- 1Department of Clinical Psychology and Neuropsychology, Institute of Psychology, Johannes Gutenberg-University Mainz, Mainz, Germany
- 2Department of Psychology, University of Zurich, Zurich, Switzerland
- 3Department of Psychiatry, Psychotherapy and Psychosomatics, Psychiatric University Hospital Zurich, Zurich, Switzerland
- 4Leibniz Institute for Resilience Research, Research Group Wessa, Mainz, Germany
The COVID-19 pandemic has prompted severe restrictions on everyday life to curb the spread of infections. For example, teaching at universities has been switched to an online format, reducing students' opportunities for exchange, and social interaction. Consequently, their self-reported mental health has significantly decreased and there is a pressing need to elucidate the underlying mechanisms—ideally considering not only data collected during the pandemic, but also before. One hundred seventeen German university students aged 18-27 were assessed for known resilience factors (optimism, self-care, social support, generalized self-efficacy) and subsequently completed surveys on stress experiences and mental health every 3 months over a period of 9 months before the outbreak of the pandemic and once during the first lockdown in Germany. For each timepoint before the pandemic, we regressed participants' mental health against the reported stressor load, such that the resulting residuals denote better or worse than expected outcomes, i.e., the degree of resilient functioning. We then tested whether different expressions in the resilience factors were predictive of distinct resilient functioning trajectories, which were identified through latent class growth analysis. Finally, we investigated whether trajectory class, resilience factors, and perceived stress predicted resilience during the pandemic. Results show rather stable resilient functioning trajectories, with classes differing mainly according to degree rather than change over time. More self-care was associated with a higher resilient functioning trajectory, which in turn was linked with the most favorable pandemic response (i.e., lower perceived stress and more self-care). Although findings should be interpreted with caution given the rather small sample size, they represent a rare examination of established resilience factors in relation to resilience over an extended period and highlight the relevance of self-care in coping with real-life stressors such as the pandemic.
Introduction
On March 11, 2020, in response to the rapidly increasing number of cases and growing list of affected countries, the World Health Organization (WHO) declared the coronavirus disease 2019 (COVID-19) a global pandemic (1). Governments worldwide began imposing restrictions on everyday social life to curb the spread of infections. Germany entered its first lockdown in mid-March 2020, closing all non-essential stores, cultural and sports facilities, restaurants, bars, kindergartens, schools, universities, and banning public meetings of more than two people (2, 3). For most, these measures meant an abrupt and serious change in their habits and lifestyle. Although restrictions were gradually lifted in the beginning of May 2020 (4), many measures remained in place or were reintroduced over the course of at least 1.5 years as the country navigated further waves of the pandemic (5). Because of its pervasive impact, the pandemic has been described as a complex, multidimensional stressor that disrupts individuals' daily lives as well as social systems in general, prevents access to protective factors, and has no foreseeable end (6). In line with this, many studies have shown increases in mental health problems and worsening of pre-existing conditions (7, 8). Vulnerable populations include university students whose elevated and rising prevalence rates of depression and anxiety have previously been recognized as a growing problem (9–11). Indeed, evidence from cross-sectional studies investigating students during the pandemic in, e.g., China (12), Spain (13), Germany, and Egypt (14), as well as Italy (15) showed alarming rates of mental health problems, psychological distress, depressive symptoms, and significantly higher levels of psychopathology compared to general workers, respectively. Matos Fialho et al. (16) surveyed over 5,000 German students and reported a perceived increase in workload which was associated with significant stress and worry. However, results from longitudinal studies that include assessments prior to the pandemic (and therefore can investigate changes within the same individuals) are less clear. While some report a pandemic-related rise in mental health problems among students [e.g., (17, 18)], others did not observe a meaningful increase (19, 20). Previous research on mental health symptom trajectories following adversity has shown that resilience, or the maintenance of mental health, is, in fact, the most common response (21). Researchers in the field of resilience have advocated for more investigations of protective features and predictors of good mental health in the face of significant pandemic-related stress (22, 23). Factors that have been established as resilience-promoting include optimism (24), social support (25, 26), perceived self-efficacy (27, 28), and self-care (29, 30).
So far, studies examining the impact of the COVID-19 pandemic on students' mental health focused primarily on identifying risk factors, and these efforts are often hampered by the lack of assessments prior to the outbreak of the pandemic. Here, we analyzed longitudinal data collected both during and before the first pandemic-related lockdown in Germany. Specifically, we aimed at predicting students' resilient functioning during the pandemic as a function of previous resilience trajectories, aforementioned resilience-promoting factors, and perceived stress. In addition, we investigated trajectory class-dependent differences in resilience factors at baseline. We expected distinct differences in students' resilient functioning trajectories over the multiple pre-pandemic assessments (e.g., decreasing, increasing, or stable trajectories). Based on the literature, we hypothesized that differences in optimism, social support, perceived self-efficacy, and self-care would distinguish putative resilience trajectory types. With respect to the pandemic, we assumed that it was associated with increased stress and poorer mental health among students. We expected that a more favorable resilient functioning trajectory (i.e., consistently high or increasing levels), higher expression in the resilience factors, and lower perceived stress would be predictive of better resilience during the lockdown.
Materials and Methods
Participants
We used data from a large-scale longitudinal intervention study in which university students were assigned to either a resilience training or a wait-list control group. Here, we considered only the 316 control participants who did not undergo any training. These participants came from two different cohorts and were matched according to data collection time points (see section Procedure for details). Since we operationalized resilient functioning as the residual resulting from the regression of mental health on experienced stress (see section Data Preparation and Statistical Analyses for details), we only included participants who provided complete data for predictor and criterion at all time points. Thus, the residuals always represent deviations from the expected relationship based on the same population. In addition, three participants had to be excluded from the analysis: one had duplicate data from both cohorts, one had not reported any stressful events at baseline, and one reported an extremely high frequency of microstressors at one time point (> 5 SD from sample mean). The final sample comprised 133 students aged 18-27 (75% female, age: M = 20.56, SD = 1.76; 67% belonging to the later cohort), all of whom were fluent in German, had not received psychotherapeutic or psychiatric treatment within the last 5 years, reported no regular alcohol or drug use, no self-harming behavior or suicidal ideation within the last 6 months, and had not experienced a major traumatic event. Nearly all these participants completed a follow-up online survey, yielding a sample of 117 students (74% female, age: M = 21.69, SD = 1.76; 66% belonging to the later cohort) for analyses focusing on resilient functioning during the pandemic. Only one participant reported having tested positive for COVID-19 and experiencing symptoms including fever which were treated at home. 6% stated they belonged to a risk group for a severe course of the disease and 5% had been quarantining at home. This study was approved by the ethics committee of the Institute of Psychology, Johannes Gutenberg-University, Mainz, Germany (2018-JGU-psychEK-001, 27/03/2018), and was conducted in accordance with the Declaration of Helsinki.
Procedure
Participants took part in an initial 1.5 h on-site assessment (T0) in the Mainz Behavioral and Experimental Laboratory (MABELLA) at Johannes Gutenberg-University Mainz. Upon arrival, they received information on the study and planned procedures and gave written informed consent. Participants then provided self-report data on demographics and established resilience factors; namely, optimism, self-care, social support, and generalized self-efficacy. They also completed questionnaires measuring stressor load, mental health, and well-being. Following this baseline assessment, participants were asked to fill out the latter questionnaires online every 3 months over a 9-month period before the outbreak of the pandemic (T1-T3). During the first pandemic-related lockdown in Germany, we conducted another online follow-up (T4; 27 April to 13 May 2020) in which participants were again asked to report on their stress experiences and mental health, but this time against the specific backdrop of the ongoing pandemic. In addition, we re-assessed the aforementioned resilience factors. Upon completion of each session, participants were remunerated with 15 €. Note that, in order to match the data of the two cohorts by time of assessment and to ensure an equal number of assessments before the pandemic, we had to disregard the first two time points of the first cohort. We accounted for possible effects of previous sessions in this cohort by including cohort as a covariate in our analyses. Figure 1 illustrates the timing of data collection for both cohorts separately and relates it to pandemic events. The following section provides details on the questionnaires we used for this study.
Questionnaires
First, we describe the questionnaires used for the resilience factors listed before at T0. Optimism was measured using the corresponding three-item subscale of the German version of the revised Life Orientation Test [LOT-R; (31); original English version by (32)], which has an acceptable internal consistency (Cronbach's α = 0.69). Self-care was assessed using the mean across all 12 items of the Hamburg Self-Care Questionnaire [HamSCQ; (33)], subsuming the subscales pacing (i.e., mindful handling of oneself and one's limits) and positive experience (i.e., accepting and enjoying positive behaviors; Cronbach's α > 0.9 for both scales). The 14-item short form of the Social Support Questionnaire [F-SozU-K14; (34); Cronbach's α = 0.94] was included as a measure of social support, and the German version of the Generalized Self-Efficacy Scale [GSE; (35); 10 items; Cronbach's α > 0.7] provided an indicator of generalized self-efficacy.
Second, we list the stress and mental health questionnaires that participants completed at T0-T4. The German version of the Brief Symptom Inventory-18 [BSI-18; (36); original English version by (37)] uses six items each to capture psychological distress in the past week via the subscales somatization, depression, and anxiety. However, here we used the Global Severity Index (GSI) which covers all items and has excellent internal consistency [Cronbach's α = 0.93; (38)]. The German version of the WHO Well-Being Index [WHO-5; (39); original English publication by (40)] was used as another indicator of mental health, comprising five items that refer to the past 2 weeks (Cronbach's α = 0.92). Participants also completed the Mainz Inventory of Microstressors [MIMIS; (41)] which measures the frequency and intensity of 58 microstressors (e.g., commute to work, problem with a pet, time pressure) within the past week. A 27-item life events checklist [(42); adapted from (43)] provided a count of more severe stressors (e.g., death of a friend, law violations, serious financial problems) encountered in the past 3 months. Since the checklist also includes items that may not be perceived as stressful by all participants (e.g., marriage plans, child starting school), we only counted life events if they were rated as at least a bit burdensome (i.e., 1 on a scale ranging from 0 = not at all burdensome to 4 = very burdensome). At T0, participants were instructed to rate all events they had experienced up to that date.
Third, we elaborate on additional questionnaires assessed at T4 (i.e., during the lockdown). These included items on COVID-19 risk group status, infection, symptom severity, and quarantine, as well as a 29-item list of stressors specific to the context of the pandemic (44). For stressors that had occurred to them, participants provided intensity ratings on a scale from 1 (not at all burdensome) to 5 (extremely burdensome) and we calculated stressor count as well as mean scores reflecting stressor burden. We also assessed participants' agreement with government-mandated restrictions and the degree to which they were following official recommendations. For both items, we used a Likert scale ranging from 1 (not at all) to 5 (very). In addition, we measured perceived stress using the German version of the Perceived Stress Scale [PSS; (45); original English version by (46)]. The PSS can be split into the subscales helplessness and self-efficacy, comprising six and four items, respectively. However, here we used the total score across all items as an indicator of general subjective stress level in the past seven days (Cronbach's α = 0.88). Whereas, optimism, self-care, and generalized self-efficacy were re-assessed using the same instruments as at T0, we used the 4-item subscale perceived emotional support of the Berlin Social Support Scales [BSSS; (47); Cronbach's α = 0.81] to assess social support during the pandemic. The BSSS items were presented in the past tense and participants were instructed to refer to the past 4 weeks.
Data Preparation and Statistical Analyses
We calculated a measure of resilient functioning for each time point T0-T4 based on the following variables: the GSI of the BSI-18, the WHO-5, the frequency of microstressor encounters, and the count of stressful life events. In computing the score, we followed established procedures described in previous publications [e.g., (48–51)]. To obtain a single indicator of both mental health and stress, we first conducted a principal component analysis of the standardized GSI and WHO-5 scores and the standardized microstressor and life events scores, respectively. To match the WHO-5 response format, we used the inverted score of the GSI such that higher values indicated fewer symptoms i.e., a more positive outcome. The extracted first component taken to reflect mental health was then regressed on the first component representing stressor load. The resulting residuals therefore denoted better or worse than expected mental health based on the given stress experience. Hence, we obtained a continuous measure of resilient functioning.
For our investigation of distinct classes of resilient functioning trajectories, we followed instructions by Wickrama et al. (52). Prior to conducting a latent class growth analysis (LCGA) to identify trajectory classes, we determined its appropriateness through univariate growth curve modeling. We fitted an unconditional single growth curve (linear and quadratic) to the resilient functioning scores from T0-T3 and verified adequate model fit (53) based on the root mean square error of approximation (RMSEA ≤ 0.05), the Comparative Fit Index (CFI ≥ 0.95), the Tucker-Lewis Index (TLI ≥ 0.95), and the standardized root mean square residual (SRMR ≤ 0.07). Next, we compared LCGA results for unconditional models with one to five classes, fixing all within-class variances to zero. Unlike LCGA, growth mixture modeling (GMM) does not assume homogeneous growth curves within classes and freely estimates within-class variances. It is therefore generally preferred, but our attempts at such a model failed to converge, perhaps reflecting sample size constraints. We decided to use LCGA to ensure model convergence. We specified 500 random sets of starting values and 10 final optimizations to avoid local maxima (54). The optimal number of trajectory classes was determined by comparing standard fit indices listed below, examining latent class membership probabilities, and considering theoretical interpretability. Lower values of the Akaike information criterion (AIC), the Bayesian information criterion (BIC), and the sample size adjusted BIC (SSABIC) suggest better model fit. Entropy values approaching 1 indicate high classification accuracy, and a significant adjusted Lo-Mendell-Rubin likelihood ratio test (adj. LMR-LRT) and bootstrapped LRT (BLRT) show that adding a class significantly improves model fit (55).
To investigate the different resilience factors assessed at T0 as predictors of latent trajectory class, we performed a multinomial logistic regression analysis. First, we checked for extreme values [above the third quartile plus three times the interquartile range (IQR) or below the first quartile minus three times the IQR; (56)] and for multicollinearity (i.e., correlation coefficients of r > 0.70) among predictors. Then we set up our model including gender, age, and cohort as predictors alongside the resilience factors.
We analyzed the impact of the pandemic by conducting paired samples t-tests to compare participants' stressor load and mental health before the lockdown (T3) with assessments during the lockdown (T4).
Finally, we used multiple regression to examine the predictive value of trajectory class, resilience factors (re-assessed during the pandemic), and perceived stress on participants' resilient functioning during the lockdown. Class-dependent differences in resilient functioning during the pandemic were further investigated by comparing all groups. We applied Holm-Bonferroni correction for multiple comparisons.
Analyses were mainly performed in R, version 4.0.5 (https://www.r-project.org), latent trajectory classes, however, were identified using Mplus, version 7.3 (57).
Results
Resilient Functioning Scores
The principal component analysis conducted for each of the five time points (T0-T4) resulted in components for mental health and stressor load, each of which explained above 60% of variance. As intended, higher values in mental health components indicated better mental health and higher values in stressor load components reflected higher stressor load. Results of the linear regressions performed for each time point showed that stressor load was a significant predictor of mental health (see Supplementary Table 1 for detailed results). Participants reported lower mental health with increasing stressor load. The resulting residuals were taken to reflect participants' level of resilient functioning (see Supplementary Figure 1 for visualization).
Growth Curve Modeling
A linear growth curve model of the resilient functioning scores from T0-T3 showed excellent fit to the data (CFI = 1.000; TLI = 1.000; RMSEA = 0.000; SRMS = 0.021). Although the mean slope was not significantly different from zero (p > 0.05), an intercept-only model (assuming no change in resilient functioning over time) demonstrated much worse data fit (CFI = 0.833; TLI = 0.875; RMSEA = 0.153; SRMS = 0.093). Significant variance of intercept and slope (both p < 0.001) also indicated interindividual differences in initial levels of resilient functioning as well as in change over time, suggesting the appropriateness of investigating potentially underlying distinct trajectory classes with LCGA. To test for curvilinear patterns of change, we incorporated a quadratic term into the model, but resulting fit indices showed only slight improvement (CFI = 1.000; TLI = 1.000; RMSEA = 0.000; SRMS = 0.009) and information criteria were higher, suggesting worse model fit (e.g., BIClinear = 1543.654; BICquadratic = 1557.599). The nested χ2 difference test was also not significant ( = 0.726 well below critical cut-off value of 7.81, based on α = 0.05 and df = 3), therefore we retained the more parsimonious linear model.
Latent Class Growth Analysis
We compared fit indices of unconditional models with one to five latent classes (Table 1). Decreases in AIC, BIC, and SSABIC across consecutive models, reflected better model fit with increasing number of classes. However, information criteria increased from the four-class to the five-class solution, indicating worse fit of the latter model. Entropy was highest for the three-class solution, although classification accuracy was similar for the four-class solution. In fact, adj. LMR-LRT (p = 0.011) and BLRT (p < 0.001) indicated significant improvement in model fit for the four-class solution compared to the three-class solution. Moreover, one of the three latent classes contained only eight participants, barely more than the recommended minimum of 5% of the total sample (52). We therefore selected the four-class model which also had high average latent class probabilities (0.93, 0.89, 0.87, and 0.87, for classes 1-4, respectively), meaning that participants were assigned to the latent class to which they were most likely to belong.
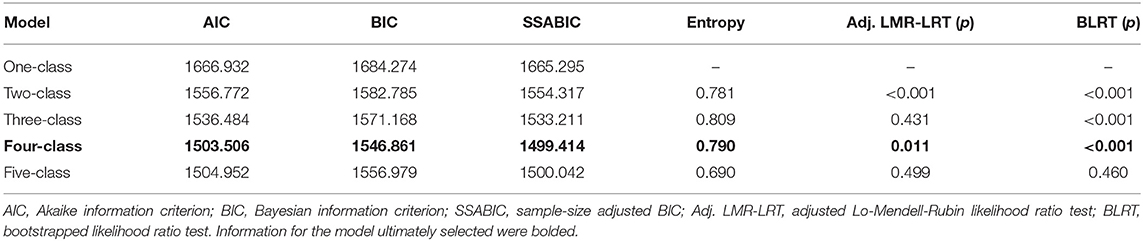
Table 1. Goodness of fit statistics for one- to five-class models of resilient functioning trajectories.
In the four-class model (Figure 2), the largest class comprised 46.6% of the sample, with participants showing the expected level of resilient functioning (intercept M = −0.24 ± 0.11, p = 0.032) with a marginal increase over time (slope M = 0.13 ± 0.07, p = 0.055). Because resilient functioning was operationalized as the residual from the regression of mental health on stressor load, values around zero denote the expected level of mental health given reported stress experience. Therefore, these participants exhibited neither high nor low, but rather expected or “medium” levels. The second-largest class (28.6%) followed a stable trajectory at “high” levels of resilient functioning (intercept M = 0.88 ± 0.29, p < 0.002; slope M = 0.04 ± 0.10, p = 0.706), i.e., participants consistently reported better than expected mental health given their stressor load. A third class, “medium-to-low” (15.8%), was characterized by expected levels of resilient functioning at baseline (intercept M = 0.34 ± 0.30, p = 0.254) and a marked decline over time (slope M = −0.70 ± 0.13, p <0.001). The last class (9%) included participants at rather “low” levels of resilient functioning (intercept M = −2.27 ± 0.41, p < 0.001), but whose trajectories indicated some improvement over time (slope M = 0.42 ± 0.20, p = 0.035).
To better compare the four trajectories, Table 2 provides details on demographics, resilience factors at baseline, and average mental health and stressor load across T0-T3 of each class. In addition, for each class, we plotted the trajectories of the variables from which the resilient functioning score was derived (Supplementary Figure 2).
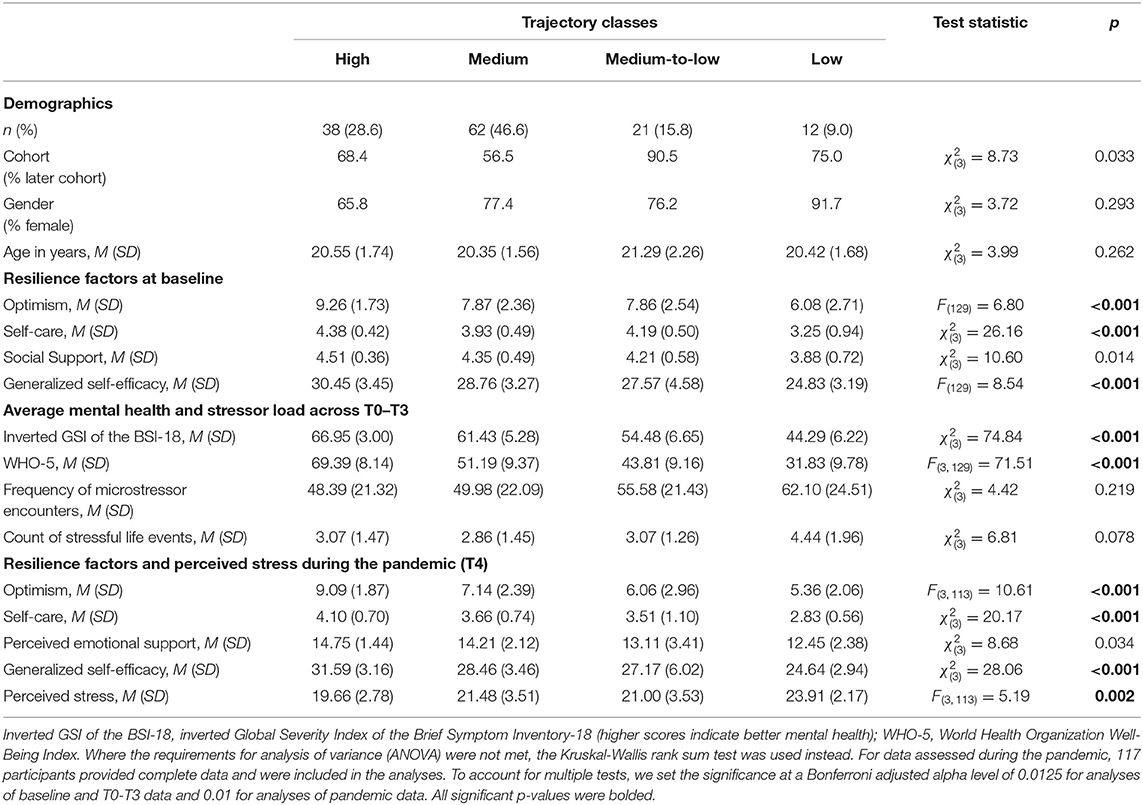
Table 2. Demographics, resilience factors at baseline, average stress and mental health across T0-T3, and resilience factors and perceived stress during the pandemic by class.
Predictors of Resilient Functioning Trajectory Class
We investigated the resilience factors (optimism, self-care, social support, and generalized self-efficacy) assessed at T0 as predictors of most likely latent class membership in a multinomial logistic regression analysis. Data screening revealed no apparent problems in terms of extreme outliers or multicollinearity among predictors. Overall, the model demonstrated satisfactory fit [ = 68.05, p <0.001; McFadden R2 = 0.21], correctly classifying 60% of the cases. However, prediction accuracy was not very good for smaller classes, likely reflecting unbalanced class sizes and ultimately sample size constraints. Whereas, 82% of participants assigned to the medium trajectory class and 53% of participants assigned to the high trajectory class were classified correctly, the rate of correct classification for the low and medium-to-low trajectory classes was only 33 and 24%, respectively. We set the low trajectory class as the reference category, comparing each of the other classes to this group. Only self-care emerged as a significant predictor of latent class membership. Compared to the low trajectory class, participants in the high and medium-to-low classes engaged in more self-care. Detailed results for all predictors included in the model are reported in Table 3.
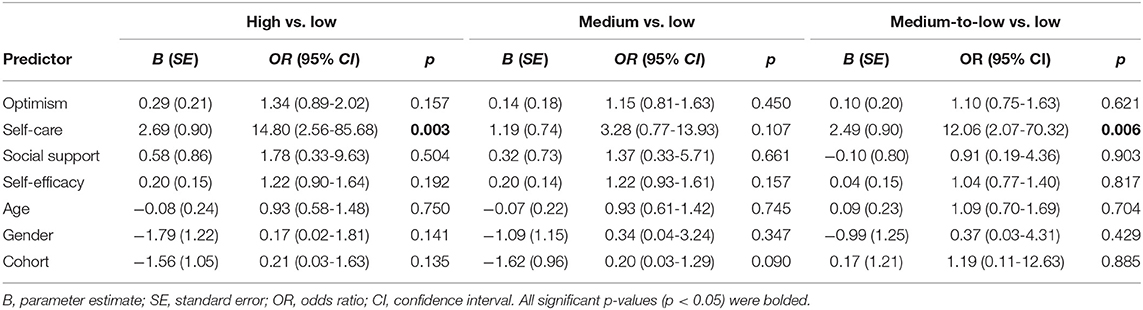
Table 3. Multinomial logistic regression results for predicting resilient functioning trajectory class.
Stress and Mental Health During the Lockdown
We analyzed data across the whole sample to characterize the impact of the COVID-19 pandemic, irrespective of resilient functioning trajectory class. Most of the participants agreed with government-mandated restrictions (M = 4.22, SD = 0.81) and reported that they followed recommendations to contain the spread of the virus (M = 4.50, SD = 0.57). Of 29 pandemic-specific stressors, participants experienced on average 10.4 (SD = 3.20; intensity: M = 3.23, SD = 0.65) with the most frequent being media reports (100%), loss of opportunity for recreational activities (97%), and loss of social contacts (90%). PSS scores indicated moderate levels of perceived stress overall (M = 21.14, SD = 3.39). Comparisons of data assessed at T3 and T4 showed no significant change in the frequency of microstressors [t(116) = 1.38, p = 0.170] or the count of stressful life events [t(116) = −1.08, p = 0.283]. Note that at T4 participants reported on stressful life events in the past 3 months (i.e., including the weeks before the pandemic). The comparison of inverted GSI scores of the BSI-18, assessed during and before the pandemic, revealed a significant decrease in symptoms during the lockdown [t(116) = −2.71, p = 0.008]. Correspondingly, WHO-5 scores showed a significant increase [t(116) = −2.17, p = 0.032], reflecting improved well-being during the lockdown.
Predicting Resilient Functioning During the Lockdown
Using multiple regression analysis, we examined resilient functioning trajectory class, resilience factors, and perceived stress as predictors of resilient functioning during the pandemic. Importantly, we focused on indices of optimism, self-care, perceived emotional support, and generalized self-efficacy re-assessed during the lockdown (see Table 2 for descriptive statistics by trajectory class). The data were checked for extreme outliers and parametric assumptions, with no apparent problems. There was also no evidence of multicollinearity among predictors (generalized variance inflation factors <3; see Supplementary Table 2 for zero-order correlations among predictors). All continuous predictors were mean centered and the reference level for resilient functioning trajectory class was set to the low trajectory class. The overall model was significant [F(8, 108) = 20.20, p <0.001] and the adjusted R2 indicated that 57% of the variation in resilient functioning was accounted for. We conducted a separate regression that included age, gender, and cohort as covariates, but a model comparison indicated no significant improvement [F(3, 105) = 1.07, p = 0.363]. We therefore retained the more parsimonious model with six predictors. In this model, trajectory class, self-care, and perceived stress emerged as significant predictors (see Table 4 for all results). Since the assumptions for ANOVA were not met, we conducted a Kruskal-Wallis rank sum test and Dunn's test with Holm-Bonferroni correction to follow up on class-dependent differences in resilient functioning. Results revealed significant differences [ = 53.37, p <0.001, η2H = 0.44] with participants in the high trajectory class showing significantly higher resilient functioning than participants in all other classes (all p <0.001) and participants in the low trajectory class showing lower resilient functioning than participants in all other classes (low vs. medium: p = 0.008; low vs. medium-to-low: p = 0.075). The contrast of medium vs. medium-to-low (p = 0.456) was not significant. More self-care and lower perceived stress was predictive of higher resilient functioning scores. Descriptive statistics (Table 2) indicated that participants in the high trajectory class took greater care of themselves during the pandemic compared to participants in the other classes, especially those in the low trajectory class. Hence, including an interaction term of class x self-care in the regression model yielded significant improvement in model fit [F(3, 105) = 3.93, p = 0.011] and the coefficient for medium-to-low class × self-care was significant (p = 0.031). This improved model explained 60% variation in resilient functioning during the lockdown (see Table 4 for results of both models).
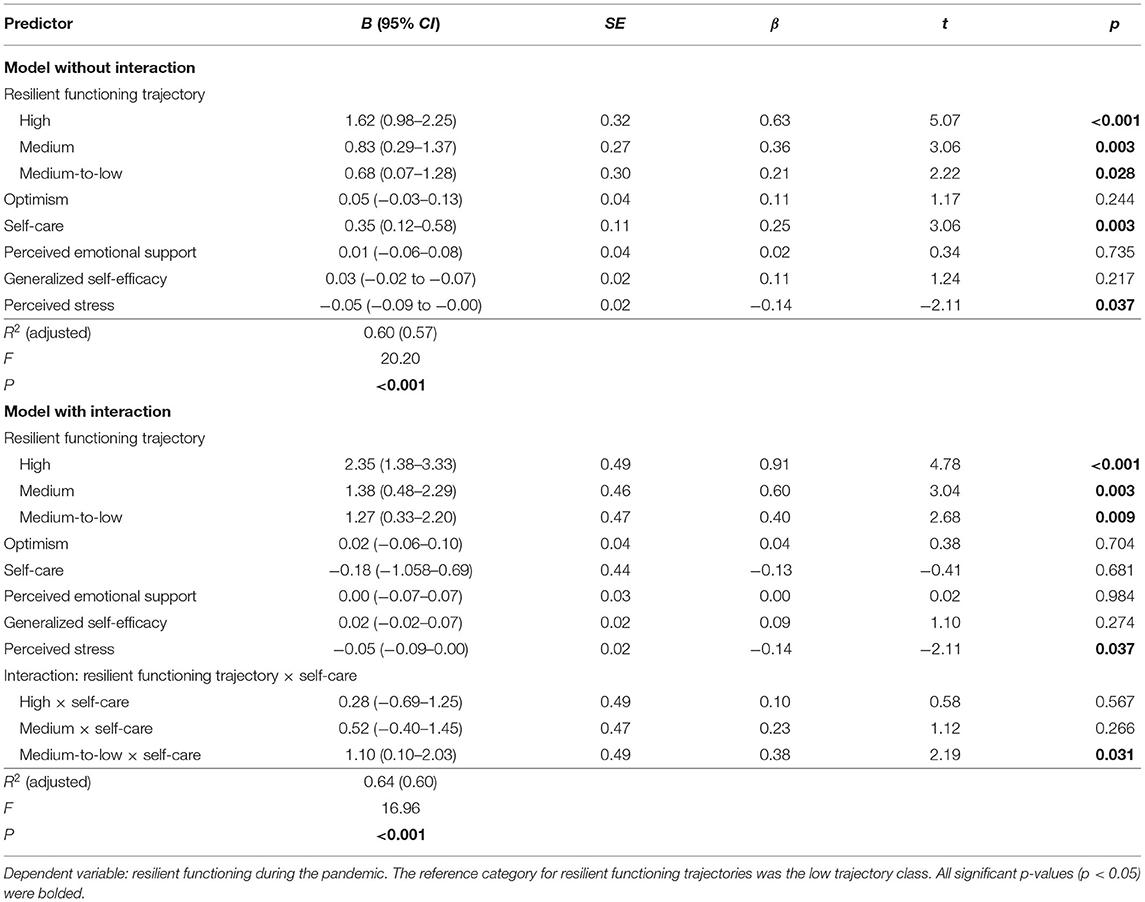
Table 4. Multiple regression results showing predictors of resilient functioning during the lockdown.
Discussion
In this study, we explored students' resilient functioning over a 9-month period before the outbreak of the COVID-19 pandemic and investigated links with baseline assessments of established resilience factors and with resilience during the first pandemic-related lockdown in Germany. Four distinct trajectories of pre-pandemic resilient functioning were identified: high, low, medium, and medium-to-low (i.e., progressive decline). Most participants' trajectories could be described as rather stable at expected levels of resilience. Given that we operationalized resilient functioning as the residual resulting from the regression of mental health on stressor load, it was expected that most participants would fall close to the regression line. However, the second-largest class showed higher than expected levels of resilient functioning and only a small proportion of the sample demonstrated markedly lower resilience. In line with this, studies tracking the course of psychological outcomes in the wake of a traumatic event (58–60), generally find that most participants maintained good levels of mental health. Moreover, because we focused on trajectories of resilience during everyday life, we observed relatively low counts of stressful life events that could affect students' mental health. In fact, trajectories seemed to be best distinguished by intercept rather than slope, suggesting little perturbation by stress. Although university students have been reported to show increased prevalence rates of anxiety and depression (9–11), we had initially screened potential participants for eligibility for the intervention study, thus our sample represents rather healthy students. They may not have faced very severe stressors or already have adaptive strategies at hand for coping with stress. Indeed, our analyses showed that participants with consistently high levels of resilient functioning scored highest on optimism, self-care, social-support, and perceived self-efficacy while participants with markedly lower levels of resilient functioning scored lowest. This confirms our expectation that higher expressions in these established resilience-promoting factors should go along with better mental health despite stress. However, in a multinomial logistic regression, only self-care emerged as a significant predictor of resilient functioning trajectory class. Self-care generally describes health-promoting behaviors, such as adequate sleep, healthy eating, exercise, and relaxation (61, 62). Previous research in different student populations has linked greater engagement in self-care to lower levels of stress and greater well-being (63–66). Self-care has also been reported to weaken the association between stress and quality of life (67). Our findings are in line with this and expand upon existing research in students by focusing specifically on resilience.
To investigate the effects of the pandemic as a global stressor, we first sought to assess all students' perceived stress as well as potential changes in their mental health and stressor load compared to the last assessment prior to the lockdown (independent of resilient functioning trajectory). All participants reported having experienced some pandemic-specific stressors, such as alarming reports by the media, but levels of perceived stress were moderate overall. We did not observe any significant increase in stressor load, nor any decrease in mental health. On the contrary, participants reported less symptoms of mental health problems and increased well-being during the pandemic. This was unexpected but ties in with a previous report on changes from before the pandemic to the first lockdown in a sample of the general population in Germany (68). Kohls et al. (69) provided a very comprehensive picture of over 3,000 university students assessed during this lockdown. According to their reports, more than half of the sample did not feel personally affected by the pandemic at that time and a majority perceived not only negative, but also positive aspects. In line with this, Ahrens et al. (68) discuss the concept of psychosocial gains from adversity (70), surmising that the pandemic, as a collectively experienced adverse event, may have strengthened social bonds.
Critically, the picture is very different when considering other populations. In March 2020, the WHO issued advice on how best to deal with the pandemic, paying particular attention to groups such as healthcare staff, carers of children, or older adults (71). Many studies have reported alarming rates of stress and mental health problems among healthcare workers during the first wave of the pandemic (72–74). It is crucial to note, however, that the first wave of the pandemic is unlikely to be representative of how students fared during subsequent waves. To date, there is a lack of research on stress and well-being during the later stages of the pandemic, but large-scale longitudinal projects are ongoing (75). In a preprint, Shevlin et al. (76) observed that most people consistently showed low levels of anxiety and depression and trajectories appeared to stabilize over the first year of the COVID-19 pandemic. Similarly, Prati and Mancini (77) noted that lockdowns did not show uniformly detrimental effects and most people appeared resilient. However, based on a systematic review of the prevalence of post-traumatic stress disorder in the wake of infectious disease pandemics in the 21st century, Yuan et al. (78) cautioned that more studies with longer follow-up times were needed to fully characterize the impact of this pandemic.
We found previous levels of resilient functioning to be predictive of students' resilience during the first lockdown. Specifically, those who had exhibited consistently high resilience and engaged in more self-care behaviors during the pandemic were characterized by high resilient functioning. However, given that students in our sample did not appear to be negatively affected by the lockdown and considering that self-care predicted higher resilience at baseline (i.e., several months before the outbreak of the pandemic), we cannot be sure that self-care presents a critical factor in dealing with pandemic-related stressors. Rather, it seems that self-care is generally important for mental health in the face of everyday life stress. It may be, however, that self-care played a more important role than other resilience factors during the first lockdown. After all, pandemic events were largely uncontrollable and associated with a loss of social contacts, thus, active coping driven by high perceived self-efficacy and the maintenance of social networks was complicated. This may have brought the self and, in turn, self-care behaviors to the fore. In general, intervention studies aiming to boost self-care behaviors, could show significant reductions in stress and depressed mood (79, 80). In cross-sectional studies comparing different coping strategies and protective factors during the initial stages of the pandemic, keeping regular routines, going outside, and limiting screen time emerged as particularly effective health-promoting behaviors (13, 81). Ornell et al. (82) also list self-care behaviors among mental health recommendations during pandemics. Given the severe strain healthcare workers in particular are under, psychosocial support programmes have been called for (83) and some hospitals have acted quickly to implement appropriate measures (84). Notably, many have advocated for organizations to promote self-care as an effective strategy to reduce stress and prevent mental health problems (85–87). Blake et al. (88) developed a digital learning package to promote well-being in healthcare workers during the pandemic, involving healthcare staff in the design process. A core component of the resulting package forms strategies for better self-care, underscoring the importance of self-care for mental health. Future studies should therefore determine the critical contribution of self-care to resilient functioning in students, especially during the later stages of the pandemic.
Some limitations must be considered when interpreting the findings of this study. First, our sample was rather small and the observed effects may therefore not be very robust. Given the unexpected and unprecedented outbreak of the COVID-19 pandemic, most studies that investigated its effects are cross-sectional in nature. Since we had data on students' stress and mental health available from before the pandemic, we focused on following up with these participants. While this restricted our sample size, our findings can shed further light on how characteristics assessed prior to the pandemic link to students' response to the first lockdown. Second, we are lacking potentially very interesting follow-up data from subsequent waves of the pandemic. Third, our sample is selective since we only assessed students from one university in Germany. Research has uncovered striking differences in the impact of the pandemic by country (89, 90), and initial regulations in Germany were county-specific (91), complicating comparisons even between different regions within the country.
Although findings should be interpreted with caution, they represent a rare examination of established resilience factors in relation to resilience over an extended time period and highlight the relevance of self-care in coping with real-life stressors such as the pandemic.
Data Availability Statement
Data and code (including Mplus files) are available on the Open Science Framework: https://osf.io/embcr/.
Ethics Statement
The studies involving human participants were reviewed and approved by the Ethics Committee of the Institute of Psychology, Johannes Gutenberg-University, Mainz, Germany. The participants provided their written informed consent to participate in this study.
Author Contributions
LM and MW: conceptualization. LM: methodology, software, formal analysis, writing-original draft preparation, and visualization. ES, SS, EE, and AB: investigation. LM and ES: data curation. LM, ES, and MW: writing-review and editing. MW: supervision and funding acquisition. ES, SS, EE, AB, and MW: project administration. All authors contributed to the article and approved the submitted version.
Funding
This research was funded by the State of Rhineland-Palatinate and the Leibniz-Institute for Resilience Research.
Conflict of Interest
The authors declare that the research was conducted in the absence of any commercial or financial relationships that could be construed as a potential conflict of interest.
Publisher's Note
All claims expressed in this article are solely those of the authors and do not necessarily represent those of their affiliated organizations, or those of the publisher, the editors and the reviewers. Any product that may be evaluated in this article, or claim that may be made by its manufacturer, is not guaranteed or endorsed by the publisher.
Acknowledgments
We thank all members of the BoosterIT team for their vital contribution, and all participants for taking part in this study.
Supplementary Material
The Supplementary Material for this article can be found online at: https://www.frontiersin.org/articles/10.3389/fpsyt.2021.784381/full#supplementary-material
References
1. World Health Organization. WHO Director-General's Opening Remarks at the Media Briefing on COVID-19 - 11 March 2020. (2020). Available online at: https://www.who.int/director-general/speeches/detail/who-director-general-s-opening-remarks-at-the-media-briefing-on-covid-19–11-march-2020 (accessed September 26, 2021).
2. Bundesregierung. Vereinbarung zwischen der Bundesregierung und den Regierungschefinnen und Regierungschefs der Bundesländer angesichts der Corona-Epidemie in Deutschland. (2020). Available online at: https://www.bundesregierung.de/breg-de/suche/vereinbarung-zwischen-der-bundesregierung-und-den-regierungschefinnen-und-regierungschefs-der-bundeslaender-angesichts-der-corona-epidemie-in-deutschland-1730934 (accessed September 26, 2021).
3. Bundesregierung. Besprechung der Bundeskanzlerin mit den Regierungschefinnen und Regierungschefs der Länder vom 22.03.2020. (2020). Available online at: https://www.bundesregierung.de/breg-de/themen/coronavirus/besprechung-der-bundeskanzlerin-mit-den-regierungschefinnen-und-regierungschefs-der-laender-vom-22-03-2020-1733248 (accessed September 26, 2021).
4. Bundesregierung. Pressekonferenz von Bundeskanzlerin Merkel, Ministerpräsident Söder und dem Ersten Bürgermeister Tschentscher im Anschluss an das Gespräch mit den Regierungschefinnen und Regierungschefs der Länder. (2020). Available online at: https://www.bundesregierung.de/breg-de/aktuelles/pressekonferenzen/pressekonferenz-von-bundeskanzlerin-merkel-ministerpraesident-soeder-und-dem-ersten-buergermeister-tschentscher-im-anschluss-an-das-gespraech-mit-den-regierungschefinnen-und-regierungschefs-der-laender-1751050 (accessed September 26, 2021).
5. Handelsblatt. Corona in Deutschland in der aktuellen Zusammenfassung. (2021). Available online at: https://www.handelsblatt.com/politik/deutschland/covid-19-corona-in-deutschland-in-der-aktuellen-zusammenfassung/25584942.html (accessed 24 August, 2021).
6. Gruber J, Prinstein MJ, Clark LA, Rottenberg J, Abramowitz JS, Albano AM, et al. Mental health and clinical psychological science in the time of COVID-19: challenges, opportunities, and a call to action. Am Psychol. (2020) 76:409–26. doi: 10.1037/amp0000707
7. Vindegaard N, Benros ME. COVID-19 pandemic and mental health consequences: systematic review of the current evidence. Brain Behav Immun. (2020) 89:531–42. doi: 10.1016/j.bbi.2020.05.048
8. Xiong J, Lipsitz O, Nasri F, Lui LMW, Gill H, Phan L, et al. Impact of COVID-19 pandemic on mental health in the general population: a systematic review. J Affect Disord. (2020) 277:55–64. doi: 10.1016/j.jad.2020.08.001
9. Auerbach RP, Mortier P, Bruffaerts R, Alonso J, Benjet C, Cuijpers P, et al. The WHO world mental health surveys international college student project: prevalence and distribution of mental disorders. J Abnorm Psychol. (2018) 127:623–38. doi: 10.1037/abn0000362
10. Eisenberg D, Gollust SE, Golberstein E, Hefner JL. Prevalence and correlates of depression, anxiety, and suicidality among university students. Am J Orthopsychiatry. (2007) 77:534–42. doi: 10.1037/0002-9432.77.4.534
11. Eissler C, Sailer M, Walter S, Jerg-Bretzke L. Psychische Gesundheit und Belastung bei Studierenden. Prävent Gesundheitsförderung. (2020) 15:242–9. doi: 10.1007/s11553-019-00746-z
12. Ma Z, Zhao J, Li Y, Chen D, Wang T, Zhang Z, et al. Mental health problems and correlates among 746 217 college students during the coronavirus disease 2019 outbreak in China. Epidemiol Psychiatr Sci. (2020) 29:e181. doi: 10.1017/S2045796020000931
13. Padrón I, Fraga I, Vieitez L, Montes C, Romero E. A study on the psychological wound of COVID-19 in university students. Front Psychol. (2021) 12:589927. doi: 10.3389/fpsyg.2021.589927
14. Herbert C, El Bolock A, Abdennadher S. How do you feel during the COVID-19 pandemic? A survey using psychological and linguistic self-report measures, and machine learning to investigate mental health, subjective experience, personality, and behaviour during the COVID-19 pandemic among university students. BMC Psychol. (2021) 9:90. doi: 10.1186/s40359-021-00574-x
15. Romeo A, Benfante A, Castelli L, Di Tella M. Psychological distress among italian university students compared to general workers during the COVID-19 pandemic. Int J Environ Res Public Health. (2021) 18:2503. doi: 10.3390/ijerph18052503
16. Matos Fialho PM, Spatafora F, Kühne L, Busse H, Helmer SM, Zeeb H. Perceptions of study conditions and depressive symptoms during the COVID-19 pandemic among university students in germany: results of the international COVID-19 student well-being study. Front Public Health. (2021) 9:674665. doi: 10.3389/fpubh.2021.674665
17. Elmer T, Mepham K, Stadtfeld C. Students under lockdown: Comparisons of students' social networks and mental health before and during the COVID-19 crisis in Switzerland. PLoS ONE. (2020) 15:e0236337. doi: 10.1371/journal.pone.0236337
18. Huckins JF, daSilva AW, Wang W, Hedlund E, Rogers C, Nepal SK, et al. Mental health and behavior of college students during the early phases of the COVID-19 pandemic: longitudinal smartphone and ecological momentary assessment study. J Med Internet Res. (2020) 22:e20185. doi: 10.2196/20185
19. van Zyl LE, Rothmann S, Zondervan-Zwijnenburg MAJ. Longitudinal trajectories of study characteristics and mental health before and during the COVID-19 lockdown. Front Psychol. (2021) 12:633533. doi: 10.3389/fpsyg.2021.633533
20. Voltmer E, Köslich-Strumann S, Walther A, Kasem M, Obst K, Kötter T. The impact of the COVID-19 pandemic on stress, mental health and coping behavior in German University students – a longitudinal study before and after the onset of the pandemic. BMC Public Health. (2021) 21:1385. doi: 10.1186/s12889-021-11295-6
21. Bonanno GA. Loss, trauma, and human resilience: have we underestimated the human capacity to thrive after extremely aversive events? Am Psychol. (2004) 59:20–8. doi: 10.1037/0003-066X.59.1.20
22. Chen S, Bonanno GA. Psychological adjustment during the global outbreak of COVID-19: a resilience perspective. Psychol Trauma. (2020) 12:S51–4. doi: 10.1037/tra0000685
23. Kaye-Kauderer H, Feingold JH, Feder A, Southwick S, Charney D. Resilience in the age of COVID-19. BJPsych Adv. (2021) 27:166–78. doi: 10.1192/bja.2021.5
24. Carver CS, Scheier MF, Segerstrom SC. Optimism. Clin Psychol Rev. (2010) 30:879–89. doi: 10.1016/j.cpr.2010.01.006
25. Cobb S. Social support as a moderator of life stress. Psychosom Med. (1976) 38:300–14. doi: 10.1097/00006842-197609000-00003
26. Ozbay F, Johnson DC, Dimoulas E, Morgan CA, Charney D, Southwick S. Social support and resilience to stress. Psychiatry. (2007) 4:35–40.
27. Bandura A. Self-efficacy: toward a unifying theory of behavioral change. Psychol Rev. (1977) 84:191–215. doi: 10.1037/0033-295X.84.2.191
28. Brown AD, Kouri NA, Rahman N, Joscelyne A, Bryant RA, Marmar CR. Enhancing self-efficacy improves episodic future thinking and social-decision making in combat veterans with posttraumatic stress disorder. Psychiatry Res. (2016) 242:19–25. doi: 10.1016/j.psychres.2016.05.026
29. Bender A, Ingram R. Connecting attachment style to resilience: contributions of self-care and self-efficacy. Pers Individ Differ. (2018) 130:18–20. doi: 10.1016/j.paid.2018.03.038
30. Riegel B, Dunbar SB, Fitzsimons D, Freedland KE, Lee CS, Middleton S, et al. Self-care research: where are we now? Where are we going? Int J Nurs Stud. (2021) 116:103402. doi: 10.1016/j.ijnurstu.2019.103402
31. Glaesmer H, Hoyer J, Klotsche J, Herzberg PY. Die deutsche Version des Life-Orientation-Tests (LOT-R) zum dispositionellen Optimismus und Pessimismus. Zeitschrift für Gesundheitspsychol. (2008) 16:26–31. doi: 10.1026/0943-8149.16.1.26
32. Scheier MF, Carver CS, Bridges MW. Distinguishing optimism from neuroticism (and trait anxiety, self-mastery, and self-esteem): a reevaluation of the Life Orientation Test. J Pers Soc Psychol. (1994) 67:1063–78. doi: 10.1037/0022-3514.67.6.1063
33. Harfst T, Ghods C, Mösko M, Schulz H. Erfassung von positivem Verhalten und Erleben bei Patienten mit psychischen und psychosomatischen Erkrankungen in der Rehabilitation – der Hamburger Selbstfürsorgefragebogen (HSF). Die Rehabil. (2009) 48:277–82. doi: 10.1055/s-0029-1239546
34. Fydrich T, Sommer G, Tydecks S, Brähler E. Fragebogen zur sozialen Unterstützung (F-SozU): Normierung der Kurzform (K-14). Zeitschrift Für Medizinische Psychol. (2009) 18:43–8.
35. Schwarzer R, Jerusalem M. Skalen zur Erfassung von Lehrer- und Schülermerkmalen: Dokumentation der psychometrischen Verfahren im Rahmen der wissenschaftlichen Begleitung des Modellversuchs Selbstwirksame Schulen. Berlin: Freie Universität Berlin (1999).
36. Spitzer C, Hammer S, Löwe B, Grabe H, Barnow S, Rose M, et al. Die Kurzform des Brief Symptom Inventory (BSI−18): Erste Befunde zu den psychometrischen Kennwerten der deutschen Version. Fortschritte der Neurologie Psychiatrie. (2011) 79:517–23. doi: 10.1055/s-0031-1281602
37. Derogatis LR. Brief Symptom Inventory (BSI)-18: Administration, Scoring and Procedures Manual. Minneapolis, MN: NCS Pearson. (2001) doi: 10.1037/t07502-000
38. Franke GH, Jaeger S, Glaesmer H, Barkmann C, Petrowski K, Braehler E. Psychometric analysis of the brief symptom inventory 18 (BSI-18) in a representative German sample. BMC Med Res Methodol. (2017) 17:14. doi: 10.1186/s12874-016-0283-3
39. Brähler E, Mühlan H, Albani C, Schmidt S. Teststatistische Prüfung und Normierung der deutschen Versionen des EUROHIS-QOL Lebensqualität-Index und des WHO-5 Wohlbefindens-Index. Diagnostica. (2007) 53:83–96. doi: 10.1026/0012-1924.53.2.83
40. Staehr Johansen K. The Use of Well-Being Measures in Primary Health Care – the DepCare Project. World Health Organization, Regional Office for Europe. Well-Being Measures in Primary Health Care – the DepCare Project. Geneva: World Health Organization (1998).
41. Chmitorz A, Kurth K, Mey LK, Wenzel M, Lieb K, Tüscher O, et al. Assessment of microstressors in adults: questionnaire development and ecological validation of the mainz inventory of microstressors. JMIR Ment Health. (2020) 7:e14566. doi: 10.2196/14566
42. Chmitorz A, Neumann RJ, Kollmann B, Ahrens KF, Öhlschläger S, Goldbach N, et al. Longitudinal determination of resilience in humans to identify mechanisms of resilience to modern-life stressors: the longitudinal resilience assessment (LORA) study. Eur Arch Psychiatry Clin Neurosci. (2020) 271:1035–51. doi: 10.1007/s00406-020-01159-2
43. Canli T, Qiu M, Omura K, Congdon E, Haas BW, Amin Z, et al. Neural correlates of epigenesis. Proc Natl Acad Sci USA. (2006) 103:16033–8. doi: 10.1073/pnas.0601674103
44. Veer IM, Riepenhausen A, Zerban M, Wackerhagen C, Puhlmann LMC, Engen H, et al. Psycho-social factors associated with mental resilience in the Corona lockdown. Transl Psychiatry. (2021) 11:67. doi: 10.1038/s41398-020-01150-4
45. Schneider EE, Schönfelder S, Domke-Wolf M, Wessa M. Measuring stress in clinical and nonclinical subjects using a German adaptation of the Perceived Stress Scale. Int J Clin Health Psychol. (2020) 20:173–81. doi: 10.1016/j.ijchp.2020.03.004
46. Cohen S, Kamarck T, Mermelstein R. A global measure of perceived stress. J Health Soc Behav. (1983) 24:385–96. doi: 10.2307/2136404
47. Schwarzer R, Schulz U. Soziale Unterstützung bei der Krankheitsbewältigung: Die Berliner Social Support Skalen (BSSS). Diagnostica. (2003) 49:73–82. doi: 10.1026//0012-1924.49.2.73
48. Amstadter AB, Myers JM, Kendler KS. Psychiatric resilience: longitudinal twin study. Br J Psychiatry. (2014) 205:275 −80. doi: 10.1192/bjp.bp.113.130906
49. Kalisch R, Köber G, Binder H, Ahrens KF, Basten U, Chmitorz A, et al. The frequent stressor and mental health monitoring-paradigm: a proposal for the operationalization and measurement of resilience and the identification of resilience processes in longitudinal observational studies. Front Psychol. (2021) 12:710493. doi: 10.3389/fpsyg.2021.710493
50. van Harmelen A-L, Kievit RA, Ioannidis K, Neufeld S, Jones PB, Bullmore E, et al. Adolescent friendships predict later resilient functioning across psychosocial domains in a healthy community cohort. Psychol Med. (2017) 47:2312–22. doi: 10.1017/S0033291717000836
51. Schüler K, Wessa M. Scripts to Calculate Stress Resilience Scores as Regression Residuals (2020). doi: 10.17605/OSF.IO/S7U23
52. Wickrama K, Lee TK, O'Neal CW, Lorenz F. Higher-Order Growth Curves and Mixture Modeling With Mplus: A Practical Guide. New York, NY: Routledge. (2016). doi: 10.4324/9781315642741
53. Hu L, Bentler PM. Cutoff criteria for fit indexes in covariance structure analysis: conventional criteria versus new alternatives. Struct Equ Model. (1999) 6:1–55. doi: 10.1080/10705519909540118
54. Nylund KL, Asparouhov T, Muthén BO. Deciding on the number of classes in latent class analysis and growth mixture modeling: a monte carlo simulation study. Struct Equ Model. (2007) 14:535–69. doi: 10.1080/10705510701575396
55. Geiser C. Datenanalyse mit Mplus. Wiesbaden: VS Verlag für Sozialwissenschaften (2011). doi: 10.1007/978-3-531-93192-0
56. Kassambara A. rstatix: Pipe-Friendly Framework for Basic Statistical Tests. R package version 0.5.0 (2020) Available online at: https://CRAN.R-project.org/package=rstatix
58. Bonanno GA, Galea S, Bucciarelli A, Vlahov D. Psychological resilience after disaster: New York City in the aftermath of the september 11th terrorist attack. Psychol Sci. (2006) 17:181–6. doi: 10.1111/j.1467-9280.2006.01682.x
59. Bryant RA, Waters E, Gibbs L, Gallagher HC, Pattison P, Lusher D, et al. Psychological outcomes following the Victorian Black Saturday bushfires. Aust N Z J Psychiatry. (2014) 48:634–43. doi: 10.1177/0004867414534476
60. Miller-Graff LE, Howell KH. Posttraumatic stress symptom trajectories among children exposed to violence. J Trauma Stress. (2015) 28:17–24. doi: 10.1002/jts.21989
61. Ayala EE, Omorodion AM, Nmecha D, Winseman JS, Mason HRC. What do medical students do for self-care? A student-centered approach to well-being. Teach Lern Med. (2017) 29:237–46. doi: 10.1080/10401334.2016.1271334
62. Walker SN, Sechrist KR, Pender NJ. The Health Promotion Lifestyle Profile II Omaha. Omaha, NE: University of Nebraska Medical Center, College of Nursing (1995).
63. Di Benedetto M, Towt CJ, Jackson ML. A cluster analysis of sleep quality, self-care behaviors, and mental health risk in australian university students. Behav Sleep Med. (2019) 18:309–20. doi: 10.1080/15402002.2019.1580194
64. Myers SB, Sweeney AC, Popick V, Wesley K, Bordfeld A, Fingerhut R. Self-care practices and perceived stress levels among psychology graduate students. Train Educ Profess Psychol. (2012) 6:55–66. doi: 10.1037/a0026534
65. O'Neill M, Yoder Slater G, Batt D. Social work student self-care and academic stress. J Soc Work Educ. (2019) 55:141–52. doi: 10.1080/10437797.2018.1491359
66. Zahniser E, Rupert PA, Dorociak KE. Self-care in clinical psychology graduate training. Train Educ Prof Psychol. (2017) 11:283–9. doi: 10.1037/tep0000172
67. Ayala EE, Winseman JS, Johnsen RD, Mason HRC. U.S. medical students who engage in self-care report less stress and higher quality of life. BMC Med Educ. (2018) 18:189. doi: 10.1186/s12909-018-1296-x
68. Ahrens KF, Neumann RJ, Kollmann B, Brokelmann J, von Werthern NM, Malyshau A, et al. Impact of COVID-19 lockdown on mental health in Germany: longitudinal observation of different mental health trajectories and protective factors. Transl Psychiatry. (2021) 11:392. doi: 10.1038/s41398-021-01508-2
69. Kohls E, Baldofski S, Moeller R, Klemm S-L, Rummel-Kluge C. Mental health, social and emotional well-being, and perceived burdens of university students during COVID-19 pandemic lockdown in Germany. Front Psychiatry. (2021) 12:643957. doi: 10.3389/fpsyt.2021.643957
70. Mancini AD. When acute adversity improves psychological health: a social–contextual framework. Psychol Rev. (2019) 126:486–505. doi: 10.1037/rev0000144
71. World Health Organization. Mental Health and Psychosocial Considerations During the COVID-19 Outbreak. Available online at: https://www.who.int/docs/default-source/coronaviruse/mental-health-considerations.pdf (accessed November 10, 2021).
72. Bohlken J, Schömig F, Lemke MR, Pumberger M, Riedel-Heller SG. COVID-19-pandemie: Belastungen des medizinischen personals. Psychiatrische Praxis. (2020) 47:190–7. doi: 10.1055/a-1159-5551
73. Greenberg N, Weston D, Hall C, Caulfield T, Williamson V, Fong K. Mental health of staff working in intensive care during COVID-19. Occup Med. (2021) 71:62–7. doi: 10.1093/occmed/kqaa220
74. Morawa E, Schug C, Geiser F, Beschoner P, Jerg-Bretzke L, Albus C, et al. Psychosocial burden and working conditions during the COVID-19 pandemic in Germany: the VOICE survey among 3678 health care workers in hospitals. J Psychosom Res. (2021) 144:110415. doi: 10.1016/j.jpsychores.2021.110415
75. Rusch T, Han Y, Liang D, Hopkins A, Lawrence C, Maoz U, et al. COVID-Dynamic: a large-scale multifaceted longitudinal study of socioemotional and behavioral change across the pandemic. PsyArXiv. (2021) doi: 10.31234/osf.io/75eyx
76. Shevlin M, Butter S, McBride O, Murphy J, Miller JG, Hartman TK, et al. Psychological responses to the COVID-19 pandemic are heterogeneous but have stabilised over time: one year longitudinal follow-up of the COVID-19 Psychological Research Consortium (C19PRC) Study. Psychol. Med. (2021) 1–3. doi: 10.1017/S0033291721004025
77. Prati G, Mancini A. The psychological impact of COVID-19 pandemic lockdowns: a review and meta-analysis of longitudinal studies and natural experiments. Psychol Med. (2021) 51:201–11. doi: 10.1017/S0033291721000015
78. Yuan K, Gong Y-M, Liu L, Sun Y-K, Tian S-S, Wang Y-J, et al. Prevalence of posttraumatic stress disorder after infectious disease pandemics in the twenty- first century, including COVID-19: a meta-analysis and systematic review. Mol Psychiatry. (2021) 26:4982–98. doi: 10.1038/s41380-021-01036-x
79. Greeson JM, Toohey MJ, Pearce MJ. An adapted, four-week mind–body skills group for medical students: reducing stress, increasing mindfulness, and enhancing self-care. Explore. (2015) 11:186–92. doi: 10.1016/j.explore.2015.02.003
80. Slatyer S, Craigie M, Heritage B, Davis S, Rees C. Evaluating the effectiveness of a brief Mindful Self-Care and Resiliency (MSCR) intervention for nurses: a controlled trial. Mindfulness. (2018) 9:534–46. doi: 10.1007/s12671-017-0795-x
81. Browning MHEM, Larson LR, Sharaievska I, Rigolon A, McAnirlin O, Mullenbach L, et al. Psychological impacts from COVID-19 among university students: risk factors across seven states in the United States. PLoS ONE. (2021) 16:e0245327 doi: 10.1371/journal.pone.0245327
82. Ornell F, Schuch JB, Sordi AO, Kessler FHP. “Pandemic fear” and COVID-19: mental health burden and strategies. Braz J Psychiatry. (2020) 42:232–5. doi: 10.1590/1516-4446-2020-0008
83. de Vroege L, van den Broek A. Results of mental support for health care professionals and mental care during the COVID-19 pandemic. J Public Health. (2021) 43:490–2. doi: 10.1093/pubmed/fdaa278
84. Chen Q, Liang M, Li Y, Guo J, Fei D, Wang L, et al. Mental health care for medical staff in China during the COVID-19 outbreak. Lancet Psychiatry. (2020) 7:e15–6. doi: 10.1016/S2215-0366(20)30078-X
86. Tierney M. Secure your mask first: the importance of self-care. J Am Psychiatr Nurses Assoc. (2021) 27:334–6. doi: 10.1177/10783903211023732
87. Clancy G, D'Arcy DG, Wlasowicz G. COVID-19 and mental health: self-care for nursing staff. Nursing. (2020) 50:60–3. doi: 10.1097/01.NURSE.0000694840.11164.75
88. Blake H, Bermingham F, Johnson G, Tabner A. Mitigating the psychological impact of COVID-19 on healthcare workers: a digital learning package. Int J Environ Res Public Health. (2020) 17:2997. doi: 10.3390/ijerph17092997
89. Aristovnik A, Keržič D, Ravšelj D, Tomaževič N, Umek L. Impacts of the COVID-19 pandemic on life of higher education students: a global perspective. Sustainability. (2020) 12:8438. doi: 10.3390/su12208438
90. Porter C, Favara M, Hittmeyer A, Scott D, Jiménez AS, Ellanki R, et al. Impact of the COVID-19 pandemic on anxiety and depression symptoms of young people in the global south: Evidence from a four-country cohort study. BMJ Open. (2021) 11:e049653. doi: 10.1136/bmjopen-2021-049653
91. Jetzig J, Markert R. Welche Corona-Regeln in welchem Bundesland gelten. Süddeutsche Zeitung. (2020). Available online at: https://www.sueddeutsche.de/politik/coronavirus-lockerungen-bundeslaender-1.4882790 (accessed November 29, 2021).
Keywords: self-care, latent class growth analysis, resilience, COVID-19 pandemic, mental health
Citation: Meine LE, Strömer E, Schönfelder S, Eckhardt EI, Bergmann AK and Wessa M (2021) Look After Yourself: Students Consistently Showing High Resilience Engaged in More Self-Care and Proved More Resilient During the COVID-19 Pandemic. Front. Psychiatry 12:784381. doi: 10.3389/fpsyt.2021.784381
Received: 27 September 2021; Accepted: 15 November 2021;
Published: 21 December 2021.
Edited by:
Haibo Yang, Tianjin Normal University, ChinaCopyright © 2021 Meine, Strömer, Schönfelder, Eckhardt, Bergmann and Wessa. This is an open-access article distributed under the terms of the Creative Commons Attribution License (CC BY). The use, distribution or reproduction in other forums is permitted, provided the original author(s) and the copyright owner(s) are credited and that the original publication in this journal is cited, in accordance with accepted academic practice. No use, distribution or reproduction is permitted which does not comply with these terms.
*Correspondence: Michèle Wessa, wessa@uni-mainz.de