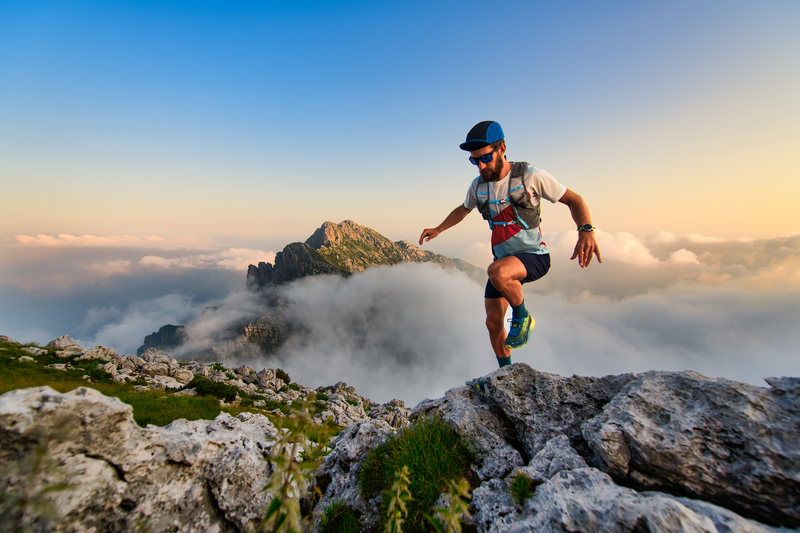
94% of researchers rate our articles as excellent or good
Learn more about the work of our research integrity team to safeguard the quality of each article we publish.
Find out more
ORIGINAL RESEARCH article
Front. Psychiatry , 19 July 2021
Sec. Mood Disorders
Volume 12 - 2021 | https://doi.org/10.3389/fpsyt.2021.683610
Background: Major depressive disorder (MDD) has demonstrated abnormalities of static intrinsic brain activity measured by amplitude of low-frequency fluctuation (ALFF). Recent studies regarding the resting-state functional magnetic resonance imaging (rs-fMRI) have found the brain activity is inherently dynamic over time. Little is known, however, regarding the temporal dynamics of local neural activity in MDD. Here, we investigated whether temporal dynamic changes in spontaneous neural activity are influenced by MDD.
Methods: We recruited 81 first-episode, drug-naive MDD patients and 64 age-, gender-, and education-matched healthy controls who underwent rs-fMRI. A sliding-window approach was then adopted for the estimation of dynamic ALFF (dALFF), which was used to measure time-varying brain activity and then compared between the two groups. The relationship between altered dALFF variability and clinical variables in MDD patients was also analyzed.
Results: MDD patients showed increased temporal variability (dALFF) mainly focused on the bilateral thalamus, the bilateral superior frontal gyrus, the right middle frontal gyrus, the bilateral cerebellum posterior lobe, and the vermis. Furthermore, increased dALFF variability values in the right thalamus and right cerebellum posterior lobe were positively correlated with MDD symptom severity.
Conclusions: The overall results suggest that altered temporal variability in corticocerebellar–thalamic–cortical circuit (CCTCC), involved in emotional, executive, and cognitive, is associated with drug-naive, first-episode MDD patients. Moreover, our study highlights the vital role of abnormal dynamic brain activity in the cerebellar hemisphere associated with CCTCC in MDD patients. These findings may provide novel insights into the pathophysiological mechanisms of MDD.
Major depressive disorder (MDD) is a common psychiatric illness, with clinical manifestations of persistently depressed mood, loss of interest, low self-esteem and energy, weight change, and cognitive dysfunction (1, 2). These heterogeneous symptoms often impair daily life function and raise the risk of suicide (3, 4). It is predicted that MDD may become the leading cause of disability in high-income countries by 2030 (5). Considering the high disability, high recurrence, severe distress, and heavy financial burden, it is important to achieve a deeper understanding of the underlying neural mechanism to reduce the risks posed by MDD.
To determine the neurobiological mechanisms of MDD, neuroimaging researches have attempted to discover the underlying mechanisms of MDD by investigating functional abnormalities in the brain of MDD patients. For example, an increasing number of abnormal resting-state networks have been found in MDD patients, such as hyperactivity in task performance and hyperconnectivity of default mode network and decreased connectivity within salience network (6–8), supporting internally directed and self-referential thought and involved in pathological rumination in MDD (9). Another finding is that the aberrance of limbic regions led to dysregulation of emotion in MDD (10–12). In addition, structural impairments including reduced gray-matter volume in frontal cortex, hippocampus, cingulate cortex, amygdala, thalamus, putamen, and striatum were observed in MDD patients (13–16). Furthermore, some researches focused on the abnormal local brain activity in MDD using resting-state functional magnetic resonance imaging (rs-fMRI). An rs-fMRI study reported that increased amplitude of low-frequency fluctuation (ALFF) in the right ventral median frontal gyrus and higher ALFF in the right putamen in MDD patients (17). Another article showed increased ALFF primarily in the forebrain, while there was decreased ALFF in the posterior brain regions in treatment-naive MDD patients (18). A meta-analysis found decreased ALFF activity of the cerebellum in drug-naive MDD patients and increased ALFF activity in the anterior cingulate cortex (19). Liu et al. used fractional ALFF (fALFF), an improved ALFF method to measure the ratio of power spectrum of low-frequency range to that of the whole frequency range. In addition, they found that patients with MDD showed significantly decreased fALFF in the right cerebellum posterior lobe (CPL), left parahippocampal gyrus, and right middle frontal gyrus (MFG) and increased fALFF in the left superior occipital gyrus/cuneus (20). However, most of these studies were based on the implicit assumption that brain activity remains stationary during rs-fMRI scanning. Recently, an accumulating number of researches suggest that brain activity is dynamic over time (21–27).
Dynamic brain activity may indicate information on the variability in the strength or spatial dynamic organization of the brain, according to neuroimaging studies (28, 29). The fact that dynamics can capture uncontrollable yet repeated patterns of brain networks, which cannot be identified by static analysis, is a major inspiration for such studies (30, 31). As previously reported, dynamic functional connectivity can be used to distinguish patients from healthy controls in MDD, epilepsy, and schizophrenia (32–34). Xue et al. showed reduced dynamic regional homogeneity (dReHo) in both fusiform gyri and in the right temporal pole and hippocampus in MDD compared with healthy controls and further demonstrated the expression profiles of 16 gene modules were correlated with dReHo alterations in MDD (35). However, research into the dynamics of local brain activity is still limited. The traditional static ALFF (sALFF), as a novel, non-invasive method, can characterize the energy intensity of brain activity over a period of time and effectively delineate the potential pathophysiological mechanisms of diseases (29, 36, 37). ALFF is demonstrated to have higher test–retest reliability than ReHo and high intrascanner reliability and can provide reliable information for studies (38). By integrating the ALFF with the “sliding-window” method, the dynamic ALFF (dALFF), based on the theory that resting-state brain is a highly dynamic system on a variety of time scales, offers a new way to evaluate the variance of ALFF over time by calculating the temporal variability of local brain activity amplitude among voxels (21, 22, 24, 28, 39–43). Put in a nutshell, the sALFF demonstrates the stable activity intensity of brain regions, which represents baseline energy consumption for sustaining essential brain functions. The dALFF reflects the plasticity and flexibility of spontaneous brain activity through the variability of energy expenditure. Taken together, we combine sALFF and dALFF to explore the abnormality of brain activity in MDD patients, which may provide novel insight in the study of MDD.
Here, we employed the ALFF combined with a sliding-window approach to assess the temporal variability of intrinsic brain activity in MDD patients. We expected that patients with MDD would show altered dALFF patterns compared to those of HCs. Furthermore, dALFF may detect some underlying abnormal intrinsic brain activity that sALFF cannot obtain, which can deepen our understanding of the physiological mechanism of MDD. We also hypothesized that the dynamic indexes may be associated with MDD clinical characteristics.
The institutional review board of the First Affiliated Hospital of Zhengzhou University approved this prospective study, and written informed consent was obtained from each subject.
This study consists of 145 participants totally, including 81 first-episode drug-naive individuals with MDD (63% female) and 64 age-, gender-, and education level–matched healthy control subjects (50% female). The diagnosis of first-episode MDD was conducted according to the Structured Clinical Interview of the Diagnostic and Statistical Manual of Mental Disorders, Fourth Edition (DSM-IV) Patient Edition. The severity of MDD was assessed with the 24-item Hamilton Depression Scale (HAMD) (44, 45). All healthy control participants were screened for a current or past diagnosis of any Axis I or II disorder using the Structured Clinical Interview of the DSM-IV Non–Patient Edition and Structured Clinical Interview for DSM-IV Axis II Personality Disorders. Inclusion criteria of MDD included (1) 10–60 years of age and right-handed; (2) drug-naive and at first episode of depression; (3) currently suffering an episode of depression with HAMD total score ≥21; and (4) a duration of depression >2 weeks but ≤40 weeks. Exclusion criteria included (1) a history of medical condition of antipsychotic medicine; (2) other current or past psychiatric disorders such as bipolar or schizophrenia; (3) a history of cardiovascular or other serious systemic diseases; (4) dementia; (5) other neurological disease or prior head trauma leading to cognitive disorder; (6) current alcohol or substance addiction such as alcohol, tobacco, or drug dependence; (7) head movement >2.5 mm or 2.5°. All healthy participants were right-handed and had no first-degree family history of psychiatric disorders.
MR data acquisition was conducted on a 3-T GE MR scanner (Discovery 750 System, Milwaukee, WI, USA) with a 16-channel head coil. The rs-fMRI data were obtained using the following parameters: 32 axial slices without slice gap, repetition time (TR)/echo time = 2,000/41 ms, field of view = 220 × 220 mm2, in-plane matrix = 64 × 64, section thickness = 5 mm, slice gap = 0.4 mm, flip angle = 90°, and a total of 240 volumes and lasted 480 s for each subject. All participants were placed in a supine position with wearing earplugs to reduce the noise. To reduce head excessive motion, a foam pad was fixed on the both sides of head. During MR scanning, all participants were required to keep their eyes closed and heads still without falling asleep and try not to think about anything in particular.
Functional images were preprocessed using a software (Data Processing and Analysis for Brain Imaging toolbox; http://rfmri.org/DPABI) (46). The main steps were included: (1) exclusion of the initial 10 volumes to ensure signal stability; (2) slice timing and realignment; (3) spatial normalization to the standard Montreal Neurological Institute (MNI 152) space and resampled to 3 × 3 × 3 mm3; (4) spatial smoothing using a 6-mm full-width half-maximum Gaussian kernel; (5) detrending the BOLD signals to correct a linear trend; (6) regression out of the nuisance covariates including the averaged signals from global mean signals (22, 28, 30, 47), cerebrospinal fluid signals, white matter signals, and Friston-24 head motion parameters; (7) temporal filtering (bandpass, 0.01–0.08 Hz) of BOLD signals; (8) to exclude the influence of head motion and ensure the contiguous time points, scrubbing with cubic spline interpolation was used; (9) additionally, to evaluate the head movement, we also calculate the mean frame-wise displacement (FD) (48, 49). In the group-level analysis, we also used the mean FD as a covariate to reduce the impact of motion artifact in the fMRI signal.
A sliding-window approach was applied to characterize the dynamic patterns equipped in Dynamic Brain Connectome (DynamicBC) toolbox (V2.2 http://restfmri.net/forum/DynamicBC) (42). Window length is an important parameter in resting-state dynamics computation. A shorter window length may increase the risk of introducing spurious fluctuations in the observed dALFF (50). By contrast, a longer window length may hinder the description of the temporal variability dynamics of ALFF (28). Based on this, according to the previous researches (22, 28, 51), we selected a window length of 50 TRs (100 s) and a window overlap of 60% (step size by 20 TRs) to compute the dALFF of each participant. The ALFF maps for each subject were computed within each window, generating a series of ALFF maps. Subsequently, the variance of dALFF maps across time was calculated to measure the temporal variability of intrinsic brain activity. Finally, the dALFF variability of all participants was then transformed into standardized z scores by subtracting the mean and dividing by the SD across each voxel to enhance data normality. To verify whether dALFF and sALFF exhibited similar or complementary information for our deep understanding of the neuropathological mechanisms about MDD, we obtained the sALFF map of each participant and then transformed into standardized z scores (37).
Demographic and clinical results were evaluated using a set of independent two-sample t-tests, p < 0.05 was considered as statistically significant including age and education, and χ2-test was used for gender. In order to further investigate the variations in temporal variability of dALFF, a two-sample t-test was conducted between the MDD and HC groups, with age, gender, education level, and mean FD as covariates. Multiple comparison was corrected for two-sample t-test using a topological false discovery rate (FDR) approach, with the initial height threshold of uncorrected p < 0.001 and topological FDR: q < 0.05. Similarly, two-sample t-test with the same covariates was applied to assess group differences of sALFF.
Once significant differences in dALFF and sALFF were detected in any brain regions, we extracted the mean dALFF variability values of the region of interest (ROI) of each dALFF and mean sALFF values of ROI of each sALFF using toolkit (rs-fMRI data analysis, http://www.restfmri.net/forum/REST) (52). Then, a two-tailed partial correlation analysis was conducted to further assess the relationship between the mean values (dALFF variability and sALFF) and clinical variables (HAMD score) in the MDD group, controlling for age, gender, education level, and head motion. A statistically significant threshold of p < 0.05/8 (Bonferroni corrected) was set for all correlation analyses.
We validated our main results with different window lengths and different overlap rates (30 TRs, 0.6 overlap; 80 TRs, 0.6 overlap; 50 TRs, 0.8 overlap). An ALFF map was obtained for each sliding window, and the dALFF of each voxel was standardized using z transformation.
To test the reproducibility of our main findings, we randomly selected 40 cases from 81 MDD patients as the MDD group compared with 64 in the HC group.
The demographics and clinical features of the two groups are summarized in Table 1. No significant differences were detected between MDD patients and HC subjects in terms of demographic characteristics, such as age, gender, and education level. For MDD patients, the HAMD scores ranged from 21 to 68.
As shown in Figure 1 and Table 2, the main results reported were based on the dALFF analysis using 50 TRs (100 s) as the window length. According to two-sample t-test, MDD patients, relative to controls, showed higher dALFF variability in the bilateral thalamus, bilateral superior frontal gyrus (SFG), bilateral CPL, right MFG, and vermis (q < 0.05, topological FDR corrected).
Figure 1. Brain regions with significant group differences in dALFF variability (50 TRs; 0.6 overlap). Group differences of temporal variability of dALFF between the MDD and HC groups were identified using a two-sample t-test. The statistical significance level was set at p < 0.05, topological FDR corrected. Patients with MDD showed significantly increased dALFF variability in the bilateral CPL, SFG, thalamus, and right MFG and vermis. dALFF, dynamic amplitude of low-frequency fluctuation; MDD, major depressive disorder; HC, healthy control; CPL, cerebellum posterior lobe; SFG, superior frontal gyrus; MFG, middle frontal gyrus.
Table 2. Brain regions showing significant differences in dALFF between MDD patients and HC subjects.
Compared with HC, the MDD group showed decreased sALFF in the right CPL, left calcarine, left occipital, left fusiform gyrus, and right calcarine. The increased sALFF was located in the vermis (q < 0.05, topological FDR corrected) (Supplementary Figure 1 and Supplementary Table 1).
The 24-HAMD score was positively associated with dALFF variability in the right CPL and the right thalamus (r = 0.43, p = 0.004; r = 0.37, p = 0.003, Bonferroni corrected, respectively, Figure 2); No significant correlation was found in the relationship between abnormal sALFF and HAMD score in MDD patients.
Figure 2. dALFF variability in the right thalamus and that in CPL were positively correlated with HAMD score of patients with MDD. dALFF, dynamic amplitude of low-frequency fluctuation; Tha, thalamus; CPL, cerebellum posterior lobe; HAMD, Hamilton Depression Rating Scale; MDD, major depressive disorder.
In our study, we validated our results by using different sliding-window lengths and different overlap rates. Finally, the results of the remaining two window lengths (30 TRs, 0.6 overlap; 80 TRs, 0.6 overlap) and one another overlap (50 TRs, 0.8 overlap) were presented in Supplementary Figures 2–4.
We randomly selected 40 cases from the total 84 patients as MDD group compared with 64 in the HC group, and this validation analysis replicated this higher dALFF variability of the corticocerebellar–thalamic–cortical circuit (CCTCC), and the detailed results are presented in Supplementary Figure 5.
To the best of our knowledge, we observed that only several researches employed a dALFF method to elucidate the dynamics of spontaneous neural-activity characteristics of MDD. In our study, increased dALFF variability in patients with MDD was prominently located in CCTCC, including bilateral thalamus, bilateral SFG, bilateral CPL, right MFG, and vermis, representing the anatomical substratum of functional networks governing behavior: executive, salience, default mode, dorsal attentional, and motor networks (53). Furthermore, the altered temporal ALFF variability values in right CPL and right thalamus had a significantly positive correlation in MDD patients. Overall, this study provides convincing evidence for dysfunction of intrinsic brain activity in CCTCC of MDD patients, which may underscore the importance of considering brain dynamics and truly inform the potential mechanism underlying MDD.
MDD showed increased dALFF in CCTCC, which are involved in emotional, executive, and cognitive functions. Of note, emotional dysfunction and cognitive deficits are the most prominent features of MDD (1), and Pessoa (54) suggested that emotion and cognition are often integrated and jointly contribute to behavior. Although previous neuroimaging studies have shown that CCTCC may be the primary abnormality in schizophrenia (55–58), the dysfunction of CCTCC has not been reported in MDD. CCTCC is the substrate of synchrony, which can integrate cerebral cortex and the cerebellum to ensure fluidly coordinating sequences of thought and action, occurring as a consequence of very rapid online processing and feedback (55). According to the hypothesis of cognitive disorder, the emergence of psychiatric illnesses may result from the altered of specific parts of the CCTCC, which could lead to the dysfunction of mental coordination processes, such as schizophrenia and Parkinson disease (55, 59–61). MDD is a complicated disease with the dysfunction of cortical and subcortical structures that modulate cognitive and emotional aspects of behavior and the deficiency of neural systems related to decision-making and energy–vitality in thought and action. Therefore, we speculated that increased dALFF variability in the CCTCC may deepen our sight on the abnormal cognitive and emotional processing in MDD patients.
Our study found increased dALFF in the bilateral CPL, whereas there was a decreased sALFF in the right CPL, showing dysfunctional intrinsic activity of the posterior cerebellum in MDD. The cerebellum has proven bidirectional connections with brain areas involved in emotional control and the interpretation of socially salient emotional information, according to anatomic proof (62). In particular, substantial associations have been established between the cerebellum and the posterior parietal and prefrontal cortical regions (63–66), as well as limbic regions, including the amygdala, the hippocampus, and the septal nuclei (67, 68). The cerebellum, as part of CCTCC, has been proposed to have an activity in the production of a variety of symptoms and higher cognitive impairments in mental disorders (69–72). Abnormal structure and function in the cerebellum have been demonstrated in MDD patients (73), including abnormalities of gray-matter volume (74, 75), glucose metabolism (76, 77), functional activity, and connectivity (78–84). A previous meta-analysis demonstrated decreased sALFF in the bilateral cerebellum in MDD (85), which was in line with our results. Moreover, the current findings of increased dALFF (more variability) in cerebellum were in line with previous studies and may reflect the instability of neurofluctuation and information integration in MDD patients. Taken together, we speculate that the increased dALFF in the bilateral CPL and decreased sALFF in the right CPL may reflect the abnormity or dysfunction of cerebellum, which may disrupt brain top-down processing in emotional, executive, and cognitive functions.
We found another considerably increased dALFF and sALFF in the vermis. The vermis, also known as the limbic cerebellum, has been reported to have wide connections with the limbic systems, thus providing the anatomic substrate for cerebellar involvement in emotional and affective behaviors (66, 68, 86, 87). Interestingly, several articles demonstrated that activation of the posterior cerebellar vermis has been identified when participants process their own traumatic encounter, whereas activation of the posterior cerebellar hemisphere has been found when subjects experience empathy for the suffering of others (88, 89). Above all, these results indicate that the vermis is essential to emotional/affective processing. In our study, the increased dALFF variability and sALFF exhibit aberrant temporal fluctuation and hyperactivation of local brain activity in these regions in MDD patients. Such abnormalities may disable to engage the network between limbic cerebellum and limbic cerebrum in emotion regulation in MDD.
We observed increased dALFF in the bilateral thalamus in MDD relative to the HC group, suggesting that abnormal brain instantaneous activity in thalamus may affect the association of CCTCC. The thalamus is an integral part of the emotional salience network, emotion modulation network, and cognitive/executive network (90), which are associated with MDD. The volume atrophy of thalamus was thought to better account for the deficits in top-down regulation of negative emotions among persons more vulnerable to developing depressive state (91). In addition, neuroimaging study has demonstrated that increased thalamic functional connectivity was related to decreased cognitive function in disease conditions (92–96). Notably, the present study also showed positive association between the dALFF variability in the right thalamus and the HAMD-24 score, suggesting that the dALFF variability of the right thalamus could progressively increase during the development of the severity of MDD. Therefore, these findings suggest that increased dALFF in the thalamus may disturb cognitive activity in MDD.
Our study found increased dALFF variance in the bilateral SFG and the right MFG in the MDD group. SFG and MFG are essential parts of dorsolateral prefrontal cortex (DLPFC), which is the core region of depressive episode and maintenance, involving cognitive control such as biased attention, negative self-referential schemas, and rumination (97). Beyond that, the DLPFC is a critical part of dorsal neural system implicated in regulating the parameters of affective states (98). According to previous reports, the changes in the volume or function of DLPFC regions have been considered to be the most common abnormal region in MDD. For example, previous articles have shown hypermetabolism and hyperactivity in the right DLPFC in MDD patients with application of different imaging techniques (positron emission tomography, fMRI, transcranial magnetic stimulation) (99–106). And also, some articles have proved that the altered volume of DLPFC correlates well with the activation during working memory updating and during conscious negative emotion processing in fMRI studies (107–109). Thus, our findings are compatible with these previous studies, demonstrating that increased dALFF variability may underlie the phenomenon of abnormal cognitive control and affective states.
sALFF and dALFF revealed a small overlap group differences in our study, including right CPL and vermis. In addition, decreased sALFF in the bilateral calcarine, left occipital lobe, and fusiform gyrus was also observed. Several studies have reported alterations along the visual pathway including occipital lobe and calcarine, as well as more downstream areas (e.g., fusiform gyrus) during working memory, attention, and visual categorization tasks in MDD (110–112). And also, there are some differences in spatial activation patterns in visual regions between healthy and depressed individuals (113–118). In addition, increased dALFFs in the right CPL and thalamus were positively correlated with HAMD score, whereas those associations were not detected in sALFF. These findings suggest that dALFF provides complementary information to probing pathological changes in MDD.
This study has some limitations. First, the optimal window size for capturing the dynamics of brain activity is still uncertain. In current study, we chose 50 TRs as the window length, which was proposed in previous articles (22, 28, 50). In our validation analysis, the main results of 50 TRs were close to the results of different sliding-window lengths and different overlap, showing that our findings of dALFF variability were relatively stable. Second, dALFF was used in current research to detect the temporal dynamics of local brain activity. However, further studies could also explore other parameters to characterize dynamic features precisely. Third, the small sample may affect the power of the statistical analysis. Future studies with a large sample size are needed to provide a comprehensive interpretation of the findings.
In our study, the most parsimonious conclusion is that patients with MDD exhibited increased temporal variability of dALFF in regions mainly focused on CCTCC implicated in emotional, executive, and cognitive. More broadly, the dALFF abnormalities in the right thalamus and the right CPL were associated with the severity of illness, whereas sALFF could not detect this association. This study may emphasize the importance of applying the dynamic local brain activity in the pathophysiology underlying MDD and also demonstrated that dALFF could be a potential imaging biomarker for the diagnosis of MDD.
Our current study provide evidence for intrinsic brain activity changes associated with MDD in CCTCC, which previous literatures rarely have detected in MDD. This may be due to an artifact of methodological constraints shared across the existing literature, and it also shows the sensitivity of dALFF in monitoring intrinsic brain activity. We look forward to future work that advances beyond the methodological constraints of the existing literature in order to make meaningful inroads in detecting the pathological mechanism of MDD. In addition, the present work also highlights the vital role of abnormal dynamic brain activity in the cerebellar hemisphere associated with CCTCC in MDD patients. Thus, future work could seek for a more comprehensive evidence to validate our speculation that the dysregulation of neuron in the cerebellar hemisphere may lead to the dysfunction of CCTCC in MDD.
The data analyzed in this study is subject to the following licenses/restrictions: The datasets used and analyzed during the current study are available from the corresponding author on reasonable request. Requests to access these datasets should be directed to ZmNjY2hlbmdqbEB6enUuZWR1LmNu.
The studies involving human participants were reviewed and approved by Zhengzhou University First Affiliated Hospital. Written informed consent to participate in this study was provided by the participants' legal guardian/next of kin.
RZ, YC, MW, and SH conceived and designed the study. RZ and MW supervised the conduct of the study. YC, YJ, BZ, SL, and ZY are responsible for data acquisition. RZ and YC analyzed the data and takes responsibility for the paper. RZ, YC, YJ, and YW assisted with literature review. RZ, YC, and SH drafted the initial manuscript. JC, YZ, and SH reviewed and revised the manuscript. All authors read and approved the final manuscript.
This research study was supported by the Natural Science Foundation of China (Nos. 81601467, 81871327, and 81601472). Medical Science and Technology research project of Henan province (201701011).
The authors declare that the research was conducted in the absence of any commercial or financial relationships that could be construed as a potential conflict of interest.
The Supplementary Material for this article can be found online at: https://www.frontiersin.org/articles/10.3389/fpsyt.2021.683610/full#supplementary-material
1. Association AP. Diagnostic and Statistical Manual of Mental Disorders (DSM-5®). Washington, DC: American Psychiatric Pub. (2013).
2. Yu M, Linn KA, Shinohara RT, Oathes DJ, Cook PA, Duprat R, et al. Childhood trauma history is linked to abnormal brain connectivity in major depression. Proc Natl Acad Sci USA. (2019) 116:8582–90. doi: 10.1073/pnas.1900801116
3. Chesney E, Goodwin GM, Fazel S. Risks of all-cause and suicide mortality in mental disorders: a meta-review. World Psychiatry. (2014) 13:153–60. doi: 10.1002/wps.20128
4. Hawton K, van Heeringen K. Suicide. Lancet. (2009) 373:1372–81. doi: 10.1016/S0140-6736(09)60372-X
5. Wiles N, Thomas L, Abel A, Ridgway N, Turner N, Campbell J, et al. Cognitive behavioural therapy as an adjunct to pharmacotherapy for primary care based patients with treatment resistant depression: results of the CoBalT randomised controlled trial. Lancet. (2013) 381:375–84. doi: 10.1016/S0140-6736(12)61552-9
6. Han S, Wang X, He Z, Sheng W, Zou Q, Li L, et al. Decreased static and increased dynamic global signal topography in major depressive disorder. Prog Neuropsychopharmacol Biol Psychiatry. (2019) 94:109665. doi: 10.1016/j.pnpbp.2019.109665
7. Veer IM, Beckmann CF, van Tol MJ, Ferrarini L, Milles J, Veltman DJ, et al. Whole brain resting-state analysis reveals decreased functional connectivity in major depression. Front Syst Neurosci. (2010) 4:41. doi: 10.3389/fnsys.2010.00041
8. Whitfield-Gabrieli S, Ford JM. Default mode network activity and connectivity in psychopathology. Annu Rev Clin Psychol. (2012) 8:49–76. doi: 10.1146/annurev-clinpsy-032511-143049
9. Johnson MK, Nolen-Hoeksema S, Mitchell KJ, Levin Y. Medial cortex activity, self-reflection and depression. Soc Cogn Affect Neurosci. (2009) 4:313–27. doi: 10.1093/scan/nsp022
10. Betts J, Gullone E, Allen JS. An examination of emotion regulation, temperament, and parenting style as potential predictors of adolescent depression risk status: a correlational study. Br J Dev Psychol. (2009) 27:473–85. doi: 10.1348/026151008X314900
11. Erk S, Mikschl A, Stier S, Ciaramidaro A, Gapp V, Weber B, et al. Acute and sustained effects of cognitive emotion regulation in major depression. J Neurosci. (2010) 30:15726–34. doi: 10.1523/JNEUROSCI.1856-10.2010
12. Sliz D, Hayley S. Major depressive disorder and alterations in insular cortical activity: a review of current functional magnetic imaging research. Front Hum Neurosci. (2012) 6:323. doi: 10.3389/fnhum.2012.00323
13. Arnone D, Job D, Selvaraj S, Abe O, Amico F, Cheng Y, et al. Computational meta-analysis of statistical parametric maps in major depression. Hum Brain Mapp. (2016) 37:1393–404. doi: 10.1002/hbm.23108
14. Arnone D, McIntosh AM, Ebmeier KP, Munafò MR, Anderson IM. Magnetic resonance imaging studies in unipolar depression: systematic review and meta-regression analyses. Eur Neuropsychopharmacol. (2012) 22:1–16. doi: 10.1016/j.euroneuro.2011.05.003
15. Nugent AC, Davis RM, Zarate CA Jr, Drevets WC. Reduced thalamic volumes in major depressive disorder. Psychiatry Res. (2013) 213:179–85. doi: 10.1016/j.pscychresns.2013.05.004
16. Sexton CE, Mackay CE, Ebmeier KP. A systematic review and meta-analysis of magnetic resonance imaging studies in late-life depression. Am J Geriatr Psychiatry. (2013) 21:184–95. doi: 10.1016/j.jagp.2012.10.019
17. Jing B, Liu CH, Ma X, Yan HG, Zhuo ZZ, Zhang Y, et al. Difference in amplitude of low-frequency fluctuation between currently depressed and remitted females with major depressive disorder. Brain Res. (2013) 1540:74–83. doi: 10.1016/j.brainres.2013.09.039
18. Liu J, Ren L, Womer FY, Wang J, Fan G, Jiang W, et al. Alterations in amplitude of low frequency fluctuation in treatment-naive major depressive disorder measured with resting-state fMRI. Hum Brain Mapp. (2014) 35:4979–88. doi: 10.1002/hbm.22526
19. Zhou M, Hu X, Lu L, Zhang L, Chen L, Gong Q, et al. Intrinsic cerebral activity at resting state in adults with major depressive disorder: a meta-analysis. Prog Neuropsychopharmacol Biol Psychiatry. (2017) 75:157–64. doi: 10.1016/j.pnpbp.2017.02.001
20. Liu F, Guo W, Liu L, Long Z, Ma C, Xue Z, et al. Abnormal amplitude low-frequency oscillations in medication-naive, first-episode patients with major depressive disorder: a resting-state fMRI study. J Affect Disord. (2013) 146:401–6. doi: 10.1016/j.jad.2012.10.001
21. Allen EA, Damaraju E, Plis SM, Erhardt EB, Eichele T, Calhoun VD. Tracking whole-brain connectivity dynamics in the resting state. Cereb Cortex. (2014) 24:663–76. doi: 10.1093/cercor/bhs352
22. Cui Q, Sheng W, Chen Y, Pang Y, Lu F, Tang Q, et al. Dynamic changes of amplitude of low-frequency fluctuations in patients with generalized anxiety disorder. Hum Brain Mapp. (2020) 41:1667–76. doi: 10.1002/hbm.24902
23. Guo WB, Liu F, Xue ZM, Xu XJ, Wu RR, Ma CQ, et al. Alterations of the amplitude of low-frequency fluctuations in treatment-resistant and treatment-response depression: a resting-state fMRI study. Prog Neuropsychopharmacol Biol Psychiatry. (2012) 37:153–60. doi: 10.1016/j.pnpbp.2012.01.011
24. Hutchison RM, Womelsdorf T, Allen EA, Bandettini PA, Calhoun VD, Corbetta M, et al. Dynamic functional connectivity: promise, issues, and interpretations. Neuroimage. (2013) 80:360–78. doi: 10.1016/j.neuroimage.2013.05.079
25. Li R, Liao W, Yu Y, Chen H, Guo X, Tang YL, et al. Differential patterns of dynamic functional connectivity variability of striato-cortical circuitry in children with benign epilepsy with centrotemporal spikes. Hum Brain Mapp. (2018) 39:1207–17. doi: 10.1002/hbm.23910
26. Liu F, Hu M, Wang S, Guo W, Zhao J, Li J, et al. Abnormal regional spontaneous neural activity in first-episode, treatment-naive patients with late-life depression: a resting-state fMRI study. Prog Neuropsychopharmacol Biol Psychiatry. (2012) 39:326–31. doi: 10.1016/j.pnpbp.2012.07.004
27. Lu F, Liu P, Chen H, Wang M, Xu S, Yuan Z, et al. More than just statics: Abnormal dynamic amplitude of low-frequency fluctuation in adolescent patients with pure conduct disorder. J Psychiatr Res. (2020) 131:60–8. doi: 10.1016/j.jpsychires.2020.08.027
28. Li J, Duan X, Cui Q, Chen H, Liao W. More than just statics: temporal dynamics of intrinsic brain activity predicts the suicidal ideation in depressed patients. Psychol Med. (2019) 49:852–60. doi: 10.1017/S0033291718001502
29. Liao W, Li J, Ji GJ, Wu GR, Long Z, Xu Q, et al. Endless Fluctuations: Temporal Dynamics of the Amplitude of Low Frequency Fluctuations. IEEE Trans Med Imaging. (2019) 38:2523–32. doi: 10.1109/TMI.2019.2904555
30. Guo J, Biswal BB, Han S, Li J, Yang S, Yang M, et al. Altered dynamics of brain segregation and integration in poststroke aphasia. Hum Brain Mapp. (2019) 40:3398–409. doi: 10.1002/hbm.24605
31. Ma S, Calhoun VD, Phlypo R, Adali T. Dynamic changes of spatial functional network connectivity in healthy individuals and schizophrenia patients using independent vector analysis. Neuroimage. (2014) 90:196–206. doi: 10.1016/j.neuroimage.2013.12.063
32. Damaraju E, Allen EA, Belger A, Ford JM, McEwen S, Mathalon DH, et al. Dynamic functional connectivity analysis reveals transient states of dysconnectivity in schizophrenia. Neuroimage Clin. (2014) 5:298–308. doi: 10.1016/j.nicl.2014.07.003
33. Liu F, Wang Y, Li M, Wang W, Li R, Zhang Z, et al. Dynamic functional network connectivity in idiopathic generalized epilepsy with generalized tonic-clonic seizure. Hum Brain Mapp. (2017) 38:957–73. doi: 10.1002/hbm.23430
34. Yao Z, Shi J, Zhang Z, Zheng W, Hu T, Li Y, et al. Altered dynamic functional connectivity in weakly-connected state in major depressive disorder. Clin Neurophysiol. (2019) 130:2096–104. doi: 10.1016/j.clinph.2019.08.009
35. Xue K, Liang S, Yang B, Zhu D, Xie Y, Qin W, et al. Local dynamic spontaneous brain activity changes in first-episode, treatment-naïve patients with major depressive disorder and their associated gene expression profiles. Psychol Med. (2020) 2020:1–10. doi: 10.1017/S0033291720003876
36. Hoptman MJ, Zuo XN, Butler PD, Javitt DC, D'Angelo D, Mauro CJ, et al. Amplitude of low-frequency oscillations in schizophrenia: a resting state fMRI study. Schizophr Res. (2010) 117:13–20. doi: 10.1016/j.schres.2009.09.030
37. Zang YF, He Y, Zhu CZ, Cao QJ, Sui MQ, Liang M, et al. Altered baseline brain activity in children with ADHD revealed by resting-state functional MRI. Brain Dev. (2007) 29:83–91. doi: 10.1016/j.braindev.2006.07.002
38. Zhao N, Yuan LX, Jia XZ, Zhou XF, Deng XP, He HJ, et al. Intra- and Inter-Scanner Reliability of Voxel-Wise Whole-Brain Analytic Metrics for Resting State fMRI. Front Neuroinform. (2018) 12:54. doi: 10.3389/fninf.2018.00054
39. Allen EA, Erhardt EB, Damaraju E, Gruner W, Segall JM, Silva RF, et al. A baseline for the multivariate comparison of resting-state networks. Front Syst Neurosci. (2011) 5:2. doi: 10.3389/fnsys.2011.00002
40. Fu Z, Tu Y, Di X, Du Y, Pearlson GD, Turner JA, et al. Characterizing dynamic amplitude of low-frequency fluctuation and its relationship with dynamic functional connectivity: an application to schizophrenia. Neuroimage. (2018) 180:619–31. doi: 10.1016/j.neuroimage.2017.09.035
41. Hutchison RM, Morton JB. Tracking the brain's functional coupling dynamics over development. J Neurosci. (2015) 35:6849–59. doi: 10.1523/JNEUROSCI.4638-14.2015
42. Liao W, Wu GR, Xu Q, Ji GJ, Zhang Z, Zang YF, et al. DynamicBC: a MATLAB toolbox for dynamic brain connectome analysis. Brain Connect. (2014) 4:780–90. doi: 10.1089/brain.2014.0253
43. Marusak HA, Calhoun VD, Brown S, Crespo LM, Sala-Hamrick K, Gotlib IH, et al. Dynamic functional connectivity of neurocognitive networks in children. Hum Brain Mapp. (2017) 38:97–108. doi: 10.1002/hbm.23346
44. Hamilton M. A rating scale for depression. J Neurol Neurosurg Psychiatry. (1960) 23:56–62. doi: 10.1136/jnnp.23.1.56
45. Wu F, Tu Z, Sun J, Geng H, Zhou Y, Jiang X, et al. Abnormal functional and structural connectivity of amygdala-prefrontal circuit in first-episode adolescent depression: a combined fMRI and DTI study. Front Psychiatry. (2019) 10:983. doi: 10.3389/fpsyt.2019.00983
46. Yan CG, Wang XD, Zuo XN, Zang YF. DPABI: data processing & analysis for (resting-state) brain imaging. Neuroinformatics. (2016) 14:339–51. doi: 10.1007/s12021-016-9299-4
47. Jiang S, Luo C, Huang Y, Li Z, Chen Y, Li X, et al. Altered static and dynamic spontaneous neural activity in drug-naïve and drug-receiving benign childhood epilepsy with centrotemporal spikes. Front Hum Neurosci. (2020) 14:361. doi: 10.3389/fnhum.2020.00361
48. He Z, Lu F, Sheng W, Han S, Long Z, Chen Y, et al. Functional dysconnectivity within the emotion-regulating system is associated with affective symptoms in major depressive disorder: a resting-state fMRI study. Aust N Z J Psychiatry. (2019) 53:528–39. doi: 10.1177/0004867419832106
49. Lu FM, Liu CH, Lu SL, Tang LR, Tie CL, Zhang J, et al. Disrupted Topology of Frontostriatal Circuits Is Linked to the Severity of Insomnia. Front Neurosci. (2017) 11:214. doi: 10.3389/fnins.2017.00214
50. Leonardi N, Van De Ville D. On spurious and real fluctuations of dynamic functional connectivity during rest. Neuroimage. (2015) 104:430–6. doi: 10.1016/j.neuroimage.2014.09.007
51. Han S, Cui Q, Wang X, Li L, Li D, He Z, et al. Resting state functional network switching rate is differently altered in bipolar disorder and major depressive disorder. Hum Brain Mapp. (2020) 41:3295–304. doi: 10.1002/hbm.25017
52. Song XW, Dong ZY, Long XY, Li SF, Zuo XN, Zhu CZ, et al. REST: a toolkit for resting-state functional magnetic resonance imaging data processing. PLoS ONE. (2011) 6:e25031. doi: 10.1371/journal.pone.0025031
53. Habas C, Manto M, Cabaraux P. The cerebellar thalamus. Cerebellum. (2019) 18:635–48. doi: 10.1007/s12311-019-01019-3
54. Pessoa L. On the relationship between emotion and cognition. Nat Rev Neurosci. (2008) 9:148–58. doi: 10.1038/nrn2317
55. Andreasen NC, Paradiso S, O'Leary DS. “Cognitive dysmetria” as an integrative theory of schizophrenia: a dysfunction in cortical-subcortical-cerebellar circuitry? Schizophr Bull. (1998) 24:203–18. doi: 10.1093/oxfordjournals.schbul.a033321
56. Guo W, Liu F, Chen J, Wu R, Zhang Z, Yu M, et al. Resting-state cerebellar-cerebral networks are differently affected in first-episode, drug-naive schizophrenia patients and unaffected siblings. Sci Rep. (2015) 5:17275. doi: 10.1038/srep17275
57. Hamoda HM, Makhlouf AT, Fitzsimmons J, Rathi Y, Makris N, Mesholam-Gately RI, et al. Abnormalities in thalamo-cortical connections in patients with first-episode schizophrenia: a two-tensor tractography study. Brain Imaging Behav. (2019) 13:472–81. doi: 10.1007/s11682-018-9862-8
58. Rasser PE, Schall U, Peck G, Cohen M, Johnston P, Khoo K, et al. Cerebellar grey matter deficits in first-episode schizophrenia mapped using cortical pattern matching. Neuroimage. (2010) 53:1175–80. doi: 10.1016/j.neuroimage.2010.07.018
59. Brady RO Jr, Gonsalvez I, Lee I, Öngür D, Seidman LJ, Schmahmann JD, et al. Cerebellar-Prefrontal Network Connectivity and Negative Symptoms in Schizophrenia. Am J Psychiatry. (2019) 176:512–20. doi: 10.1176/appi.ajp.2018.18040429
60. Dirkx MF, den Ouden HE, Aarts E, Timmer MH, Bloem BR, Toni I, et al. Dopamine controls Parkinson's tremor by inhibiting the cerebellar thalamus. Brain. (2017) 140:721–34. doi: 10.1093/brain/aww331
61. Parker KL, Kim YC, Kelley RM, Nessler AJ, Chen KH, Muller-Ewald VA, et al. Delta-frequency stimulation of cerebellar projections can compensate for schizophrenia-related medial frontal dysfunction. Mol Psychiatry. (2017) 22:647–55. doi: 10.1038/mp.2017.50
62. Middleton FA, Strick PL. Cerebellar output channels. Int Rev Neurobiol. (1997) 41:61–82. doi: 10.1016/S0074-7742(08)60347-5
63. Dum RP, Strick PL. An unfolded map of the cerebellar dentate nucleus and its projections to the cerebral cortex. J Neurophysiol. (2003) 89:634–9. doi: 10.1152/jn.00626.2002
64. Kelly RM, Strick PL. Cerebellar loops with motor cortex and prefrontal cortex of a nonhuman primate. J Neurosci. (2003) 23:8432–44. doi: 10.1523/JNEUROSCI.23-23-08432.2003
65. Middleton FA, Strick PL. Cerebellar projections to the prefrontal cortex of the primate. J Neurosci. (2001) 21:700–12. doi: 10.1523/JNEUROSCI.21-02-00700.2001
66. Schmahmann JD. An emerging concept. The cerebellar contribution to higher function. Arch Neurol. (1991) 48:1178–87. doi: 10.1001/archneur.1991.00530230086029
67. Annoni JM, Ptak R, Caldara-Schnetzer AS, Khateb A, Pollermann BZ. Decoupling of autonomic and cognitive emotional reactions after cerebellar stroke. Ann Neurol. (2003) 53:654–8. doi: 10.1002/ana.10549
68. Schmahmann JD. Disorders of the cerebellum: ataxia, dysmetria of thought, and the cerebellar cognitive affective syndrome. J Neuropsychiatry Clin Neurosci. (2004) 16:367–78. doi: 10.1176/jnp.16.3.367
69. Adamaszek M, D'Agata F, Ferrucci R, Habas C, Keulen S, Kirkby KC, et al. Consensus paper: cerebellum and emotion. Cerebellum. (2017) 16:552–76. doi: 10.1007/s12311-016-0815-8
70. Andreasen NC, O'Leary DS, Cizadlo T, Arndt S, Rezai K, Ponto LL, et al. Schizophrenia and cognitive dysmetria: a positron-emission tomography study of dysfunctional prefrontal-thalamic-cerebellar circuitry. Proc Natl Acad Sci USA. (1996) 93:9985–90. doi: 10.1073/pnas.93.18.9985
71. Andreasen NC, Pierson R. The role of the cerebellum in schizophrenia. Biol Psychiatry. (2008) 64:81–8. doi: 10.1016/j.biopsych.2008.01.003
72. Sokolov AA, Miall RC, Ivry RB. The cerebellum: adaptive prediction for movement and cognition. Trends Cogn Sci. (2017) 21:313–32. doi: 10.1016/j.tics.2017.02.005
73. Lupo M, Olivito G, Siciliano L, Masciullo M, Bozzali M, Molinari M, et al. Development of a psychiatric disorder linked to cerebellar lesions. Cerebellum. (2018) 17:438–46. doi: 10.1007/s12311-018-0926-5
74. Peng J, Liu J, Nie B, Li Y, Shan B, Wang G, et al. Cerebral and cerebellar gray matter reduction in first-episode patients with major depressive disorder: a voxel-based morphometry study. Eur J Radiol. (2011) 80:395–9. doi: 10.1016/j.ejrad.2010.04.006
75. Redlich R, Almeida JJ, Grotegerd D, Opel N, Kugel H, Heindel W, et al. Brain morphometric biomarkers distinguishing unipolar and bipolar depression. A voxel-based morphometry-pattern classification approach. JAMA Psychiatry. (2014) 71:1222–30. doi: 10.1001/jamapsychiatry.2014.1100
76. Su L, Cai Y, Xu Y, Dutt A, Shi S, Bramon E. Cerebral metabolism in major depressive disorder: a voxel-based meta-analysis of positron emission tomography studies. BMC Psychiatry. (2014) 14:321. doi: 10.1186/s12888-014-0321-9
77. Zhao L, Wang Y, Jia Y, Zhong S, Sun Y, Zhou Z, et al. Cerebellar microstructural abnormalities in bipolar depression and unipolar depression: a diffusion kurtosis and perfusion imaging study. J Affect Disord. (2016) 195:21–31. doi: 10.1016/j.jad.2016.01.042
78. Chen G, Zhao L, Jia Y, Zhong S, Chen F, Luo X, et al. Abnormal cerebellum-DMN regions connectivity in unmedicated bipolar II disorder. J Affect Disord. (2019) 243:441–7. doi: 10.1016/j.jad.2018.09.076
79. Cheng C, Dong D, Jiang Y, Ming Q, Zhong X, Sun X, et al. State-related alterations of spontaneous neural activity in current and remitted depression revealed by resting-state fMRI. Front Psychol. (2019) 10:245. doi: 10.3389/fpsyg.2019.00245
80. Emam H, Steffens DC, Pearlson GD, Wang L. Increased ventromedial prefrontal cortex activity and connectivity predict poor sertraline treatment outcome in late-life depression. Int J Geriatr Psychiatry. (2019) 34:730–7. doi: 10.1002/gps.5079
81. Lupo M, Siciliano L, Leggio M. From cerebellar alterations to mood disorders: a systematic review. Neurosci Biobehav Rev. (2019) 103:21–8. doi: 10.1016/j.neubiorev.2019.06.008
82. Wang J, Zhang JR, Zang YF, Wu T. Consistent decreased activity in the putamen in Parkinson's disease: a meta-analysis and an independent validation of resting-state fMRI. Gigascience. (2018) 7:giy071. doi: 10.1093/gigascience/giy071
83. Wang Y, Wang J, Jia Y, Zhong S, Zhong M, Sun Y, et al. Topologically convergent and divergent functional connectivity patterns in unmedicated unipolar depression and bipolar disorder. Transl Psychiatry. (2017) 7:e1165. doi: 10.1038/tp.2017.117
84. Wang Y, Zhong S, Jia Y, Sun Y, Wang B, Liu T, et al. Disrupted resting-state functional connectivity in nonmedicated bipolar disorder. Radiology. (2016) 280:529–36. doi: 10.1148/radiol.2016151641
85. Gong J, Wang J, Qiu S, Chen P, Luo Z, Wang J, et al. Common and distinct patterns of intrinsic brain activity alterations in major depression and bipolar disorder: voxel-based meta-analysis. Transl Psychiatry. (2020) 10:353. doi: 10.1038/s41398-020-01036-5
86. Heath RG. Modulation of emotion with a brain pacemamer. Treatment for intractable psychiatric illness. J Nerv Ment Dis. (1977) 165:300–17. doi: 10.1097/00005053-197711000-00002
87. Schmahmann JD. From movement to thought: anatomic substrates of the cerebellar contribution to cognitive processing. Hum Brain Mapp. (1996) 4:174–98.
88. Singer T, Seymour B, O'Doherty J, Kaube H, Dolan RJ, Frith CD. Empathy for pain involves the affective but not sensory components of pain. Science. (2004) 303:1157–62. doi: 10.1126/science.1093535
89. Stoodley CJ, Schmahmann JD. Evidence for topographic organization in the cerebellum of motor control versus cognitive and affective processing. Cortex. (2010) 46:831–44. doi: 10.1016/j.cortex.2009.11.008
90. Yamamura T, Okamoto Y, Okada G, Takaishi Y, Takamura M, Mantani A, et al. Association of thalamic hyperactivity with treatment-resistant depression and poor response in early treatment for major depression: a resting-state fMRI study using fractional amplitude of low-frequency fluctuations. Transl Psychiatry. (2016) 6:e754. doi: 10.1038/tp.2016.18
91. Webb CA, Weber M, Mundy EA, Killgore WD. Reduced gray matter volume in the anterior cingulate, orbitofrontal cortex and thalamus as a function of mild depressive symptoms: a voxel-based morphometric analysis. Psychol Med. (2014) 44:2833–43. doi: 10.1017/S0033291714000348
92. Bisecco A, Rocca MA, Pagani E, Mancini L, Enzinger C, Gallo A, et al. Connectivity-based parcellation of the thalamus in multiple sclerosis and its implications for cognitive impairment: a multicenter study. Hum Brain Mapp. (2015) 36:2809–25. doi: 10.1002/hbm.22809
93. Houtchens MK, Benedict RH, Killiany R, Sharma J, Jaisani Z, Singh B, et al. Thalamic atrophy and cognition in multiple sclerosis. Neurology. (2007) 69:1213–23. doi: 10.1212/01.wnl.0000276992.17011.b5
94. Koenig KA, Rao SM, Lowe MJ, Lin J, Sakaie KE, Stone L, et al. The role of the thalamus and hippocampus in episodic memory performance in patients with multiple sclerosis. Mult Scler. (2019) 25:574–84. doi: 10.1177/1352458518760716
95. Schoonheim MM, Hulst HE, Brandt RB, Strik M, Wink AM, Uitdehaag BM, et al. Thalamus structure and function determine severity of cognitive impairment in multiple sclerosis. Neurology. (2015) 84:776–83. doi: 10.1212/WNL.0000000000001285
96. Tewarie P, Schoonheim MM, Schouten DI, Polman CH, Balk LJ, Uitdehaag BM, et al. Functional brain networks: linking thalamic atrophy to clinical disability in multiple sclerosis, a multimodal fMRI and MEG study. Hum Brain Mapp. (2015) 36:603–18. doi: 10.1002/hbm.22650
97. Disner SG, Beevers CG, Haigh EA, Beck AT. Neural mechanisms of the cognitive model of depression. Nat Rev Neurosci. (2011) 12:467–77. doi: 10.1038/nrn3027
98. Mayberg HS. Limbic-cortical dysregulation: a proposed model of depression. J Neuropsychiatry Clin Neurosci. (1997) 9:471–81. doi: 10.1176/jnp.9.3.471
99. Burt T, Lisanby SH, Sackeim HA. Neuropsychiatric applications of transcranial magnetic stimulation: a meta analysis. Int J Neuropsychopharmacol. (2002) 5:73–103. doi: 10.1017/S1461145702002791
100. Davidson RJ, Irwin W, Anderle MJ, Kalin NH. The neural substrates of affective processing in depressed patients treated with venlafaxine. Am J Psychiatry. (2003) 160:64–75. doi: 10.1176/appi.ajp.160.1.64
101. Fahim C, Stip E, Mancini-Marie A, Mensour B, Leroux JM, Beaudoin G, et al. Abnormal prefrontal and anterior cingulate activation in major depressive disorder during episodic memory encoding of sad stimuli. Brain Cogn. (2004) 54:161–3.
102. Gershon AA, Dannon PN, Grunhaus L. Transcranial magnetic stimulation in the treatment of depression. Am J Psychiatry. (2003) 160:835–45. doi: 10.1176/appi.ajp.160.5.835
103. Keedwell PA, Andrew C, Williams SC, Brammer MJ, Phillips ML. The neural correlates of anhedonia in major depressive disorder. Biol Psychiatry. (2005) 58:843–53. doi: 10.1016/j.biopsych.2005.05.019
104. Lawrence NS, Williams AM, Surguladze S, Giampietro V, Brammer MJ, Andrew C, et al. Subcortical and ventral prefrontal cortical neural responses to facial expressions distinguish patients with bipolar disorder and major depression. Biol Psychiatry. (2004) 55:578–87. doi: 10.1016/j.biopsych.2003.11.017
105. Mayberg HS. Modulating dysfunctional limbic-cortical circuits in depression: towards development of brain-based algorithms for diagnosis and optimised treatment. Br Med Bull. (2003) 65:193–207. doi: 10.1093/bmb/65.1.193
106. Phillips ML, Drevets WC, Rauch SL, Lane R. Neurobiology of emotion perception II: implications for major psychiatric disorders. Biol Psychiatry. (2003) 54:515–28. doi: 10.1016/S0006-3223(03)00171-9
107. Fitzgerald PB, Oxley TJ, Laird AR, Kulkarni J, Egan GF, Daskalakis ZJ. An analysis of functional neuroimaging studies of dorsolateral prefrontal cortical activity in depression. Psychiatry Res. (2006) 148:33–45. doi: 10.1016/j.pscychresns.2006.04.006
108. Korgaonkar MS, Grieve SM, Etkin A, Koslow SH, Williams LM. Using standardized fMRI protocols to identify patterns of prefrontal circuit dysregulation that are common and specific to cognitive and emotional tasks in major depressive disorder: first wave results from the iSPOT-D study. Neuropsychopharmacology. (2013) 38:863–71. doi: 10.1038/npp.2012.252
109. Zhong X, Pu W, Yao S. Functional alterations of fronto-limbic circuit and default mode network systems in first-episode, drug-naïve patients with major depressive disorder: a meta-analysis of resting-state fMRI data. J Affect Disord. (2016) 206:280–6. doi: 10.1016/j.jad.2016.09.005
110. Desseilles M, Balteau E, Sterpenich V, Dang-Vu TT, Darsaud A, Vandewalle G, et al. Abnormal neural filtering of irrelevant visual information in depression. J Neurosci. (2009) 29:1395–403. doi: 10.1523/JNEUROSCI.3341-08.2009
111. Desseilles M, Schwartz S, Dang-Vu TT, Sterpenich V, Ansseau M, Maquet P, et al. Depression alters “top-down” visual attention: a dynamic causal modeling comparison between depressed and healthy subjects. Neuroimage. (2011) 54:1662–8. doi: 10.1016/j.neuroimage.2010.08.061
112. Furey ML, Drevets WC, Hoffman EM, Frankel E, Speer AM, Zarate CA Jr. Potential of pretreatment neural activity in the visual cortex during emotional processing to predict treatment response to scopolamine in major depressive disorder. JAMA Psychiatry. (2013) 70:280–90. doi: 10.1001/2013.jamapsychiatry.60
113. Costafreda SG, Khanna A, Mourao-Miranda J, Fu CH. Neural correlates of sad faces predict clinical remission to cognitive behavioural therapy in depression. Neuroreport. (2009) 20:637–41. doi: 10.1097/WNR.0b013e3283294159
114. Fu CH, Williams SC, Cleare AJ, Scott J, Mitterschiffthaler MT, Walsh ND, et al. Neural responses to sad facial expressions in major depression following cognitive behavioral therapy. Biol Psychiatry. (2008) 64:505–12. doi: 10.1016/j.biopsych.2008.04.033
115. Han X, Berg AC, Oh H, Samaras D, Leung HC. Multi-voxel pattern analysis of selective representation of visual working memory in ventral temporal and occipital regions. Neuroimage. (2013) 73:8–15. doi: 10.1016/j.neuroimage.2013.01.055
116. Harrison SA, Tong F. Decoding reveals the contents of visual working memory in early visual areas. Nature. (2009) 458:632–5. doi: 10.1038/nature07832
117. Suslow T, Konrad C, Kugel H, Rumstadt D, Zwitserlood P, Schöning S, et al. Automatic mood-congruent amygdala responses to masked facial expressions in major depression. Biol Psychiatry. (2010) 67:155–60. doi: 10.1016/j.biopsych.2009.07.023
Keywords: major depressive disorder, amplitude of low-frequency fluctuations, dynamics, intrinsic brain activity, resting-state fMRI
Citation: Zheng R, Chen Y, Jiang Y, Wen M, Zhou B, Li S, Wei Y, Yang Z, Wang C, Cheng J, Zhang Y and Han S (2021) Dynamic Altered Amplitude of Low-Frequency Fluctuations in Patients With Major Depressive Disorder. Front. Psychiatry 12:683610. doi: 10.3389/fpsyt.2021.683610
Received: 29 March 2021; Accepted: 14 June 2021;
Published: 19 July 2021.
Edited by:
Chien-Han Lai, National Yang-Ming University, TaiwanReviewed by:
Feng Liu, Tianjin Medical University General Hospital, ChinaCopyright © 2021 Zheng, Chen, Jiang, Wen, Zhou, Li, Wei, Yang, Wang, Cheng, Zhang and Han. This is an open-access article distributed under the terms of the Creative Commons Attribution License (CC BY). The use, distribution or reproduction in other forums is permitted, provided the original author(s) and the copyright owner(s) are credited and that the original publication in this journal is cited, in accordance with accepted academic practice. No use, distribution or reproduction is permitted which does not comply with these terms.
*Correspondence: Jingliang Cheng, ZmNjY2hlbmdqbEB6enUuZWR1LmNu; Yong Zhang, enp1emhhbmd5b25nMjAxM0AxNjMuY29t; Shaoqiang Han, c2hhb3FpYW5naGFuQDE2My5jb20=
†These authors have contributed equally to this work
Disclaimer: All claims expressed in this article are solely those of the authors and do not necessarily represent those of their affiliated organizations, or those of the publisher, the editors and the reviewers. Any product that may be evaluated in this article or claim that may be made by its manufacturer is not guaranteed or endorsed by the publisher.
Research integrity at Frontiers
Learn more about the work of our research integrity team to safeguard the quality of each article we publish.