- 1Psychiatric Laboratory and Mental Health Center, West China Hospital of Sichuan University, Chengdu, China
- 2West China Brain Research Center, West China Hospital of Sichuan University, Chengdu, China
- 3College of Foreign Languages and Cultures, Sichuan University, Chengdu, China
Background: Major depressive disorder (MDD) is a common disease which is complicated by metabolic disorder. Although MDD has been studied relatively intensively, its metabolism is yet to be elucidated.
Methods: To profile the global pathophysiological processes of MDD patients, we used metabolomics to identify differential metabolites and applied a new database Metabolite set enrichment analysis (MSEA) to discover dysfunctions of metabolic pathways of this disease. Hydrophilic metabolomics were applied to identify metabolites by profiling the plasma from 55 MDD patients and 100 sex-, gender-, BMI-matched healthy controls. The metabolites were then analyzed in MSEA in an attempt to discover different metabolic pathways. To investigate dysregulated pathways, we further divided MDD patients into two cohorts: (1) MDD patients with anxiety symptoms and (2) MDD patients without anxiety symptoms.
Results: Metabolites which were hit in those pathways correlated with depressive and anxiety symptoms. Altogether, 17 metabolic pathways were enriched in MDD patients, and 23 metabolites were hit in those pathways. Three metabolic pathways were enriched in MDD patients without anxiety, including glycine and serine metabolism, arginine and proline metabolism, and phenylalanine and tyrosine metabolism. In addition, L-glutamic acid was positively correlated with the severity of depression and retardation if hit in MDD patients without anxiety symptoms.
Conclusions: Different kinds of metabolic pathophysiological processes were found in MDD patients. Disorder of glycine and serine metabolism was observed in both MDD patients with anxiety and those without.
Introduction
Major depressive disorder (MDD) has been the leading cause of disability globally, affecting over one tenth of the world's population (1, 2). Even today, however, it is still challenging for us to assess MDD patients using an objective and established diagnostic method (3) due to huge heterogeneity in both clinical details and etiopathology of this disease (4). Unfortunately, failure in early diagnosis and treatment of MDD can lead to a higher rate of suicide and relapse (5). Therefore, it is essential to investigate the potential biomarkers and pathophysiological progress of MDD.
Former researches identifying biomarkers of MDD have indicated that metabolic disturbances may be correlated with the pathophysiology of MDD (6). Promising results from a study on drug-naïve patients with MDD indicated that their glucose levels had already been much higher than those of healthy controls when first diagnosed, and researchers concluded that the dysfunction of glucometabolic may result from MDD through hypercortisolemia (7). Accumulating evidence suggested that hypothalamic-pituitary-adrenal (HPA)-axis may play a critical role in the onset of MDD, and the disorder of this axis may also contribute to metabolic syndromes in depressive individuals (8). Some previous study had indicated the disturbance of amino acid metabolism in MDD, including high levels of glutamate, lysine, aspartate, serine, and so on (9). Moreover, a number of metabolic disturbances have been reported. The dysfunction of tryptophan pathway, kynurenine pathway, and more pathways (10–12), except classic metabolic pathways of neurotransmitters (13), was proved to be associated with MDD. Nevertheless, until now, findings on metabolic dysfunction in MDD are not consistent for many reasons such as pleomorphism of this disease and defects in methodological sources (14–16). Therefore, the existing evidences are hardly applicable to investigating the function of metabolic disorder in the MDD pathogenesis.
MDD patients may also have symptoms of anxiety (17, 18), which predict a longer disease course, higher level of depression, greater risk of suicide, and more possibility of treatment nonresponse (19–21). Therefore, it is necessary to investigate the difference of pathophysiological progress between MDD patients with anxiety symptoms (MDD-A) and MDD patients without anxiety symptoms (MDD-T). Previous researches had indicated that MDD patients with anxiety symptoms were more liable to present metabolic dysfunction. Data from a large, community-based study showed that a group of patients who had severe depression and anxiety symptoms showed higher HbA1c levels and a greater need for insulin than those who showed no anxiety symptoms (22). In addition, another research showed that, in MDD patients, the elevated thyroid peroxidase levels indicated a higher level of anxiety symptoms (23). Therefore, the metabolic difference between MDD patients with and without anxiety symptoms should be further studied.
Metabolomics, which has been defined as the quantitative detection method of the global metabolic response of multiple living systems to environmental influence or other changes in the body, has recently been widely used to investigate the potential pathophysiological mechanisms (24). A majority of researchers focused on the application of metabolomics to mental illnesses studies have identified potential diagnostic biomarkers for MDD (25). In a systematic review focusing on the development of metabolomics in affective disorders showed that over 249 metabolites were found dysregulated in MDD. Moreover, almost half of those metabolites were reported in more than two studies (26). However, the search for biomarkers has been hindered by many reasons such as methodological differences (14). Studies suggested diagnostic systems such as metabolic set may contribute to better diagnostic efficacy (13).
Enrichment analysis and multivariate method have been widely used in omics researches, and omics-related databases, such as KEGG and DAVID, are built to identify pathways. Nevertheless, these databases are mainly used in genetic research (16); enrichment analysis in metabolomics research needs more professional databases. Metabolite set enrichment analysis (MSEA) has enabled researchers to identify and interpret patterns of mammalian (mainly human) metabolite concentration alterations in a biological context (27). Quantitative enrichment analysis (QEA), which is one of analytic methods of MSEA, can help provide information on pathways with global compounds that are identified when even just one of the compounds is significantly changed or when multi compounds are only slightly but consistently and exactly altered. Hence, more metabolic information could be kept (28).
In the present research, we applied liquid chromatography-mass spectroscopy (LC-MS) to identify plasma metabolites in MDD and healthy controls (HCs), and used MSEA to analyze the quantities of metabolic data in MDD.
Materials and Methods
Participants
This study recruited 155 right-handed participants from the Mental Health Center of West China Hospital, Sichuan University, consisting of 55 MDD patients and 100 sex-, gender-, and BMI-matched healthy controls (HCs). Permission was granted by West China Hospital of Sichuan University. All procedures in this research were designed and carried out in accordance with the guidelines issued by the Ethical Committee of Sichuan University. And all assessments were carried out after the participants and their legal guardians signed the informed consent forms. All patients were diagnosed as having major depressive disorder (MDD) according to the Diagnostic and Statistical Manual of Mental Disorders, Fourth Edition (DSM-IV). Then, we assessed the severity of depression and of anxiety using the 17-item Hamilton Depression Rating Scale (HAMD-17) and the 14-item Hamilton Anxiety rating Scale (HAMA-14), respectively. The total scores of HAMD-17 in all MDD patients involved in the present study were above 17. Depressive symptoms were then evaluated in five dimensions of HAMD, namely, anxiety, weight, cognition, retardant, and sleep disorder. Two dimensions of HAMA were also evaluated, namely, somatic anxiety and psychological anxiety (29, 30). Participants were excluded if they had (1) endocrine diseases, metabolic disorders, or receiving hormone medication; (2) any serious physical diseases; (3) other psychiatric disorders, such as dementia, schizophrenia, and substance abuse; (4) obvious psychosocial factors; or (5) any psychotropic medications during the past 12 weeks.
Plasma Sample Detection
We collected venous blood of recruited participants in anticoagulated EDTA tubes on their first day of taking part in our research. Venipuncture for blood was set at 10:00 am or 4:00 pm. Plasma samples were stored at −80°C within 1 h after collection.
Then, liquid nitrogen was used to ground plasma samples individually; the homogenate was resuspended by being well vertexed with pre-chilled methanol as well as 0.1% formic acid. During the following 5 min, the samples were incubated on ice to keep the temperature low, and were then centrifuged at 15,000 rpm, 4°C. After that, liquid chromatography-mass spectroscopy (LC-MS) grade water was applied to some of the supernatant which had been diluted to the final concentration that contained 60% methanol. Subsequently, the samples were transferred to a fresh Eppendorf tube with 0.22 μm filter and were centrifuged at 15,000 g, 4°C for 10 min. The LC-MS/MS system analysis was injected with the filtrate. Finally, LC-MS/MS analyses were performed using a Vanquish UHPLC system (Thermo Fisher) as well as an Orbitrap Q Exactive HF-X mass spectrometer (Thermo Fisher). By using the 16-min linear gradient at a flow rate of 0.2 ml/min, we injected the plasma of participants onto a Hyperil Gold column (100 × 2.1 mm, 1.9 μm). Both eluent A (0.1% FA in Water) and methanol B were the positive polarity mode of the eluents. The solvent gradient was set as follows: 2% B for 12.0 min; 100% B for 14.0 min; 100–2% B for 1.5 min; 2–100% B for 14.1 min; and 2% B for 16 min. Then, we operated Q Exactive HF-X mass spectrometer in bipolarity modes with the help of capillary temperature at the temperature of 320°C, spray voltage of 3.2 kV, aux gas flow rate of 10 arb., and sheath gas flow rate of 35 arb. We processed the raw data files generated by UHPLC-MS/MS using the Compound Discoverer 3.0 for to detect and quantify performance peak. We then set the main parameters, including actual mass tolerance, minimum intensity, retention time tolerance, signal/noise ratio, and signal intensity tolerance. Following this step, we normalized peak intensities to the total spectral intensity. To forecast the molecular formula based on additive ions, normalized data on fragment ions and molecular ion peaks were used. During metabolite detection, QC samples, which were equally mixed experimental samples, were used to balance LC-MS system and monitor the status of the instrument.
Finally, the raw data files generated by LC-MS system were processed using the Compound Discoverer 3.0 (CD 3.0, Thermo Fisher) to perform peak alignment, peak picking, and quantitation for each metabolite. The main parameters were set as follows: retention time tolerance, 0.2 min; actual mass tolerance, 5 ppm; signal intensity tolerance, 30%; signal/noise ratio, 3; and minimum intensity, 100,000. After that, peak intensities were normalized to the total spectral intensity. The normalized data were used to predict the molecular formula bases on additive ions, molecular ion peaks, and fragment ions. Then, peaks were matched with the mzCloud (https://www.mzcloud.org/) and ChemSpider (http://www.chemspider.com/) database to obtain the accurate qualitative and relative quantitative outcomes.
Statistical Analysis
First, to check inter-batch bias during metabolite detection, QC samples were evaluated using principal component analysis (PCA). Then, to alleviate the influence of environment, metabolites were identified using the human metabolome database (HMDB), and those that could be found in endogenous human bodies were included. The endogenous metabolites were normalized for subsequent analyses using log transformation and auto scaling, and SIMCAP+ 14.0 software was used for further analyses. The samples were used to build PCA model, and then outliers in these samples were eliminated. After that, the remaining samples were used to build the partial least squares-discriminant analysis model (PLS-DA), which was applied to explore the metabolic differences between HCs and depressive patients (31). Meanwhile, the samples of patients with and without anxiety symptoms were used to explore metabolic differences between MDD patients and HCs. Finally, to identify the possible biological functions of these metabolites responsible for MDD, metabolite set enrichment analysis (MSEA) was used to analyze the metabolic pathways in the online software MetaboAnalyst 5.0. P-value was set at <0.05 for metabolites resulting from Bonferroni method (HOLM), and the number of hits ≥2 was deemed as greatly enriched and being able to identify biological processes that warranted further investigation (32). Metabolites found in pathways of MDD with or without anxiety symptoms and dimensions found in HAMD and HAMA were analyzed respectively using spearman's rank correlation (33).
Results
Clinical Information of Participants
This study included 55 MDD patients and 100 healthy controls (HCs), and no demographic difference was observed between them. The MDD group was further divided in to two subgroups based on their total scores of HAMA: MDD with anxiety symptoms (n = 35, HAMA scores equal to or higher than 14) and MDD without anxiety symptoms (n = 20). No difference in demography was observed between these two subgroups, either. Demographic and clinical characteristics of all participants were summarized (Table 1 and Supplementary Table 1).
Metabolite Overview
Altogether, 822 metabolites were found in the raw data using LC-MS detection. The PCA plot of QC samples showed that, during the whole test process, the LC-MS system was stable and convincing (Supplementary Figure 1). Then, HMDB database was used to identify the source of the metabolites, excluding those that could only be found in the natural environment. A total of 222 endogenous metabolites were identified for further analyses (Supplementary Table 2). After log transformation and auto scaling processing, Kolmogorov-Smirnov test was used to assess the normality of the processed data, over half of which did not follow a normal distribution. In the PCA model of all samples, five outliers were found, including three MDD samples and two HCs samples (Supplementary Figure 2).
MDD Samples vs. Healthy Controls
The PLS-DA plot score showed that MDD patients were clearly separated from HCs with little overlap (R2Y = 80%, Q2Y = 67%; Figure 1). To determine pathways in MDD patients different from those in HCs, MSEA analysis was performed, and then 17 pathways were significantly enriched in MDD patients (Table 2). In addition, 23 metabolites were hit in the pathways of MDD (Table 3).
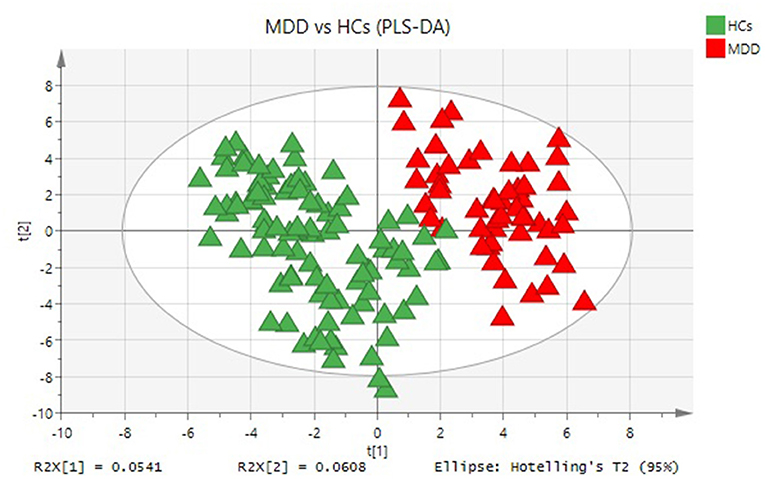
Figure 1. Metabolomic analysis of plasma samples from all participants. PLS-DA model indicates a significant difference between MDD (red triangle) and HCs (green triangle).
MDD Patients With and Without Anxiety Symptoms vs. HCs
The PLS-DA plot score also showed that metabolites we found could possibly distinguish anxious MDD patients, MDD patients lacking anxiety symptoms, and HCs (R2Y = 50%, Q2Y = 34%; Supplementary Figure 3). Then, using MESA analysis, 13 metabolic pathways were enriched in MDD patients with anxiety symptoms including ammonia recycling, amino sugar metabolism, glutamate metabolism, urea cycle, pyrimidine metabolism, Warburg effect, phenylacetate metabolism, nicotinate and nicotinamide metabolism, aspartate metabolism, fatty acid biosynthesis, purine metabolism, glycine and serine metabolism, and methionine metabolism (Supplementary Table 3A). Furthermore, we found 19 metabolites which were hit in those pathways of MDD (Supplementary Table 4A). In MDD patients without anxiety symptoms subgroup, only three metabolic pathways are significantly enriched including glycine and serine metabolism, arginine and proline metabolism, and phenylalanine and tyrosine metabolism (Supplementary Table 3B). Nine metabolites were hit in the pathways of MDD without anxiety symptoms (Supplementary Table 4B).
Spearman's Rank Correlation
The metabolites found individually in two groups of MDD patients were analyzed using spearman' rank correlation associated with symptoms. In anxious MDD patient cohort, an inverse correlation was determined between adenine and weight in HAMD (r:−0.398, p = 0.022), and a great and inverse correlation was determined between hypoxanthine and psychological anxiety (r: −0.377, p = 0.031). 2-Oxobutyric acid (r: 0.362, p = 0.038) had also been found positively correlated with retardation in HAMD. In addition, in non-anxious MDD group, the highest positive correlations were determined respectively in L-glutamic acid (r: 0.718, p = 0.0005) and pyruvic acid (r: 0.679, p = 0.001) with retardation in HAMD. The L-glutamic acid (r: 0.491, p = 0.033) also had positive correlation with the level of depression.
Discussion
In our study, the identified metabolic pathways were used to investigate the metabolic alterations in MDD individuals. Finally, 17 differential metabolic pathways were found in MDD group, and 23 metabolites were hit in those pathways. Dysfunction of glycine and serine metabolism was found in MDD patients in each subgroup, but dysfunction of arginine and proline metabolism and phenylalanine and tyrosine metabolism was only found in MDD patients without anxiety symptoms. In addition, pyruvic acid which was found in MDD patient group was related with the severity of depression. We noticed that L-glutamic acid, glutamine, and pyruvic acid had been hit in multiple pathways. Besides, L-glutamic acid in MDD patients lacking anxiety symptoms had correlation with retardation and the level of depression in HAMD.
In this research, we used quantitative enrichment analysis in MSEA to find metabolites and metabolic pathways that have biological functions. In previous studies, PCA, PLS-DA, and orthogonal least squares discriminant analysis (OPLS-DA) had been the most commonly used multivariate component methods in metabolomics analysis, but those approaches still had significant limitations (25). Those approaches usually focused on comparing the differences in metabolites production between different groups, focusing on a few significantly upregulated or downregulated metabolites, which tends to omit some metabolites with insignificant differentially produced but important biological significance. Nevertheless, QEA can help us find metabolic pathways with biological functions in diseases and metabolites with biological characteristics (34). In our study, the significantly biological changed L-glutamic acid, glutamine, pyruvic acid, and other metabolites were not found by the PLS-DA modal. However, quantitative enrichment analysis still viewed them as differential metabolites responsible for the discrimination between HCs and MDD patients, and metabolic pathways with biological functions were found. These results fully showed the advantage of QEA in finding more reproducible and more interpretable metabolites and pathways in untargeted metabolomics analysis (35).
Different kinds of metabolic pathophysiological processes were found in MDD patients. The 17 metabolite pathways that were identified in MDD group can be divided into four groups. Nine of the 17 pathways were associated with amino acid metabolism, 3 had correlation with nucleic acid metabolism, 3 had correlation with energy metabolism, 1 was related to lipid metabolism, and 1 was related to steroid metabolism. Previous studies showed that MDD had been a multifactorial disease, and environmental influence in MDD could not been ignored (36). For this reason, multidisciplinary theories, including neurotrophic alterations, neuroinflammation, neurovascular, and endocrine-immune system dysfunction, had attempted to explain the pathogenesis of MDD (37, 38). Our results are consistent with those previous studies. However, it is worth noticing that significantly disturbed amino acid metabolism was found in MDD individuals because most of the metabolic pathways are related to amino acid metabolism. Previous studies have mentioned that amino acids are important brain neurotransmitters (39), and they also showed that many amino acids may help the diagnosis of psychiatric diseases (39–41). In our study, we found the dysfunction of glycine and serine metabolism is consistent in MDD patients with or lacking anxiety symptoms.
Glycine or serine binding glutamate as co-agonist can help to activate N-methyl-D-aspartate receptors (NMDARs) (42), and the abnormality in NMDARs activity has been proven to result in both affective and cognitive disorder which is the core symptom of affective disorder by decreasing neuroplasticity (43). Reducing serine levels impairs NMDAR-mediated processes in hippocampus, prefrontal cortex, and amygdala and so on (44, 45), and these brain structures are closely related to mood disorders (46). Additionally, clinical studies demonstrated that ketamine, as a non-competitive NMDA receptor antagonist, has a rapid antidepressant response. Based on this, the new prodrug 4-Chlorokynurenine as a potent antagonist of NMDAR glycine agonist site was found to possess the potential of producing sustained and rapid antidepressant effect without the side effect profile of ketamine in MDD (47). Hence, NMDAR glycine site shows the potential to facilitate the development of antidepressant. However, the abnormality of NMDARs like NMDA/glycine-B site also contribute to anxiety symptoms. For instance, mutant mice that had reduction in NMDAR glycine affinity showed anxiety-like symptoms (48–50). Thus, we can conclude that the dyshomeostasis of glycine and serine metabolism can lead to both depressive and anxious symptoms.
The irregularity of arginine and proline metabolism was only found in the MDD group without anxiety symptoms. A double-blind, placebo-controlled crossover study on the mechanism of action of ketamine in depression clearly demonstrated that MDD patients had lower biological activity in arginine and proline metabolism, and that ketamine could modulate the bioavailability of arginine (51). Low level of arginine contributes to increased arginase activity which can lead to the severity of depression (52). Arginine-proline metabolism is connected with nitric oxide cycling (53). Arginine which is the precursor of proline is converted to nitric oxide and citrulline under the help of nitric oxide synthases (54). A recent research focusing on the influence factor of Alzheimer's disease indicated that NO is generated by NMDARS and is catalyzed neuronal nitric oxide synthase, and that high concentration of NO in brain is associated with the elevated IL-6 level and TNF-α which can also lead to cognitive disorder through the tau phosphorylation (55). Actually, our previous study on the relationship between the levels of inflammation cytokines and major depressive disorder had already found that MDD patients showed elevated IL-6 level (56). Therefore, arginine and proline metabolism may be involved in depressive symptoms by mediating inflammatory responses.
The disturbance of phenylalanine and tyrosine metabolism had been found in non-anxious MDD patients. Some previous study had indicated that MDD patients showed low levels of tyrosine, and after 4-week-treatment, tyrosine was significantly increased (57). Felger et al. (58) also found that compared with HCs, tyrosine metabolism was lower in MDD patients, and tyrosine metabolism was negatively associated with the level of C-reactive protein (CRP). They suggested that inflammation in patients could prevent the conversion of phenylalanine to tyrosine, and low level of tyrosine could induce the reduction of dopamine synthesis (59). A research focusing on the change of small RNA molecules in MDD indicated that the differentially expressed miRNAs in the brains of MDD patients could downregulate the expression of erb-b2 receptor tyrosine kinase 4, and influence phenylalanine and tyrosine metabolism (60).
Nevertheless, we did not find the disorder of arginine and proline metabolism, or phenylalanine and tyrosine metabolism in MDD patients with anxiety symptoms. We suspected that the anxiety symptoms may also impact arginine and proline metabolism in the opposite direction of MDD without anxiety symptoms.
We also noticed that L-glutamic acid, glutamine, and pyruvic acid had been hit in multiple pathways, indicating that these metabolites may be potential biomarker candidates for MDD. Higher level of glutamic acid was found in patients with mood disorders, compared with HCs and schizophrenia (61). Metabolic disorders in MDD patients could increase the burden of glutamate receptors in synapses, induce the release of glutamate in microglia cell, and reduce glutamate clearance, and thus interfering with glutamate transmission in the brain (62). Clinical studies have demonstrated that the abnormal L-glutamic acid and glutamine which are amino acid neurotransmitters in the brains of affective disorders' individuals contribute to changes in cortical excitability and inhibition (63, 64). Also, they had been discovered to influence energy metabolism as one of the tricarboxylic acids (TCA) cycle intermediates (65). Furthermore, in the study of relationship between metabolites and symptoms, we found a significantly high level of positive correlation between pyruvic acid and retardant/the level of depression in MDD. Pyruvate as the carboxylate anion of pyruvic acid is the end product of glycolysis and can further participate in TCA cycle which is the main process of energy metabolism (66). The low level of pyruvic acid had been found in MDD patients, and some studies suggested the interaction between amino acid metabolism and energy metabolism contributed to the onset of depression (67). However, even those metabolites may play an important role in MDD, we still lack evidence to validate a causal relationship between the discovered metabolites and MDD in this study. Further studies are needed to really raise a biomarker for MDD diagnosis.
Additionally, our study showed hypoxanthine was relatively associated with psychological anxiety, which suggested that the abnormality of purine metabolism and fatty acid metabolism may contribute to the severity of anxiety symptoms in MDD. A review of purinergic system changing in psychiatric diseases suggested that alterations in purine metabolism might be involved in anxiety disorder and depression because purinergic receptors are proved to be related to anxiety and depressive symptoms (68). A study on cell research implicated an important link between purine metabolic dysfunction in CD4+ T cells and anxiety-like behaviors (69). However, these results are mainly from rodent experiments. The instability of animal models and the differences between rodents and human beings may lead to these disagreements.
Despite the findings aforementioned, our study has several limitations. First, we did not include anxious patients lacking depressive symptoms; hence, the change of those pathways in this kind of patients was still unclear. Second, we did not control the fasting time of the enrolled participants. Although the interference of exogenous metabolites was excluded, our results still need further validation. Third, plasma was the only compartment used in this study to verify the identified pathways. Other kinds of samples, especially the cerebrospinal fluid, should be applied to validate our results. The phases of menstrual cycle in female participants were not taken into account in our study, but the influence of the cycle should also be noticed. Finally, we did not have a testing set to validate our results. Therefore, future researches should take those factors into consideration when identifying the potential pathways involved in major depressive disorder.
In conclusion, amino acid metabolism plays a key role in the pathophysiology of depression. The dysfunction of glycine and serine metabolism which is consistent in MDD patients with or lacking anxiety symptoms needs further investigation. Furthermore, the interaction between amino acid metabolism and energy metabolism may contribute to changes in the brains of MDD patients.
Conclusions
In conclusion, different kinds of metabolic pathophysiological processes were found in MDD patients. The dysfunction of arginine and proline metabolism was observed only in MDD without anxiety symptoms, but the irregular of glycine and serine metabolism was found in both MDD with and without anxiety symptoms.
Data Availability Statement
The original contributions presented in the study are included in the article/Supplementary Material, further inquiries can be directed to the corresponding author/s.
Ethics Statement
The studies involving human participants were reviewed and approved by the Ethical Committee of Sichuan University. Written informed consent to participate in this study was provided by the participants' legal guardian/next of kin. Written informed consent was obtained from the individual(s), and minor(s)' legal guardian/next of kin, for the publication of any potentially identifiable images or data included in this article.
Author Contributions
All authors have made significant scientific contributions to this manuscript. YD, XM, JW, and TL conceived and designed the experiments. YD, JW, ZZ, TL, XY, MW, YW, XQ, LZ, WG, QW, WD, ML, and XM performed the experiments. YD, JW, and ZZ analyzed the data. YD wrote the manuscript, and XM and DL reviewed the manuscript.
Funding
We received funds from the National Natural Science Foundation of China (Grant No.: 81671344), Major International (Regional) Joint Research Project from National Natural Science Foundation of China (Grant No.: 81920108018), National Natural Science Foundation of China (Nos.: 82001432 and 81671344), China Postdoctoral Science Foundation (Nos.: 2020TQ0213 and 2020M683319), the 1.3.5 Project for Disciplines of Excellence, Special Foundation for Brain Research from Science and Technology Program of Guangdong (Grant No.: 2018B030334001), and West China Hospital of Sichuan University (Grant Nos.: ZY2016103 and ZY2016203) to support this study.
Conflict of Interest
The authors declare that the research was conducted in the absence of any commercial or financial relationships that could be construed as a potential conflict of interest.
Publisher's Note
All claims expressed in this article are solely those of the authors and do not necessarily represent those of their affiliated organizations, or those of the publisher, the editors and the reviewers. Any product that may be evaluated in this article, or claim that may be made by its manufacturer, is not guaranteed or endorsed by the publisher.
Acknowledgments
The phenomenal contribution of participants is acknowledged by all authors of this study.
Supplementary Material
The Supplementary Material for this article can be found online at: https://www.frontiersin.org/articles/10.3389/fpsyt.2021.644555/full#supplementary-material
References
1. Belmaker RH, Agam G. Major depressive disorder. N Engl J Med. (2008) 358:55–68. doi: 10.1056/NEJMra073096
2. Murray CJ, Lopez AD. Global mortality, disability, and the contribution of risk factors: Global Burden of Disease Study. Lancet. (1997) 349:1436–42. doi: 10.1016/S0140-6736(96)07495-8
3. Schmidt HD, Shelton RC, Duman RS. Functional biomarkers of depression: diagnosis, treatment, and pathophysiology. Neuropsychopharmacology. (2011) 36:2375–94. doi: 10.1038/npp.2011.151
4. Ostergaard SD, Jensen SOW, Bech P. The heterogeneity of the depressive syndrome: when numbers get serious. Acta Psychiat Scand. (2011) 124:495–6. doi: 10.1111/j.1600-0447.2011.01744.x
5. Kraus C, Kadriu B, Lanzenberger R, Zarate CA Jr, Kasper S Prognosis and improved outcomes in major depression: a review. Transl Psychiatry. (2019) 9:127. doi: 10.1038/s41398-019-0460-3
6. Ma YJ, Wang DF, Yuan M, Zhang XJ, Long J, Chen SB, et al. The prevalence, metabolic disturbances and clinical correlates of recent suicide attempts in Chinese inpatients with major depressive disorder. BMC Psychiatry. (2019) 19:144. doi: 10.1186/s12888-019-2131-6
7. Garcia-Rizo C, Kirkpatrick B, Fernandez-Egea E, Oliveira C, Bernardo M. Abnormal glycemic homeostasis at the onset of serious mental illnesses: A common pathway. Psychoneuroendocrinology. (2016) 67:70–5. doi: 10.1016/j.psyneuen.2016.02.001
8. Penninx B, Lange SMM. Metabolic syndrome in psychiatric patients: overview, mechanisms, and implications. Dialogues Clin Neurosci. (2018) 20:63–73. doi: 10.31887/DCNS.2018.20.1/bpenninx
9. Mauri MC, Ferrara A, Boscati L, Bravin S, Zamberlan F, Alecci M, et al. Plasma and platelet amino acid concentrations in patients affected by major depression and under fluvoxamine treatment. Neuropsychobiology. (1998) 37:124–9. doi: 10.1159/000026491
10. Helton SG, Lohoff FW. Serotonin pathway polymorphisms and the treatment of major depressive disorder and anxiety disorders. Pharmacogenomics. (2015) 16:541–53. doi: 10.2217/pgs.15.15
11. Lai WT, Deng WF, Xu SX, Zhao J, Xu D, Liu YH, et al. Shotgun metagenomics reveals both taxonomic and tryptophan pathway differences of gut microbiota in major depressive disorder patients. Psychol Med. (2019) 5:1–12. doi: 10.1017/S0033291719003027
12. Zhou Y, Zheng W, Liu W, Wang C, Zhan Y, Li H, et al. Cross-sectional relationship between kynurenine pathway metabolites and cognitive function in major depressive disorder. Psychoneuroendocrinology. (2019) 101:72–9. doi: 10.1016/j.psyneuen.2018.11.001
13. Pan JX, Xia JJ, Deng FL, Liang WW, Wu J, Yin BM, et al. Diagnosis of major depressive disorder based on changes in multiple plasma neurotransmitters: a targeted metabolomics study. Transl Psychiat. (2018) 10:8. doi: 10.1038/s41398-018-0183-x
14. Ulaszewska MM, Weinert CH, Trimigno A, Portmann R, Andres Lacueva C, Badertscher R, et al. Nutrimetabolomics: an integrative action for metabolomic analyses in human nutritional studies. Mol Nutr Food Res. (2019) 63:e1800384. doi: 10.1002/mnfr.201970001
15. Leuchter AF, Hunter AM, Krantz DE, Cook IA. Intermediate phenotypes and biomarkers of treatment outcome in major depressive disorder. Dialogues Clin Neurosci. (2014) 16:525–37. doi: 10.31887/DCNS.2014.16.4/aleuchter
16. Barupal DK, Fan S, Fiehn O. Integrating bioinformatics approaches for a comprehensive interpretation of metabolomics datasets. Curr Opin Biotechnol. (2018) 54:1–9. doi: 10.1016/j.copbio.2018.01.010
17. Thase ME, Weiller E, Zhang P, Weiss C, McIntyre RS. Adjunctive brexpiprazole in patients with major depressive disorder and anxiety symptoms: post hoc analyses of three placebo-controlled studies. Neuropsychiatr Dis Treat. (2019) 15:37–45. doi: 10.2147/NDT.S185815
18. Lyndon GJ, Prieto R, Wajsbrot DB, Allgulander C, Bandelow B. Efficacy of venlafaxine extended release in major depressive disorder patients: effect of baseline anxiety symptom severity. Int Clin Psychopharmacol. (2019) 34:110–8. doi: 10.1097/YIC.0000000000000256
19. Zimmerman M, Chelminski I, Young D, Dalrymple K, Walsh E, Rosenstein L, et al. Clinically useful self-report measure of the DSM-5 anxious distress specifier for major depressive disorder. J Clin Psychiat. (2014) 75:601–7. doi: 10.4088/JCP.13m08961
20. Goldberg D, Fawcett J. The importance of anxiety in both major depression and bipolar disorder. Depress Anxiety. (2012) 29:471–8. doi: 10.1002/da.21939
21. Pfeiffer PN, Ganoczy D, Ilgen M, Zivin K, Valenstein M. Comorbid anxiety as a suicide risk factor among depressed veterans. Depress Anxiety. (2009) 26:752–7. doi: 10.1002/da.20583
22. Starkstein SE, Davis WA, Dragovic M, Cetrullo V, Davis TM, Bruce DG. Diagnostic criteria for depression in type 2 diabetes: a data-driven approach. PLoS ONE. (2014) 9:e112049. doi: 10.1371/journal.pone.0112049
23. Carta MG, Loviselli A, Hardoy MC, Massa S, Cadeddu M, Sardu C, et al. The link between thyroid autoimmunity (antithyroid peroxidase autoantibodies) with anxiety and mood disorders in the community: a field of interest for public health in the future. BMC Psychiatry. (2004) 4:25. doi: 10.1186/1471-244X-4-25
24. Nicholson JK, Wilson ID. Opinion: understanding ‘global’ systems biology: metabonomics and the continuum of metabolism. Nat Rev Drug Discov. (2003) 2:668–76. doi: 10.1038/nrd1157
25. Pedrini M, Cao B, Nani JVS, Cerqueira RO, Mansur RB, Tasic L, et al. Advances and challenges in development of precision psychiatry through clinical metabolomics on mood and psychotic disorders. Prog Neuro-Psychoph. (2019) 93:182–8. doi: 10.1016/j.pnpbp.2019.03.010
26. MacDonald K, Krishnan A, Cervenka E, Hu G, Guadagno E, Trakadis Y. Biomarkers for major depressive and bipolar disorders using metabolomics: a systematic review. Am J Med Genet B. (2019) 180:122–37. doi: 10.1002/ajmg.b.32680
27. Xia JG, Wishart DS. Web-based inference of biological patterns, functions and pathways from metabolomic data using MetaboAnalyst. Nat Protoc. (2011) 6:743–60. doi: 10.1038/nprot.2011.319
28. Xia J, Wishart DS. MSEA: a web-based tool to identify biologically meaningful patterns in quantitative metabolomic data. Nucleic Acids Res. (2010) 38:W71–7. doi: 10.1093/nar/gkq329
29. Hamilton M. The assessment of anxiety states by rating. Br J Med Psychol. (1959) 32:50–5. doi: 10.1111/j.2044-8341.1959.tb00467.x
30. Baumann HR, Cottier P. Sodium fluorescein in determination of heart minute volume with the aid of the Hamilton-Stewart dilution method. Helv Med Acta. (1960) 27:18–36.
31. Maes M, Moraes JB, Congio A, Bonifacio KL, Barbosa DS, Vargas HO, et al. Development of a novel staging model for affective disorders using partial least squares bootstrapping: effects of lipid-associated antioxidant defenses and neuro-oxidative stress. Mol Neurobiol. (2019) 56:6626–44. doi: 10.1007/s12035-019-1552-z
32. Li N, Zhao L, Guo C, Liu C, Liu Y. Identification of a novel DNA repair-related prognostic signature predicting survival of patients with hepatocellular carcinoma. Cancer Manag Res. (2019) 11:7473–84. doi: 10.2147/CMAR.S204864
33. Liu Q, Li C, Wanga V, Shepherd BE. Covariate-adjusted Spearman's rank correlation with probability-scale residuals. Biometrics. (2018) 74:595–605. doi: 10.1111/biom.12812
34. Nam D, Kim SY. Gene-set approach for expression pattern analysis. Brief Bioinform. (2008) 9:189–97. doi: 10.1093/bib/bbn001
35. Subramanian A, Tamayo P, Mootha VK, Mukherjee S, Ebert BL, Gillette MA, et al. Gene set enrichment analysis: a knowledge-based approach for interpreting genome-wide expression profiles. Proc Natl Acad Sci U S A. (2005) 102:15545–50. doi: 10.1073/pnas.0506580102
36. Deussing JM, Jakovcevski M. Histone modifications in major depressive disorder and related rodent models. Adv Exp Med Biol. (2017) 978:169–83. doi: 10.1007/978-3-319-53889-1_9
37. Zheng P, Zeng B, Zhou C, Liu M, Fang Z, Xu X, et al. Gut microbiome remodeling induces depressive-like behaviors through a pathway mediated by the host's metabolism. Mol Psychiatr. (2016) 21:786–96. doi: 10.1038/mp.2016.44
38. Jeon SW, Kim YK. The role of neuroinflammation and neurovascular dysfunction in major depressive disorder. J Inflamm Res. (2018) 11:179–92. doi: 10.2147/JIR.S141033
39. Altamura C, Maes M, Dai J, Meltzer HY. Plasma concentrations of excitatory amino acids, serine, glycine, taurine and histidine in major depression. Eur Neuropsychopharmacol. (1995) 5:71–5. doi: 10.1016/0924-977X(95)00033-L
40. Do KQ, Lauer CJ, Schreiber W, Zollinger M, Gutteck-Amsler U, Cuenod M, et al. Gamma-Glutamylglutamine and taurine concentrations are decreased in the cerebrospinal fluid of drug-naive patients with schizophrenic disorders. J Neurochem. (1995) 65:2652–62. doi: 10.1046/j.1471-4159.1995.65062652.x
41. Xu HB, Fang L, Hu ZC, Chen YC, Chen JJ Li FF, Lu J, et al. Potential clinical utility of plasma amino acid profiling in the detection of major depressive disorder. Psychiatry Res. (2012) 200:1054–7. doi: 10.1016/j.psychres.2012.05.027
42. Meunier CN, Dallerac G, Le Roux N, Sacchi S, Levasseur G, Amar M, et al. D-serine and glycine differentially control neurotransmission during visual cortex critical period. PLoS ONE. (2016) 11:e0151233. doi: 10.1371/journal.pone.0151233
43. Peyrovian B, Rosenblat JD, Pan Z, Iacobucci M, Brietzke E, McIntyre RS. The glycine site of NMDA receptors: A target for cognitive enhancement in psychiatric disorders. Prog Neuropsychopharmacol Biol Psychiatry. (2019) 92:387–404. doi: 10.1016/j.pnpbp.2019.02.001
44. Fossat P, Turpin FR, Sacchi S, Dulong J, Shi T, Rivet JM, et al. Glial D-serine gates NMDA receptors at excitatory synapses in prefrontal cortex. Cereb Cortex. (2012) 22:595–606. doi: 10.1093/cercor/bhr130
45. Curcio L, Podda MV, Leone L, Piacentini R, Mastrodonato A, Cappelletti P, et al. Reduced D-serine levels in the nucleus accumbens of cocaine-treated rats hinder the induction of NMDA receptor-dependent synaptic plasticity. Brain. (2013) 136:1216–30. doi: 10.1093/brain/awt036
46. Castanheira L, Silva C, Cheniaux E, Telles-Correia D. Neuroimaging correlates of depression-implications to clinical practice. Front Psychiatry. (2019) 10:703. doi: 10.3389/fpsyt.2019.00703
47. Zanos P, Piantadosi SC, Wu HQ, Pribut HJ, Dell MJ, Can A, et al. The prodrug 4-chlorokynurenine causes ketamine-like antidepressant effects, but not side effects, by NMDA/GlycineB-Site inhibition. J Pharmacol Exp Ther. (2015) 355:76–85. doi: 10.1124/jpet.115.225664
48. Rorick-Kehn LM, Hart JC, McKinzie DL. Pharmacological characterization of stress-induced hyperthermia in DBA/2 mice using metabotropic and ionotropic glutamate receptor ligands. Psychopharmacology. (2005) 183:226–40. doi: 10.1007/s00213-005-0169-2
49. Santos P, Bittencourt AS, Schenberg LC, Carobrez AP. Elevated T-maze evaluation of anxiety and memory effects of NMDA/glycine-B site ligands injected into the dorsal periaqueductal gray matter and the superior colliculus of rats. Neuropharmacology. (2006) 51:203–12. doi: 10.1016/j.neuropharm.2006.03.016
50. Labrie V, Clapcote SJ, Roder JC. Mutant mice with reduced NMDA-NR1 glycine affinity or lack of D-amino acid oxidase function exhibit altered anxiety-like behaviors. Pharmacol Biochem Behav. (2009) 91:610–20. doi: 10.1016/j.pbb.2008.09.016
51. Moaddel R, Shardell M, Khadeer M, Lovett J, Kadriu B, Ravichandran S, et al. Plasma metabolomic profiling of a ketamine and placebo crossover trial of major depressive disorder and healthy control subjects. Psychopharmacology (Berl). (2018) 235:3017–30. doi: 10.1007/s00213-018-4992-7
52. Baranyi A, Amouzadeh-Ghadikolai O, Rothenhausler HB, Theokas S, Robier C, Baranyi M, et al. Nitric oxide-related biological pathways in patients with major depression. PLoS ONE. (2015) 10:e0143397. doi: 10.1371/journal.pone.0143397
53. Morris SM Jr. Arginine: beyond protein. Am J Clin Nutr. (2006) 83:508S−512S. doi: 10.1093/ajcn/83.2.508S
54. Wegener G, Volke V. Nitric oxide synthase inhibitors as antidepressants. Pharmaceuticals (Basel). (2010) 3:273–99. doi: 10.3390/ph3010273
55. Faraco G, Hochrainer K, Segarra SG, Schaeffer S, Santisteban MM, Menon A, et al. Dietary salt induces cognitive impairment by promoting tau pathology. Ann Neurol. (2019) 86:S134–S134. doi: 10.1101/470666
56. Wang M, Wei J, Yang X, Ni P, Wang Y, Zhao L, et al. The level of IL-6 was associated with sleep disturbances in patients with major depressive disorder. Neuropsychiatr Dis Treat. (2019) 15:1695–700. doi: 10.2147/NDT.S202329
57. Bhattacharyya S, Ahmed AT, Arnold M, Liu D, Luo C, Zhu H, et al. Metabolomic signature of exposure and response to citalopram/escitalopram in depressed outpatients. Transl Psychiatry. (2019) 9:173. doi: 10.1038/s41398-019-0507-5
58. Bekhbat M, Treadway MT, Goldsmith DR, Woolwine BJ, Haroon E, Miller AH, et al. Gene signatures in peripheral blood immune cells related to insulin resistance and low tyrosine metabolism define a sub-type of depression with high CRP and anhedonia. Brain Behav Immun. (2020) 88:161–5. doi: 10.1016/j.bbi.2020.03.015
59. Felger JC Li L, Marvar PJ, Woolwine BJ, Harrison DG, Raison CL, Miller AH. Tyrosine metabolism during interferon-alpha administration: association with fatigue and CSF dopamine concentrations. Brain Behav Immun. (2013) 31:153–60. doi: 10.1016/j.bbi.2012.10.010
60. Fiori LM, Kos A, Lin R, Theroux JF, Lopez JP, Kuhne C, et al. miR-323a regulates ERBB4 and is involved in depression. Mol Psychiatry. (2020) 1–14. doi: 10.1038/s41380-020-00953-7. [Epub ahead of print].
61. Altamura CA, Mauri MC, Ferrara A, Moro AR, D'Andrea G, Zamberlan F. Plasma and platelet excitatory amino acids in psychiatric disorders. Am J Psychiatry. (1993) 150:1731–3. doi: 10.1176/ajp.150.11.1731
62. Ida T, Hara M, Nakamura Y, Kozaki S, Tsunoda S, Ihara H. Cytokine-induced enhancement of calcium-dependent glutamate release from astrocytes mediated by nitric oxide. Neurosci Lett. (2008) 432:232–6. doi: 10.1016/j.neulet.2007.12.047
63. Levinson AJ, Fitzgerald PB, Favalli G, Blumberger DM, Daigle M, Daskalakis ZJ. Evidence of cortical inhibitory deficits in major depressive disorder. Biol Psychiatry. (2010) 67:458–64. doi: 10.1016/j.biopsych.2009.09.025
64. Abdallah CG, Jiang L, De Feyter HM, Fasula M, Krystal JH, Rothman DL, Mason GF, Sanacora G. Glutamate metabolism in major depressive disorder. Am J Psychiatry. (2014) 171:1320–7. doi: 10.1176/appi.ajp.2014.14010067
65. Rathod R, Gajera B, Nazir K, Wallenius J, Velagapudi V. Simultaneous measurement of tricarboxylic acid cycle intermediates in different biological matrices using liquid chromatography-tandem mass spectrometry; quantitation and comparison of TCA cycle intermediates in human serum, plasma, kasumi-1 cell and murine liver tissue. Metabolites. (2020) 10:103. doi: 10.3390/metabo10030103
66. Kladna A, Marchlewicz M, Piechowska T, Kruk I, Aboul-Enein HY. Reactivity of pyruvic acid and its derivatives towards reactive oxygen species. Luminescence. (2015) 30:1153–8. doi: 10.1002/bio.2879
67. Pu J, Liu Y, Zhang H, Tian L, Gui S, Yu Y, et al. An integrated meta-analysis of peripheral blood metabolites and biological functions in major depressive disorder. Mol Psychiatry. (2020) 1–12. doi: 10.1038/s41380-020-0645-4. [Epub ahead of print].
68. Cheffer A, Castillo ARG, Correa-Velloso J, Goncalves MCB, Naaldijk Y, Nascimento IC, et al. Purinergic system in psychiatric diseases. Mol Psychiatry. (2018) 23:94–106. doi: 10.1038/mp.2017.188
Keywords: major depressive disorder, metabolomics, metabolic pathway, glycine and serine metabolism, anxiety
Citation: Du Y, Wei J, Zhang Z, Yang X, Wang M, Wang Y, Qi X, Zhao L, Tian Y, Guo W, Wang Q, Deng W, Li M, Lin D, Li T and Ma X (2021) Plasma Metabolomics Profiling of Metabolic Pathways Affected by Major Depressive Disorder. Front. Psychiatry 12:644555. doi: 10.3389/fpsyt.2021.644555
Received: 21 December 2020; Accepted: 17 August 2021;
Published: 27 September 2021.
Edited by:
Xenia Gonda, Semmelweis University, HungaryReviewed by:
Massimo Carlo Mauri, IRCCS Ca 'Granda Foundation Maggiore Policlinico Hospital, ItalyZili You, University of Electronic Science and Technology of China, China
Copyright © 2021 Du, Wei, Zhang, Yang, Wang, Wang, Qi, Zhao, Tian, Guo, Wang, Deng, Li, Lin, Li and Ma. This is an open-access article distributed under the terms of the Creative Commons Attribution License (CC BY). The use, distribution or reproduction in other forums is permitted, provided the original author(s) and the copyright owner(s) are credited and that the original publication in this journal is cited, in accordance with accepted academic practice. No use, distribution or reproduction is permitted which does not comply with these terms.
*Correspondence: Xiaohong Ma, bWF4aWFvaG9uZ0BzY3UuZWR1LmNu