- 1Department of Neuroscience and Psychiatry, Uppsala University Hospital, Uppsala, Sweden
- 2Theoretical Neuroscience Group, Wigner Research Centre for Physics, Hungarian Academy of Sciences, Budapest, Hungary
- 3Agora for Biosystems, Sigtuna Foundation, Sigtuna, Sweden
Obsessive compulsive disorder (OCD) can manifest as a debilitating disease with high degrees of co-morbidity as well as clinical and etiological heterogenity. However, the underlying pathophysiology is not clearly understood. Computational psychiatry is an emerging field in which behavior and its neural correlates are quantitatively analyzed and computational models are developed to improve understanding of disorders by comparing model predictions to observations. The aim is to more precisely understand psychiatric illnesses. Such computational and theoretical approaches may also enable more personalized treatments. Yet, these methodological approaches are not self-evident for clinicians with a traditional medical background. In this mini-review, we summarize a selection of computational OCD models and computational analysis frameworks, while also considering the model predictions from a perspective of possible personalized treatment. The reviewed computational approaches used dynamical systems frameworks or machine learning methods for modeling, analyzing and classifying patient data. Bayesian interpretations of probability for model selection were also included. The computational dissection of the underlying pathology is expected to narrow the explanatory gap between the phenomenological nosology and the neuropathophysiological background of this heterogeneous disorder. It may also contribute to develop biologically grounded and more informed dimensional taxonomies of psychopathology.
1. Introduction
In this review, we assessed the evolution of the computational modeling efforts that aim to study some aspects of obsessive compulsive disorder (OCD) pathophysiology. The computational and theoretical investigations support the move from the currently used nosological classification toward trans-dimensional approaches (1). This trend is motivated by a necessity to gain a deeper and more biologically grounded understanding of the disease in order to develop personalized interventions. A more precisely defined micro-behavioral analysis is often able to leverage specific and more objective biomarkers than the currently used phenomenological observations in diagnostic procedures. We reviewed computational models which utilize non-linear differential equation systems, where some aspects of the pathological neural network dynamics can be represented by perturbation of the dynamical systems (2–7). Some supervised and unsupervised machine learning (ML) methods were integrated in the review, which are utilized for classification (8–13). A plethora of reinforcement learning (RL) articles and several diverse computational analysis studies are also reviewed (14–18). Both model-based and model-free RL are utilized to examine pathological aspects of goal-directed and habitual systems in OCD. Under certain circumstances, one approach may have more explanatory power than the other. However, the gap of this dichotomic separation between model-free and model-based learning approaches is perhaps narrower than suggested in earlier studies. Some recent investigations point toward more integrated forms of RL, which can exploit richer representations and can be utilized to better explain certain aspects of OCD pathology. In this review, we selected and integrated articles that utilized data-driven approaches, for example, to predict clinical outcomes or responses to treatment, as well as theory-driven attempts where the altered information processing is modeled as the cause of psychiatric symptoms at the behavioral and neuronal level (19). Table 1 summarizes the reviewed modeling/computational articles. Figure 1 is a schematic representation of some of the brain regions, which were included in the current review.
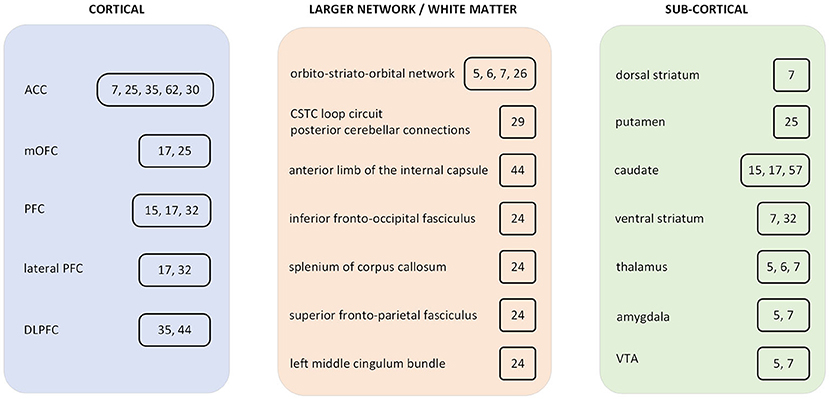
Figure 1. Summary diagram of brain regions which were included in obsessive compulsive disorder (OCD) computational studies. The included studies are cited in a box next to the brain region. ACC, anterior cingulate cortex; PFC, prefrontal cortex; mOFC, medial orbitofrontal cortex; DLPFC, dorsolateral prefrontal cortex; CSTC, cortico-striatal-thalamic-cortical; VTA, ventral tegmental area.
2. Dynamical Systems Approach
Several computational approaches utilizing dynamical systems have been developed, which can provide mechanistic insights about pathological neural dynamics in OCD. In these modeling frameworks, coupled non-linear differential equation systems were manipulated and perturbed. The solutions of these non-linear dynamical systems can exhibit a steep attractor state (e.g., fixed-point attractor), which can mimic states of perseveration, obsessions, and compulsions (2, 3, 46). Rumination or recurring chains of thought and stereotypical movement patterns were also modeled with non-linear differential equations where the solution of the dynamical system results in heteroclinic chains of meta-stable clusters and possible sequential chains of attractor basins (20, 21). Maia et al. (54) gave a comprehensive review on the neuropathological correlates and etiology of childhood and adult OCD. Verduzco-Flores and colleagues described their differential equation system as a model of working memory with increased stability of states or sequences, implicated to be associated with OCD (4). As a reflection on Verduzco-Flores' work, Maia pointed out that reduced inhibition does not map well to any known disturbance in OCD. However, what perhaps matters in the model is the balance between excitation and inhibition. Thus, the same pathological dynamics should occur with increased excitation and that would be consistent with evidence of glutamatergic hyperactivity (47, 55).
Other computational studies found that changes in the excitatory and inhibitory balance pushes a cortico-striatal-thalamo-cortical (CSTC) pathway to states of generalized hyper-activity. Certain changes in global E/I and specifically in the local inhibition may trigger network oscillations and generate hyper-activity throughout the entire CSTC pathway in OCD (5, 6). This framework was further developed and analyzed by taking into account the functional and structural network changes of the CSTC circuit in the schizo-obsessive population (7). The study predicted the importance of pathological activity propagation between the ventral and dorsal striatum, and highlighted other disruptive mechanisms in the CSTC pathway which could result in pathological repetitive behavior in this heterogeneous population.
3. Supervised and Unsupervised ML Approaches
Several computational studies utilizing ML techniques investigated certain aspects of neuropathophysiology and symptom phenomenology by analyzing and classifying OCD patient data. We review some of them.
A study using Random Forest decision trees found that clinically useful predictions of remission may not require an extensive battery of measures. A small set of assessments may efficiently distinguish between higher and lower risk OCD patients to inform clinical decision-making (9). Relevant predictors of suicide attempts by OCD patients were examined with Elastic net regression, a linear combination of Lasso and Ridge methods. Previous suicide planning, previous suicide thoughts, lifetime depressive episodes, and intermittent explosive disorder symptoms were found to be relevant predictors (10). Applying Support Vector Regression (SVR) identified gray matter volumes in the cortical-subcortical loops to predict OCD symptom severity. The left medial orbitofrontal cortex (OFC) and the left putamen gray matter volume were identified as neurobiological markers. The same study demonstrated that the best predictors of the “sexual/religious” OCD dimensions were the left medial OFC, right lateral OFC, and left anterior cingulate cortex (ACC) (25). Four different ML algorithms performed well as compared to multivariate logistic regression, in the prediction of treatment response to Internet-delivered cognitive behavior therapy (ICBT) for pediatric OCD treatment. The methods used were a linear model with best subset predictor selection, Elastic net (Lasso only), Random Forest, and Support Vector Machine (SVM) (23). In another integrative study, SVM and naïve Bayes methods identified predictors of diagnostic outcomes in patients with early onset OCD (12).
To identify brain regions relevant for OCD diagnosis, bagged linear SVMs were applied to structural MRI (sMRI) data for discrimination across 86 OCD patients and 86 control subjects. 39 brain regions were identified showing the largest differences between OCD patients and healthy controls and 36 of those were located in the frontal, temporal, and parietal cortices or in subcortical structures (11). A multivariate SVM method was also applied to fractional anisotropy of white matter using diffusion tensor imaging (DTI) on 28 OCD patients and 28 healthy controls. Successful discrimination was based on bilateral prefrontal and temporal regions, the inferior fronto-occipital and superior fronto-parietal fasciculi, splenium of corpus callosum, and the left middle cingulum bundle (24).
OCD is a heterogeneous disorder with varied symptom presentations, each of which may relate to distinct neuropsychological features. Traditionally, this heterogeneity was approached by using a symptom-based evaluation, but an alternative can involve focusing on underlying symptom motivations (8). Note that 60–70% of OCD patients also can experience sensory phenomena, consisting of uncomfortable sensations or perceptions that may drive compulsions. Supervised ML methods (Random Forest, SVM, and K-nearest neighbor) were tested in one set to discriminate between OCD patients and healthy controls and another set to discriminate between OCD patients with sensory phenomena, without sensory phenomena and healthy controls. All three ML methods performed better than logistic or multimodal regression on the same datasets. Decision-making measurements best distinguished between groups based on sensory phenomena (8).
With unsupervised learning, a combination of Principle Component Analysis (PCA) and a K-means clustering algorithm was utilized to separate subgroups in the compulsive-impulsive dimensions. Clustering converged to yield four subgroups: low compulsivity–low impulsivity group; two groups showing roughly equal clinical severity, but with opposing dimensions (i.e., high compulsivity and low impulsivity, and vice versa); and the fourth with both high compulsivity and impulsivity and recording the highest clinical severity. The largest cluster of individuals with OCD was characterized by high impulsivity and low compulsivity (28). The identification of these subgroups might have potential implications for OCD treatment.
A recent study based on multi-level brain imaging and behavioral data from children using the Random Forest classification algorithm identified four new brain-behavior groups cutting across neurodevelopmental disorders such as autism spectrum disorder, OCD, and attention-deficit/hyperactivity disorder (13). It was demonstrated that children within these groups had more similar profiles on brain and behavioral measures than found among conventional diagnostic groupings (13).
4. Reinforcement Learning: Goal-Directed and Habitual Systems
Another class of models were developed to simulate goal-directed behavior, where OCD patients may have impairment. Deficits in goal-directed control implies vulnerability for developing rigid habits (16). These models are usually computationally formalized as a type of RL (56) and can be regarded formally as dynamical systems as well (46).
Model-based RL learns to represent the environment for goal-directed predictions and allows learning to guide actions most accurately, at the expense of high computational and energy costs. Model-free RL optimizes dynamics and heuristics for habit learning without external representations and it demands less computational and memory resources, but is inflexible and generalizes poorly (15).
In healthy cohorts, individual differences in model-based learning predicted sensitivity to outcome devaluation, suggesting that an associative mechanism underlies a bias toward habit formation. But no evidence was found of a causal relationship between model-free learning and devaluation sensitivity (15).
Most previous work focused on distinguishing between only two RL systems: model-based and model-free RL (14), as prototype extremes. Recent evidence shows that there are likely several parallel systems present in the brain, which are involved in OCD pathology and their dynamics is best captured by a mixture of RL algorithms (18, 53). It has been suggested that model-free learning might simply be an imperfect formalization of habit-learning (1). A review article proposed that inflexible reliance on habit in OCD may reflect a functional weakness in the mechanism for context-appropriate dynamic arbitration between model-free and model-based decision-making (52). Thus, re-consideration is needed about this model-free/model-based dichotomy. For example, it was found that model-free spatial-motor outcome-irrelevant learning generalized across distinct state features (31, 53). In a meta-study of juvenile OCD (53), subjects had difficulties in model-based complex decision-making and set shifting. However, unlike adults, there was only limited evidence for pathologies such as distorted habit formation.
Model-based (over model-free) strategies were found to be positively correlated with gray matter volume in the ventromedial prefrontal cortex (PFC) and caudate, regions that are critical for goal-directed control (15, 17). Dysfunctional caudate hyperactivity was shown in OCD patients when performing habits (15, 57). In a healthy population, ventral striatal presynaptic dopamine levels reflected a balance in behavioral and neural signatures of model-free and model-based control. Higher presynaptic dopamine levels were associated with stronger coding of model-based information in lateral PFC and diminished coding of model-free prediction errors in ventral striatum (32).
In adults, stimulant addiction and OCD were associated with a significant shift in habit formation and this abnormality can be quantified as model-free learning. Lower gray matter volumes in the caudate, medial OFC, and lateral prefrontal cortices were associated with a greater shift toward model-free habit formation (17).
5. Bayesian Approaches for OCD
A plethora of studies have built on the idea that the brain implements Bayesian inference. This can be formalized in a Bayesian state-space model that aims to infer the current state of the environment by combining prior knowledge and current evidence, weighting each by its relative uncertainty. With this, learning is governed by the balance between uncertainty on state transitions and observational uncertainty (36).
Some theoretical works analyzed the assumption that OCD patients have excessive uncertainty regarding state transitions. In this case, high transition uncertainty results in increased relative weighting of prediction errors. This could explain findings of increased responses to predictable stimuli. The increased weighting of prediction errors seems more likely to be the result of high transition uncertainty than underestimation of sensory noise. Increased weighting of prediction errors are related to perceiving the world as more unstable. Further, the above alterations could account for sensory over-responsiveness in OCD, as well as the experience of intrusive thoughts. Overweighting of sensory data often implies an impairment in processing, as it leads to a failure of the use of prior information and less attenuation of sensory noise. As a further consequence, this can manifest in patients' experiences that actions were not performed correctly, obsessional thoughts, compulsions, and sensory over-responsiveness (37, 39).
Severe cognitive flexibility impairments in OCD have been described in several studies (49), although other computational works and meta-analysis pointed out that inflexibility in OCD is controversial (36, 58). A decreased reliance on the past, excessive uncertainty and an assigned lower weight to prior experience has been shown to lead to over-exploratory behavior. Also, OCD patients require longer response times, higher decision boundaries and more evidence in perceptual contexts with high uncertainties (43). Somewhat counterintuitively, OCD symptoms correlated with over-flexibility in another set of computational studies (36). Excessive uncertainty and distrust of past experiences rather than perseveration were identified and these results might challenge pre-conceptions of OCD as a disorder of inflexibility (36).
In a combined experimental and computational study, it was shown that OCD patients develop an accurate internal model of the environment but they use it less to guide behavior. This suggests a cognitive architecture that separately interprets the environment independently of performance (39). Different memory systems separately influence repeated decisions. In a study of perceptual dot motion decisions, Solway et al. found that both the actual choice made during the first decision episode as implicit memory and the choice people explicitly remember making influenced the subsequent decision. Transfers specifically driven by implicit memory were reduced in individuals with higher levels of OCD symptoms (41). Verbal recognition memory was also investigated as a function of OCD symptoms, using a drift-diffusion model selected with model evidence using a multi-level Bayesian framework (42). It was found that discriminability defined as how well one is able to tell the old vs. new stimuli apart was reduced as a function of OCD symptoms, and that the degree of impairment was larger for easier recognition decisions (42).
6. Additional Computational Analysis of Neuropathological Correlates
Enhanced activation in the fronto-cingulate system in OCD patients and task-related modulation of effective connectivity from the dorsal anterior cingulate cortex (ACC) to left dorsolateral PFC was demonstrated by using dynamical causal modeling (DCM) on patient fMRI data. These findings implicated an overactive error control system in OCD (35). Another method was utilized to characterize patients with OCD based on resting-state fMRI. The Riemann kernel PCA method extracted features from functional connectivity matrices and demonstrated stronger connections between basal ganglia and cortex and weaker cerebellum-related connections in OCD (29).
6.1. Insight
Patient insight in OCD is crucial. The diagnostic status of poor insight is ambiguous but is a key clinical factor that influences therapy outcome (59). Poor insight has been associated with earlier age-at-onset, longer duration of illness, and a more chronic course of OCD (60). Checking-related uncertainty was correlated with the level of insight in OCD patients (61). Information gathering was found to be related to indecisiveness, but not symptom severity in OCD (38). This absence of a correlation with symptom severity was implicated to be caused by an imprecise estimate of the OCD severity, which was related to a lack of insight in juvenile OCD (38).
OCD patients with good and poor insight (OCD-GI and OCD-PI) have partly distinct brain structural alterations (62). OCD-PI patients have decreased cortical thickness in the left superior frontal gyrus, left anterior ACC, and right inferior parietal gyrus, compared to both OCD-GI and healthy controls (62). It was also indicated that the OCD-GI group had significantly increased functional connectivity between the right anterior insula (AI) ← → left dorsal anterior cingular cortex (dACC) than healthy controls (63). The connectivity alterations between the AI ← → OFC and AI ← → ACC may be important neural correlates of insight in OCD and even in schizophrenia (7). Alterations have been demonstrated at the theta (θ) EEG band in a small-world network framework and these changes existed only in the OCD-PI patients but not in the OCD-GI patients. Thus, poor insight OCD may be associated with disruptive functional integrity in the brain functional network in the theta band (45).
6.2. Co-morbidity and Trans-dimensional Analysis
Trans-dimensional biologically grounded approaches to OCD symptoms are supported by the obvious existence of OCD sub-types. A shift from a categorized disease framework to a dimensional one may enable more personalized treatment choices (16). We list some examples of such approaches.
RL models were able to capture certain behavioral microstructure differences between stimulant use disorder (SUD) and OCD. Stimulus-bound perseveration is a measure of how a subject is responding to a repeated stimulus, irrespective of outcome. This measure was found to be significantly increased in SUD, but decreased in OCD, compared to controls. Individuals with SUD exhibited reduced reward-driven learning, while both the SUD and OCD groups showed increased learning from punishment. Dopamine receptor D2/3 agonists and antagonists had similar effects on OCD groups, as both increased punishment-driven learning (33). In addition, a pharmacological fMRI study of RL has shown an abnormally increased signaling of prediction errors in the anterior ACC. This effect was reduced by both a D2/3 agonist and an antagonist (30). Modeling results did not demonstrate the same effects but did show a marginally significant reduction in prediction error learning rates in OCD patients (30).
Another aspect of behavioral microstructure was analyzed by 2-level factor modeling in OCD patients. This modeling study found that heterogeneous symptoms (as quantified, e.g., in Yale-Brown Obsessive Compulsive Scale) reflect four underlying symptom dimensions with deviations from previous results (40).
Obsessions and compulsions might independently contribute to the pathophysiology (1, 16). An alternative possibility posits that rather than goal-directed avoidance behaviors, compulsions derive from manifestations of excessive habit formation (50, 51), thus obsessive thoughts may develop as a result of compulsive behavior. It has even been suggested that the acronym OCD be rearranged to COD (50). Compulsivity and impulsivity might be only partially independent dimensions, considering that patients with substance abuse can transition from impulsivity to compulsivity (16). A “Compulsive Behavior and Intrusive Thought” dimension has been described as deficits in goal-directed control and presented in multiple psychiatric disorders such as OCD, addiction, and eating disorders (16).
The neuropathophysiology of co-morbid OCD and schizophrenia was examined in a phenomenological computational model (7). It was found that cortical self-inhibition alterations (e.g., SSRI treatment) together with dopaminergic input to the striatum (e.g., anti-dopaminergic medication) has non-trivial complex effects on the network oscillatory behavior, with an optimal modulatory window. Also, the modeling results predicted that as a consequence of over-compensation of the primary pathology, emergence of the other disorder might occur (7).
6.3. Personalized Computational Approaches
The clinical implications of certain computational results suggest possible development of personalized medicine to identify and optimize specific therapies for individual OCD patients. We list some of those efforts. Pre-treatment functional connectivity patterns within the default mode network and visual network significantly predicted the effect of cognitive behavioral therapy (CBT) and post-treatment OCD severity. These networks were stronger predictors than pre-treatment clinical scores (64). Abnormally strong cingulate signaling was measured using fMRI during reward processing with OCD patients. Bidirectional re-mediation by dopaminergic modulation suggests that exaggerated cingulate error signals in OCD may be of dopaminergic origin (30).
Transcranial magnetic stimulation (TMS) has shown promise as an adjunct treatment for the symptoms of OCD (48). Quantitative EEG was found to be helpful for predicting TMS treatment response for OCD patients. Using artificial neural network (ANN) classifiers with Particle Swarm Optimization (PSO) it was found that repetitive TMS responders had higher pre-treatment theta band power at all electrodes than did the non-responders (27).
Therapy refractory OCD patients have benefited from deep brain stimulation (DBS). Optimal therapeutic results are associated with the activation of distinct fiber pathways. The stimulation of the right anterior middle frontal gyrus (DLPFC) has shown a positive response. Focused stimulation of specific fiber pathways, which allows stimulation with lower amplitudes, may be superior to activation of a wide array of pathways, typically associated with higher stimulation amplitudes (44). Closed-loop neuromodulation is an emerging field in DBS. Model-based prediction was proposed for an optimal sub-thalamic nucleus (STN) DBS on treatment-refractory OCD with a combination of a stochastic dynamical model and microelectrode recording datasets (22).
A recent meta-analysis using a Bayesian hierarchical model framework examined adverse effects of selective serotonin reuptake inhibitors (SSRIs) and serotonin-norepinephrine reuptake inhibitors (SNRIs) treatments in pediatric OCD and anxiety disorders. It was found that compared with SNRIs, SSRIs are more likely to produce activation such as insomnia, irritability, hyperactivity, and impulsivity. The results suggested that although SSRIs are superior to SNRIs and the treatment of choice, for those patients who become activated on SSRIs, SNRIs might represent a good second choice given their reported efficacy and lower risk of activation (34).
7. Limitations
There are computational contributions that were not included in the current work. The contents of the obsessive-compulsive symptoms (sexual, religious, aggressive, contamination) were only partially explored. Computational work with brain histology was also excluded. We did not include comprehensive aspects of the developmental trajectories of the disease (age of onset, etc.). Further reviews are necessary to follow and categorize this rapidly growing field.
8. Conclusion
To summarize, we reviewed some of the computational modeling efforts which were developed to explain certain aspects of OCD pathophysiology and symptomology. These models span from mechanistic dynamical systems approaches, across ML techniques which aim to integrate and classify patient data (including supervised, unsupervised models, RL), to include Bayesian model selection frameworks. We related the modeling evidence and results to diagnostic procedures, co-morbid states, and therapeutical consequences. In conclusion, computational psychiatry has powerful methods, which can arm psychiatrists with more quantitative tools (46). Although it is challenging to move from a phenomenologically based thought process to a dynamical approach, we claim that a phase transition in understanding psychiatric disease as dynamical pathologies is inevitable. To this end, computational/theoretical frameworks have been synthesized to capture how OCD symptoms can be further analyzed from a trans-diagnostic and computational perspective (1).
Author Contributions
All authors listed have made a substantial, direct and intellectual contribution to the work, and approved it for publication.
Funding
KS was supported by ALF, RUFU, Märta and Nasvells Stiftelse research grants provided by the Uppsala University Hospital. Support for DS was provided by a joint grant from the John Templeton Foundation and the Fetzer Institute.
Author Disclaimer
The opinions expressed in this publication are those of the author(s) and do not necessarily reflect the views of the John Templeton Foundation or the Fetzer Institute.
Conflict of Interest
The authors declare that the research was conducted in the absence of any commercial or financial relationships that could be construed as a potential conflict of interest.
Publisher's Note
All claims expressed in this article are solely those of the authors and do not necessarily represent those of their affiliated organizations, or those of the publisher, the editors and the reviewers. Any product that may be evaluated in this article, or claim that may be made by its manufacturer, is not guaranteed or endorsed by the publisher.
Acknowledgments
We wish to thank the Theoretical Neuroscience Group of the Wigner Research Centre for Physics and the Centre for Integrated Research on Culture and Society (CIRCUS, Uppsala University): Anxiety, an Interdisciplinary Research Network (Grant No. 226301800).
References
1. Gillan G. Recent developments in the habit hypothesis of OCD and compulsive disorders. Curr Top Behav Neurosci. (2021) 49:147–67. doi: 10.1007/7854_2020_199
2. Rolls E, Loh M, Deco G. An attractor hypothesis of obsessive-compulsive disorder. Eur J Neurosci. (2008) 28:782–93. doi: 10.1111/j.1460-9568.2008.06379.x
3. Rolls E. Glutamate, obsessive-compulsive disorder, schizophrenia, and the stability of cortical attractor neuronal networks. Pharmacol Biochem Behav. (2012) 100:736–51. doi: 10.1016/j.pbb.2011.06.017
4. Verduzco-Flores S, Ermentrout B, Bodner M. Modeling neuropathologies as disruption of normal sequence generation in working memory networks. Neural Netw. (2012) 27:21–31. doi: 10.1016/j.neunet.2011.09.007
5. Rădulescu A, Marra R. A mathematical model of reward and executive circuitry in obsessive compulsive disorder. J Theor Biol. (2017) 414:165–75. doi: 10.1016/j.jtbi.2016.11.025
6. Rădulescu A, Herron J, Kennedy C, Scimemi A. Global and local excitation and inhibition shape the dynamics of the cortico-striatal-thalamo-cortical pathway. Sci Rep. (2017) 7:7608. doi: 10.1038/s41598-017-07527-8
7. Szalisznyó K, Silverstein D, Tóth J. Neural dynamics in co-morbid schizophrenia and OCD: A computational approach. J Theor Biol. (2019) 473:80–94. doi: 10.1016/j.jtbi.2019.01.038
8. Stamatis C, Batistuzzo M, Tanamatis T, Miguel E, Hoexter M, Timpano K. Using supervised machine learning on neuropsychological data to distinguish OCD patients with and without sensory phenomena from healthy controls. Br J Clin Psychol. (2021) 60:77–98. doi: 10.1111/bjc.12272
9. Askland K, Garnaat S, Sibrava N, Boisseau C, Strong D, Mancebo M, et al. Prediction of remission in obsessive compulsive disorder using a novel machine learning strategy. Int J Methods Psychiatr Res. (2015) 24:156–69. doi: 10.1002/mpr.1463
10. Agne N, Tisott C, Ballester P, Passos I, Ferrão Y. Predictors of suicide attempt in patients with obsessive-compulsive disorder: an exploratory study with machine learning analysis. Psychol Med. (2020) 1–11. doi: 10.1017/S0033291720002329
11. Parrado-Hernández E, Gómez-Verdejo V, Martínez-Ramón M, Shawe-Taylor J, Alonso P, Pujol J, et al. Discovering brain regions relevant to obsessive-compulsive disorder identification through bagging and transduction. Med Image Anal. (2014) 18:435–48. doi: 10.1016/j.media.2014.01.006
12. Mas S, Gassó P, Morer A, Calvo A, Bargalló N, Lafuente A, et al. Integrating genetic, neuropsychological and neuroimaging data to model early-onset obsessive compulsive disorder severity. PLoS ONE. (2016) 11:e0153846. doi: 10.1371/journal.pone.0153846
13. Jacobs G, Voineskos A, Hawco C, Stefanik L, Forde N, Dickie E, et al. Integration of brain and behavior measures for identification of data-driven groups cutting across children with ASD, ADHD, or OCD. Neuropsychopharmacology. (2021) 46:643–53. doi: 10.1038/s41386-020-00902-6
14. Daw ND, Niv Y, Dayan P. Uncertainty-based competition between prefrontal and dorsolateral striatal systems for behavioral control. Nat Neurosci. (2005) 8:1704–11. doi: 10.1038/nn1560
15. Gillan C, Otto A, Phelps E, Daw N. Model-based learning protects against forming habits. Cogn Affect Behav Neurosci. (2015) 15:523–36. doi: 10.3758/s13415-015-0347-6
16. Gillan C, Kosinski M, Whelan R, Phelps E, Daw N. Characterizing a psychiatric symptom dimension related to deficits in goal-directed control. Elife. (2016) 5:e11305. doi: 10.7554/eLife.11305
17. Voon V, Derbyshire K, Rück C, Irvine MA, Worbe Y, Enander J, el al. Disorders of compulsivity: a common bias towards learning habits. Mol Psychiatry. (2015) 20:345–52. doi: 10.1038/mp.2014.44
18. Deserno L, Hauser T. Beyond a cognitive dichotomy: can multiple decision systems prove useful to distinguish compulsive and impulsive symptom dimensions? Biol Psychiatry. (2020) 88:e49–51. doi: 10.1016/j.biopsych.2020.03.004
19. Huys Q, Maia T, Frank M. Computational psychiatry as a bridge from neuroscience to clinical applications. Nat Neurosci. (2016) 19:404–13. doi: 10.1038/nn.4238
20. Rabinovich M, Varona P. Discrete sequential information coding: heteroclinic cognitive dynamics. Front Comput Neurosci. (2018) 12:73. doi: 10.3389/fncom.2018.00073
21. Rabinovich M, Muezzinoglu M, Strigo I, Bystritsky A. Dynamical principles of emotion-cognition interaction: mathematical images of mental disorders. PLoS ONE. (2010) 5:e12547. doi: 10.1371/journal.pone.0012547
22. Karamintziou S, Deligiannis N, Piallat B, Polosan M, Chabardès S, David O, et al. Dominant efficiency of nonregular patterns of subthalamic nucleus deep brain stimulation for Parkinson's disease and obsessive-compulsive disorder in a data-driven computational model. J Neural Eng. (2016) 13:016013. doi: 10.1088/1741-2560/13/1/016013
23. Lenhard F, Sauer S, Andersson E, Månsson KN, Mataix-Cols D, Rück C, et al. Prediction of outcome in internet-delivered cognitive behaviour therapy for paediatric obsessive-compulsive disorder: a machine learning approach. Int J Methods Psychiatr Res. (2018) 27:e1576. doi: 10.1002/mpr.1576
24. Li F, Huang X, Tang W, Yang Y, Li B, Kemp G, et al. Multivariate pattern analysis of DTI reveals differential white matter in individuals with obsessive-compulsive disorder. Hum Brain Mapp. (2014) 35:2643–51. doi: 10.1002/hbm.22357
25. Hoexter M, Miguel E, Diniz J, Shavitt R, Busatto G, Sato J. Predicting obsessive-compulsive disorder severity combining neuroimaging and machine learning methods. J Affect Disord. (2013) 150:1213–6. doi: 10.1016/j.jad.2013.05.041
26. Ownby R. Computational model of obsessive-compulsive disorder: examination of etiologic hypothesis and treatment strategies. Depress Anxiety. (1998) 8:91–103. doi: 10.1002/(SICI)1520-6394(1998)8:3<91::AID-DA1>3.0.CO;2-Q
27. Metin S, Altuglu T, Metin B, Erguzel T, Yigit S, Arıkan M, et al. Use of EEG for predicting treatment response to transcranial magnetic stimulation in obsessive compulsive disorder. Clin EEG Neurosci. (2020) 51:139–45. doi: 10.1177/1550059419879569
28. Prochazkova L, Parkes L, Dawson A, Youssef G, Ferreira G, Lorenzetti V, et al. Unpacking the role of self-reported compulsivity and impulsivity in obsessive-compulsive disorder. CNS Spectr. (2018) 23:51–8. doi: 10.1017/S1092852917000244
29. Xing X, Jin L, Li Q, Yang Q, Han H, Xu C, et al. Modeling essential connections in obsessive-compulsive disorder patients using functional MRI. Brain Behav. (2020) 10:e01499. doi: 10.1002/brb3.1499
30. Murray GK, Knolle F, Ersche KD, Craig KJ, Abbott S, Shabbir SS, et al. Dopaminergic drug treatment remediates exaggerated cingulate prediction error responses in obsessive-compulsive disorder. Psychopharmacology. (2019) 236:2325–36. doi: 10.1007/s00213-019-05292-2
31. Shahar N, Moran R, Hauser TU, Kievit RA, McNamee D, Moutoussis M, et al. Credit assignment to state-independent task representations and its relationship with model-based decision making. Proc Natl Acad Sci USA. (2019) 116:15871–6. doi: 10.1073/pnas.1821647116
32. Deserno L, Huys QJ, Boehme R, Buchert R, Heinze HJ, Grace AA, et al. Ventral striatal dopamine reflects behavioral and neural signatures of model-based control during sequential decision making. Proc Natl Acad Sci USA. (2015) 112:1595–600. doi: 10.1073/pnas.1417219112
33. Kanen J, Ersche K, Fineberg N, Robbins T, Cardinal R. Computational modelling reveals contrasting effects on reinforcement learning and cognitive flexibility in stimulant use disorder and obsessive-compulsive disorder: remediating effects of dopaminergic D2/3 receptor agents. Psychopharmacology. (2019) 236:2337–58. doi: 10.1007/s00213-019-05325-w
34. Mills J, Strawn J. Antidepressant tolerability in pediatric anxiety and obsessive-compulsive disorders: a bayesian hierarchical modeling meta-analysis. J Am Acad Child Adolesc Psychiatry. (2020) 59:1240–51. doi: 10.1016/j.jaac.2019.10.013
35. Schlösser R, Wagner G, Schachtzabel C, Peikert G, Koch K, Reichenbach J, et al. Fronto-cingulate effective connectivity in obsessive compulsive disorder: a study with fMRI and dynamic causal modeling. Hum Brain Mapp. (2010) 31:1834–50. doi: 10.1002/hbm.20980
36. Fradkin I, Ludwig C, Eldar E, Huppert J. Doubting what you already know: uncertainty regarding state transitions is associated with obsessive compulsive symptoms. PLoS Comput Biol. (2020) 16:e1007634. doi: 10.1371/journal.pcbi.1007634
37. Fradkin I, Adams R, Parr T, Roiser J, Huppert J. Searching for an anchor in an unpredictable world: a computational model of obsessive compulsive disorder. Psychol Rev. (2020) 127:672–99. doi: 10.1037/rev0000188
38. Hauser T, Moutoussis M, Iannaccone R, Brem S, Walitza S, Drechsler R, et al. Increased decision thresholds enhance information gathering performance in juvenile Obsessive-Compulsive Disorder (OCD). PLoS Comput Biol. (2017) 13:e1005440. doi: 10.1371/journal.pcbi.1005440
39. Vaghi MM, Luyckx F, Sule A, Fineberg NA, Robbins TW, De Martino B. Compulsivity reveals a novel dissociation between action and confidence. Neuron. (2017) 96:348–54. doi: 10.1016/j.neuron.2017.09.006
40. Schulze D, Kathmann N, Reuter B. Getting it just right: a reevaluation of OCD symptom dimensions integrating traditional and Bayesian approaches. J Anxiety Disord. (2018) 56:63–73. doi: 10.1016/j.janxdis.2018.04.003
41. Solway A, Lin Z, Vinaik E. Transfer of information across repeated decisions in general and in obsessive-compulsive disorder. Proc Natl Acad Sci USA. (2021) 118:e2014271118. doi: 10.1073/pnas.2014271117
42. Solway A, Lin Z, Kaplan C. Revisiting verbal recognition memory in obsessive-compulsive disorder: a computational approach. J Psychiatr Res. (2021) 138:428–35. doi: 10.1016/j.jpsychires.2021.04.015
43. Banca P, Vestergaard MD, Rankov V, Baek K, Mitchell S, Lapa T, et al. Evidence accumulation in obsessive-compulsive disorder: the role of uncertainty and monetary reward on perceptual decision-making thresholds. Neuropsychopharmacology. (2015) 40:1192–202. doi: 10.1038/npp.2014.303
44. Hartmann CJ, Lujan JL, Chaturvedi A, Goodman WK, Okun MS, McIntyre CC, et al. Tractography activation patterns in dorsolateral prefrontal cortex suggest better clinical responses in OCD DBS. Front Neurosci. (2016) 9:519. doi: 10.3389/fnins.2015.00519
45. Lei H, Cui Y, Fan J, Zhang X, Zhong M, Yi J, et al. Abnormal small-world brain functional networks in obsessive-compulsive disorder patients with poor insight. J Affect Disord. (2017) 219:119–25. doi: 10.1016/j.jad.2017.05.032
46. Durstewitz D, Huys Q, Koppe G. Psychiatric illnesses as disorders of network dynamics. Biol Psychiatry Cogn Neurosci Neuroimaging. (2020) 6:865–76. doi: 10.1016/j.bpsc.2020.01.001
47. Maia T, McClelland J. A neurocomputational approach to obsessive-compulsive disorder. Trends Cogn Sci. (2012) 16:14–5. doi: 10.1016/j.tics.2011.11.011
48. Cocchi L, Zalesky A, Nott Z, Whybird G, Fitzgerald P, Breakspear M. Transcranial magnetic stimulation in obsessive-compulsive disorder: a focus on network mechanisms and state dependence. Neuroimage Clin. (2018) 19:661–74. doi: 10.1016/j.nicl.2018.05.029
49. Uddin L. Cognitive and behavioural flexibility: neural mechanisms and clinical considerations. Nat Rev Neurosci. (2021) 22:167–79. doi: 10.1038/s41583-021-00428-w
50. Gillan CM, Robbins TW. Goal-directed learning and obsessive-compulsive disorder. Philos Trans R Soc Lond B Biol Sci. (2014) 369:20130475. doi: 10.1098/rstb.2013.0475
51. Gillan CM, Robbins TW, Sahakian BJ, van den Heuvel OA, van Wingen G. The role of habit in compulsivity. Eur Neuropsychopharmacol. (2016) 26:828–40. doi: 10.1016/j.euroneuro.2015.12.033
52. Gruner P, Anticevic A, Lee D, Pittenger C. Arbitration between action strategies in obsessive-compulsive disorder. Neuroscientist. (2016) 22:188–98. doi: 10.1177/1073858414568317
53. Loosen AM, Hauser TU. Towards a computational psychiatry of juvenile obsessive-compulsive disorder. Neurosci Biobehav Rev. (2020) 118:631–42. doi: 10.1016/j.neubiorev.2020.07.021
54. Maia W, Cooney R, Peterson B. The neural bases of obsessive-compulsive disorder in children and adults. Dev Psychopathol. (2008) 20:1251–83. doi: 10.1017/S0954579408000606
55. Pittenger C, Krystal J, Coric V. Glutamate-modulating drugs as novel pharmacotherapeutic agents in the treatment of obsessive-compulsive disorder. NeuroRx. (2006) 3:69–81. doi: 10.1016/j.nurx.2005.12.006
56. Daw N, Gershman S, Seymour B, Dayan P, Dolan R. Model-based influences on humans' choices and striatal prediction errors. Neuron. (2011) 69:1204–15. doi: 10.1016/j.neuron.2011.02.027
57. Gillan CM, Apergis-Schoute AM, Morein-Zamir S, Urcelay GP, Sule A, Fineberg NA, et al. Functional neuroimaging of avoidance habits in obsessive-compulsive disorder. Am J Psychiatry. (2014) 172:284–93. doi: 10.1176/appi.ajp.2014.14040525
58. Fradkin I, Strauss A, Pereg M, Huppert J. Rigidly applied rules? Revisiting inflexibility in obsessive compulsive disorder using multilevel meta-analysis. Clin Psychol Rev. (2018) 6:481–505. doi: 10.1177/2167702618756069
59. Oulis P, Konstantakopoulos G, Lykouras L, Michalopoulou P. Differential diagnosis of obsessive-compulsive symptoms from delusions in schizophrenia: a phenomenological approach. World J Psychiatry. (2013) 3:50–6. doi: 10.5498/wjp.v3.i3.50
60. Jacob M, Larson M, Storch E. Insight in adults with obsessive-compulsive disorder. Compr Psychiatry. (2014) 55:896–903. doi: 10.1016/j.comppsych.2013.12.016
61. Jaafari N, Aouizerate B, Tignol J, El-Hage W, Wassouf I, Guehl D, et al. The relationship between insight and uncertainty in obsessive-compulsive disorder. Psychopathology. (2011) 44:272–6. doi: 10.1159/000323607
62. Liu W, Gan J, Fan J, Zheng H, Li S, Chan RCK, et al. Associations of cortical thickness, surface area and subcortical volumes with insight in drug-naïve adults with obsessive-compulsive disorder. Neuroimage Clin. (2019) 24:102037. doi: 10.1016/j.nicl.2019.102037
63. Fan J, Zhong M, Zhu X, Gan J, Liu W, Niu C, et al. Resting-state functional connectivity between right anterior insula and right orbital frontal cortex correlate with insight level in obsessive-compulsive disorder. Neuroimage Clin. (2017) 15:1–7. doi: 10.1016/j.nicl.2017.04.002
Keywords: OCD, computational modeling, trans-diagnostic perspective, computational psychiatry, personalized treatment
Citation: Szalisznyó K and Silverstein DN (2021) Computational Predictions for OCD Pathophysiology and Treatment: A Review. Front. Psychiatry 12:687062. doi: 10.3389/fpsyt.2021.687062
Received: 28 March 2021; Accepted: 01 June 2021;
Published: 01 October 2021.
Edited by:
Roseli Gedanke Shavitt, University of São Paulo, BrazilReviewed by:
Ygor Arzeno Ferrão, Federal University of Health Sciences of Porto Alegre, BrazilGuaraci Requena, Universidade Federal de Viçosa, Brazil
Copyright © 2021 Szalisznyó and Silverstein. This is an open-access article distributed under the terms of the Creative Commons Attribution License (CC BY). The use, distribution or reproduction in other forums is permitted, provided the original author(s) and the copyright owner(s) are credited and that the original publication in this journal is cited, in accordance with accepted academic practice. No use, distribution or reproduction is permitted which does not comply with these terms.
*Correspondence: Krisztina Szalisznyó, krisztina.szalisznyo@neuro.uu.se