DEmRNA, co-expression pattern of DElncRNA and DEmRNA, and PPIs to indicate the lncRNAs associated ceRNA axes in the brain BA10 region that are related to SCZ (Figure 4). CeRNA axes consisted of two DElncRNAs [PEG3 Antisense RNA 1 (PEG3-AS1), MIR570 Host Gene (MIR570HG)], eight DEmRNAs [Early Growth Response 1 (EGR1), ETS Variant Transcription Factor 1 (ETV1), Dual specificity phosphatase 6 (DUSP6), Procollagen-Lysine, 2-Oxoglutarate 5-Dioxygenase 2 (PLOD2), CD93, Serpin Family B Member 9 (SERPINB9), Angiopoietin Like 4 (ANGPTL4), Transforming Growth Factor Beta 2 (TGFB2)] and seven miRNAs (hsa-miR-124-3p, hsa-miR-17-5p, hsa-miR-181a-5p, hsa-miR-191-5p, hsa-miR-26a-5p, hsa-miR-29a-3p, hsa-miR-29b-3p).
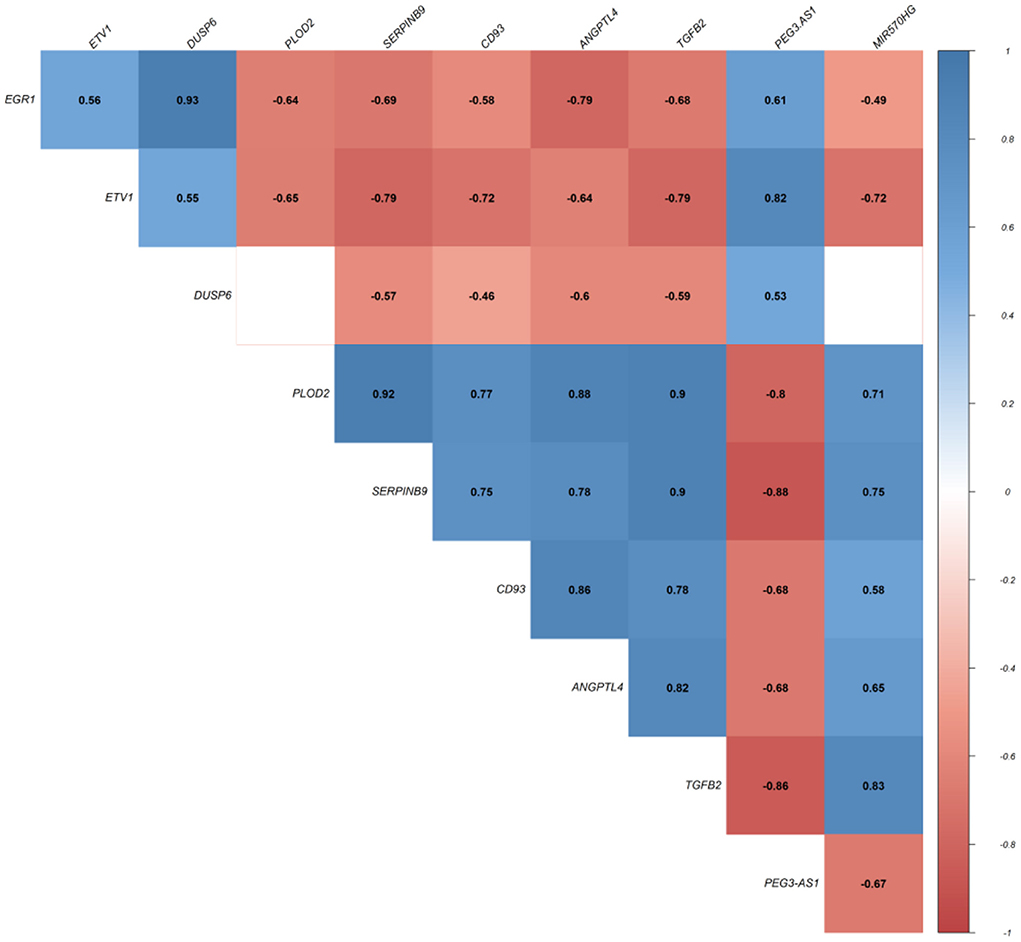
Figure 3. Pearson correlation analysis. Values represent Pearson's correlation coefficient (r). Positive correlations are shown in blue, while negative correlations are represented in red. False discovery rate (FDR) values greater than 0.001 are deemed unimportant (blank), and the intensity is proportional to correlation coefficients.
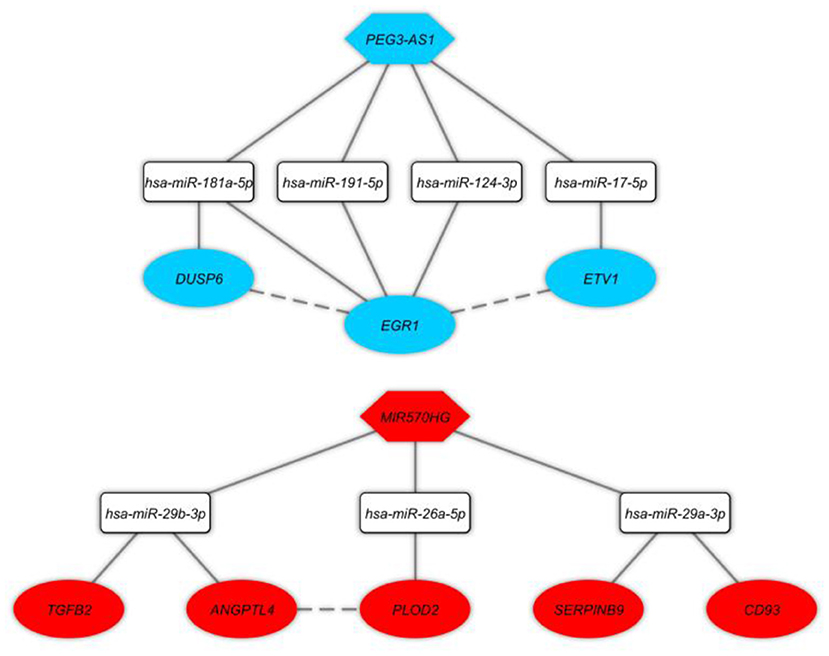
Figure 4. Long non-coding RNA (lncRNA)-associated competing endogenous RNA axes. Red and blue represents upregulation and downregulation of ceRNAs, respectively. LncRNAs, miRNAs, and mRNAs are represented by hexagon, round rectangle, and ellipse, respectively. The dash lines between nodes represent the interactions between proteins.
DEmRNAs pathway enrichment analysis
The KEGG pathway enrichment analysis was performed on all DEmRNAs in the ceRNA network. Hence, the top enriched KEGG pathways are mentioned as follows: “AGE-RAGE signaling pathway in diabetic complications”, “Amoebiasis”, “Transcriptional misregulation in cancer”, “Human T-cell leukemia virus 1 infection”, and “MAPK signaling pathway” (Figure 5).
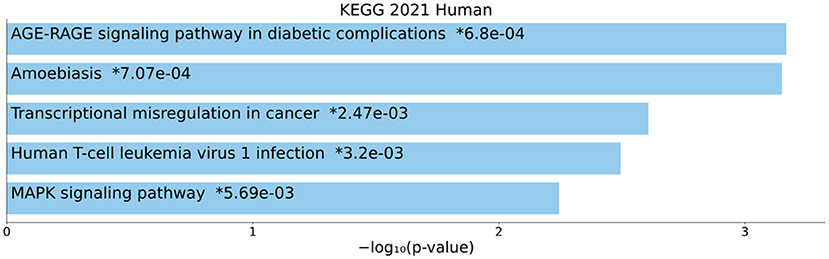
Figure 5. Kyoto Encyclopedia of Genes and Genomes (KEGG) pathway enrichment analysis. The top five enriched pathways are displayed along with the corresponding P-values in a bar graph. Those with significant P-values (i.e., <0.05) are matched with the colored bars. A P-value (shown with *) shows the significant adjusted P-value (<0.05).
Discussion
Several studies have shown brain BA9, and BA10 regions are affected by SCZ pathology and cortical dysfunction. The involvement of these regions is associated with cognitive impairment (7, 8, 30, 31). Furthermore, it has been suggested that ceRNA regulatory axes and their related networks contribute to a variety of neurological disorders (32). Organ, cell, and the subcellular environment have important impacts on the expression level of the ceRNA. Different RNA transcripts such as lncRNAs, circular RNAs (circRNAs), pseudogenes, and mRNAs can be involved in the ceRNA network. LncRNAs are one of the main elements of the ceRNA network and have a pivotal role in both normal and pathological conditions (33). It is believed that lncRNAs have different expression patterns depending on the development stage, cell type, and organ. The expression of lncRNAs is strictly regulated based on their tissue specificity and subcellular localization (34). The lncRNA-associated ceRNA axes may have a critical role in SCZ pathogenesis, according to the aforementioned theoretical assumptions. In the current study, we discovered the DElncRNA-miRNA-DEmRNA, which included two key lncRNAs, seven key miRNAs, and eight key mRNAs.
We found two major lncRNAs (PEG3-AS1, MIR570HG) in this investigation. It has been demonstrated that PEG3-AS1 has been correlated with acute myeloid leukemia (35), colorectal cancer (36), and head and neck squamous cell carcinoma (37); however, the exact function and mechanisms of PEG3-AS1 are poorly understood. As far as we know, the correlation between PEG3-AS1 and SCZ has been reported in this study for the first time; thus, additional research is required to confirm our findings. MIR570HG (originally designated as LINC00969) is another new lncRNA that has been reported to be linked to intervertebral disk degeneration (IDD) (38). Zhao et al. (38) studied the differential expression of lncRNAs in patients with IDD in comparison to control subjects affected with spinal cord injury using RNA-sequencing combined with quantitative real-time PCR and identified several differentially expressed lncRNAs in IDD patients. Among them, LINC00969 lncRNA was among the top 10 upregulated lncRNA in nucleus pulposus samples of IDD patients. To our knowledge, our study is the first to identify a link between MIR570HG and SCZ, so additional research should be done to verify the reported findings. In line with our result, a previous study showed that LINC00969 positively regulates the expression of the thioredoxin-interacting protein (TXNIP) and increases IDD degeneration by acting as a ceRNA for miR-335-3p and sponging it and modulating activation of NLRP3 inflammasome (39). Moreover, Lee et al. (40) explored possible gene-gene interaction in susceptibility to SCZ by intensively searching for SNP-SNP interactions in three GWAS datasets. They observed that one of these SNP-SNP interactions could be understood as the interaction between FHIT and LINC00969 (40). FHIT and LINC00969 were discovered to have an expression in the brain and could represent a novel discovery in SCZ research (40).
We predicted that the sponging of seven key miRNAs (hsa-miR-124-3p, hsa-miR-17-5p, hsa-miR-181a-5p, hsa-miR-191-5p, hsa-miR-26a-5p, hsa-miR-29a-3p, hsa-miR-29b-3p) by key lncRNAs might influence target genes. By binding to the non-transcript region of the target gene, miRNAs can regulate the expression of the gene. This might have an impact on cellular signaling and biological pathways, which may influence the initiation and course of SCZ (41). Among the key miRNAs, the correlation between hsa-miR-124-3p, hsa-miR-17-5p, hsa-miR-29a-3p, and hsa-miR-29b-3p and SCZ has been studied, already. In accordance with the multi-hit and multi-layered genetic mechanisms implicated in this and other forms of SCZ, Ying et al. (42) proposed a potential role for miR-17-5p and hsa-miR-124-3p perturbation involving genes affected by rare genome-wide copy number variations in the elevated risk for SCZ in 22q11.2 deletion syndrome. In accord with our results, elevated levels of miR-124-3p in SCZ patients compared with healthy controls was reported in a previous investigation (43). In another study, Xu et al. (44) reported upregulation of miR-124-3p in patients with SCZ. They also identified a composite feed-forward loop consisting of EGR1-miR-124-3p-SKIL that may have clinical significance (44). In a previous research, it has been shown that expression of miR-29a-3p decreased in the prefrontal cortex of SCZ patients compared to individuals without psychiatric disorders (45), which is in line with our findings. Finally, it has been indicated that miR-29b-3p is one of the SCZ susceptibility loci (46). Although these findings support our results, additional functional studies must be done to validate our anticipated ceRNA axes.
In this study, we performed KEGG enrichment analysis on DEmRNAs and found that significantly enriched pathways include: “AGE-RAGE signaling pathway in diabetic complications”, “Amoebiasis”, “Transcriptional misregulation in cancer”, “Human T-cell leukemia virus 1 infection”, and “MAPK signaling pathway”. Advanced glycation end products (AGEs), produced through glycation of lipids or proteins with reducing sugars in a non-enzymatic manner, have been associated with different illnesses, like cardiovascular complications in patients with diabetes mellitus (47), chronic renal failure (48), and Alzheimer's disease (49). Plasma pentosidine AGEs were shown to be connected to SCZ in earlier investigations (50–52) and serve as a helpful biomarker for the treatment-resistant-like phenotype (53). Elevated levels of AGEs may result in psychotic symptoms by inducing brain inflammation (54). In fact, AGEs cause elevation of proinflammatory cytokines through binding to a membrane-bound receptor, RAGE, that is present on membrane of astrocytes, microglia, and neurons (55). The blood and cerebrospinal fluid of SCZ patients have elevated levels of pro-inflammatory indicators, such as cytokines (56). A comprehensive epidemiological investigation has conclusively shown that severe infections and autoimmune illnesses are risk factors for SCZ (57). The vulnerability-stress-inflammation paradigm may be useful for elucidating the role of inflammation in SCZ since stress can enhance pro-inflammatory cytokines and even lead to a persistent pro-inflammatory state (56). SCZ is characterized by risk genes that induce inflammation, environmental stress factors, and immune system modifications. Typical abnormalities in dopaminergic, serotonergic, noradrenergic, and glutamatergic neurotransmission observed in SCZ have also been seen in low-level neuroinflammation, and hence may be important contributors to the onset of SCZ symptoms (56). Neuroimaging evidence of volume loss in the central nervous system (CNS) and microglial activation provides additional support for the importance of a low-level neuroinflammatory process in SCZ (56). The early twentieth century-famous infectious theory of psychosis (58) has now gained additional scientific justification (59–62). It has been shown that SCZ is a multifactorial disease that results from the interaction of SCZ susceptibility genes with environmental factors. In addition to lots of SCZ susceptibility genes, viral infections during the prenatal or adult period and toxoplasmosis or Lyme disease also can have a role in this disease (63). The discovery of infectious pathogens that are linked to SCZ etiopathogenesis might result in the development of novel approaches to prophylaxis, diagnosis, and therapy of SCZ (58). The gene expression patterns playing an important role in creating and sustaining particular cell states are exactly regulated by a substantial number of transcription factors, cofactors, and chromatin regulators. Perturbation of these gene expression patterns can contribute to a wide range of illnesses, from cancers to neurological disorders like SCZ (64). According to available data, SCZ may be caused by changes to the way that different neurotransmitter systems signals are integrated. Recently, dysregulation of cyclic adenosine monophosphate (cAMP) and mitogen-activated protein kinase (MAPK) signaling pathways has been observed in SCZ patients in brain dorsolateral prefrontal cortex and anterior cingulate (65).
Primarily we found eight mRNAs in the ceRNA network, including EGR1, ETV1, DUSP6, PLOD2, CD93, SERPINB9, ANGPTL4, and TGFB2. Among these key mRNAs, EGR1, DUSP6, PLOD2, CD93, SERPINB9, and TGFB2 have already been mentioned to be related to the SCZ. The EGR1 gene is from the EGR family of Cys2-His2-type zinc-finger proteins, which encodes the early protein (66). Additionally, it is involved in cell proliferation, female reproduction, immune response, cell growth, neuronal plasticity, and memory formation (67). EGR1 is a nuclear protein functioning in neural development (as a transcriptional regulator) (67). Animal studies revealed that brain function, cognitive aging, and antipsychotic drug administration could affect the EGR1 gene (68, 69). Moreover, downregulation of EGR1 has been reported in several studies in SCZ patients (44, 70–73). For instance, an analysis of reverse transcription-quantitative PCR illustrated a considerable decline in the EGR1 expression level in peripheral blood mononuclear cells and the prefrontal cortex in SCZ patients (compared with controls) (44, 70, 71, 73). Also, compared to the control samples, the downregulation of EGR1 in the anterior cingulate cortex of SCZ samples was observed by Ramaker et al. (72). These findings are similar to our results which declare that the EGR1 gene is related to the pathophysiology of SCZ. Nevertheless, some other expression studies showed up-regulation of the EGR1 gene in fibroblasts, peripheral blood cells (74), and in post-mortem superior temporal cortex of SCZ (75), in which these incompatible observations could be justified by SCZ's heterogeneity, medications, and epigenetic processes (76). The human DUSP6 gene is located at 12q22–q23 (77), overlapping a locus correlated with susceptibility to bipolar disorder. This gene's genetic association with SCZ and bipolar disorder has been represented (78). Furthermore, DUSP6 is referred to as MAP Kinase Phosphatase 3 (MKP3) (a cytoplasmic phosphatase), which is more selective for extracellular signal-regulated kinase1/2 (ERK1/2) compared to other MAPK isoforms (79, 80). Additionally, PLOD2 is recognized as an enzyme for mediating the formation of stabilized collagen cross-links in collagen (through the hydroxylation of lysyl residues) (81). Our results from the up-regulation of PLOD2 are concordant with previous studies (82). A negative regulator in astrogenesis and participating in the regulation of CNS inflammation is CD93, familiar for its immune functions (83, 84). CD93 was widely and ubiquitously expressed in the brain and may be involved in the regulation of innate and adaptive immunity in the CNS. Similarly, we reported results from the bioinformatics analysis, such as up-regulation of CD93 in ceRNA axes in the hippocampus, BA46, and striatum samples obtained from patients with SCZ (14). An intracellular inhibitor of the cytotoxic protease granzyme B (grB) is SERPINB9 which was previously named PI-9 in humans (SPI6 in mice) (85). It is generated in CD8+ T and NK cells (with a nucleo-cytoplasmic distribution), which is vital for these cells' protection against grB-mediated apoptosis (85). With regards to the former investigations, SERPINB9 protein was upregulated in SCZ organoids (86), which meets our results. The TGFBs manage various cells' growth, differentiation, and function and have been involved in several disease processes (87). Notably, according to the reports, TGFB2 seems to be a critical driver in the dysregulation of numerous genes related to SCZ (88). Moreover, aberrant epigenetic regulation of TGFB2 and alteration in TGFβ signaling might contribute to loss or reversal of brain laterality in SCZ cases. A previous study revealed that clozapine exposure is correlated with down-regulation of ANGPTl4 (89). Clozapine, an uncommon antipsychotic treatment, is used for SCZ patients with other antipsychotic drug resistance (90). ANGPTL4 is linked with triglyceride and high-density lipoprotein cholesterol levels (91); hence it could be related to the higher risk of type 2 diabetes in SCZ patients treated with clozapine drug (89, 92). Delplanque et al. reported that ETV1, another key mRNA in the ceRNA network, correlates with spinocerebellar ataxia 21 (another mental disorder) (93). A member of the ETS family (E twenty-six) of transcription factors is encoded by ETV1. This transcription factor can attach to distinct DNA sequences in the promoter/enhancer regions of genes and regulate several biological pathways (94).
It should be noted that a number of technical factors, including various methodologies, patient characteristics, sample preparation, data analysis, and platforms, could have an impact on the gene expression profiles. Furthermore, small sample size may compromise statistical power. On the other hand, potential covariates (e.g., RNA extraction quality, post-mortem interval, and antipsychotic treatment) were not statistically controlled. Finally, our findings must be supported by confirmatory experimental work and comparisons to reanalysis of modified microarray gene expression.
Conclusion
Our study discovered ceRNA axes associated with lncRNAs, which may be important for SCZ. These axes consist of eight key mRNAs (EGR1, ETV1, DUSP6, PLOD2, CD93, SERPINB9, ANGPTL4, TGFB2), two key lncRNAs (PEG3-AS1, MIR570HG), and seven key miRNAs (hsa-miR-124-3p, hsa-miR-17-5p, hsa-miR-181a-5p, hsa-miR-191-5p, hsa-miR-26a-5p, hsa-miR-29a-3p, hsa-miR-29b-3p). Despite the need to understand the functions of these axes, this work provides possible research targets for looking at molecular pathways that might be important for SCZ pathogenesis.
Data availability statement
Publicly available datasets were analyzed in this study. This data can be found here: https://www.ncbi.nlm.nih.gov/geo/query/acc.cgi?acc=GSE17612.
Author contributions
HS, MR, MT, and BMH wrote the draft and revised the manuscript. MT, SMN, and SB designed and supervised the study. MG, MRA, SA, and MS-B collected the data and performed the bioinformatic analysis. All authors read and approved the submitted version.
Acknowledgments
We would like to thank the Clinical Research Development Unit of Tabriz Valiasr Hospital, Tabriz University of Medical Sciences, Tabriz, Iran for their assistance in this research.
Conflict of interest
The authors declare that the research was conducted in the absence of any commercial or financial relationships that could be construed as a potential conflict of interest.
Publisher's note
All claims expressed in this article are solely those of the authors and do not necessarily represent those of their affiliated organizations, or those of the publisher, the editors and the reviewers. Any product that may be evaluated in this article, or claim that may be made by its manufacturer, is not guaranteed or endorsed by the publisher.
Supplementary material
The Supplementary Material for this article can be found online at: https://www.frontiersin.org/articles/10.3389/fpsyt.2022.1010977/full#supplementary-material
References
1. Rodrigues-Amorim D, Rivera-Baltanás T, López M, Spuch C, Olivares JM, Agís-Balboa RC. Schizophrenia: a review of potential biomarkers. J Psychiatr Res. (2017) 93:37–49. doi: 10.1016/j.jpsychires.2017.05.009
2. Jablensky A. Epidemiology of schizophrenia: the global burden of disease and disability. Eur Arch Psychiatry Clin Neurosci. (2000) 250:274–85. doi: 10.1007/s004060070002
3. Birnbaum R, Weinberger DR. Genetic insights into the neurodevelopmental origins of schizophrenia. Nat Rev Neurosci. (2017) 18:727–40. doi: 10.1038/nrn.2017.125
4. van Os S, Rutten B, Myin-Germeys I. European Network of National Networks studying Gene-Environment InteractionsIdentifying gene-environment interactions in schizophrenia: contemporary challenges for integrated, large-scale investigations. Schizophr Bull. (2014) 40:729–36. doi: 10.1093/schbul/sbu069
5. Iwamoto K, Kato T. Gene expression profiling in schizophrenia and related mental disorders. Neuroscientist. (2006) 12:349–61. doi: 10.1177/1073858406287536
6. Gatta E, Saudagar V, Drnevich J, Forrest MP, Auta J, Clark LV, et al. Concordance of immune-related markers in lymphocytes and prefrontal cortex in schizophrenia. Schizophr Bull Open. (2021) 2:sgab002. doi: 10.1093/schizbullopen/sgab002
7. Barnes MR, Huxley-Jones J, Maycox PR, Lennon M, Thornber A, Kelly F, et al. Transcription and pathway analysis of the superior temporal cortex and anterior prefrontal cortex in schizophrenia. J Neurosci Res. (2011) 89:1218–27. doi: 10.1002/jnr.22647
8. Maycox PR, Kelly F, Taylor A, Bates S, Reid J, Logendra R, et al. Analysis of gene expression in two large schizophrenia cohorts identifies multiple changes associated with nerve terminal function. Mol Psychiatry. (2009) 14:1083–94. doi: 10.1038/mp.2009.18
9. Li R, Wang Q, Qiu Y, Meng Y, Wei L, Wang H, et al. A potential autophagy-related competing endogenous RNA network and corresponding diagnostic efficacy in schizophrenia. Front Psychiatry. (2021) 12:628361. doi: 10.3389/fpsyt.2021.628361
10. Safari MR, Komaki A, Arsang-Jang S, Taheri M, Ghafouri-Fard S. Expression pattern of long non-coding RNAs in schizophrenic patients. Cell Mol Neurobiol. (2019) 39:211–21. doi: 10.1007/s10571-018-0640-3
11. Salmena L, Poliseno L, Tay Y, Kats L, Pandolfi PP. A ceRNA hypothesis: the Rosetta Stone of a hidden RNA language? Cell. (2011) 146:353–8. doi: 10.1016/j.cell.2011.07.014
12. Liao F, Zhu L, Yang J, Wu X, Zhao Z, Xu B, et al. Whole transcriptome sequencing identified circRNA profiles and the related networks in schizophrenia. J Mol Neurosci. (2022) 72:1622–35. doi: 10.1007/s12031-022-02013-x
13. Sabaie H, Mazaheri Moghaddam M, Mazaheri Moghaddam M, Amirinejad N, Asadi MR, Daneshmandpour Y, et al. Long non-coding RNA-associated competing endogenous RNA axes in the olfactory epithelium in schizophrenia: a bioinformatics analysis. Sci Rep. (2021) 11:24497. doi: 10.1038/s41598-021-04326-0
14. Sabaie H, Moghaddam MM, Moghaddam MM, Ahangar NK, Asadi MR, Hussen BM, et al. Bioinformatics analysis of long non-coding RNA-associated competing endogenous RNA network in schizophrenia. Sci Rep. (2021) 11:24413. doi: 10.1038/s41598-021-03993-3
15. Yu A-M, Choi YH, Tu M-J. RNA Drugs and RNA targets for small molecules: principles, progress, and challenges. Pharmacol Rev. (2020) 72:862–98. doi: 10.1124/pr.120.019554
16. Anthony K. RNA-based therapeutics for neurological diseases. RNA Biol. (2022) 19:176–90. doi: 10.1080/15476286.2021.2021650
17. Gibbons A, Udawela M, Dean B. Non-coding RNA as novel players in the pathophysiology of schizophrenia. Noncoding RNA. (2018) 4:11. doi: 10.3390/ncrna4020011
18. Moreno-García L, López-Royo T, Calvo AC, Toivonen JM, de la Torre M, Moreno-Martínez L, et al. Competing endogenous RNA networks as biomarkers in neurodegenerative diseases. Int J Mol Sci. (2020) 21:9582. doi: 10.3390/ijms21249582
19. Irizarry RA, Hobbs B, Collin F, Beazer-Barclay YD, Antonellis KJ, Scherf U, et al. Exploration, normalization, and summaries of high density oligonucleotide array probe level data. Biostatistics. (2003) 4:249–64. doi: 10.1093/biostatistics/4.2.249
20. von Heydebreck A, Huber W, Gentleman R. Differential expression with the Bioconductor Project. In: Jorde L, Little P, Dunn M, Subramaniam S, editor. Encyclopedia of Genetics, Genomics, Proteomics and Bioinformatics. Berkeley, CA: The Berkeley Electronic Press (bepress) (2005). doi: 10.1002/047001153X.g405208
21. Ritchie ME, Phipson B, Wu D, Hu Y, Law CW, Shi W, et al. limma powers differential expression analyses for RNA-sequencing and microarray studies. Nucleic Acids Res. (2015) 43:e47. doi: 10.1093/nar/gkv007
22. Huber W, Carey VJ, Gentleman R, Anders S, Carlson M, Carvalho BS, et al. Orchestrating high-throughput genomic analysis with Bioconductor. Nat Methods. (2015) 12:115–21. doi: 10.1038/nmeth.3252
23. Tweedie S, Braschi B, Gray K, Jones TEM, Seal RL, Yates B, et al. Genenames.org: the HGNC and VGNC resources in 2021. Nucleic Acids Res. (2021) 49:D939–46. doi: 10.1093/nar/gkaa980
24. Karagkouni D, Paraskevopoulou MD, Tastsoglou S, Skoufos G, Karavangeli A, Pierros V, et al. DIANA-LncBase v3: indexing experimentally supported miRNA targets on non-coding transcripts. Nucleic Acids Res. (2020) 48:D101–10. doi: 10.1093/nar/gkz1036
25. Huang HY, Lin YC, Li J, Huang KY, Shrestha S, Hong HC, et al. miRTarBase 2020: updates to the experimentally validated microRNA-target interaction database. Nucleic Acids Res. (2020) 48:D148–54. doi: 10.1093/nar/gkz896
26. Szklarczyk D, Gable AL, Lyon D, Junge A, Wyder S, Huerta-Cepas J, et al. STRING v11: protein-protein association networks with increased coverage, supporting functional discovery in genome-wide experimental datasets. Nucleic Acids Res. (2019) 47:D607–13. doi: 10.1093/nar/gky1131
27. Shannon P, Markiel A, Ozier O, Baliga NS, Wang JT, Ramage D, et al. Cytoscape: a software environment for integrated models of biomolecular interaction networks. Genome Res. (2003) 13:2498–504. doi: 10.1101/gr.1239303
28. Chen EY, Tan CM, Kou Y, Duan Q, Wang Z, Meirelles GV, et al. Enrichr: interactive and collaborative HTML5 gene list enrichment analysis tool. BMC Bioinformatics. (2013) 14:128. doi: 10.1186/1471-2105-14-128
29. Kuleshov MV, Jones MR, Rouillard AD, Fernandez NF, Duan Q, Wang Z, et al. Enrichr: a comprehensive gene set enrichment analysis web server 2016 update. Nucleic Acids Res. (2016) 44:W90–7. doi: 10.1093/nar/gkw377
30. MacDonald AW 3rd, Carter CS, Kerns JG, Ursu S, Barch DM, Holmes AJ, et al. Specificity of prefrontal dysfunction and context processing deficits to schizophrenia in never-medicated patients with first-episode psychosis. Am J Psychiatry. (2005) 162:475–84. doi: 10.1176/appi.ajp.162.3.475
31. Yoon JH, Minzenberg MJ, Ursu S, Ryan Walter BS, Wendelken C, Ragland JD, et al. Association of dorsolateral prefrontal cortex dysfunction with disrupted coordinated brain activity in schizophrenia: relationship with impaired cognition, behavioral disorganization, and global function. Am J Psychiatry. (2008) 165:1006–14. doi: 10.1176/appi.ajp.2008.07060945
32. Ala U. Competing Endogenous RNAs, non-coding RNAs and diseases: an intertwined story. Cells. (2020) 9:1574. doi: 10.3390/cells9071574
33. Cai Y, Wan J. Competing endogenous RNA regulations in neurodegenerative disorders: current challenges and emerging insights. Front Mol Neurosci. (2018) 11:370. doi: 10.3389/fnmol.2018.00370
34. Gloss BS, Dinger ME. The specificity of long noncoding RNA expression. Biochim Biophys Acta. (2016) 1859:16–22. doi: 10.1016/j.bbagrm.2015.08.005
35. Yang MY, Lin PM, Yang CH, Hu ML, Chen IY, Lin SF, et al. Loss of ZNF215 imprinting is associated with poor five-year survival in patients with cytogenetically abnormal-acute myeloid leukemia. Blood Cells Mol Dis. (2021) 90:102577. doi: 10.1016/j.bcmd.2021.102577
36. Zhang L, Chen S, Wang B, Su Y, Li S, Liu G, et al. An eight-long noncoding RNA expression signature for colorectal cancer patients' prognosis. J Cell Biochem. (2019) 120:5636–43. doi: 10.1002/jcb.27847
37. Hsu CM, Lin PM, Lin HC, Lai CC, Yang CH, Lin SF, et al. Altered expression of imprinted genes in squamous cell carcinoma of the head and neck. Anticancer Res. (2016) 36:2251–8. Available online at: https://ar.iiarjournals.org/content/36/5/2251
38. Zhao B, Lu M, Wang D, Li H, He X. Genome-wide identification of long noncoding RNAs in human intervertebral disc degeneration by RNA sequencing. Biomed Res Int. (2016) 2016:3684875. doi: 10.1155/2016/3684875
39. Yu L, Hao Y, Xu C, Zhu G, Cai Y. LINC00969 promotes the degeneration of intervertebral disk by sponging miR-335-3p and regulating NLRP3 inflammasome activation. IUBMB Life. (2019) 71:611–8. doi: 10.1002/iub.1989
40. Lee KY, Leung KS, Ma SL, So HC, Huang D, Tang NL, et al. Genome-wide search for SNP interactions in GWAS data: algorithm, feasibility, replication using schizophrenia datasets. Front Genet. (2020) 11:1003. doi: 10.3389/fgene.2020.01003
41. Caputo V, Ciolfi A, Macri S, Pizzuti A. The emerging role of MicroRNA in schizophrenia. CNS Neurol Disord Drug Targets. (2015) 14:208–21. doi: 10.2174/1871527314666150116124253
42. Ying S, Heung T, Zhang Z, Yuen RKC, Bassett AS. Schizophrenia risk mediated by microRNA target genes overlapped by genome-wide rare copy number variation in 22q11.2 deletion syndrome. Front Genet. (2022) 13:812183. doi: 10.3389/fgene.2022.812183
43. Fu X, Liu Y, Baranova A, Zhang F. Deregulatory miRNA-BDNF network inferred from dynamic expression changes in schizophrenia. Brain Sci. (2022) 12:167. doi: 10.3390/brainsci12020167
44. Xu Y, Yue W, Yao Shugart Y, Li S, Cai L, Li Q, et al. Exploring transcription factors-microRNAs Co-regulation networks in schizophrenia. Schizophr Bull. (2016) 42:1037–45. doi: 10.1093/schbul/sbv170
45. Perkins DO, Jeffries CD, Jarskog LF, Thomson JM, Woods K, Newman MA, et al. microRNA expression in the prefrontal cortex of individuals with schizophrenia and schizoaffective disorder. Genome Biol. (2007) 8:R27. doi: 10.1186/gb-2007-8-2-r27
46. Hauberg ME, Roussos P, Grove J, Børglum AD, Mattheisen M. Analyzing the role of microRNAs in schizophrenia in the context of common genetic risk variants. JAMA Psychiatry. (2016) 73:369–77. doi: 10.1001/jamapsychiatry.2015.3018
47. Hanssen NM, Beulens JW, van Dieren S, Scheijen JL, van der AD, Spijkerman AM, et al. Plasma advanced glycation end products are associated with incident cardiovascular events in individuals with type 2 diabetes: a case-cohort study with a median follow-up of 10 years (EPIC-NL). Diabetes. (2015) 64:257–65. doi: 10.2337/db13-1864
48. Miyata T, van Ypersele de Strihou C, Kurokawa K, Baynes JW. Alterations in nonenzymatic biochemistry in uremia: origin and significance of “carbonyl stress” in long-term uremic complications. Kidney Int. (1999) 55:389–99. doi: 10.1046/j.1523-1755.1999.00302.x
49. Meli M, Perier C, Ferron C, Parssegny F, Denis C, Gonthier R, et al. Serum pentosidine as an indicator of Alzheimer's disease. J Alzheimers Dis. (2002) 4:93–6. doi: 10.3233/JAD-2002-4203
50. Arai M, Yuzawa H, Nohara I, Ohnishi T, Obata N, Iwayama Y, et al. Enhanced carbonyl stress in a subpopulation of schizophrenia. Arch Gen Psychiatry. (2010) 67:589–97. doi: 10.1001/archgenpsychiatry.2010.62
51. Miyashita M, Arai M, Yuzawa H, Niizato K, Oshima K, Kushima I, et al. Replication of enhanced carbonyl stress in a subpopulation of schizophrenia. Psychiatry Clin Neurosci. (2014) 68:83–4. doi: 10.1111/pcn.12081
52. Kobori A, Miyashita M, Miyano Y, Suzuki K, Toriumi K, Niizato K, et al. Advanced glycation end products and cognitive impairment in schizophrenia. PLoS ONE. (2021) 16:e0251283. doi: 10.1371/journal.pone.0251283
53. Miyashita M, Arai M, Kobori A, Ichikawa T, Toriumi K, Niizato K, et al. Clinical features of schizophrenia with enhanced carbonyl stress. Schizophr Bull. (2014) 40:1040–6. doi: 10.1093/schbul/sbt129
54. Miyashita M, Yamasaki S, Ando S, Suzuki K, Toriumi K, Horiuchi Y, et al. Fingertip advanced glycation end products and psychotic symptoms among adolescents. NPJ Schizophr. (2021) 7:37. doi: 10.1038/s41537-021-00167-y
55. Hofmann MA, Drury S, Fu C, Qu W, Taguchi A, Lu Y, et al. RAGE mediates a novel proinflammatory axis: a central cell surface receptor for S100/calgranulin polypeptides. Cell. (1999) 97:889–901. doi: 10.1016/S0092-8674(00)80801-6
56. Müller N. Inflammation in schizophrenia: pathogenetic aspects and therapeutic considerations. Schizophr Bull. (2018) 44:973–82. doi: 10.1093/schbul/sby024
57. Müller N, Weidinger E, Leitner B, Schwarz MJ. The role of inflammation in schizophrenia. Front Neurosci. (2015) 9:372. doi: 10.3389/fnins.2015.00372
58. Yolken RH, Torrey EF. Are some cases of psychosis caused by microbial agents? A review of the evidence. Mol Psychiatry. (2008) 13:470–9. doi: 10.1038/mp.2008.5
59. Zheng H, Savitz J. Effect of cytomegalovirus infection on the central nervous system: implications for psychiatric disorders. Curr Top Behav Neurosci. (2022). doi: 10.1007/7854_2022_361. [Epub ahead of print].
60. Webster MJ. Infections, inflammation, and psychiatric illness: review of postmortem evidence. Curr Top Behav Neurosci. (2022). doi: 10.1007/7854_2022_362. [Epub ahead of print].
61. Lampard-Scotford AR, McCauley A, Kuebel JA, Ibbott R, Mutapi F. Impact of parasitic infection on mental health and illness in humans in Africa: a systematic review. Parasitology. (2022) 149:1003–18. doi: 10.1017/S0031182022000166
62. Massarali A, Adhya D, Srivastava DP, Baron-Cohen S, Kotter MR. Virus-induced maternal immune activation as an environmental factor in the etiology of autism and schizophrenia. Front Neurosci. (2022) 16:834058. doi: 10.3389/fnins.2022.834058
63. Carter CJ. Schizophrenia: a pathogenetic autoimmune disease caused by viruses and pathogens and dependent on genes. J Pathog. (2011) 2011:128318. doi: 10.4061/2011/128318
64. Lee TI, Young RA. Transcriptional regulation and its misregulation in disease. Cell. (2013) 152:1237–51. doi: 10.1016/j.cell.2013.02.014
65. Funk AJ, McCullumsmith RE, Haroutunian V, Meador-Woodruff JH. Abnormal activity of the MAPK- and cAMP-associated signaling pathways in frontal cortical areas in postmortem brain in schizophrenia. Neuropsychopharmacology. (2012) 37:896–905. doi: 10.1038/npp.2011.267
66. Sukhatme VP. The Egr family of nuclear signal transducers. Am J Kidney Dis. (1991) 17:615–8. doi: 10.1016/S0272-6386(12)80333-7
67. Hu TM, Chen SJ, Hsu SH, Cheng MC. Functional analyses and effect of DNA methylation on the EGR1 gene in patients with schizophrenia. Psychiatry Res. (2019) 275:276–82. doi: 10.1016/j.psychres.2019.03.044
68. Cheng MC, Liao DL, Hsiung CA, Chen CY, Liao YC, Chen CH. Chronic treatment with aripiprazole induces differential gene expression in the rat frontal cortex. Int J Neuropsychopharmacol. (2008) 11:207–16. doi: 10.1017/S1461145707008048
69. Penner MR, Parrish RR, Hoang LT, Roth TL, Lubin FD, Barnes CA. Age-related changes in Egr1 transcription and DNA methylation within the hippocampus. Hippocampus. (2016) 26:1008–20. doi: 10.1002/hipo.22583
70. Liu S, Zhang F, Shugart YY, Yang L, Li X, Liu Z, et al. The early growth response protein 1-miR-30a-5p-neurogenic differentiation factor 1 axis as a novel biomarker for schizophrenia diagnosis and treatment monitoring. Transl Psychiatry. (2017) 7:e998. doi: 10.1038/tp.2016.268
71. Pérez-Santiago J, Diez-Alarcia R, Callado LF, Zhang JX, Chana G, White CH, et al. A combined analysis of microarray gene expression studies of the human prefrontal cortex identifies genes implicated in schizophrenia. J Psychiatr Res. (2012) 46:1464–74. doi: 10.1016/j.jpsychires.2012.08.005
72. Ramaker RC, Bowling KM, Lasseigne BN, Hagenauer MH, Hardigan AA, Davis NS, et al. Post-mortem molecular profiling of three psychiatric disorders. Genome Med. (2017) 9:72. doi: 10.1186/s13073-017-0458-5
73. Yamada K, Gerber DJ, Iwayama Y, Ohnishi T, Ohba H, Toyota T, et al. Genetic analysis of the calcineurin pathway identifies members of the EGR gene family, specifically EGR3, as potential susceptibility candidates in schizophrenia. Proc Natl Acad Sci USA. (2007) 104:2815–20. doi: 10.1073/pnas.0610765104
74. Cattane N, Minelli A, Milanesi E, Maj C, Bignotti S, Bortolomasi M, et al. Altered gene expression in schizophrenia: findings from transcriptional signatures in fibroblasts and blood. PLoS ONE. (2015) 10:e0116686. doi: 10.1371/journal.pone.0116686
75. Iwakura Y, Kawahara-Miki R, Kida S, Sotoyama H, Gabdulkhaev R, Takahashi H, et al. Elevation of EGR1/zif268, a neural activity marker, in the auditory cortex of patients with schizophrenia and its animal model. Neurochem Res. (2022) 47:2715–27. doi: 10.1007/s11064-022-03599-9
76. Thomas EA. Histone posttranslational modifications in schizophrenia. In: Delgado-Morales R, editor. Neuroepigenomics in Aging and Disease. Cham: Springer International Publishing (2017). p. 237–54. doi: 10.1007/978-3-319-53889-1_13
77. Smith A, Price C, Cullen M, Muda M, King A, Ozanne B, et al. Chromosomal localization of three human dual specificity phosphatase genes (DUSP4, DUSP6, and DUSP7). Genomics. (1997) 42:524–7. doi: 10.1006/geno.1997.4756
78. Kim SH, Shin SY, Lee KY, Joo EJ, Song JY, Ahn YM, et al. The genetic association of DUSP6 with bipolar disorder and its effect on ERK activity. Prog Neuropsychopharmacol Biol Psychiatry. (2012) 37:41–9. doi: 10.1016/j.pnpbp.2011.11.014
79. Groom LA, Sneddon AA, Alessi DR, Dowd S, Keyse SM. Differential regulation of the MAP, SAP and RK/p38 kinases by Pyst1, a novel cytosolic dual-specificity phosphatase. EMBO J. (1996) 15:3621–32. doi: 10.1002/j.1460-2075.1996.tb00731.x
80. Muda M, Theodosiou A, Rodrigues N, Boschert U, Camps M, Gillieron C, et al. The dual specificity phosphatases M3/6 and MKP-3 are highly selective for inactivation of distinct mitogen-activated protein kinases. J Biol Chem. (1996) 271:27205–8. doi: 10.1074/jbc.271.44.27205
81. Yamauchi M, Sricholpech M. Lysine post-translational modifications of collagen. Essays Biochem. (2012) 52:113–33. doi: 10.1042/bse0520113
82. Mistry M, Gillis J, Pavlidis P. Genome-wide expression profiling of schizophrenia using a large combined cohort. Mol Psychiatry. (2013) 18:215–25. doi: 10.1038/mp.2011.172
83. Griffiths MR, Botto M, Morgan BP, Neal JW, Gasque P. CD93 regulates central nervous system inflammation in two mouse models of autoimmune encephalomyelitis. Immunology. (2018) 155:346–55. doi: 10.1111/imm.12974
84. Liang Q, Su L, Zhang D, Jiao J. CD93 negatively regulates astrogenesis in response to MMRN2 through the transcriptional repressor ZFP503 in the developing brain. Proc Natl Acad Sci USA. (2020) 117:9413–22. doi: 10.1073/pnas.1922713117
85. Mangan MS, Vega-Ramos J, Joeckel LT, Mitchell AJ, Rizzitelli A, Roediger B, et al. Serpinb9 is a marker of antigen cross-presenting dendritic cells. Mol Immunol. (2017) 82:50–6. doi: 10.1016/j.molimm.2016.12.011
86. Notaras M, Lodhi A, Fang H, Greening D, Colak D. The proteomic architecture of schizophrenia iPSC-derived cerebral organoids reveals alterations in GWAS and neuronal development factors. Transl Psychiatry. (2021) 11:541. doi: 10.1038/s41398-021-01664-5
87. Goris A, Williams-Gray CH, Foltynie T, Brown J, Maranian M, Walton A, et al. Investigation of TGFB2 as a candidate gene in multiple sclerosis and Parkinson's disease. J Neurol. (2007) 254:846–8. doi: 10.1007/s00415-006-0414-6
88. Abdolmaleky HM, Gower AC, Wong CK, Cox JW, Zhang X, Thiagalingam A, et al. Aberrant transcriptomes and DNA methylomes define pathways that drive pathogenesis and loss of brain laterality/asymmetry in schizophrenia and bipolar disorder. Am J Med Genet B Neuropsychiatr Genet. (2019) 180:138–49. doi: 10.1002/ajmg.b.32691
89. Viana J, Wildman N, Hannon E, Farbos A, Neill PO, Moore K, et al. Clozapine-induced transcriptional changes in the zebrafish brain. NPJ Schizophr. (2020) 6:3. doi: 10.1038/s41537-019-0092-x
90. Siskind D, McCartney L, Goldschlager R, Kisely S. Clozapine v. first- and second-generation antipsychotics in treatment-refractory schizophrenia: systematic review and meta-analysis. Br J Psychiatry. (2016) 209:385–92. doi: 10.1192/bjp.bp.115.177261
91. Romeo S, Pennacchio LA, Fu Y, Boerwinkle E, Tybjaerg-Hansen A, Hobbs HH, et al. Population-based resequencing of ANGPTL4 uncovers variations that reduce triglycerides and increase HDL. Nat Genet. (2007) 39:513–6. doi: 10.1038/ng1984
92. Wang PS, Glynn RJ, Ganz DA, Schneeweiss S, Levin R, Avorn J. Clozapine use and risk of diabetes mellitus. J Clin Psychopharmacol. (2002) 22:236–43. doi: 10.1097/00004714-200206000-00002
93. Delplanque J, Devos D, Vuillaume I, De Becdelievre A, Vangelder E, Maurage CA, et al. Slowly progressive spinocerebellar ataxia with extrapyramidal signs and mild cognitive impairment (SCA21). Cerebellum. (2008) 7:179–83. doi: 10.1007/s12311-008-0014-3
Keywords: bioinformatics analysis, competing endogenous RNA, long non-coding RNA, microarray, schizophrenia
Citation: Sabaie H, Gholipour M, Asadi MR, Abed S, Sharifi-Bonab M, Taheri M, Hussen BM, Brand S, Neishabouri SM and Rezazadeh M (2022) Identification of key long non-coding RNA-associated competing endogenous RNA axes in Brodmann Area 10 brain region of schizophrenia patients. Front. Psychiatry 13:1010977. doi: 10.3389/fpsyt.2022.1010977
Received: 03 August 2022; Accepted: 14 October 2022;
Published: 03 November 2022.
Edited by:
Adam K. Walker, Neuroscience Research Australia, AustraliaReviewed by:
Amin Safa, Complutense University of Madrid, SpainHayley North, Neuroscience Research Australia, Australia
Copyright © 2022 Sabaie, Gholipour, Asadi, Abed, Sharifi-Bonab, Taheri, Hussen, Brand, Neishabouri and Rezazadeh. This is an open-access article distributed under the terms of the Creative Commons Attribution License (CC BY). The use, distribution or reproduction in other forums is permitted, provided the original author(s) and the copyright owner(s) are credited and that the original publication in this journal is cited, in accordance with accepted academic practice. No use, distribution or reproduction is permitted which does not comply with these terms.
*Correspondence: Seyedeh Morvarid Neishabouri, dr.s.m.neishabouri@gmail.com; Maryam Rezazadeh, rezazadehm@tbzmed.ac.ir
†These authors have contributed equally to this work