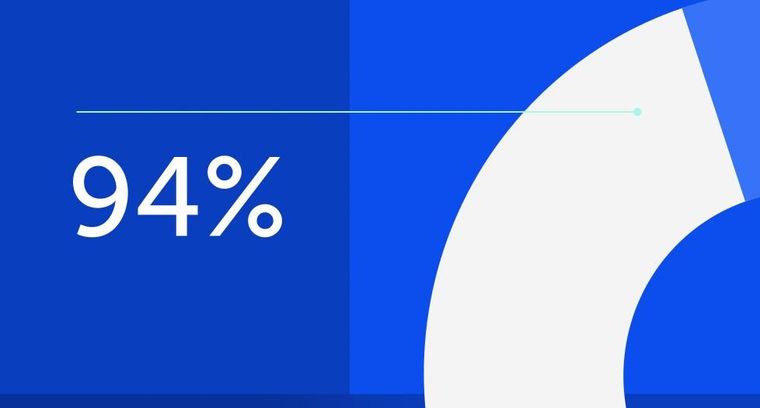
94% of researchers rate our articles as excellent or good
Learn more about the work of our research integrity team to safeguard the quality of each article we publish.
Find out more
MINI REVIEW article
Front. Psychiatry, 28 June 2021
Sec. Addictive Disorders
Volume 12 - 2021 | https://doi.org/10.3389/fpsyt.2021.643107
This article is part of the Research TopicPsychological Aspects of Cannabis Use and Cannabis Use DisorderView all 14 articles
Globally, cannabis is the most frequently used controlled substance after alcohol and tobacco. Rates of cannabis use are steadily increasing in many countries and there is emerging evidence that there is likely to be greater risk due to increased concentrations of delta-9-tetrahydrocannabinol (THC). Cannabis use and Cannabis Use Disorder (CUD) has been linked to a wide range of adverse health outcomes. Several biological, psychological, and social risk factors are potential targets for effective evidence-based treatments for CUD. There are no effective medications for CUD and psychological interventions are the main form of treatment. Psychological treatments based on Social Cognitive Theory (SCT) emphasize the importance of targeting 2 keys psychological mechanisms: drug outcome expectancies and low drug refusal self-efficacy. This mini-review summarizes the evidence on the role of these mechanisms in the initiation, maintenance, and cessation of cannabis use. It also reviews recent evidence showing how these psychological mechanisms are affected by social and biologically-based risk factors. A new bioSocial Cognitive Theory (bSCT) is outlined that integrates these findings and implications for psychological cannabis interventions are discussed. Preliminary evidence supports the application of bSCT to improve intervention outcomes through better targeted treatment.
Cannabis is the most widely used illicit drug worldwide and more jurisdictions are decriminalizing or legalizing use (1, 2). In 2018, it was estimated that 3.9% of the global adult population reported past-year cannabis use [~192 million people (3)]. North America (12.4%), West and Central Africa (12.4%), and Oceania (10.3%) are among the highest cannabis-using regions in the world, with rates of use increasing in many nations (3). Cannabis may be more harmful now than it has ever been, due to increased delta-9-tetrahydrocannabinol (THC) content—the key psychoactive ingredient (3–5). Higher THC potency cannabis is associated with an increased incidence of adverse side effects (6, 7).
The most severe and likely adverse side effect of cannabis use is developing a Cannabis Use Disorder [CUD; (8)]. Of those who have ever tried cannabis, 1 in 10 will develop moderate-severe CUD, formerly labeled cannabis dependence in the DSM-IV (9, 10). This risk increases to 1 in 6 if use commenced during adolescence (11). Daily users hold the greatest risk with ~1 in 2 developing moderate-severe CUD (12). Moderate-severe CUD impacts several areas of functioning with those affected more likely to experience comorbid psychiatric problems, relationship and financial difficulties, insomnia, withdrawal symptoms, reduced energy, low self-esteem and self-confidence, and reduced productivity (13–15). There are no effective medications for CUD and psychological interventions are the main form of treatment (2, 16).
The effectiveness of cognitive-behavior therapy (CBT), motivational enhancement therapy and contingency management as treatment for CUD is well-established [e.g., (16–18)]. CBT for substance use disorder and, to a lesser extent, motivational enhancement therapy with its focus on self-efficacy, are based on Social Cognitive Theory (SCT) (19). Social Cognitive Theory (SCT) conceptualizes cannabis use as a learned behavior that is believed to serve some adaptive and coping functions (e.g., stress reduction, social facilitation). CBT targets the (perceived) functional role that cannabis use plays in a patient's life and seeks to alter the cognitive and behavioral mechanisms precipitating use (20, 21). Patients are taught skills to aid cannabis reduction/cessation and maintain this change. This could involve, for example, teaching patients to identify situations likely to trigger motivation to cannabis use and how to avoid them, or how to address the thoughts and emotions underlying the motivation to use (22, 23). Other components of CBT include building drug refusal skills and problem-solving skills, and making healthy lifestyle modifications (24). The main goals of CBT are to increase patient self-efficacy to resist cannabis use and expand their repertoire of coping skills (21). While effective, CBT and other evidence-based treatments produce modest long-term outcomes in moderate-severe CUD (25–27), less than one third of those with CUD seek treatment and, among those, almost half prematurely discontinue treatment (26, 28–31). Further refinement of effective treatments like CBT could lead to improved patient retention and outcomes. The aim of this mini-review is to summarize the evidence on key psychological mechanisms in CUD and how they are affected by social and biologically-based risk factors. A theoretical review was conducted on published studies of Social Cognitive Theory and cannabis use, encompassing related relevant literature on other drug expectancies, self-efficacy, and temperament/personality. There were no a priori restrictions on the type of published studies included. In integrating these findings, a new bioSocial Cognitive Theory (bSCT) is reviewed that could facilitate a more precise application of evidence-based treatments like CBT.
In its application to substance use, SCT predicts that the likelihood of using substances is the result of an individual's drug outcome expectancies and refusal self-efficacy beliefs (32–34). These beliefs can develop through vicarious conditioning (observing others), even before substance use is initiated (19). Individuals who have never used cannabis already hold beliefs about the expected positive and negative outcomes of use, which are called cannabis outcome expectancies, and these beliefs predict future use (35–37).
Cannabis outcome expectancies are beliefs that an individual holds regarding the expected consequences of engaging in cannabis use, which may be positive or negative (19, 37). Positive cannabis expectancies play an influential role in motivating substance use, whilst negative expectancies generally serve to inhibit use (35, 37–40). Their effect on cannabis use behavior may not be equal. Some studies have found negative cannabis expectancies are no longer associated with consumption when controlling for the effects of positive expectancies (37, 39, 41). Negative expectancies are also a stronger correlate of cannabis-related problems in clinical samples. Therefore, high negative expectancies may be more the result of problematic cannabis use rather than low negative expectancies being an initial cause (37, 41–43).
Expectancies affect motivation to attempt, and ability to succeed in, cannabis cessation. Positive cannabis expectancies are associated with less positive cessation expectancies (i.e., beliefs that quitting cannabis will result in positive outcomes), while negative expectancies are associated with more positive cessation expectancies and perceived benefit of reducing use (44, 45). Boden et al. (39) found baseline positive cannabis expectancies predicted greater odds of lapse/relapse during a self-initiated cessation attempt in military veterans with CUD. Negative expectancies predicted lower odds of lapse/relapse. In moderate-severe CUD outpatients, Gullo et al. (46) found that higher levels of negative expectancies predicted greater likelihood of abstinence and fewer days of use over 6 weeks of CBT. While positive expectancies did not directly influence cannabis use, their effect was fully mediated by a negative association with cannabis refusal self-efficacy. That is, positive expectancies may increase relapse risk by undermining confidence in the ability to resist cannabis in cued situations.
Cannabis refusal self-efficacy is the confidence that an individual has in their ability to resist or refuse using cannabis in cued situations (47). Generally speaking, the strength of self-efficacy beliefs determine whether a person will attempt to cope with a difficult situation and how much effort is exerted (19, 48). Cannabis refusal self-efficacy plays an important protective role at several stages of cannabis use. For instance, high levels of cannabis refusal self-efficacy are associated with non-use in adolescents (49–51). Among frequent cannabis users, high levels of refusal self-efficacy are associated with fewer cannabis-related problems, less severe dependence and fewer days of use (41, 42, 47, 52).
Cannabis refusal self-efficacy may play a role in motivating behavior change among heavy cannabis users. One study found that cannabis refusal self-efficacy was associated with greater readiness to change, and predicted initiation of behavior change among men with cannabis dependence (53). Another study revealed that in female users who had previously tried to quit cannabis, refusal self-efficacy was associated with greater motivation to try again (54). These findings are consistent with Bandura's (19, 48) conceptualization of self-efficacy whereby individuals are more likely to engage in behaviors in which they are confident that they can enact successfully.
Cannabis refusal self-efficacy is consistently associated with better treatment response. Pre-treatment levels of cannabis refusal self-efficacy are associated with greater odds of abstinence during CUD treatment (46, 53) and predict less cannabis use and fewer cannabis-related problems for up to 6 months post-treatment (55). Post-treatment levels of cannabis refusal self-efficacy have an even stronger positive effect, predicting less cannabis consumption at 3, 6, and 12-months post-treatment (56–58). Low self-efficacy in response to negative emotion may be particularly salient (46).
Cannabis refusal self-efficacy may also be an important mechanism of change in treatments for CUD. Several studies have demonstrated that changes in cannabis refusal self-efficacy that occur during treatment are the strongest predictor of long-term abstinence—up to 14 months (25, 56–58). Regardless of specific treatment received, individuals who report the greatest improvements in cannabis refusal self-efficacy in treatment experience the most successful outcomes (33, 58, 59). These results indicate that increased cannabis refusal self-efficacy is a mechanism of change in psychological treatments for CUD.
One study has examined the means through which cannabis refusal self-efficacy may translate into improved treatment outcomes. Litt and Kadden (58) combined data from 3 cannabis treatment trials (N = 901) and found that the effects of refusal self-efficacy on cannabis use and cannabis-related problems were partially mediated by increased use of coping skills and by reductions in emotional distress. These findings support Bandura's (19, 48) hypothesis that self-efficacy determines whether a person will attempt to cope with a difficult situation and how much effort is exerted. However, the indirect/mediated effects reported by Litt and Kadden were small and the larger direct effects of self-efficacy remained unexplained. Further research is needed to obtain a better understanding of precisely how increased self-efficacy leads to better treatment outcomes.
Consistent with Bandura's (19, 48) contention that self-efficacy is the final pathway that influences human behavior, cannabis refusal self-efficacy has been found to mediate the effects of other psychological risk factors on cannabis use and related problems: cannabis outcome expectancies, cannabis coping motives and descriptive peer norms (41, 46, 52, 60). Despite the importance of cannabis expectancies and refusal self-efficacy, there is a paucity of research examining these constructs together. This is also true of the wider substance use literature. Theoretically, outcome expectancies should affect refusal self-efficacy in the development of CUD (33). For example, an individual expecting greater reinforcement from cannabis (high positive expectancies) is more likely to believe it to be harder to resist in cued situations (low refusal self-efficacy). The impact of cannabis expectancies on consumption is likely to be mediated in large part by self-efficacy, and this has been demonstrated empirically (41, 46).
In summary, SCT provides a valuable framework to conceptualize CUD and already informs evidence-based treatments. Empirical studies of SCT applied to cannabis use show that the aggregate positive outcomes an individual expects from cannabis, the more likely they are to engage in problematic use. Conversely, the stronger the negative outcomes expected and the more confident that an individual is in their ability to resist using cannabis, the more likely they are to abstain. Positive cannabis expectancies may have a stronger impact on behavior than negative expectancies. However, the important role of refusal self-efficacy as a mediator of expectancy effects complicates simple interpretations, and there is a need for more integrative research to advance the field. Increasing self-efficacy is a primary goal of existing evidence-based treatments. A better understanding of the factors that strengthen refusal self-efficacy could serve to improve upon them.
Individual differences exist in the strength of one's drug outcome expectancy and refusal self-efficacy beliefs. According to Bandura's (19, 48) notion of triadic reciprocal causation, these beliefs are influenced by, and in turn influence, one's behavior, their environment and personal factors within the individual. Gullo et al. (61) proposed that, when applied to substance use, biologically-based personality traits, specifically reward sensitivity/drive and rash impulsiveness, should act as important personal factors affecting social cognition and behavior (61). These traits are robust predictors of cannabis use (62, 63) and studies have found selective associations between reward drive and positive cannabis expectancies on the 1 hand, and rash impulsiveness and cannabis refusal self-efficacy on the other (51, 64). This bioSocial Cognitive Theory (bSCT) of temperamental risk factors, outcome expectancies, and drug refusal self-efficacy is depicted in Figure 1.
Reward drive reflects individual differences in one's sensitivity to reward stimuli and subsequent motivation to approach and obtain them, including substances (65–67). Individual differences in reward drive are biologically-based, reflecting variation in mesolimbic dopamine system functioning (68–70). Higher reward drive predicts greater reactivity to substance-related cues and unconditioned responses to their ingestion (71–75). As a result, individuals high in reward drive are more likely to attend to, encode, and recall reinforcement from cannabis use, creating stronger positive outcome expectancies. Studies of young adults and cannabis users referred to treatment have indeed observed a selective association between individual differences in reward drive and positive cannabis expectancies (51, 64). In moderate-severe CUD, positive expectancies are related to poorer treatment response because of their association with lower refusal self-efficacy (46).
Rash impulsiveness reflects individual differences in the capacity to inhibit/modify prepotent approach behavior in light of potential negative consequences (65, 67). Individual differences in rash impulsiveness are biologically-based, reflecting variation in the functioning of the orbitofrontal and anterior cingulate cortices (70, 76–78). Higher rash impulsiveness is associated with poorer reversal learning (79, 80), inhibitory control deficits in substance-dependent individuals (81), and mediates behavioral disinhibition associated with a family history of alcohol use disorder (82, 83). Individuals high in rash impulsiveness are typically aware of their difficulties with inhibitory control, which increases the likelihood of developing a generalized lower self-efficacy for situations requiring reward refusal, including substances (80). This lowered self-efficacy, in turn, increases the likelihood of cannabis use, further exacerbating risk (48). Studies of young adults and cannabis users referred to treatment have indeed observed a selective association between individual differences in rash impulsiveness and lower refusal self-efficacy (51, 64). In moderate-severe CUD, lower cannabis refusal self-efficacy predicts poorer response to treatment (46, 58).
The etiology of CUD is complex and several risk factors have been identified. bioSocial Cognitive Theory (bSCT) synthesizes some of the key risk factors in a way that may help practitioners better understand their combined and interacting effects, including how they manifest in the patient in front of them. This understanding is essential to optimizing treatment (i.e., precision mental health care (84, 85)]. In this mini-review, our focus started in the clinic with an established treatment (CBT) and its proposed mechanism of action (social cognition). We then broadened this focus to incorporate biologically-based factors theorized to directly affect these modifiable mechanisms (impulsivity traits). In outlining the interactions between these biological and cognitive factors, and their effect on behavior, bSCT can reveal individualized targets for CUD treatment.
bioSocial Cognitive Theory (bSCT) proposes modifiable pathways of risk that may be altered directly or indirectly during CUD treatment for different patients. For example, while it is known that increasing refusal self-efficacy is important, the most effective means of doing so will vary between patients (33, 58, 86). Directly increasing it with refusal skills training will work for patients who need to learn skills in how to assertively say “no” when offered or pressured to use cannabis (87, 88). But, according to bSCT, it is less likely to be effective in isolation for those high in rash impulsiveness and holding strong positive expectancies (51, 64). Such patients would be likely to face more significant challenges saying “no” in the first place, because of the greater salience of expected short-term reinforcement (e.g., intoxication) and lesser salience of future negative consequences (e.g., negative urine drug test result at work). For these patients, cognitive restructuring to reduce positive expectancies and strategies to increase reflection and problem-solving would be indicated, according to bSCT. An assessment of patient bSCT factors could reveal high-value therapeutic targets, facilitating tailored treatment.
As a proof-of-concept for bSCT's utility in precision mental health care, Papinczak et al. (89) drew on bSCT to develop a theoretically-driven instant assessment and feedback system (iAx) for CUD. iAx electronically administers and instantly scores validated, standardized assessments and synthesizes this information through the theoretical lens of bSCT. Compared to treatment-as-usual, which administered the same assessments, iAx-enhanced brief intervention led to significantly greater motivation to reduce cannabis use in 87 non-treatment seeking users referred for assessment. Papinczak et al. proposed that iAx may have improved practitioners' formulation of the case, increasing treatment precision. Amidst a sea of assessment results, iAx may have provided a clearer focus on the modifiable factors likely to maximize outcomes for that patient. A CUD and alcohol use disorder version of iAx is now freely available at gullo.com.au/iaxsite.
This mini-review summarizes evidence on the application of bioSocial Cognitive Theory (bSCT) to cannabis use disorder (CUD). Findings are encouraging and consistent with those previously reported in alcohol use disorder (61, 90–92). Further examination of temporal dynamics and reciprocal causation would strengthen clinical application, as there is some evidence of differences in bSCT pathway strength across the continuum of addiction (51, 64). The role of craving is also yet to be explicitly outlined, despite its importance to CUD. Recent developments in cognitive theories of craving and its measurement will facilitate integration (93, 94). There is also scope for inclusion of more fundamental biological factors, such as genetics, building on earlier work in SCT (95, 96), identification of genetic associations with impulsivity and psychiatric comorbidity (97, 98), and incorporating recent methodological advances [e.g., polygenic risk scores (99)]. However, the utility of bSCT in its current form is clear. It provides a coherent theoretical framework for integrating SCT and impulsivity theories of addiction, pointing toward new avenues for targeted treatment. bSCT constructs are predictive of CUD risk, motivation to seek treatment, and response to treatment. Preliminary evidence shows that simply presenting clinical assessment data through the lens of bSCT enhances delivery of brief intervention. These are valuable initial steps toward developing greater precision in the treatment of CUD.
MG and ZP created the outline of the review and wrote the first draft. ZP conducted the literature review. All authors contributed to subsequent revisions and approved the final manuscript.
MG was supported by a Medical Research Future Fund Translating Research into Practice (TRIP) Fellowship (1167986). ZP was supported by an Australian Government Research Training Stipend. The Australian National Centre for Youth Substance Use Research (MG, JC, and RY) was supported by funding from the Australian Government provided under the Commonwealth Drug and Alcohol Program grant. The funders had no role in the design or writing of the manuscript, or the decision to submit the paper for publication.
The authors declare that the research was conducted in the absence of any commercial or financial relationships that could be construed as a potential conflict of interest.
1. Hall WD. What has research over the past two decades revealed about the adverse health effects of recreational cannabis use? Addiction. (2015) 110:19–35. doi: 10.1111/add.12703
2. Connor JP, Stjepanović D, Le Foll B, Hoch E, Budney AJ, Hall WD. Cannabis use and cannabis use disorder. Nat Rev Dis Primers. (2021) 7:16. doi: 10.1038/s41572-021-00247-4
3. United Nations Office on Drugs and Crime UNODC. World Drug Report 2020. Geneva: United Nations (2020).
4. Drug Enforcement Administration. National Drug Threat Assessment Survey. Washington, DC: US Dept of Justice (2016).
5. Koob GF, Arends MA, Le Moal M. Drugs, Addiction, and the Brain. Boston, MA: Academic Press (2014).
6. Di Forti M, Marconi A, Carra E, Fraietta S, Trotta A, Bonomo M, et al. Proportion of patients in south London with first-episode psychosis attributable to use of high potency cannabis: a case-control study. Lancet Psychiatry. (2015) 2:233–8. doi: 10.1016/S2215-0366(14)00117-5
7. Freeman TP, Winstock AR. Examining the profile of high-potency cannabis and its association with severity of cannabis dependence. Psychol Med. (2015) 45:3181–9. doi: 10.1017/S0033291715001178
8. Hall WD, Degenhardt L. Adverse health effects of non-medical cannabis use. Lancet. (2009) 374:1383–91. doi: 10.1016/S0140-6736(09)61037-0
9. Anthony JC, Warner LA, Kessler RC. Comparative epidemiology of dependence on tobacco, alcohol, controlled substances, and inhalants: basic findings from the National Comorbidity Survey. In: Marlatt GA, editor. Addictive Behaviors: Readings on Etiology, Prevention, and Treatment. Washington, DC: American Psychological Association (1997). p. 3–39. doi: 10.1037/10248-001
10. Degenhardt L, Hall WD. Extent of illicit drug use and dependence, and their contribution to the global burden of disease. Lancet. (2012) 379:55–70. doi: 10.1016/S0140-6736(11)61138-0
11. Anthony J. The epidemiology of cannabis dependence. In: Roffman R, and Stephens R, editors. Cannabis Dependence: Its Nature, Consequences and Treatment (International Research Monographs in the Addictions). Cambridge: Cambridge University Press (2006). p. 58–105.
12. van der Pol P, Liebregts N, de Graaf R, Korf DJ, van den Brink W, van Laar M. Predicting the transition from frequent cannabis use to cannabis dependence: a three-year prospective study. Drug Alcohol Depend. (2013) 133:352–9. doi: 10.1016/j.drugalcdep.2013.06.009
13. Gruber AJ, Pope HG, Hudson JI, Yurgelun-Todd D. Attributes of long-term heavy cannabis users: a case-control study. Psychol Med. (2003) 33:1415–22. doi: 10.1017/S0033291703008560
14. Looby A, Earleywine M. Negative consequences associated with dependence in daily cannabis users. Subst Abuse Treat Prev Policy. (2007) 2:3. doi: 10.1186/1747-597X-2-3
15. Stephens RS, Babor TF, Kadden R, Miller M, Marijuana Treatment Project Research Group. The Marijuana Treatment Project: rationale, design and participant characteristics. Addiction. (2002) 97:109–24. doi: 10.1046/j.1360-0443.97.s01.6.x
16. Budney AJ, Sofis MJ, Borodovsky JT. An update on cannabis use disorder with comment on the impact of policy related to therapeutic and recreational cannabis use. Eur Arch Psychiatry Clin Neurosci. (2019)269:73–86. doi: 10.1007/s00406-018-0976-1
17. Davis ML, Powers MB, Handelsman P, Medina JL, Zvolensky M, Smits JAJ. Behavioral therapies for treatment-seeking cannabis users: a meta-analysis of randomized controlled trials. Eval Health Prof . (2015). 38:94–114. doi: 10.1177/0163278714529970
18. Magill M, Ray L a. Cognitive-behavioral treatment with adult alcohol and illicit drug users: a meta-analysis of randomized controlled trials. J Stud Alcohol Drugs. (2009) 70:516–27. doi: 10.15288/jsad.2009.70.516
19. Bandura A. Social Foundations of Thought and Action: A Social Cognitive Theory. Englewood Cliffs, NJ: Prentice-Hall (1986).
20. Larimer ME, Palmer RS, Marlatt GA. Relapse prevention. An overview of Marlatt's cognitive-behavioral model. Alcohol Res Health. (1999) 23:151–60.
21. Stephens RS, Roffman RA, Copeland J, Swift W. Cognitive-behavioral and motivational enhancement treatments for cannabis dependence. In: Roffman RA, Stephens RS, editors. Cannabis Dependence: Its Nature, Treatment and Consequences. Cambridge: Cambridge University Press (2006). p. 131–53. doi: 10.1017/CBO9780511544248.008
22. Marlatt GA, Gordon JR. Relapse Prevention: A Self-Control Strategy for the Maintenance of Behavior Change. New York, NY: Guilford (1985).
23. Beck AT, Wright FD, Newman CF, Liese BS. Cognitive therapy of substance abuse: theoretical rationale. NIDA Res Monograph. (1993) 137:123. doi: 10.1037/e495912006-008
24. Steinberg KL, Roffman RA, Carroll KM, Kabela E, Kadden R, Miller M, et al. Tailoring cannabis dependence treatment for a diverse population. Addiction. (2002) 971:135–42. doi: 10.1046/j.1360-0443.97.s01.5.x
25. Kadden RM, Litt MD, Kabela-Cormier E, Petry NM. Abstinence rates following behavioral treatments for marijuana dependence. Addict Behav. (2007) 32:1220–36. doi: 10.1016/j.addbeh.2006.08.009
26. Budney AJ, Moore BA, Rocha HL, Higgins ST. Clinical trial of abstinence-based vouchers and cognitive–behavioral therapy for cannabis dependence. J Consult Clin Psychol. (2006) 74:307–16. doi: 10.1037/0022-006X.74.2.307
27. Litt MD, Kadden RM, Petry NM. Behavioral treatment for marijuana dependence: randomized trial of contingency management and self-efficacy enhancement. Addict Behav. (2013) 38:1764–75. doi: 10.1016/j.addbeh.2012.08.011
28. Agosti V, Levin FR. Predictors of treatment contact among individuals with cannabis dependence. Am J Drug Alcohol Abuse. (2004) 30:121–7. doi: 10.1081/ADA-120029869
29. Wu L-T, Zhu H, Mannelli P, Swartz MS. Prevalence and correlates of treatment utilization among adults with cannabis use disorder in the United States. Drug Alcohol Depend. (2017) 177:153–62. doi: 10.1016/j.drugalcdep.2017.03.037
30. Vendetti J, McRee B, Miller M, Christiansen K, Herrell J, The Marjuana Treatment Project Research Group. Correlates of pre-treatment drop-out among persons with marijuana dependence. Addiction. (2002) 97:125–34. doi: 10.1046/j.1360-0443.97.s01.8.x
31. Carroll KM, Nich C, LaPaglia DM, Peters EN, Easton CJ, Petry NM. Combining cognitive behavioral therapy and contingency management to enhance their effects in treating cannabis dependence: less can be more, more or less. Addiction. (2012) 107:1650–9. doi: 10.1111/j.1360-0443.2012.03877.x
32. Bandura A. A sociocognitive analysis of substance abuse: an agentic perspective. Psychol Sci. (1999) 10:214–7. doi: 10.1111/1467-9280.00138
33. Kadden RM, Litt MD. The role of self-efficacy in the treatment of substance use disorders. Addict Behav. (2011) 36:1120–6. doi: 10.1016/j.addbeh.2011.07.032
34. Oei TPS, Baldwin AR. Expectancy theory: a two-process model of alcohol use and abuse. J Stud Alcohol. (1994) 55:525–34. doi: 10.15288/jsa.1994.55.525
35. Aarons GA, Brown SA, Stice E, Coe MT. Psychometric evaluation of the marijuana and stimulant effect expectancy questionnaires for adolescents. Addict Behav. (2001) 26:219–36. doi: 10.1016/S0306-4603(00)00103-9
36. Alfonso J, Dunn ME. Differences in the marijuana expectancies of adolescents in relation to marijuana use. Subst Misuse. (2007) 42:1009–25. doi: 10.1080/10826080701212386
37. Connor JP, Gullo MJ, Feeney GFX, Young RM. Validation of the Cannabis Expectancy Questionnaire (CEQ) in adult cannabis users in treatment. Drug Alcohol Depend. (2011) 115:167–74. doi: 10.1016/j.drugalcdep.2010.10.025
38. Schafer J, Brown S. Marijuana and cocaine effect expectancies and drug use patterns. J Consult Clin Psychol. (1991) 59:558–65. doi: 10.1037/0022-006X.59.4.558
39. Boden MT, McKay JR, Long WR, Bonn-Miller MO. The effects of cannabis use expectancies on self-initiated cannabis cessation. Addiction. (2013) 108:1649–57. doi: 10.1111/add.12233
40. Skenderian JJ, Siegel JT, Crano WD, Alvaro EE, Lac A. Expectancy change and adolescents' intentions to use marijuana. Psychol Addict Behav. (2008) 22:563–9. doi: 10.1037/a0013020
41. Connor JP, Gullo MJ, Feeney GFX, Kavanagh DJ, Young RM. The relationship between cannabis outcome expectancies and cannabis refusal self-efficacy in a treatment population. Addiction. (2014) 109:111–9. doi: 10.1111/add.12366
42. Hayaki J, Herman DS, Hagerty CE, de Dios M a, Anderson BJ, Stein MD. Expectancies and self-efficacy mediate the effects of impulsivity on marijuana use outcomes: an application of the acquired preparedness model. Addict Behav. (2011) 36:389–96. doi: 10.1016/j.addbeh.2010.12.018
43. Foster DW, Jeffries ER, Zvolensky MJ, Buckner JD. The interactive influence of cannabis-related negative expectancies and coping motives on cannabis use behavior and problems. Subst Misuse. (2016) 51:1504–11. doi: 10.1080/10826084.2016.1188947
44. Brackenbury LM, Ladd BO, Anderson KG. Marijuana use/cessation expectancies and marijuana use in college students. Am J Drug Alcohol Abuse. (2016) 42:25–31. doi: 10.3109/00952990.2015.1105242
45. Metrik J, Farris SG, Aston ER, Kahler CW. Development and initial validation of a marijuana cessation expectancies questionnaire. Drug Alcohol Depend. (2017) 177:163–70. doi: 10.1016/j.drugalcdep.2017.04.005
46. Gullo MJ, Matveeva M, Feeney GFX, Young RM, Connor JP. Social cognitive predictors of treatment outcome in cannabis dependence. Drug Alcohol Depend. (2017) 170:74–81. doi: 10.1016/j.drugalcdep.2016.10.030
47. Young RM, Gullo MJ, Feeney GFX, Connor JP. Development and validation of the Cannabis Refusal Self-Efficacy Questionnaire (CRSEQ) in adult cannabis users in treatment. Drug Alcohol Depend. (2012) 125:244–51. doi: 10.1016/j.drugalcdep.2012.02.018
48. Bandura A. Self-efficacy: toward a unifying theory of behavioral change. Psychol Rev. (1977) 84:191–215. doi: 10.1037/0033-295X.84.2.191
49. Newton NC, Havard A, Teesson M. The association between moral disengagement, psychological distress, resistive self-regulatory efficacy and alcohol and cannabis use among adolescents in Sydney, Australia. Addict Res Theory. (2012) 20:261–9. doi: 10.3109/16066359.2011.614976
50. Victoir A, Eertmans A, Van den Bergh O, Van den Broucke S. Association of substance-use behaviours and their social-cognitive determinants in secondary school students. Health Educ Res. (2007) 22:81–94. doi: 10.1093/her/cyl050
51. Papinczak ZE, Connor JP, Harnett P, Gullo MJ. A biosocial cognitive model of cannabis use in emerging adulthood. Addict Behav. (2018) 76:229–35. doi: 10.1016/j.addbeh.2017.08.011
52. Walker DD, Neighbors C, Rodriguez LM, Stephens RS, Roffman RA. Social norms and self-efficacy among heavy using adolescent marijuana smokers. Psychol Addict Behav. (2011) 25:727–32. doi: 10.1037/a0024958
53. Sherman BJ, Baker NL, McRae-Clark AL. Gender differences in cannabis use disorder treatment: change readiness and taking steps predict worse cannabis outcomes for women. Addict Behav. (2016) 60:197–202. doi: 10.1016/j.addbeh.2016.04.014
54. Caviness CM, Hagerty CE, Anderson BJ, de Dios MA, Hayaki J, Herman D, et al. Self-efficacy and motivation to quit marijuana use among young women. Am J Addict. (2013) 22:373–80. doi: 10.1111/j.1521-0391.2013.12030.x
55. Stephens RS, Wertz JS, Roffman RA. Predictors of marijuana treatment outcomes: the role of self-efficacy. J Subst Abuse. 1993 5:341–53. doi: 10.1016/0899-3289(93)90003-T
56. Stephens RS, Wertz JS, Roffman RA. Self-efficacy and marijuana cessation: a construct validity analysis. J Consult Clin Psychol. (1995) 63:1022–31. doi: 10.1037/0022-006X.63.6.1022
57. Litt MD, Kadden RM. Willpower versus “skillpower”: examining how self-efficacy works in treatment for marijuana dependence. Psychol Addict Behav. (2015) 29:532–40. doi: 10.1037/adb0000085
58. Litt MD, Kadden RM, Kabela-Cormier E, Petry NM. Coping skills training and contingency management treatments for marijuana dependence: exploring mechanisms of behavior change. Addiction. (2008) 103:638–48. doi: 10.1111/j.1360-0443.2008.02137.x
59. Litt MD, Kadden RM, Stephens RS, Marijuana Treatment Project Research Group. Coping and self-efficacy in marijuana treatment: results from the marijuana treatment project. J Consult Clin Psychol. (2005) 73:1015–25. doi: 10.1037/0022-006X.73.6.1015
60. Blevins CE, Banes KE, Stephens RS, Walker DD, Roffman RA. Motives for marijuana use among heavy-using high school students: an analysis of structure and utility of the Comprehensive Marijuana Motives Questionnaire. Addict Behav. (2016) 57:42–7. doi: 10.1016/j.addbeh.2016.02.005
61. Gullo MJ, Dawe S, Kambouropoulos N, Staiger PK, Jackson CJ. Alcohol expectancies and drinking refusal self-efficacy mediate the association of impulsivity with alcohol misuse. Alcohol Clin Exp Res. (2010) 34:1386–99. doi: 10.1111/j.1530-0277.2010.01222.x
62. Vergés A, Littlefield AK, Arriaza T, Alvarado ME. Impulsivity facets and substance use initiation: a comparison of two models of impulsivity. Addict Behav. (2019) 88:61–6. doi: 10.1016/j.addbeh.2018.08.018
63. Spechler PA, Allgaier N, Chaarani B, Whelan R, Watts R, Orr C, et al. The initiation of cannabis use in adolescence is predicted by sex-specific psychosocial and neurobiological features. Eur J Neurosci. (2019) 50:2346–56. doi: 10.1111/ejn.13989
64. Papinczak ZE, Connor JP, Feeney GFX, Harnett P, Young RM, Gullo MJ. Testing the biosocial cognitive model of substance use in cannabis users referred to treatment. Drug Alcohol Depend. (2019) 194:216–24. doi: 10.1016/j.drugalcdep.2018.09.032
65. Dawe S, Loxton NJ. The role of impulsivity in the development of substance use and eating disorders. Neurosci Biobehav Rev. (2004) 28:343–51. doi: 10.1016/j.neubiorev.2004.03.007
66. Wasserman AM, Mathias CW, Hill-Kapturczak N, Karns-Wright TE, Dougherty DM. The development of impulsivity and sensation seeking: associations with substance use among at-risk adolescents. J Res Adolesc. (2020) 30:1051–66. doi: 10.1111/jora.12579
67. Gullo MJ, Loxton NJ, Dawe S. Impulsivity: four ways five factors are not basic to addiction. Addict Behav. (2014) 39:1547–56. doi: 10.1016/j.addbeh.2014.01.002
68. Depue RA, Collins PF. Neurobiology of the structure of personality: dopamine, facilitation of incentive motivation, and extraversion. Behav Brain Sci. (1999) 22:491–517. doi: 10.1017/S0140525X99002046
70. Dawe S, Gullo MJ, Loxton NJ. Reward drive and rash impulsiveness as dimensions of impulsivity: implications for substance misuse. Addict Behav. (2004) 29:1389–405. doi: 10.1016/j.addbeh.2004.06.004
71. Glautier S, Bankart J, Williams A. Flavour conditioning and alcohol: a multilevel model of individual differences. Biol Psychol. (2000) 52:17–36. doi: 10.1016/S0301-0511(99)00022-8
72. Kambouropoulos N, Staiger PK. Reactivity to alcohol-related cues: relationship among cue type, motivational processes, and personality. Psychol Addict Behav. (2004) 18:275–83. doi: 10.1037/0893-164X.18.3.275
73. Kambouropoulos N, Staiger PK. The influence of sensitivity to reward on reactivity to alcohol-related cues. Addiction. (2001) 96:1175–85. doi: 10.1046/j.1360-0443.2001.968117510.x
74. Brunelle C, Assaad J-M, Barrett SP, Avila C, Conrod PJ, Tremblay RE, et al. Heightened heart rate response to alcohol intoxication is associated with a reward-seeking personality profile. Alcohol Clin Exp Res. (2004) 28:394–401. doi: 10.1097/01.ALC.0000117859.23567.2E
75. Zisserson RN, Palfai TP. Behavioral Activation System (BAS) sensitivity and reactivity to alcohol cues among hazardous drinkers. Addict Behav. (2007) 32:2178–86. doi: 10.1016/j.addbeh.2007.02.016
76. Rolls ET, Vatansever D, Li Y, Cheng W, Feng J. Rapid rule-based reward reversal and the lateral orbitofrontal cortex. Cereb Cortex Comm. (2020) 1:tgaa087. doi: 10.1093/texcom/tgaa087
77. Cheetham A, Allen NB, Whittle S, Simmons J, Yücel M, Lubman DI. Orbitofrontal cortex volume and effortful control as prospective risk factors for substance use disorder in adolescence. Eur Addict Res. (2016) 23:37–44. doi: 10.1159/000452159
78. Horn NR, Dolan M, Elliott R, Deakin JFW, Woodruff PWR. Response inhibition and impulsivity: an fMRI study. Neuropsychologia. (2003) 41:1959–66. doi: 10.1016/S0028-3932(03)00077-0
79. Franken IH, van Strien JW, Nijs I, Muris P. Impulsivity is associated with behavioral decision-making deficits. Psychiatry Res. (2008) 158:155–63. doi: 10.1016/j.psychres.2007.06.002
80. Gullo MJ, Jackson CJ, Dawe S. Impulsivity and reversal learning in hazardous alcohol use. Pers Individ Dif . (2010) 48:123–7. doi: 10.1016/j.paid.2009.09.006
81. Finn PR, Mazas C, Justus AN, Steinmetz J. Early-onset alcoholism with conduct disorder: go/no go learning deficits, working memory capacity, and personality. Alcohol Clin Exp Res. (2002) 26:186–206. doi: 10.1111/j.1530-0277.2002.tb02524.x
82. Lovallo WR, Yechiam E, Sorocco KH, Vincent AS, Collins FL. Working memory and decision-making biases in young adults with a family history of alcoholism: studies from the Oklahoma family health patterns project. Alcohol Clin Exp Res. (2006) 30:763–73. doi: 10.1111/j.1530-0277.2006.00089.x
83. Saunders B, Farag N, Vincent AS, Collins FL, Sorocco KH, Lovallo WR. Impulsive errors on a Go-NoGo reaction time task: disinhibitory traits in relation to a family history of alcoholism. Alcohol Clin Exp Res. (2008) 32:888–94. doi: 10.1111/j.1530-0277.2008.00648.x
84. DeRubeis RJ. The history, current status, and possible future of precision mental health. Behav Res Ther. (2019) 123:103506. doi: 10.1016/j.brat.2019.103506
85. Delgadillo J, Lutz W. A development pathway towards precision mental health care. JAMA Psychiatry. (2020) 77:889–90. doi: 10.1001/jamapsychiatry.2020.1048
86. Gullo MJ, Coates JM, Patton K, Connor JP. Beyond CBT: what is the future of alcohol use disorder treatment and prevention? In: Menzies RG, Kyrios M, Kazantzis N, editors. Innovations and Future Directions in the Behavioural and Cognitive Therapies. Samford Valley, QLD: Australian Academic Press (2016). p. 157–62.
87. Witkiewitz K, Donovan DM, Hartzler B. Drink refusal training as part of a combined behavioral intervention: effectiveness and mechanisms of change. J Consult Clin Psychol. (2012) 80:440–9. doi: 10.1037/a0026996
88. Onrust SA, Otten R, Lammers J, Smit F. School-based programmes to reduce and prevent substance use in different age groups: what works for whom? Systematic review and meta-regression analysis. Clin Psychol Rev. (2016) 44:45–59. doi: 10.1016/j.cpr.2015.11.002
89. Papinczak ZE, Connor JP, Feeney GFX, Gullo MJ. Additive effectiveness and feasibility of a theory-driven instant assessment and feedback system in brief cannabis intervention: a randomised controlled trial. Addict Behav. (2021) 113:106690. doi: 10.1016/j.addbeh.2020.106690
90. Kabbani R, Kambouropoulos N. Positive expectancies and perceived impaired control mediate the influence of reward drive and rash impulsiveness on alcohol use. Pers Individ Dif . (2013) 54:294–7. doi: 10.1016/j.paid.2012.08.008
91. Gullo MJ, St.John N, Young RM, Saunders JB, Noble EP, Connor JP. Impulsivity-related cognition in alcohol dependence: is it moderated by DRD2/ANKK1 gene status and executive dysfunction? Addict Behav. (2014) 39:1663–9. doi: 10.1016/j.addbeh.2014.02.004
92. Connor JP, Haber PS, Hall WD. Alcohol use disorders. Lancet. (2016) 387:988–98. doi: 10.1016/S0140-6736(15)00122-1
93. Kavanagh DJ, Andrade J, May J. Imaginary relish and exquisite torture: the elaborated intrusion theory of desire. Psychol Rev. (2005) 112:446–67. doi: 10.1037/0033-295X.112.2.446
94. May J, Andrade J, Kavanagh DJ, Feeney GFX, Gullo MJ, Statham DJ, et al. The Craving Experience Questionnaire: a brief, theory-based measure of consummatory desire and craving. Addiction. (2014) 109:728–35. doi: 10.1111/add.12472
95. Young RM, Lawford BR, Feeney GFX, Ritchie T, Noble EP. Alcohol-related expectancies are associated with the D2 dopamine receptor and GABAA receptor beta3 subunit genes. Psychiatry Res. (2004) 127:171–83. doi: 10.1016/j.psychres.2003.11.004
96. Connor JP, Young RM, Saunders JB, Lawford BR, Ho R, Ritchie TL, et al. The A1 allele of the D2 dopamine receptor gene region, alcohol expectancies and drinking refusal self-efficacy are associated with alcohol dependence severity. Psychiatry Res. (2008) 160:94–105. doi: 10.1016/j.psychres.2007.06.030
97. Leamy TE, Connor JP, Voisey J, Young RM, Gullo MJ. Alcohol misuse in emerging adulthood: association of dopamine and serotonin receptor genes with impulsivity-related cognition. Addict Behav. (2016) 63:29–36. doi: 10.1016/j.addbeh.2016.05.008
98. Cheah S-Y, Lawford BR, Young RM, Connor JP, Phillip Morris C, Voisey J. BDNF SNPs are implicated in comorbid alcohol dependence in schizophrenia but not in alcohol-dependent patients without schizophrenia. Alcohol. (2014) 49:491–7. doi: 10.1093/alcalc/agu040
Keywords: reward, impulsivity, expectancies, self-efficacy, cannabis, social cognition, bioSocial Cognitive Theory, precision medicine
Citation: Gullo MJ, Papinczak ZE, Feeney GFX, Young RMcd and Connor JP (2021) Precision Mental Health Care for Cannabis Use Disorder: Utility of a bioSocial Cognitive Theory to Inform Treatment. Front. Psychiatry 12:643107. doi: 10.3389/fpsyt.2021.643107
Received: 17 December 2020; Accepted: 27 May 2021;
Published: 28 June 2021.
Edited by:
Eva Hoch, Ludwig-Maximilians-University Munich, GermanyCopyright © 2021 Gullo, Papinczak, Feeney, Young and Connor. This is an open-access article distributed under the terms of the Creative Commons Attribution License (CC BY). The use, distribution or reproduction in other forums is permitted, provided the original author(s) and the copyright owner(s) are credited and that the original publication in this journal is cited, in accordance with accepted academic practice. No use, distribution or reproduction is permitted which does not comply with these terms.
*Correspondence: Matthew J. Gullo, bS5ndWxsb0B1cS5lZHUuYXU=
Disclaimer: All claims expressed in this article are solely those of the authors and do not necessarily represent those of their affiliated organizations, or those of the publisher, the editors and the reviewers. Any product that may be evaluated in this article or claim that may be made by its manufacturer is not guaranteed or endorsed by the publisher.
Research integrity at Frontiers
Learn more about the work of our research integrity team to safeguard the quality of each article we publish.