- 1Department of Psychiatry, Center for Cognitive Medicine, Vanderbilt University Medical Center, Nashville, TN, United States
- 2Vanderbilt Department of Hearing and Speech Sciences, Vanderbilt University Medical Center, Nashville, TN, United States
- 3Department of Veterans Affairs Medical Center, Geriatric Research, Education and Clinical Center, Tennessee Valley Healthcare System, Nashville, TN, United States
Late-life depression (LLD) is a debilitating condition that is associated with poor response to antidepressant medications and deficits in cognitive performance. Nicotinic cholinergic stimulation has emerged as a potentially effective candidate to improve cognitive performance in patients with cognitive impairment. Previous studies of nicotinic stimulation in animal models and human populations with cognitive impairment led to examining potential cognitive and mood effects of nicotinic stimulation in older adults with LLD. We report results from a pilot study of transdermal nicotine in LLD testing whether nicotine treatment would enhance cognitive performance and mood. The study used electroencephalography (EEG) recordings as a tool to test for potential mechanisms underlying the effect of nicotine. Eight non-smoking participants with LLD completed EEG recordings at baseline and after 12 weeks of transdermal nicotine treatment (NCT02816138). Nicotine augmentation treatment was associated with improved performance on an auditory oddball task. Analysis of event-related oscillations showed that nicotine treatment was associated with reduced beta desynchronization at week 12 for both standard and target trials. The change in beta power on standard trials was also correlated with improvement in mood symptoms. This pilot study provides preliminary evidence for the impact of nicotine in modulating cortical activity and improving mood in depressed older adults and shows the utility of using EEG as a marker of functional engagement in nicotinic interventions in clinical geriatric patients.
Introduction
Late Life Depression (LLD) or geriatric depression, is the occurrence of major depressive disorder in adults over 60 years of age (1). LLD is associated with impaired cognitive performance (2). A major issue for the treatment of adults with LLD is that patients have a poor response rate to current antidepressants (3). A recent meta-analysis of studies assessing antidepressant treatment response in the elderly demonstrated a 50.7% response rate of patients with LLD (4). Poor treatment response has been associated with executive dysfunction (5, 6), as well as other cognitive domains including processing speed, language and episodic memory (7). As a response to these difficulties in ensuring treatment response in older adults, new strategies are being developed to address this problem. It is also important to understand mechanistically how such treatments may contribute to the clinical response. One of the more promising techniques for use in rapidly evaluating the cortical effects of potential treatments in LLD is electroencephalography (EEG), which has the advantages of being both cost-effective and with high temporal resolution, which allows for the examination of direct markers of the cortical activity response to treatment.
EEG has been utilized in adults with LLD to identify the differences in cortical activity compared to healthy older adults. Previous research found that patients with LLD display slower information processing with longer P300 latencies to auditory stimuli, as well as diminished inhibitory processing compared to healthy controls (8, 9). LLD patients have also been observed to exhibit greater slow wave power at rest compared to age-matched controls (8, 10). As shown in younger adults, EEG may serve as a predictor of response to antidepressant treatment (11, 12). A six-week study of paroxetine in men with major depressive disorder (MDD) showed that in addition to improved mood symptoms, chronic treatment led to a reduction in alpha power and an increase in delta, theta and beta power, particularly at bilateral frontal areas (13). Baseline EEG markers, including N1 amplitudes to oddball trials and resting frontal theta power have also been associated with greater subsequent reductions of depressive symptoms over 8 weeks of treatment (14).
A novel treatment with preliminary evidence for benefit to both mood and cognitive performance in LLD is nicotine (15). Both preclinical and clinical trials of nicotine have observed improvements in mood following nicotinic stimulation (16–19). Beyond mood, nicotine appears to improve the cognitive performance of healthy adults, specifically on tasks assessing attention (20, 21). These results have also been seen in older cognitively impaired patients, with a 6-month trial of transdermal nicotine in patients with Mild Cognitive Impairment (MCI) demonstrating improved sustained attention performance compared to placebo (22).
EEG may serve as a useful measure for the effects of nicotine on brain function. Nicotine in healthy adults has been shown to reduce slow wave power and increase power in both alpha and beta bands (23–26). Nicotine also reduces the amplitude of the novelty P3a while increasing the amplitude of the salient P3b (27, 28). In contrast to healthy adults, acute nicotine treatment in adolescent females with MDD has been observed to reduce alpha power over the left hemisphere and increase power over the right hemisphere and central areas (29). However, there has been no examination of chronic nicotine treatment effects on EEG markers in MDD or LLD.
In this study, we evaluated EEG as a marker of treatment response in a small open-label pilot study of transdermal nicotine in adults with LLD. Patients with LLD completed an EEG recording at baseline and following 12 weeks of nicotine treatment. The present study included a subset of participants from a larger pilot trial of transdermal nicotine (30). We examined resting EEG activity pre- and post-nicotine intervention to assess changes to underlying frequency bands. Changes in attention were assessed using an auditory oddball task. We hypothesized that 12 weeks of nicotine treatment would result in a reduction of resting alpha power, and an increase in resting beta power over frontal regions. For the oddball task we examined the behavioral data and the event-related potentials and hypothesized that nicotine intervention would improve attention performance, resulting in greater accuracy and an increased parietal P300 peak for target compared to standard trials. We also examined event-related oscillations during the oddball task, to examine whether the nicotine intervention altered EEG power during attention task performance. Finally, an exploratory aim of the study was to determine whether changes in EEG were associated with changes in depressive symptoms.
Materials and Methods
Participants
Participants were recruited at Vanderbilt University Medical Center from clinical referrals and community advertisements from November 2016 through April 2017, with the study ending in August 2017. Core entry criteria focused on adults aged 60 years or older meeting DSM-IV-TR criteria for Major Depressive Disorder, recurrent or single episode, with a baseline depression severity measured by the Montgomery-Åsberg Depression Rating Scale [MADRS, (31)] of ≥15. Criteria related to cognitive function specified a Montreal Cognitive Assessment [MoCA, (32)] score of ≥24 but reporting subjective cognitive impairment, defined as endorsing ≥20% of items on the Cognitive Complaint Index [CCI, (33)]. Eligible participants could either be antidepressant-free or currently taking antidepressant monotherapy, however those taking antidepressants needed to be on a stable dose for at least 8 weeks. Additional exclusion criteria included: (1) Current tobacco or nicotine use in last year; (2) Other psychiatric disorders, except for anxiety symptoms occurring during a depressive episode; (3) History of alcohol or drug abuse over last 3 years; (4) Primary neurological disorders including dementia; (5) Regular use of drugs with centrally acting cholinergic or anticholinergic properties in the last 4 weeks; (6) Current psychotherapy. All participants provided written informed consent. The study was approved by the Vanderbilt University Medical Center Institutional Review Board. The study was registered with ClinicalTrials.gov (NCT02816138).
Study Design
Participants were seen every 3 weeks plus a telephone call assessing tolerability at week 1. At each study clinic visit: (1) Depression severity was assessed by the study physician using the MADRS, (2) Subjective cognitive symptoms were assessed using the PROMIS (34), (3) Vital signs were assessed including sitting blood pressure, heart rate and weight, (4) Medication adherence was assessed using the Medication Adherence Questionnaire (35), and a patch count. EEG recordings took place at baseline (week 0) and following the completion of full dose nicotine treatment and before tapering (week 12).
Study Drug Administration and Dosing
Transdermal nicotine was administered in a flexible dose escalation strategy with the ability to reduce to previous or intermediate doses for tolerability. The dose escalation strategy was: 3.5 mg (half of 7 mg patch) in week 1, 7 mg in weeks 2 and 3, 14 mg in weeks 4 through 6, and 21 mg in weeks 7 through 12. The target dose was 21 mg; however dose escalation or reductions were based on tolerability; participants were titrated to the highest dose that could be tolerated without side effects. If a participant had tolerability issues while using the 14 mg patch, the dose was initially reduced to an intermediate dose of 10.5 mg (half of 21 mg patch) and could be further reduced to 7 mg if needed. Participants were instructed to wear the study patch during the day and remove it at bedtime (~16 h daily); they were also instructed to move the patch location daily. Following trial completion, doses were tapered and discontinued over 3 weeks. Participants were seen at week 15 for a final visit.
EEG Recording
EEG activity was recorded using a 128-channel Geodesic sensor net [EGI, Inc., Eugene, OR; (36–38)]. The EEG was sampled at 250 Hz with filters set at 0.1–100 Hz. During data collection, all electrodes were referenced to vertex (Cz). During the resting state recording participants had their eyes open and focused on a gray-fixation cross placed on a black screen. The resting state recording lasted for 3 min.
An auditory oddball task was performed to assess auditory attention (39, 40). A pair of two pure tones (single formant) at 1,000 and 1,500 Hz were the stimuli. Tones were equated in duration (300 ms) and rise/decay times. Tones were presented at 75 dB SPL (measured at the ear) through a speaker positioned 1 meter in front of the participant. A total of 200 trials were presented with the inter-stimulus interval varying randomly between 1,000 and 1,300 ms to prevent habituation to stimulus onset. The assignment of stimuli (high or low frequency) to standard and target conditions (70 and 30% of the trials, respectively) was counterbalanced across participants. Each participant was asked to press a different button using their preferred hand upon presentation of the standard and the target. On average, the auditory task lasted 6–7 min.
EEG Processing
EEG data were processed using MATLAB (MATLAB, 2020) through a pipeline utilizing Fieldtrip (41), EEGLab (42), and CSD Toolbox (43) and in-house functions (A. Conley). Pre-processing was performed using Fieldtrip as follows. After being imported, EEG data was filtered using a high pass and notch filter to remove line noise and low-frequency drift (high pass: 0.1 Hz, forward phase; 60 Hz notch: zero phase). Excessively noisy channels were identified with visual inspection and excluded. For each oddball trial type (standard and target) epochs were extracted from −500 to 1,500 ms with respect to stimulus onset. For the resting state recording, the recorded data was broken up into 2,000 ms epochs. To remove blink and vertical eye-movement artifact, independent components analysis (ICA) was performed using the fastICA algorithm (44). This produces a set of components, 1 less than the number of available electrodes. Based on visual inspection by a trained observer, an average of 2.9 ± 0.5 components were removed that corresponded to ocular artifact (i.e., a deflection consistent with the time course of an eyeblink coupled with a frontal topographical distribution). The remaining components were projected back into sensor (electrode) space. The data were low pass filtered (30 Hz, zero-phase) to remove high frequency noise including muscular artifacts. Trials that contained residual artifact larger than ±150 μV were deleted. After artifact rejection, the surface Laplacian transformation of the EEG data was computed. For the surface Laplacian, a spherical spline function was applied across all scalp electrode locations, with the spline flexibility parameter, m = 4, for increased rigidness (45).
ERP Analysis
Evoked potentials were extracted for standard and target trials in the oddball task over the midline parietal cortex. ERPs were baselined between −250 and −50 ms prior to stimulus onset. For the P300 waveform mean amplitude was extracted between 250 and 500 ms post-stimulus onset. The peak latency was extracted over this time window.
EEG Power Analyses
EEG power was obtained by calculating the average of all decomposed single-trial time-frequency data using complex Morlet wavelets with 80 logarithmically scaled bins from 2 to 50 Hz (46). For the oddball task, power was calculated as a difference in respect to pre-stimulus baseline activity, which was defined as −250 to −50 ms prior to stimulus onset. No baseline correction was used to calculate the evoked power during the resting state recording. Significant changes in EEG power were identified using a process outlined by Cooper et al. (47). To identify significant and common changes in power from the pre-stimulus baseline, we combined data from both pre- and post-intervention sessions into a single task average for midline clusters at frontal, central and parietal locations [corresponding to Fz, Cz, and Pz according to the 10-10 system; (48)]. Following this, we performed one-sample t-tests at each frequency × time point for the three midline clusters for each of the tasks, with multiple comparison correction applied [false discovery rate, FDR p = 0.01; (49)]. Based on this analysis, we were able to identify common power processes associated with the tasks (see Figure 1). Next, we used these significant frequency × time clusters as masks and extracted average power for each of the four frequencies of interest (i.e., delta, theta, alpha, and beta) for each of the tasks (oddball and resting state) that were entered into the statistical analyses.
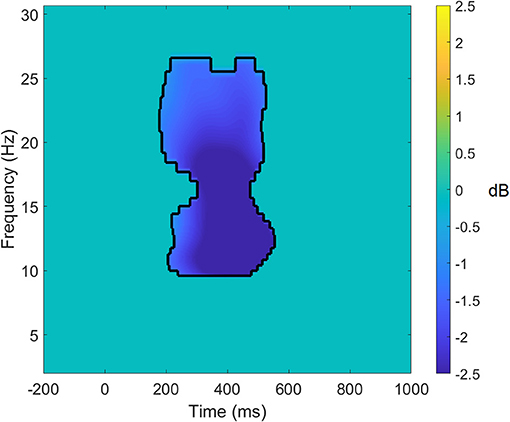
Figure 1. Significant target average data for the parietal cluster for the auditory oddball task. Data within the black lines represent frequency × time points that remain significant following false discovery rate correction of p < 0.01. All other data that does not survive the correction has been set to 0. Power differences are in dB.
Statistical Analyses
Changes in vital signs from Baseline to Week 12 were assessed using paired t-tests. Measurements of accuracy, response time, ERP and evoked oscillations derived from the oddball task were analyzed using a 2 × 2 repeated measures analysis of variance (ANOVA), with within-subjects' factors of time (baseline vs. week 12) and trial type (standard vs. target). Analysis of the different clusters (frontal, central, and parietal) of evoked EEG power were performed separately. Measures of resting EEG power were assessed by a 2 × 3 repeated measures ANOVA with within-subjects' factors of session and site (frontal, central, and parietal). Relation of evoked EEG activity to depressed symptoms (MADRS score) was completed by Pearson correlation. Statistical analyses were performed in jamovi stats version 1.2 (50).
Results
Participants
Fifteen participants were enrolled in the study, and 10 completed the EEG recordings. Markers of the safety and tolerability, as well as the clinical effectiveness of the nicotine treatment are reported in detail in Gandelman et al. (30). One participant did not complete their follow-up visit, and one participant had to be excluded due to EEG artifacts. Average trial numbers for the participants that were included in the analysis for the oddball task were for Baseline: 62.9 ± 26.7 standards and 24.8 ± 12.6 targets, and for Week 12: 68.4 ± 25.9 standards and 31.5 ± 5.3 targets. The demographic information of the final sample of 8 participants is described in Table 1. All 8 participants in the final sample were Caucasian.
Tolerability of Nicotine Intervention
No changes in blood pressure or pulse were reported across the 12 weeks of treatment. There was a significant decrease in body weight (mean decrease: 3.57 kg, t = 3.2, p = 0.015, d = 1.14), and BMI (mean decrease: 1.04, t = 3.25, p = 0.014, d = 1.15) for participants from Baseline to Week 12. The mean final patch dose was 16.63 mg (SD = 6.1 mg, range 7–21 mg) with 5 achieving the maximum 21 mg dose. Participants exhibited >90% medication adherence on average with study patches.
Auditory Oddball Results
Behavioral Results
The results of participants on the auditory oddball task are reported in Table 2. Participants' accuracy performance on the oddball task remained stable across the 12-week intervention (Baseline: 81 ± 16.1%; Week 12: 84 ± 11.1%), and there was no effect of either time or trial type (all p > 0.3). However, participants responded significantly faster after nicotine treatment compared to baseline [488 ± 28.6 vs. 434 ± 20.4 ms, F(1, 7) = 12.5, p < 0.005, = 0.64], with the greatest improvement from baseline to post-intervention being in response to targets compared to non-target standards [Standard: 474 ± 82.4 vs. 434 ± 64.6 ms; Target: 502 ± 79.4 vs. 434 ± 50.9 ms; Trial × Session: F(1, 7) = 6.4, p = 0.04, = 0.48].
Event-Related Potentials
As shown in Table 2, the P300 amplitudes decreased for both oddball and standard trials after the 12-week intervention, however the decline in amplitude was larger for standard trials (1.6 vs. 1.4 μV). The latency of the P300 evoked by participants decreased for both trial types by between 20 and 30 ms (29 vs. 25 ms) following treatment. Analysis of the ERP markers of mean amplitude and the peak latency of the parietal P300 showed no effect of time, trial type or an interaction between the two factors (all p > 0.05).
Event-Related Power
The results of the analysis of the task-averaged power evoked at the frontal cluster revealed a mask of significant time × frequency values in the delta band between 400 and 800 ms and the beta bands between 200 and 500 ms. For the central cluster, the mask showed significant time × frequency values in the delta band between 400 and 600 ms, in the alpha band between 200 and 400 ms, and in the beta band between 200 and 600 ms. In the parietal cluster, the task-averaged data showed significant differences from zero in the alpha and the beta bands between 200 and 500 ms post-stimulus.
The results of the repeated measures ANOVA for the frontal cluster identified a significant main effect of the trial type in the delta band, with greater evoked delta power for targets compared to standards [0.52 ± 0.9 vs. 1.97 ± 1.6 dB; F(1, 7) = 8.5, p = 0.02, = 0.55]. However, there was no difference in the evoked delta power between baseline and Week 12 (F <1). There was also no difference in the evoked beta power at the frontal cluster (all p > 0.2).
As with the frontal cluster, the ANOVA of the central cluster revealed large differences between trial types in all frequency bands (all p < 0.005). This trial effect evoked greater synchronization of delta power, and greater desynchronization of alpha and beta power to targets compared to standards. However, there was no impact of the nicotine treatment on power in the delta, alpha or beta bands.
The analysis of the parietal cluster (see Figure 2) showed a significant main effect of the trial type in the alpha band, with greater desynchronization of alpha power for targets compared to standards [−0.39 ± 0.25 vs. −1.77 ± 1.3 dB; F(1, 7) = 14.2, p < 0.005, = 0.67]. There was however no difference in the evoked alpha power between baseline and Week 12 (F <1). The analysis of the beta band showed that following 12 weeks of nicotine treatment, there was a reduction in the desynchronization of beta over the parietal cluster [Baseline: −0.85 ± 0.5 vs. Week 12: −0.6 ± 0.45 dB; F(1, 7) = 11.1, p = 0.013, = 0.61]. This shift in beta power across the 12-week treatment period was greater for target trials compared to standard trials [mean difference 0.37 vs. 0.14 dB; Trial × Session: F(1, 7) = 5.4, p = 0.05, = 0.44].
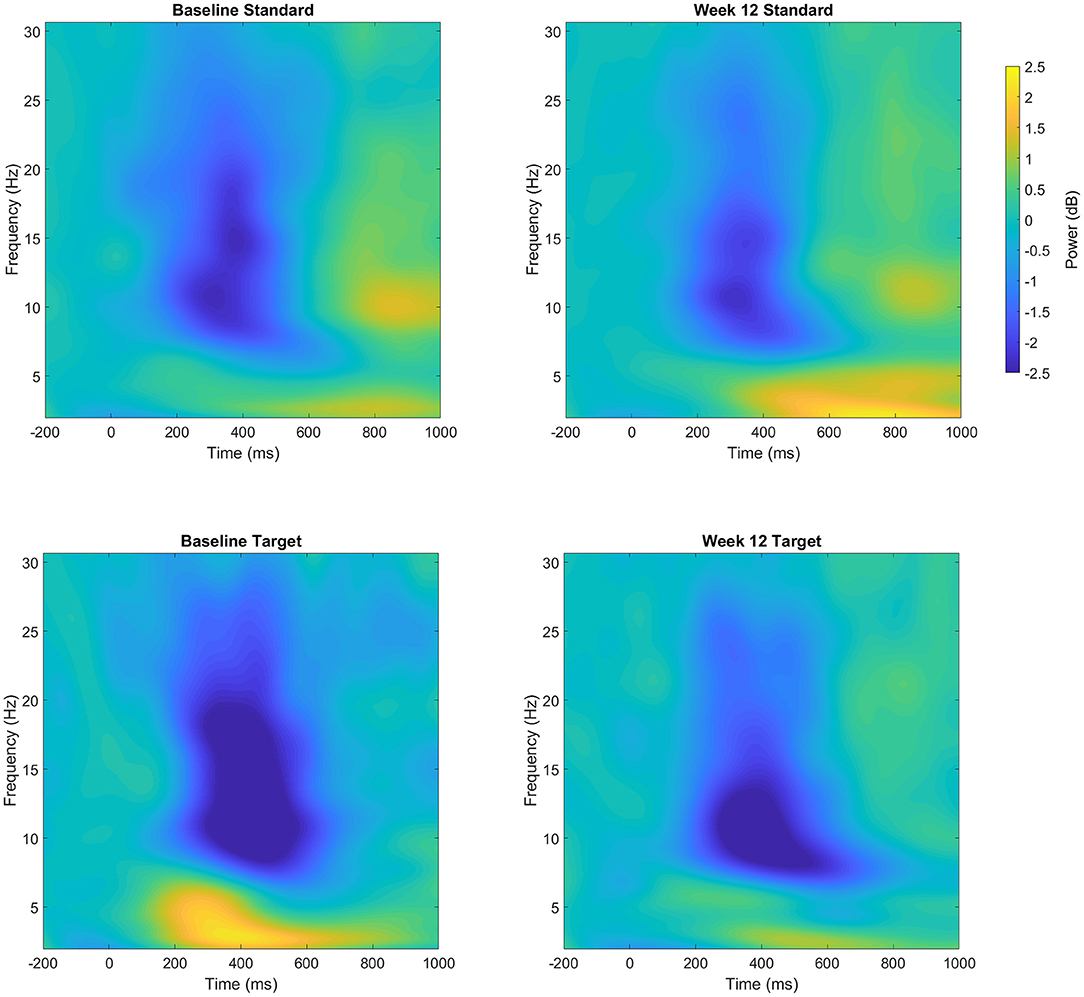
Figure 2. Event-related power (dB) at both Baseline and Week 12 following standard (top row) and target (bottom row) trials over the parietal cluster. Changes in power reflect increases or decreased magnitude compared to the pre-stimulus baseline.
Resting State EEG Results
The mean resting power at baseline and at week 12 is displayed in Table 3. The results of the session average resting data revealed significant time × frequency differences from 0 for all frequency bands at each of the three midline clusters. However, the analysis of the midline clusters did not show any change in resting power following nicotine intervention for any of the frequency bands (all p > 0.1). There was a significant difference in resting beta power across the sites, which showed a reduction in beta power from frontal to central clusters, with the lowest power at the parietal cluster [F(2, 14) = 3.96, p = 0.044, = 0.36]. However, this difference in resting power across the scalp did not change with nicotine treatment (p = 0.3).
Relationship to Depressive Symptomatology
Analysis of the relationship of improvement in depression score and EEG/ERP signals following nicotine treatment showed that the reduction in MADRS scores after nicotine treatment was significantly correlated with the post-intervention parietal beta power for standard tones (Figure 3; r = 0.8, p < 0.02). This relationship reflected that greater improvement in MADRS scores following nicotine treatment were associated with a greater reduction of parietal desynchronization to standard trials. The reaction time performance of the oddball task or the resting beta power did not correlate with the change in depressive symptoms across the nicotine treatment period (all p > 0.1).
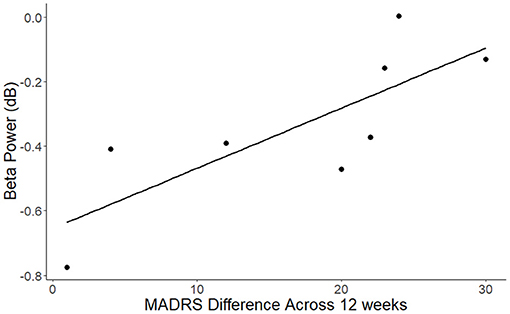
Figure 3. Association between change in MADRS scores across the nicotine treatment period and beta power for standard trials over the parietal cluster (r = 0.8, p < 0.02). Beta values reflect decreases compared to the pre-stimulus baseline.
Discussion
This pilot study showed that 12-week treatment of open-label transdermal nicotine produced improved attention performance in patients with LLD. This functional engagement of the nicotinic system was evident in reduced reaction time and the reduction of beta desynchronization during the oddball task following nicotine treatment. The change in parietal beta power was correlated with mood improvements on the MADRS.
Twelve weeks of transdermal nicotine resulted in improved performance on the oddball task, although not as we had hypothesized. While we had predicted improved accuracy and increased amplitude of the target P300, however compared to baseline, neither of these measures changed across the intervention period. Instead, participants exhibited faster reaction time, with the enhancement being greater for targets compared to standards. Consistent with the reaction time changes, desynchronization of beta power over the parietal cortex was reduced for both target and standard trials following nicotine intervention, with greater desynchronization for target trials. Moreover, the changes in beta oscillations for the standard trials was also correlated with the change in MADRS scores, with greater beta power corresponding to a greater reduction in depressive symptoms. While this was an open-label trial, and therefore we cannot rule out the change in oddball performance to be related to time or practice, the finding that the changes in evoked oscillations were associated with a positive change in MADRS scores across the intervention period suggests that we observed functional engagement of the cholinergic system, resulting in improved attention performance which correlated with improved mood. Beta oscillations are commonly associated with visual attention and motor performance (51). In addition, cholinergic stimulation, in this case through transdermal nicotine, is known to modulate arousal and attention (20, 52). Therefore, this finding suggests that observed a reduction of parietal beta band desynchronization following nicotine is related to an improvement of attention performance, through the reduction of task-irrelevant activity, resulting in improved performance on the oddball task. In contrast to prior studies and to our results from the oddball task, we did not see a significant change in resting EEG power across the nicotine treatment period.
We did not see changes in the alpha band. Attention in healthy adults has been also related to oscillations in the alpha band, and specifically a desynchronization over the parietal cortex (53). Alpha synchronization is associated with inhibition of activity (54, 55), and also mental fatigue and drowsiness (56, 57). Desynchronization of the alpha band is associated with temporal attention and sensory gating (58, 59). Specifically in auditory tasks, alpha oscillations are thought to drive attention allocation in the cortex (60, 61). Therefore, the fact that task-relevant alpha desynchronization was not substantially altered across treatment may reflect efficient processing or may be a result of the age-related slowing of the EEG spectra, whereby across healthy aging, the power of faster frequency bands decreases, while the slower power bands increase in power (62). Slowing of the spectra is increased in clinical populations including those with MCI (63). Thus, a change in beta power following treatment may be more noticeable in older adults, due to reduced power compared to the slower frequency bands.
Depression is associated with deficits in attention processes (64, 65). These deficits in attention have downstream effects on several higher order cognitive processes including executive functions (66). Attention itself has been characterized as having four hierarchical subdomains, including intrinsic alertness, sustained attention, focused or selective attention and behavioral inhibition (67, 68). Compared to healthy controls, adults with MDD show deficits on selective attention (69, 70), and sustained attention (71, 72). Within the context of the present study, the results indicate that nicotine may be enhancing sustained attention. While there was not a substantial change in accuracy across the 12 weeks, responses were faster following treatment. There may also be an impact of the improved mood, as evidenced by a decline in MADRS scores, on attention. Positive mood has previously been reported as an enhancer of other cognitive processes (73–75). For auditory attention, recent studies have shown that in healthy controls improving mood through music or mindfulness enhances performance to auditory stimuli (76, 77). As such, the improvement of attention by cholinergic stimulation, and the enhancement of mood may be additive factors for cognitive processing.
The finding of significant oscillatory changes in the parietal cortex may indicate that the cholinergic stimulation is improving frontoparietal connections in adults with LLD. Healthy aging is associated with a shift in functional activity from parietal to frontal regions (78, 79), but this shift is exacerbated in the presence of Alzheimer's pathology (80, 81) and also depressive symptoms (82, 83). As the parietal cortex is important for both auditory and visual attention (53, 84–86), the posterior-anterior shift in processing results in a reorganization of cortical activation for attention tasks (78). This is observed in healthy older adults, with P300 amplitudes shifting from parietal to frontal areas (87, 88). Adults with MDD show a reduction in frontoparietal connectivity during both visual and auditory attention tasks (89–91). In the context of the present study, cholinergic stimulation through nicotine treatment may be stimulating activity in the less functionally active areas of the parietal cortex during attention tasks. This activation during attention tasks may promote increased connectivity within the frontoparietal control network, and lead to downstream improvements in cognitive control in adults with LLD.
In contrast to previous studies, we did not find changes in resting oscillatory activity following nicotine treatment. This may be due to the differences in age or the focus of the analysis on midline clusters rather than looking at hemispheric differences. As discussed above, Jaworska et al. found an increase in global alpha power over the right hemisphere in young women with MDD following acute transdermal nicotine (29). Lateralization of brain activity have been reported consistently in younger adults with MDD (92) however, this finding has not been reliably found in adults with LLD (93). Future investigation would be needed to confirm whether functional lateralization is seen following nicotinic treatment in adults with LLD.
A limitation of this pilot study is that the nicotine was administered to patients in an open-label design, and as such there was not a placebo group to compare the effects across the 12-week period. The sample of participants was uniformly Caucasian, which does limit the generalizability of the results. The sample size of the trial was also small, which limits the ability to test for dose effects, and reduced overall power to detect changes in ERP or resting state activity. Despite this, the pilot study identifies EEG markers of activity that are both indicative of treatment effect and relate to a change in depressive symptoms.
Conclusion
In this pilot study we show that 12 weeks of transdermal nicotine treatment can enhance event-related oscillations associate with auditory attention in adults with LLD. Moreover, this change in cortical activity was related to the magnitude of improvement in mood symptoms over the course of the nicotine treatment, which highlights the potential use of EEG marker for predicting treatment response in adults with LLD. Future studies should aim to confirm this finding in larger, placebo-controlled trials which would be able to conclusively show the efficacy of an investigational treatment.
Data Availability Statement
The raw data supporting the conclusions of this article will be made available by the authors, without undue reservation.
Ethics Statement
The studies involving human participants were reviewed and approved by Vanderbilt University Medical Center Institutional Review Board. The patients/participants provided their written informed consent to participate in this study.
Author Contributions
AC contributed to the design of the experiment, processing of EEG data, and the design and preparation of the manuscript. AK contributed to the design of the procedures and the preparation of the manuscript. WT contributed to the design of the experiment and analyses, data collection and processing, and preparation of the manuscript. KA, BB, and JV contributed to the design of the preparation of the manuscript. PN contributed to the design of the experiment and analyses and the preparation of the manuscript. All authors contributed to the article and approved the submitted version.
Funding
This research was supported by NIH Grant K24 MH110598 and CTSA Award UL1TR000445 from the National Center for Advancing Translational Sciences.
Conflict of Interest
The authors declare that the research was conducted in the absence of any commercial or financial relationships that could be construed as a potential conflict of interest.
Publisher's Note
All claims expressed in this article are solely those of the authors and do not necessarily represent those of their affiliated organizations, or those of the publisher, the editors and the reviewers. Any product that may be evaluated in this article, or claim that may be made by its manufacturer, is not guaranteed or endorsed by the publisher.
Acknowledgments
We thank Katherine Anders, Ashley Antal, and Jason Gandelman for their assistance with data collection.
References
1. Taylor WD. Clinical practice. Depression in the elderly. N Engl J Med. (2014) 371:1228–36. doi: 10.1056/NEJMcp1402180
2. Sheline YI, Barch DM, Garcia K, Gersing K, Pieper C, Welsh-Bohmer K, et al. Cognitive function in late life depression: relationships to depression severity, cerebrovascular risk factors and processing speed. Biol Psychiatry. (2006) 60:58–65. doi: 10.1016/j.biopsych.2005.09.019
3. Nelson JC, Delucchi K, Schneider LS. Efficacy of second generation antidepressants in late-life depression: a meta-analysis of the evidence. Am J Geriatr Psychiatry. (2008) 16:558–67. doi: 10.1097/01.JGP.0000308883.64832.ed
4. Gutsmiedl K, Krause M, Bighelli I, Schneider-Thoma J, Leucht S. How well do elderly patients with major depressive disorder respond to antidepressants: a systematic review and single-group meta-analysis. BMC Psychiatry. (2020) 20:1–12. doi: 10.1186/s12888-020-02514-2
5. Alexopoulos GS, Kiosses DN, Murphy C, Heo M. Executive dysfunction, heart disease burden, and remission of geriatric depression. Neuropsychopharmacology. (2004) 29:2278–84. doi: 10.1038/sj.npp.1300557
6. Morimoto SS, Gunning FM, Murphy CF, Kanellopoulos D, Kelly RE, Alexopoulos GS. Executive function and short-term remission of geriatric depression: the role of semantic strategy. Am J Geriatr Psychiatry. (2011) 19:115–22. doi: 10.1097/JGP.0b013e3181e751c4
7. Sheline YI, Pieper CF, Barch DM, Welsh-Boehmer K, Mckinstry RC, Macfall JR, et al. Error in byline and author affiliations in: support for the vascular depression hypothesis in late-life depression: results of a 2-site, prospective, antidepressant treatment trial. Arch Gen Psychiatry. (2010) 67:1043. doi: 10.1001/archgenpsychiatry.2010.106
8. Köhler S, Ashton CH, Marsh R, Thomas AJ, Barnett NA, O'Brien JT. Electrophysiological changes in late life depression and their relation to structural brain changes. Int Psychogeriatr. (2011) 23:141–8. doi: 10.1017/S1041610210001250
9. Zhang BW, Xu J, Chang Y. The effect of aging in inhibitory control of major depressive disorder revealed by event-related potentials. Front Hum Neurosci. (2016) 10:1–10. doi: 10.3389/fnhum.2016.00116
10. Dahabra S, Ashton CH, Bahrainian M, Britton PG, Ferrier IN, McAllister VA, et al. Structural and functional abnormalities in elderly patients clinically recovered from early- and late-onset depression. Biol Psychiatry. (1998) 44:34–46. doi: 10.1016/S0006-3223(98)00003-1
11. Olbrich S, Arns M. EEG biomarkers in major depressive disorder: discriminative power and prediction of treatment response. Int Rev Psychiatry. (2013) 25:604–18. doi: 10.3109/09540261.2013.816269
12. Alhaj H, Wisniewski G, McAllister-Williams RH. The use of the EEG in measuring therapeutic drug action: focus on depression and antidepressants. J Psychopharmacol (Oxf). (2011) 25:1175–91. doi: 10.1177/0269881110388323
13. Knott V, Mahoney C, Kennedy S, Evans Evans K, EEG correlates of acute and chronic paroxetine treatment in depression. J Affect Disord. (2002) 69:241–9. doi: 10.1016/S0165-0327(01)00308-1
14. Spronk D, Arns M, Barnett KJ, Cooper NJ, Gordon E. An investigation of EEG, genetic and cognitive markers of treatment response to antidepressant medication in patients with major depressive disorder: a pilot study. J Affect Disord. (2011) 128:41–8. doi: 10.1016/j.jad.2010.06.021
15. Gandelman JA, Newhouse P, Taylor WD. Nicotine and networks: potential for enhancement of mood and cognition in late-life depression. Neurosci Biobehav Rev. (2018) 84:289–98. doi: 10.1016/j.neubiorev.2017.08.018
16. Picciotto MR, Brunzell DH, Caldarone BJ. Effect of nicotine and nicotinic receptors on anxiety and depression. Neuroreport. (2002) 13:1097–106. doi: 10.1097/00001756-200207020-00006
17. Mineur YS, Picciotto MR. Nicotine receptors and depression: revisiting and revising the cholinergic hypothesis. Trends Pharmacol Sci. (2010) 12:580–6. doi: 10.1016/j.tips.2010.09.004
18. Tizabi Y, Overstreet DH, Rezvani AH, Louis VA, Clark E, Janowsky DS, et al. Antidepressant effects of nicotine in an animal model of depression. Psychopharmacology (Berl). (1999) 142:193–9. doi: 10.1007/s002130050879
19. McClernon FJ, Hiott FB, Westman EC, Rose JE, Levin ED. Transdermal nicotine attenuates depression symptoms in nonsmokers: a double-blind, placebo-controlled trial. Psychopharmacology (Berl). (2006) 189:125–33. doi: 10.1007/s00213-006-0516-y
20. Levin ED, Conners CK, Silva D, Hinton SC, Meck WH, March J, et al. Transdermal nicotine effects on attention. Psychopharmacol Berl. (1998) 140:135–41. doi: 10.1007/s002130050750
21. Lawrence NS, Ross TJ, Stein EA. Cognitive mechanisms of nicotine on visual attention. Neuron. (2002) 36:539–48. doi: 10.1016/S0896-6273(02)01004-8
22. Newhouse P, Kellar K, Aisen P, White H, Wesnes K, Coderre E, et al. Nicotine treatment of mild cognitive impairment: a 6-month double-blind pilot clinical trial. Neurology. (2012) 78:91–101. doi: 10.1212/WNL.0b013e31823efcbb
23. Kadoya C, Domino EF, Matsuoka S. Relationship of electroencephalographic and cardiovascular changes to plasma nicotine levels in tobacco smokers. Clin Pharmacol Ther. (1994) 55:370–7. doi: 10.1038/clpt.1994.44
24. Lindgren M, Molander L, Verbaan C, Lunell E, Rosén I. Electroencephalographic effects of intravenous nicotine - a dose- response study. Psychopharmacology (Berl). (1999) 145:342–50. doi: 10.1007/s002130051067
25. Knott V, Bosman M, Mahoney C, Ilivitsky V, Quirt K. Transdermal nicotine: single dose effects on mood, EEG, performance, and event-related potentials. Pharmacol Biochem Behav. (1999) 63:253–61. doi: 10.1016/S0091-3057(99)00006-4
26. Griesar WS, Zajdel DP, Oken BS. Nicotine effects on alertness and spatial attention in non-smokers. Nicotine Tob Res. (2002) 4:185–94. doi: 10.1080/14622200210123617
27. Knott V, Heenan A, Shah D, Bolton K, Fisher D, Villeneuve C. Electrophysiological evidence of nicotines distracter-filtering properties in non-smokers. J Psychopharmacol (Oxf). (2011) 25:239–48. doi: 10.1177/0269881109348158
28. Knott V, Choueiry J, Dort H, Smith D, Impey D, De La Salle S, et al. Baseline-dependent modulating effects of nicotine on voluntary and involuntary attention measured with brain event-related P3 potentials. Pharmacol Biochem Behav. (2014) 122:107–17. doi: 10.1016/j.pbb.2014.03.020
29. Jaworska N, McIntosh J, Villeneuve C, Thompson A, Fishe D, Milin R, et al. Effects of nicotine on electroencephalography and affect in adolescent females with major depressive disorder: a pilot study. J Addict Med. (2011) 5:123–33. doi: 10.1097/ADM.0b013e3181e2f10f
30. Gandelman JA, Kang H, Antal A, Albert K, Boyd BD, Conley AC, et al. Transdermal nicotine for the treatment of mood and cognitive symptoms in nonsmokers with late-life depression. J Clin Psychiatry. (2018) 79:137. doi: 10.4088/JCP.18m12137
31. Montgomery SA, Asberg M. A new depression scale designed to be sensitive to change. Br J Psychiatry. (1979) 134:382–9. doi: 10.1192/bjp.134.4.382
32. Nasreddine ZS, Phillips NA, Bédirian V, Charbonneau S, Whitehead V, Collin I, et al. The montreal cognitive assessment, MoCA: a brief screening tool for mild cognitive impairment. J Am Geriatr Soc. (2005) 53:695–9. doi: 10.1111/j.1532-5415.2005.53221.x
33. Saykin AJ, Wishart HA, Rabin LA, Santulli RB, Flashman LA, West JD, et al. Older adults with cognitive complaints show brain atrophy similar to that of amnestic MCI. Neurology. (2006) 67:834–42. doi: 10.1212/01.wnl.0000234032.77541.a2
34. Saffer BY, Lanting SC, Koehle MS, Klonsky ED, Iverson GL. Assessing cognitive impairment using PROMIS® applied cognition-abilities scales in a medical outpatient sample. Psychiatry Res. (2015) 226:169–72. doi: 10.1016/j.psychres.2014.12.043
35. Morisky DE, Green LW, Levine DM. Concurrent and predictive validity of a self-reported measure of medication adherence. Med Care. (1986) 24:67–74. doi: 10.1097/00005650-198601000-00007
36. Key AP, Dykens EM. Event-related potential index of age-related differences in memory processes in adults with down syndrome. Neurobiol Aging. (2014) 35:247–53. doi: 10.1016/j.neurobiolaging.2013.07.024
37. Srinivasan R, Nunez PL, Tucker DM, Silberstein RB, Cadusch PJ. Spatial sampling and filtering of EEG with spline laplacians to estimate cortical potentials. Brain Topogr. (1996) 8:355–66. doi: 10.1007/BF01186911
38. Hindmarch I. Psychological performance models as indicators of the effects of hypnotic drugs on sleep. Psychopharmacology Suppl. (1984) 1:58–68. doi: 10.1007/978-3-642-69659-6_4
39. Comerchero MD, Polich J. P3a and P3b from typical auditory and visual stimuli. Clin Neurophysiol. (1999) 110:24–30. doi: 10.1016/S0168-5597(98)00033-1
40. Polich J. Updating P300: an integrative theory of P3a and P3b. Clin Neurophysiol. (2007) 118:2128–48. doi: 10.1016/j.clinph.2007.04.019
41. Oostenveld R, Fries P, Maris E, Schoffelen JM. FieldTrip: open source software for advanced analysis of MEG, EEG, and invasive electrophysiological data. Comput Intell Neurosci. (2011) 2011:156869. doi: 10.1155/2011/156869
42. Delorme A, Makeig S. EEGLAB: an open source toolbox for analysis of single-trial EEG dynamics including independent component analysis. J Neurosci Methods. (2004) 134:9–21. doi: 10.1016/j.jneumeth.2003.10.009
43. Kayser J, Tenke CE. Principal components analysis of Laplacian waveforms as a generic method for identifying ERP generator patterns: II. Adequacy of low-density estimates. Clin Neurophysiol. (2006) 117:369–80. doi: 10.1016/j.clinph.2005.08.033
44. Hyvärinen A, Oja E. Independent component analysis: algorithms and applications. Neural Netw. (2000) 13:411–30. doi: 10.1002/0471221317
45. Kayser J, Tenke CE. Issues and considerations for using the scalp surface Laplacian in EEG / ERP research: a tutorial review. Int J Psychophysiol. (2015) 97:189–209. doi: 10.1016/j.ijpsycho.2015.04.012
46. Cooper PS, Wong ASW, Fulham WR, Thienel R, Mansfield E, Michie PT, et al. Theta frontoparietal connectivity associated with proactive and reactive cognitive control processes. Neuroimage. (2015) 108:354–63. doi: 10.1016/j.neuroimage.2014.12.028
47. Cooper PS, Darriba Á, Karayanidis F, Barceló F. Contextually sensitive power changes across multiple frequency bands underpin cognitive control. NeuroImage. (2016) 132:499–511. doi: 10.1016/j.neuroimage.2016.03.010
48. Luu P, Ferree T. Determination of the Geodesic Sensor Nets' Average Electrode Positions and Their 10 – 10 International Equivalents. Eugene, OR: Electronic Geodesics Inc. (2005).
49. Benjamini Y, Yekutieli D. The control of the false discovery rate in multiple testing under dependency. Ann Stat. (2001) 29:1165–88. doi: 10.1214/aos/1013699998
50. The Jamovi Project. Jamovi. (Version 1.2) [Computer Software] (2021). Retrieved from https://www.jamovi.org
51. Gola M, Magnuski M, Szumska I, Wróbel A. EEG beta band activity is related to attention and attentional deficits in the visual performance of elderly subjects. Int J Psychophysiol. (2013) 89:334–41. doi: 10.1016/j.ijpsycho.2013.05.007
52. Newhouse PA, Potter A, Singh A. Effects of nicotinic stimulation on cognitive performance. Curr Opin Pharmacol. (2004) 4:36–46. doi: 10.1016/j.coph.2003.11.001
53. Deng Y, Reinhart RMG, Choi I, Shinn-Cunningham B. Causal links between parietal alpha activity and spatial auditory attention. eLife. (2019) 8:1184. doi: 10.7554/eLife.51184
54. Klimesch W, Sauseng P, Hanslmayr S. EEG alpha oscillations: the inhibition – timing hypothesis. Brain res rev. (2007) 53:63–88.
55. Klimesch W. Controlled access to stored information. Trends Cogn Sci. (2012) 16:606–17. doi: 10.1016/j.tics.2012.10.007
56. Barwick F, Arnett P, Slobounov S. EEG correlates of fatigue during administration of a neuropsychological test battery. Clin Neurophysiol. (2012) 123:278–84. doi: 10.1016/j.clinph.2011.06.027
57. Kalauzi A, Vuckovic A, Bojić T. EEG alpha phase shifts during transition from wakefulness to drowsiness. Int J Psychophysiol. (2012) 86:195–205. doi: 10.1016/j.ijpsycho.2012.04.012
58. Fodor Z, Marosi C, Tombor L, Csukly G. Salient distractors open the door of perception: alpha desynchronization marks sensory gating in a working memory task. Sci Rep. (2020) 10:19179. doi: 10.1038/s41598-020-76190-3
59. Hanslmayr S, Gross J, Klimesch W, Shapiro KL. The role of alpha oscillations in temporal attention. Brain Res Rev. (2011) 67:331–43. doi: 10.1016/j.brainresrev.2011.04.002
60. Frey JN, Mainy N, Lachaux JP, Muller N, Bertrand O, Weisz N. Selective modulation of auditory cortical alpha activity in an audiovisual spatial attention task. J Neurosci. (2014) 34:6634–9. doi: 10.1523/JNEUROSCI.4813-13.2014
61. Weisz N, Hartmann T, Müller N, Lorenz I, Obleser J. Alpha rhythms in audition: cognitive and clinical perspectives. Front Psychol. (2011) 2:e00073. doi: 10.3389/fpsyg.2011.00073
62. Scally B, Burke MR, Bunce D, Delvenne JF. Resting-state EEG power and connectivity are associated with alpha peak frequency slowing in healthy aging. Neurobiol Aging. (2018) 71:149–55. doi: 10.1016/j.neurobiolaging.2018.07.004
63. Garcés P, Vicente R, Wibral M, Pineda-Pardo JÁ, López ME, Aurtenetxe S, et al. Brain-wide slowing of spontaneous alpha rhythms in mild cognitive impairment. Front Aging Neurosci. (2013) 5:e00100. doi: 10.3389/fnagi.2013.00100
64. Keller AS, Leikauf JE, Holt-Gosselin B, Staveland BR, Williams LM. Paying attention to attention in depression. Transl Psychiatry. (2019) 9:1–12. doi: 10.1038/s41398-019-0616-1
65. Nilsson J, Thomas AJ, Stevens LH, McAllister-Williams RH, Ferrier IN, Gallagher P. The interrelationship between attentional and executive deficits in major depressive disorder. Acta Psychiatr Scand. (2016) 134:73–82. doi: 10.1111/acps.12570
66. Grahek I, Shenhav A, Musslick S, Krebs RM, Koster EHW. Motivation and cognitive control in depression. Neurosci Biobehav Rev. (2019) 102:371–81. doi: 10.1016/j.neubiorev.2019.04.011
67. Huang-Pollock CL, Nigg JT, Halperin JM. Single dissociation findings of ADHD deficits in vigilance but not anterior or posterior attention systems. Neuropsychology. (2006) 20:420–9. doi: 10.1037/0894-4105.20.4.420
68. Sohlberg MM, Mateer CA. Improving attention and managing attentional problems. Ann N Y Acad Sci. (2001) 931:359–75. doi: 10.1111/j.1749-6632.2001.tb05790.x
69. Olvet DM, Klein DN, Hajcak G. Depression symptom severity and error-related brain activity. Psychiatry Res. (2010) 179:30–7. doi: 10.1016/j.psychres.2010.06.008
70. Schmidt GJ, Barbosa AO, de Assis SG, Nicaretta DH, Schmidt SL. Attentional subdomains' deficits in Brazilian patients with major depressive episodes. Neuropsychology. (2021) 35:232–40. doi: 10.1037/neu0000719
71. Kemp Andrew H, Hopkinson PJ, Hermens DF, Rowe DL, Sumich AL, Clark CR, et al. Fronto-temporal alterations within the first 200 ms during an attentional task distinguish major depression, non-clinical participants with depressed mood and healthy controls: a potential biomarker? Hum Brain Mapp. (2008) 30:602–14. doi: 10.1002/hbm.20528
72. Maalouf FT, Klein C, Clark L, Sahakian BJ, LaBarbara EJ, Versace A, et al. Impaired sustained attention and executive dysfunction: bipolar disorder versus depression-specific markers of affective disorders. Neuropsychologia. (2010) 48:1862–8. doi: 10.1016/j.neuropsychologia.2010.02.015
73. Goschke T, Bolte A. Emotional modulation of control dilemmas: the role of positive affect, reward, and dopamine in cognitive stability and flexibility. Neuropsychologia. (2014) 62:403–23. doi: 10.1016/j.neuropsychologia.2014.07.015
74. Mitchell RLC, Phillips LH. The psychological, neurochemical and functional neuroanatomical mediators of the effects of positive and negative mood on executive functions. Neuropsychologia. (2007) 45:617–29. doi: 10.1016/j.neuropsychologia.2006.06.030
75. Storbeck J, Clore GL. Affective arousal as information: how affective arousal influences judgments, learning, and memory. Soc Personal Psychol Compass. (2008) 2:1824–43. doi: 10.1111/j.1751-9004.2008.00138.x
76. Campillo E, Ricarte JJ, Ros L, Nieto M, Latorre JM. Effects of the visual and auditory components of a brief mindfulness intervention on mood state and on visual and auditory attention and memory task performance. Curr Psychol. (2018) 37:357–65. doi: 10.1007/s12144-016-9519-y
77. Putkinen V, Makkonen T, Eerola T. Music-induced positive mood broadens the scope of auditory attention. Soc Cogn Affect Neurosci. (2017) 12:1159–68. doi: 10.1093/scan/nsx038
78. Ansado J, Monchi O, Ennabil N, Faure S, Joanette Y. Load-dependent posterior–anterior shift in aging in complex visual selective attention situations. Brain Res. (2012) 1454:14–22. doi: 10.1016/j.brainres.2012.02.061
79. Zhang H, Lee A, Qiu A. A posterior-to-anterior shift of brain functional dynamics in aging. Brain Struct Funct. (2017) 222:3665–76. doi: 10.1007/s00429-017-1425-z
80. Ishii R, Canuet L, Kurimoto R, Ikezawa K, Aoki Y, Azechi M, et al. Frontal shift of posterior alpha activity is correlated with cognitive impairment in early Alzheimer's disease: a magnetoencephalography–beamformer study. Psychogeriatrics. (2010) 10:138–43. doi: 10.1111/j.1479-8301.2010.00326.x
81. McCarthy P, Benuskova L, Franz E. The age-related posterior-anterior shift as revealed by voxelwise analysis of functional brain networks. Front Aging Neurosci. (2014) 6:301. doi: 10.3389/fnagi.2014.00301
82. Abi Zeid Daou M, Boyd BD, Donahue MJ, Albert K, Taylor WD. Anterior-posterior gradient differences in lobar and cingulate cortex cerebral blood flow in late-life depression. J Psychiatr Res. (2018) 97:1–7. doi: 10.1016/j.jpsychires.2017.11.005
83. Kaiser RH, Andrews-Hanna JR, Wager TD, Pizzagalli DA. Large-scale network dysfunction in major depressive disorder: a meta-analysis of resting-state functional connectivity. JAMA Psychiatry. (2015) 72:603–11. doi: 10.1001/jamapsychiatry.2015.0071
84. Behrmann M, Geng JJ, Shomstein S. Parietal cortex and attention. Curr Opin Neurobiol. (2004) 14:212–7. doi: 10.1016/j.conb.2004.03.012
85. Heinen K, Feredoes E, Ruff CC, Driver J. Functional connectivity between prefrontal and parietal cortex drives visuo-spatial attention shifts. Neuropsychologia. (2017) 99:81–91. doi: 10.1016/j.neuropsychologia.2017.02.024
86. Shomstein S, Yantis S. Parietal cortex mediates voluntary control of spatial and nonspatial auditory attention. J Neurosci. (2006) 26:435–9. doi: 10.1523/JNEUROSCI.4408-05.2006
87. Reuter E-M, Voelcker-Rehage C, Vieluf S, Winneke AH, Godde B. A parietal-to-frontal shift in the P300 is associated with compensation of tactile discrimination deficits in late middle-aged adults. Psychophysiology. (2013) 50:583–93. doi: 10.1111/psyp.12037
88. Alperin BR, Mott KK, Holcomb PJ, Daffner KR. Does the age-related “anterior shift” of the P3 reflect an inability to habituate the novelty response? Neurosci Lett. (2014) 577:6–10. doi: 10.1016/j.neulet.2014.05.049
89. Tenke CE, Kayser J, Shankman SA, Griggs CB, Leite P, Stewart JW, et al. Hemispatial PCA dissociates temporal from parietal ERP generator patterns: CSD components in healthy adults and depressed patients during a dichotic oddball task. Int J Psychophysiol. (2008) 67:1–16. doi: 10.1016/j.ijpsycho.2007.09.001
90. Keller AS, Ball TM, Williams LM. Deep phenotyping of attention impairments and the ‘Inattention Biotype' in Major Depressive Disorder. Psychol Med. (2020) 50:2203–12. doi: 10.1017/S0033291719002290
91. Zweerings J, Zvyagintsev M, Turetsky BI, Klasen M, König AA, Roecher E, et al. Fronto-parietal and temporal brain dysfunction in depression: a fMRI investigation of auditory mismatch processing. Hum Brain Mapp. (2019) 40:3657–68. doi: 10.1002/hbm.24623
92. Bruder GE, Stewart JW, McGrath PJ. Right brain, left brain in depressive disorders: clinical and theoretical implications of behavioral, electrophysiological and neuroimaging findings. Neurosci Biobehav Rev. (2017) 78:178–91. doi: 10.1016/j.neubiorev.2017.04.021
Keywords: nicotine, EEG, late-life depression, beta power, event-related oscillations
Citation: Conley AC, Key AP, Taylor WD, Albert KM, Boyd BD, Vega JN and Newhouse PA (2021) EEG as a Functional Marker of Nicotine Activity: Evidence From a Pilot Study of Adults With Late-Life Depression. Front. Psychiatry 12:721874. doi: 10.3389/fpsyt.2021.721874
Received: 15 June 2021; Accepted: 15 November 2021;
Published: 23 December 2021.
Edited by:
Wenbin Guo, Central South University, ChinaReviewed by:
Philip Rupert Baldwin, Baylor College of Medicine, United StatesRamcés Falfán-Valencia, Instituto Nacional de Enfermedades Respiratorias-México (INER), Mexico
Sergio Luis Schmidt, Rio de Janeiro State Federal University, Brazil
Copyright © 2021 Conley, Key, Taylor, Albert, Boyd, Vega and Newhouse. This is an open-access article distributed under the terms of the Creative Commons Attribution License (CC BY). The use, distribution or reproduction in other forums is permitted, provided the original author(s) and the copyright owner(s) are credited and that the original publication in this journal is cited, in accordance with accepted academic practice. No use, distribution or reproduction is permitted which does not comply with these terms.
*Correspondence: Paul A. Newhouse, cGF1bC5uZXdob3VzZUB2YW5kZXJiaWx0LmVkdQ==