- Department of Child and Adolescent Psychiatry, Saarland University Hospital, Homburg, Germany
Background: The aim of this study was to evaluate the role of early Emotional Dysregulation (ED) at preschool age as a risk factor or predictor of later media use behavior and Gaming Disorder (GD) in school age.
Methods: 80 patients (63.7% male; mean age = 4.2, SD = 1.23) who had attended a special outpatient program for preschoolers at measuring point time t1 were contacted at measuring point time t2 (mean age = 9.2, SD = 2.03). At t1, the comprehensive clinical assessment comprised Child Behavior Checklist—Dysregulation Profile (CBCL-DP). At t2, parents completed a questionnaire on their children's media availability, usage times, and GD.
Results: ED predicts a more intense use of digital media in the future. The daily average screen-use time at t2 varies significantly between the groups (148 min for children with ED at t1 and 85 min for children without ED at t1). The intensity of media use can be considered a significant predictor for the presence of a GD in dimensional assessment. When GD is classified categorically, according to the DSM-5 criteria, there is no significant correlation between ED and later GD diagnosis, neither between screen-use time and GD diagnosis. However, at dimensional level, preschool children with ED show significantly higher GD symptom scores at 9 years of age.
Conclusion: ED at preschool age is strongly associated with time spent video gaming and GD symptoms 5 years later. Our results strongly indicate that emotion dysregulation in preschool children is a risk factor for later problematic video game playing behavior. This strengthens the concept of ED in the etiology of media use and provides potential targets for early GD prevention.
Introduction
Emotional Dysregulation (ED) is characterized by difficulties in understanding, accepting, and dealing with emotions [i.e., emotion regulation; (1)]. Due to these deficits, children and adolescents with ED symptoms often show little flexibility and spontaneity, with a lack of control and disruptive behavior (2). Findings suggest that ED may influence the development and course of various disorders, including different substance use disorders [e.g., alcohol, cocaine, or nicotine dependencies; (3–8)] as well as behavioral addictions [e.g., pathological gambling; (9)].
These named deficits in emotion regulation also lead to assume that some individuals try to mitigate, facilitate, or avoid (especially unpleasant, negative) emotional experiences by engaging in video games as distraction (10, 11). More specifically, it is believed that children and adolescents with ED use video game playing as a maladaptive coping strategy to deal with negative emotions (10). In addition, the disruptive and often poorly controllable behavior of children with ED can also make them less likely to socialize in preschool and school, which may lead to uncertainty in face-to-face interactions. In this respect, the lack of direct interaction with others in video games can reduce social insecurity in such situations. This probably leads to a tendency for children with low social skills and especially children with ED to prefer the internet in general but also video games in particular as a place for social interactions rather than real-life interactions (12).
With regard to early media use, some studies indicate that use of digital media might positively influence attitudes to learning and reading skills (13–18). At the same time, digital media and especially computer games are entertainment products with clinical relevance.
Various studies suggest that the use of digital media can also affect children negatively in their physical, social, and psychological development [(19–29); for current reviews, see (30–32)].
Especially video gaming has increased enormously in recent years (33). Gentile et al. (34) showed in a longitudinal design that more time spent gaming is a significant predictor of a later Gaming Disorder (GD). The Internet Gaming Disorder (IGD) was first recorded in the DSM-5 and is characterized by nine diagnostic criteria: excessive involvement in Internet gaming, withdrawal symptoms, tolerance development, loss of interest in previous activities, loss of control, continued gaming despite knowledge of negative consequences, deceiving significant caregivers about the gaming's extent, use of Internet gaming to end or reduce negative feelings, and endangerment or loss of an important relationship (35). Furthermore, GD has now been included in the ICD-11 (36) which includes the main diagnostic criteria listed in the DSM-5. Whereas, population-based studies estimate the representative prevalence of IGD at 2% [a mean value of representative studies from different countries: Germany, the Netherlands, Norway, Spain, UK, the USA, and one Europe-wide study; for a systematic review, see (32)], some studies conclude that the prevalence in schoolchildren is about 8–9% or more (34, 37, 38) and likewise in healthcare utilization groups.
High prevalence rates, increased usage times, dynamic development of digital technology, and the physical, social, and psychological consequences of GD pose an increasingly important public health problem in the understanding of the development and etiology of GD, including risk factors and precursors to GD. Although playing video games seems to be more and more integrated in everyday life, there is little research on the risks of the GD development in schoolchildren.
Studies have found indications that ED seems to have a significant impact on media usage and GD (12, 39–41). Hollett and Harris (40) investigated the relationship between ED and problematic video gaming using a sample of 928 adult subjects. They identified two dimensions of ED, i.e., difficulties with impulse control and limited access to emotion-regulation strategies, as significant predictors of problematic video gaming. Hormes et al. (42) assumed in undergraduate students (20 years of age) that disordered online social networking (“craving Facebook”) endorsed more ED, including non-acceptance of emotional responses, reduced emotion regulation strategies, and poor impulse control. In addition, Wichstrøm et al. (12) also found indications that symptoms of GD in 10-year-olds could be predicted by social skills and emotion regulation deficits in children at age 8, a rare research result in childhood.
Despite the increased research interest in the correlation of ED symptoms in children and the development of GD in adolescents, to our knowledge, no study has investigated whether ED symptoms in preschool age can predict GD or GD symptoms in school age. Our basic assumption is that the relation between ED and GD starts even earlier in development.
More specifically, we investigated the following hypotheses:
1. Preschool-aged children with ED meet the criteria of GD or show higher GD symptom scores in school age compared with children without ED in pre-school age.
2. Children with ED in preschool age will use media in school age longer than children without ED at preschool age. The duration of media use is a significant predictor for the presence of a GD in school age.
Materials and Methods
Participants
Participation in the study was voluntary and there was no financial compensation. All children and their parents gave informed consent. The local ethics committee approved the study.
In the present study, we used a quasi-experimental design with two measurement points. The study group included all young children who had attended the preschool special outpatient clinic of a Clinic for Child and Adolescent Psychiatry between 2011 and 2017, regardless of the diagnosis made (measuring point time t1). These families were contacted again at measurement time t2 (at the end of 2019) with a cover letter and a questionnaire. Of the original 148 children, 25 families could not be reached at t2. Of the remaining 123 children, 10 families expressed no interest, 113 families confirmed their participation by telephone, of which 33 did not return the documents despite repeated reminders and inquiries (for a schematic overview of the methodical approach, see Figure 1). The study was finally conducted with N = 80 (70% of the original children) subjects (63.7% male; mean age (t1) = 4.2, SD = 1.23, min = 1.4, max = 6.9). These 80 patients were contacted again at the end of 2018 (mean age = 9.2, SD = 2.03, min = 4.6, max = 13); there was no further personal patient presentation at measuring time t2. The average time difference t1 – t2 is therefore M = 4.9 years (SD = 1.64).
Because all the children had previously attended child and adolescent psychiatry, most participants (N = 76) in the study at t1 had a diagnosis with at least one disorder defined by ICD-10. The most frequent diagnosis was that of the Oppositional Defiant Disorder with N = 31 (ICD-10: F91.3), followed by Attention Deficit Hyperactivity Disorder with N = 21 (ICD-10: F90). In addition, N = 19 children suffer from non-organic insomnia (ICD-10: F51), N = 15 children suffer from specific developmental disorder of motor function (ICD-10: F82), and N = 13 children had a diagnosis of specific speech articulation disorder (ICD-10: F80.0). Other diagnoses that were given multiple times include the expressive language disorder with N = 10 (ICD-10: F80.1), the receptive language disorder with N = 4 (ICD-10: F80.2), feeding disorder of infancy and childhood with N = 6 (ICD-10: F98.2), and social anxiety disorder of childhood with N = 4 (ICD-10: F93.2).
In addition, IQ data were available for 71 of 80 subjects in t1 (mean IQ (t1) = 100.61, SD = 17.16). Due to the large variability in age, different test procedures were used to measure IQ [WIPPSI-III (56.3%), K-ABC-II (13.8%), SON-R 2,5–7 (8.8%), and other tests (21.2%)].
At t2, most children were attending elementary school (51%), followed by high school (19%), community schools (13%), day care (10%), special schools (6%), and Waldorf schools (1%). The majority of the children (68%) are living with both their biological parents; 24% of them are living with only their mother (and her partner) and one child is living with only its father (and his partner). Also, 5% of the children are living in foster care and 3% are living in a different living situation. N = 11 (13.8%) of the participants were taking medication, including Methylphenidate (3.8%), Atomoxetine (1.3%), or other medication (11.3%), including Asthma spray (1.3%), Guanfacine (1.3%), Opipramol (1.3%), Melatonin (1.3%), MTX (1.3%), Naproxen (1.3%), Dekristol (1.3%), Sulgen (1.3%), Vomex, and Salbutamol (1.3%).
Instruments and Procedure
Assessment of Emotional Dysregulation
We used the Child Behavior Checklist [CBCL 1½−5; (43)] to assess ED in pre-school children at t1. CBCL 1½−5 is one of the most commonly used tools for assessing developmental psychopathology in children and adolescents (43–47). From the 99 items of CBCL 1½−5, seven scales (Emotional Reactivity; Anxiety/Depressive; Physical Complaints; Social Withdrawal; Sleep Problems; Attention Problems and Aggressive Behavior) and three superordinate scales are formed, which represent External, Internal, and Total Problem Score. Good reliability and validity have been reported for the CBCL/1.5–5; Cronbach's alpha of the superordinate scales is above 0.86 (43). We assessed deficits in emotional regulation with the Deficient Emotional Self-Regulation Profile (DESR), which is characterized by simultaneous increases (between 1 and 2 SDs) on the three syndrome scales anxiety/depression, aggression, and attention (48, 49). Furthermore, we used the Child Behavior Checklist—Dysregulation Profile (CBCL-DP) as a more severe form of the ED profile. CBCL-DP is described by simultaneous extreme values (more than 2 SD) on the three syndrome scales [(49–51), S. 192]. For categorization, the respective T-values were used, T-value >60 and <71 for DESR profile and T-value >70 for Dysregulation profile. In general, the DESR profile, as well as the CBCL-DP, are established diagnostic procedures for identifying ED (50–55).
Assessment of Media Usage Behavior
At t2, parents were sent a questionnaire assessing media use and GD. The questionnaire included items referring to media use (i.e., time spent with TV and computer or video games during the week and on weekends, availability of computer access, child's ownership of a video game console or a handheld video game) and items measuring GD. To assess media usage time, parents were asked how long their child used electronic devices such as computers, laptops, smartphones, tablets, or game consoles on average every day—separately for working days and the weekend (with the following response categories: 0, 1–30, and 30–60 min; 1–2, 2–3, 3–5 h, 5–7 h, and more than 7 h). The calculation of the media usage time in minutes was done by determining the respective category mean (0 min, 15 min, 45 min, 90 min, 150 min, 240 min, 360 min) and 450 min for “more than 7 h” at the presumption, that the mid point of each interval is used as the best and most robust estimate of the answer category.
For GD, parents should answer nine questions (e.g., “Does your child become restless, irritable, moody, angry, anxious or sad when he or she has no opportunity to play?”) on a four-level Likert scale from “never” to “always.” The questions were formulated according to the criteria proposed by Petry et al. (56), with each question comprising one of the DSM-5 criteria relating to IGD. A total score was calculated by adding up the nine items, and mean values by dividing the sum value by nine; missing items were replaced by mean values. To assess the performance of nine GD-items and the GD total score, an item analysis was performed and the reliability was determined. Reliability was high with Cronbach's α = 0.883. As confirmed by a reliability analysis, total reliability did not increase significantly by eliminating any of the nine items. In addition, discriminatory power analyses ensured a value of r >0.5 for every item. Thus, all nine items remained in the scale for the calculations. Since each item of the questionnaire includes one of the DSM-5 criteria regarding IGD, the DSM-5 classification criteria were applied, according to which at least five of the nine items must be fulfilled to comply with GD. An item was considered fulfilled if either “often” or “always” was selected on the four-level Likert scale (categorical value: GD yes or not). Besides this categorical assessment (GD: yes or no), a dimensional conceptualization of GD was calculated by adding up the nine items for a GD symptom score (never = 1; sometimes = 2; often = 3; always = 4 with individual values between 9 and 36). For more details on psychometrics properties of the used nine GD-items, see Table 1.
Statistical Analysis
Data were analyzed by IBM SPSS Statistics Version 26. Categorical variables were analyzed by Fisher–Yates test (ED and media devices) and binary logistic regression (ED as predictor of a future GD diagnosis). T-tests were performed for group analyses of continuous variables. If the requirements for a t-test for independent samples were not met, a Mann–Whitney U-test was calculated (ED and ordinally scaled daily average media usage). For modeling the relationships within the data, univariate linear regression was conducted (ED as predictor of media usage times, media usage times as predictor of GD). A significance level of 0.05 was used for all statistical tests.
Results
Emotional Dysregulation
N = 11 children (14%) had deficits in the regulation of emotions (mean age = 8.91 years, SD = 1.49, min = 7.08, max = 11.25). Of these, N = 7 (9% of all participants) showed increased values of between 1 and 2 SDs on the three syndrome scales anxiety/depression, aggression, and attention of CBCL, thus fulfilling the criteria of the Deficient Emotional Self-Regulation Profile (DESR). N = 4 children (5%) showed simultaneous extreme values (more than 2 SD) on the three syndrome scales and thus corresponded to the CBCL-DP. The gender comparison showed that N = 9 boys (18%) and N = 2 girls (7%) had deficits in the regulation of emotions. However, this descriptively discernible difference of ED between boys and girls did not reach statistical significance (Fisher's exact test: p = 0.31). With regard to medication, only one child with ED was taking medication (i.e., Opipramol). All children with ED (N = 11) had at least one disorder defined by ICD-10. The most common diagnosis was the conduct disorder with oppositional defiant behavior (ICD-10: F91.3; N = 8), followed by attention deficit hyperactivity disorder (ICD-10: F90.0; N = 5). For more details on comorbidities of children with ED, see Tables 2, 3.
Access to Media Devices and Usage Time
In terms of access to a computer/laptop (p = 0.974), smartphone (p = 0.933), Smartwatch (p = 0.362), tablet (p = 0.751), stationary game console (p = 0.169), portable game console (p = 0.726), and television (p = 0.100), there were no significant differences between children with and without ED (for more details, see Table 4). As shown in Figures 2, 3, children with deficient emotional regulation used media significantly longer on weekdays (U = 211.5, Z = − 2,413, p < 0.05) as well as on weekends (U = 214.5, Z = − 2.36, p < 0.05) than children without ED, confirming the second hypothesis. The calculation of media usage time at t2 presented children with at t1 diagnosed ED to have elevated usage times compared with those cases, when an ED was not determined. On weekdays, children with ED used media for averagely 124 min per day (vs. 68 min for children without ED, p = 0.003). On the weekend, media was used more frequently by children with ED, averaging 209 min per day (vs. 129 min for children without ED, p = 0.019). For details, see Table 5.
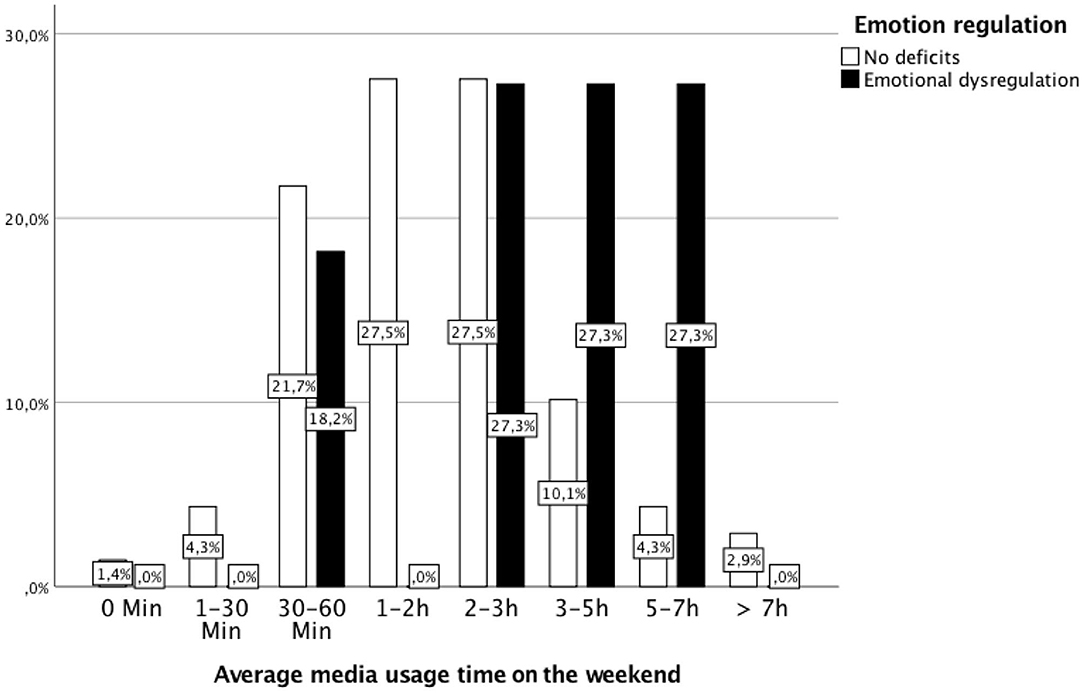
Figure 2. Daily average media usage time at the weekend by children with ED and without ED. Children with ED used media significantly longer at weekends than children without ED (Mann–Whitney U-test: Mrank with ED = 55.50, Mrank without ED = 38.11; U = 214.5, Z = −2.36, p < 0.018).
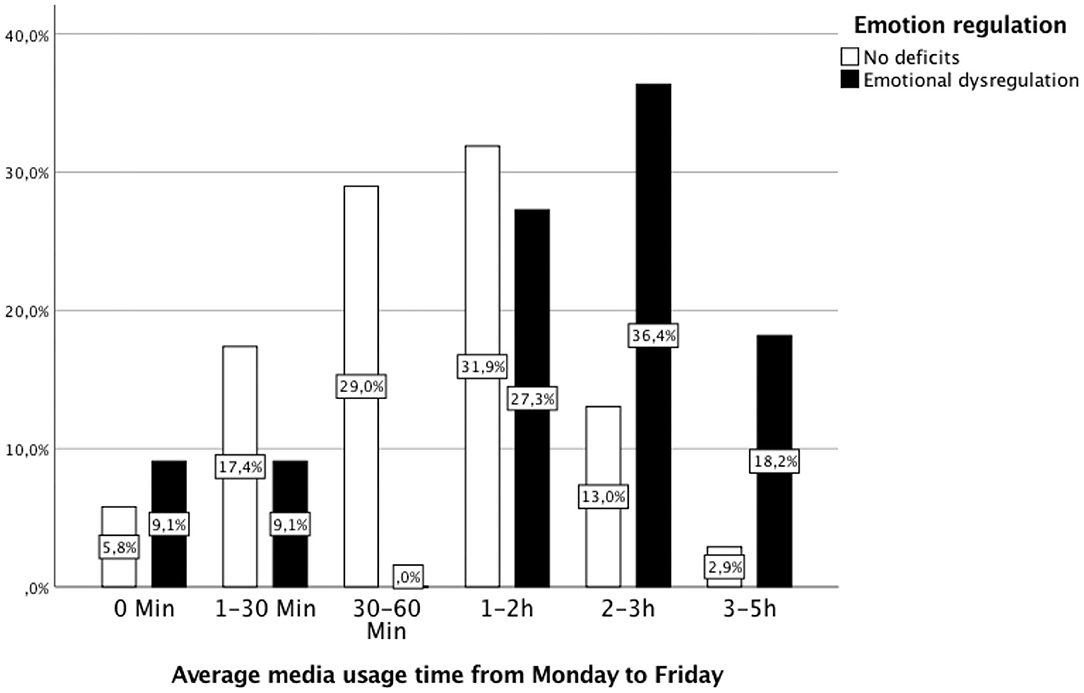
Figure 3. Daily average media usage time from Monday to Friday by children with ED and without ED. Children with ED used media significantly longer on weekdays than children without ED (Mann–Whitney U-test: Mrank with ED = 55.77, Mrank without ED = 38.07; U = 211.5, Z = −2.41, p = 0.016).
Based on the finding that longer usage times (34) as well as ED (39, 40) are significant predictors of GD, we investigated exploratively whether ED at t1 is also predictive for longer media usage times at t2. A linear regression with dimensional conceptualization of ED as predictor and the metric scaled variable of the subjects' duration of media use during the daily average as criterion showed that preschool ED at t1 significantly predicts usage times of digital media use per week (F(1) = 8.698, p = 0.004, R2 = 0.100, R2 adjusted = 0.089) 5 years later at t2. To test our second hypothesis, that duration of media use is a predictor of a GD, further linear regression analyses were carried out, which showed that the time criterion of media usage behavior is a significant predictor for the presence of a GD dimensionally (F(1, 78) = 22.863, p = 0.000, R2 = 0.227, R2 adjusted = 0.217). Categorically, it did not explain a significant amount of the variance in GD (F(1, 78) = 2.679, p = 0.106, R2 = 0.033, R2 adjusted = 0.021).
Media Use Behavior
If gaming behavior is assessed dimensionally and the mean values of the two groups are compared, a descriptive difference between children with ED (M = 15.82, SD = 4.08) and children without ED (M = 11.71, SD = 5.05) is already apparent. This difference is also statistically significant (t = −3,0; p =0.004; d = 0.895). However, if gaming behavior is categorically classified according to the DSM-5 criteria for GD (diagnosis when >4 criteria from 9), only one of the 11 children with ED (9.09%) and three of the 69 children without ED (4.35%) met the criteria for a diagnosis of GD. A binary logistic regression showed no significant correlation between ED and future GD diagnosis (Wald(1) = 31.989, p = 0.51; n.s.). The differences between children with ED and children without ED on dimensional and categorical assessment of gaming disorder are listed in Table 6.
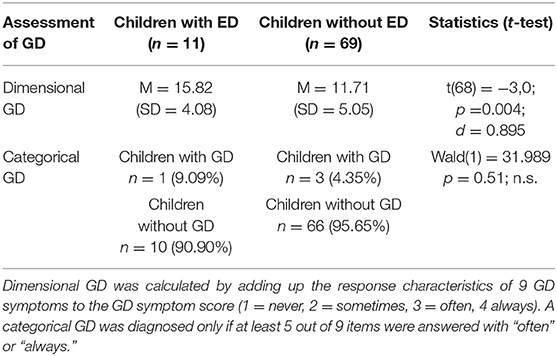
Table 6. Differences between children with emotional dysregulation and children without emotional dysregulation on dimensional and categorical assessment of gaming disorder.
Discussion
In recent years, the everyday use of digital media and especially the increase in playing video games seems to have a huge, and potentially negative, impact on child and adolescent development, to the point of a manifest GD (35, 36). A potential factor, which probably affects the development of GD, is ED (12, 40). All studies carried out on this subject so far investigated the influence of ED in schoolchildren, adolescents, or young adults on the development of GD. To the best of our knowledge, this is the first study to examine the impact of preschool ED on media use and GD in later childhood. To potentially find early prevention possibilities, we conducted the study with the aim of examining the predictive value of ED in preschool age for the development of GD in school age.
In summary, the results show higher GD symptom scores (dimensionally) in school age for children with preschool ED compared with children without ED in preschool age. ED does not predict a diagnosis of GD (categorically) (hypothesis 1). Children with ED at preschool age have significantly longer media use times 5 years later, than preschool children without emotion regulation difficulties. Temporal excessive video game playing behavior at school age is correlated with higher GD symptom scores (dimensionally), but not with the presence or absence of a GD diagnosis (categorically) (hypothesis 2).
It amounts to a difference between a dimensional and a categorical approach to GD. ED in preschool age, as well as screen time use predict higher GD symptom scores on a dimensional scale, but do not predict a GD diagnosis on a categorical scale. Possible explanations for these differences could lie in (a) larger required samples to yield sufficient statistical power. (b) It may be speculated that the GD criteria of the DSM-5 do not apply as well to the according age group (9 years) compared with adolescents or young adults (developmental adequacy). The item analysis of the nine GD criteria supports this speculation: the means lie in the lower range (see Table 1). The item difficulties are all with one exception under 0.20. (c) A reflection of the differences between the dimensional and the categorical approach of diagnostic and psychopathology is fundamental. A dimensional approach allows the clinician more latitude in assessing the severity of a condition and does not imply a concrete threshold between “normality” and the disorder, such as GD (57–59).
The reported increase of usage time in children with ED can be attributed to the ED profile because in general, children with psychiatric disorders use digital media significantly longer (60). Children with ED may use video game playing as a maladaptive coping strategy to deal with negative emotions. Because ED is characterized by difficulties in understanding and accepting unpleasant emotions, it is assumed that children with ED often try to avoid such emotional experiences, e.g., by using excessive video gaming to escape psychological difficulties (10, 11, 40, 42). Therefore, young children with ED should be seen as being at risk of developing a GD. This risk may be further increased by the game-associated induction of positive feelings. During the game, children and adolescents feel particularly free and heroic, which in turn leads to a stabilization of their self-esteem. They have a direct sense of achievement and self-efficacy, make social contacts more easily than in real life, and are able to escape the emotional difficulties of the real world, whereas the latter may be a maladaptive coping strategy leading to more excessive gaming (10). Considering all these aspects, in regard to future prevention, it could be a promising approach to limit the time spent on video games. More specifically, children prone to difficulties in dealing with negative emotions should be restricted or monitored more closely in their video game playing behavior. This falls in line with the proposition of Donald et al. (61) to reduce video gaming by restricting access to devices (despite them having considered ED as a result of GD instead of ED predicting GD, as we have found). At the same time, more adequate coping and action alternatives should be offered that both act as an adaptive strategy for dealing with negative emotions and can contribute to experiencing positive feelings.
Furthermore, in the context of the assessment of our second hypothesis, the analyses show that the time criterion of media usage behavior in school age is associated with the existence of a GD, in dimensional GD conceptualization. Therefore, our second hypothesis and the findings from Gentile et al. (34) could be confirmed, whereby the time criterion could be assumed as a manifest risk factor for the development of a GD. We conclude that the time aspect of video game playing behavior should be considered as playing a more prominent role in the development of GD. The tolerance development in DSM-5 is time associated (e.g., feeling the need to play for increasing amounts of time, augmentation of play time). This assumption is strengthened by various findings of other authors, which show a significant association between the usage time of video games and GD (62–66). Again, the time limitation of video game usage behavior, as well as the usage of alternative and adaptive activities, could be useful for the prevention of GD. To our knowledge, there are no efficient studies of possible prevention measures for GD in the context of ED. Therefore, it would be of great interest to undertake further research on prevention measures, e.g., time limitation. Studies indicate that daily use of digital media is not limited to school age and adolescence but also widespread in pre-school age, e.g., Vandewater et al. (67) reported 16% of 5–6-year-old pre-school children playing video games daily. Mendoza et al. (68) reported that already 2–5-year-old children used a computer daily. Thus, effectiveness studies on prevention programs for GD in the context of ED would also be highly relevant for children of younger age. To explore the relation between ED and GD (hypothesis 1), we used a dimensional (i.e., symptom score of GD), as well as a categorical (i.e., diagnosis of a GD), assessment of this construct. In the dimensional assessment of video game playing behavior, children with ED in preschool age show a higher GD symptom score at school age than those without ED. These findings largely confirm our hypothesis 1, as do the findings of Hollett and Harris (40) and Wichstrøm et al. (12). These findings suggests that early detection and treatment of ED could have a preventive effect on the development of GD. Especially in young age, increasing social support with a family- and parent-based approach could reduce Internet addiction (41). Nevertheless, to make more detailed statements in this regard, further research is needed.
Limitations
One restriction of the present study is the limitation to parental reports. Parents may report their children spending less time using media, either because of underestimation or because of omitting time spent on media outside home, e.g., with friends. They may also react biased toward social desirability, being aware that their children should not spend that much time online. Furthermore, for future research it would be interesting to consider parents' media use as a mediator variable.
Another limitation of this study is that we only assessed the total usage time of modern electronic media, without differentiating for computer/laptop, smartphone, smartwatch, tablet, gaming consoles, and television. It was also not differentiated between pedagogically valuable content and problematic content. For the calculation of the media usage time in minutes based on the assessed answer categories, we performed a transformation of each category in an average value in minutes with the presumption, that the mid point of the interval serves as the best estimate of the answer category.
Our study does not provide any data on socioeconomic status (SES) and psychiatric family history; therefore, an influence of SES and psychiatric family history can neither be demonstrated nor excluded. Patients of our preschool program are living in the surrounding regions which are characterized by a quite homogenous socioeconomic status. Future studies considering SES and psychiatric family history as an important variable are underway.
In addition, the group of children actually meeting criteria for ED was small. Retention rate from the original sample was 70% only; however, a time span of 5 years was covered. Also limiting this study in its generalizability is the fact that we investigated a clinical sample. Finally, our study uses a quasi-experimental design. It would be of great value to conduct an a priori defined experimental long-term study with a larger sample, in which the course of possible development of GD in the context of ED would be recorded. In addition, the gender comparison in children with ED did not reach statistical significance and medication use was also unevenly distributed across the sample, which is probably due to the small size of the sample. Therefore, a larger sample would possibly also allow the examination of these variables as covariates in statistical analyses, which would allow the results of the present study to be illuminated against the background of further potential influencing variables.
Conclusion
Our results suggest that preschool ED symptoms and duration of media use predict a higher score of GD symptoms in schoolchildren. Therefore, identifying ED could be the first step for parents to reduce the likelihood for the emergence of GD in schoolchildren. For example, parents should strive to convey the value of self-control and offer training in self-reflection with the aim of promoting self-regulated behavior. Therefore, one noteworthy strength of the present study is that it explores the rarely investigated and relevant area of risk factors of children's video gaming behavior. Excessive computer use and GD becomes a dysfunctional solution or an inadequate coping for pre-existing ED. Therefore, preventing ED contributes to the prevention of GD.
Regardless of the significant associations between ED in pre-school and later media use and GD in childhood, the question of the relationship between ED and ICD-10 nosology arises, which has not been clarified as yet. Would ED be the “Grand Unifying Theory” of psychological symptoms and disorders?
Data Availability Statement
The raw data supporting the conclusions of this article will be made available by the authors, without undue reservation.
Ethics Statement
The studies involving human participants were reviewed and approved by Ethikkommission der Ärztekammer des Saarlandes Ha 147/19. Written informed consent to participate in this study was provided by the participants' legal guardian/next of kin.
Author Contributions
FP: conceptualization, implementation, data collection, statistical analysis, text creation, and discussion. KH: data collection, data entry, and ethics application. FM: statistical analyses and text creation. EM: discussion and correction. All authors contributed to the article and approved the submitted version.
Conflict of Interest
The authors declare that the research was conducted in the absence of any commercial or financial relationships that could be construed as a potential conflict of interest.
References
1. Gratz KL, Roemer L. Multidimensional assessment of emotion regulation and dysregulation: development, factor structure, and initial validation of the difficulties in emotion regulation scale. J Psychopathol Behav Assess. (2004) 26:41–54. doi: 10.1023/B:JOBA.00000074550853994
2. Menna R, Landy S. Working with parents of aggressive preschoolers: an integrative approach to treatment. J Clin Psychol. (2001) 57:257–69. doi: 10.1002/1097-4679(200102)57:2<257::AID-JCLP10>30CO;2-3
3. Harris JS, Stewart DG, Krzyzaniak SL, Charuhas JP, Moon KC, Holdren AL, et al. Binge drinking despite consequences: the role of psychological dysregulation. J Child Adolesc Substance Abuse. (2016) 26:103–10. doi: 10.1080/1067828X20161222979
4. Fox HC, Axelrod SR, Paliwal P, Sleeper J, Sinha R. Difficulties in emotion regulation and impulse control during cocaine abstinence. Drug Alcohol Depend. (2007) 89:298–301. doi: 10.1016/j.drugalcdep.200612026
5. Fox HC, Hong KA, Sinha R. Difficulties in emotion regulation and impulse control in recently abstinent alcoholics compared with social drinkers. Addict Behav. (2008) 33:388–94. doi: 10.1016/j.addbeh.200710002
6. Weinstein SM, Mermelstein R, Shiffman S, Flay B. Mood variability and cigarette smoking escalation among adolescents. Psychol Addict Behav. (2008) 22:504. doi: 10.1037/0893-164X.224504
7. Wills TA, Simons JS, Sussman S, Knight R. Emotional self-control and dysregulation: a dual-process analysis of pathways to externalizing/internalizing symptomatology and positive well-being in younger adolescents. Drug Alcohol Depend. (2016) 163:S37–45. doi: 10.1016/j.drugalcdep.201508039
8. Wilens TE, Martelon M, Anderson JP, Shelley-Abrahamson R, Biederman J. Difficulties in emotional regulation and substance use disorders: a controlled family study of bipolar adolescents. Drug Alcohol Depend. (2013) 132:114–21. doi: 10.1016/j.drugalcdep.201301015
9. Williams AD, Grisham JR, Erskine A, Cassedy E. Deficits in emotion regulation associated with pathological gambling. Br J Clin Psychol. (2012) 51:23–8. doi: 10.1111/j.2044-8260.201102022x
10. Di Blasi M, Giardina A, Giordano C, Lo Coco G, Tosto C, Billieux J, et al. Problematic video game use as an emotional coping strategy: evidence from a sample of MMORPG gamers. J Behav Addict. (2019) 8:25–34. doi: 10.1556/2006.8.2019.02
11. Yu JJ, Kim H, Hay I. Understanding adolescents' problematic Internet use from a social/cognitive and addiction research framework. Comput Hum Behav. (2013) 29:2682–9. doi: 10.1016/j.chb.201306045
12. Wichstrøm L, Stenseng F, Belsky J, von Soest T, Hygen BW. Symptoms of internet gaming disorder in youth: predictors and comorbidity. J Abnorm Child Psychol. (2019) 47:71–83. doi: 10.1007/s10802-018-0422-x
13. Anderson DR, Huston AC, Schmitt KL, Linebarger DL, Wright JC, Larson R. Early childhood television viewing and adolescent behavior: the recontact study. Monogr Soc Res Child Dev. (2001) 66:1–147. doi: 10.1111/1540-5834.00120
14. Barr R, Wyss N. Reenactment of televised content by 2-year olds: toddlers use language learned from television to solve a difficult imitation problem. Infant Behav Dev. (2008) 31:696–703. doi: 10.1016/j.infbeh.200804006
15. Caviola S, Gerotto G, Mammarella IC. Computer-based training for improving mental calculation in third-and fifth-graders. Acta Psychol. (2016) 171:118–27. doi: 10.1016/j.actpsy.201610005
16. Li X, Atkins MS. Early childhood computer experience and cognitive and motor development. Pediatrics. (2004) 113:1715–22. doi: 10.1542/peds.11361715
17. Linebarger DL, Walker D. Infants' and toddlers' television viewing and language outcomes. Am Behav Sci. (2005) 48:624–45. doi: 10.1177/0002764204271505
18. Kwok K, Ghrear S, Li V, Haddock T, Coleman P, Birch SAJ. Children can learn new facts equally well from interactive media versus face to face instruction. Front Psychol. (2016) 7:1603. doi: 10.3389/fpsyg201601603
19. Cheng S, Maeda T, Yoichi S, Yamagata Z, Tomiwa K, Japan Children's Study Group. Early television exposure and children's behavioral and social outcomes at age 30 months. J Epidemiol. (2010) 20:482–9. doi: 10.2188/jeaJE20090179
20. Chonchaiya W, Pruksananonda C. Television viewing associates with delayed language development. Acta Paediatr. (2008) 97:977–82. doi: 10.1111/j.1651-2227.200800831x
21. Chonchaiya W, Sirachairat C, Vijakkhana N, Wilaisakditipakorn T, Pruksananonda C. Elevated background TV exposure over time increases behavioural scores of 18-month-old toddlers. Acta Paediatr. (2015) 104:1039–46. doi: 10.1111/apa13067
22. Christakis DA, Zimmerman FJ, DiGiuseppe DL, McCarty CA. Early television exposure and subsequent attentional problems in children. Pediatrics. (2004) 113:708–13. doi: 10.1542/peds.1134708
23. Cox R, Skouteris H, Rutherford L, Fuller-Tyszkiewicz M, Dell D, Hardy LL. Television viewing, television content, food intake, physical activity and body mass index: a cross-sectional study of pre-school children aged 2-6 years. Health Promot J Aust. (2012) 23:58–62. doi: 10.1071/HE12058
24. Garrison MM, Liekweg K, Christakis DA. Media use and child sleep: the impact of content, timing, and environment. Pediatrics. (2011) 128:29–35. doi: 10.1542/peds2010-3304
25. Hinkley T, Verbestel V, Ahrens W, Lissner L, Molnár D, Moreno LA, et al. Early childhood electronic media use as a predictor of poorer well-being: a prospective cohort study. JAMA Pediatr. (2014) 168:485–92. doi: 10.1001/jamapediatrics201494
26. Poulain T, Vogel M, Neef M, Abicht F, Hilbert A, Genuneit J, et al. Reciprocal associations between electronic media use and behavioral difficulties in preschoolers. Int J Environ Res Public Health. (2018) 15:814. doi: 10.3390/ijerph15040814
27. Radesky JS, Silverstein M, Zuckerman B, Christakis DA. Infant self-regulation and early childhood media exposure. Pediatrics. (2014) 133:e1172–8. doi: 10.1542/peds2013-2367
28. Takeuchi H, Taki Y, Hashizume H, Hashizume H, Asano K, Asano M., et al. The impact of television viewing on brain structures: cross-sectional and longitudinal analyses. Cereb Cortex. (2015) 25:1188–97. doi: 10.1093/cercor/bht315
29. Zhang G, Wu L, Zhou L, Lu W, Mao C. Television watching and risk of childhood obesity: a meta-analysis. Eur J Public Health. (2015) 26:13–8. doi: 10.1093/eurpub/ckv213
30. Anderson CA, Shibuya A, Ihori N, Swing EL, Bushman BJ, Sakamoto A, et al. Violent video game effects on aggression, empathy, and prosocial behavior in eastern and western countries: a meta-analytic review. Psychol Bull. (2010) 136:151–73. doi: 10.1037/a0018251
31. King DL, Delfabbro PH, Perales JC, Deleuze J., Király O, Krossbakken E, et al. Maladaptive player-game relationships in problematic gaming and gaming disorder: a systematic review. Clin Psychol Rev. (2019) 73:101777. doi: 10.1016/j.cpr2019101777
32. Paulus FW, Ohmann S, Von G.ontard A, Popow C. Internet gaming disorder in children and adolescents: a systematic review. Dev Med Child Neurol. (2018) 60:645–59. doi: 10.1111/dmcn13754
33. Rideout VJ, Foehr UG, Roberts DF. Generation M2: Media in the Lives of 8- to 18-Year-Olds. Oakland, CA: Henry J. Kaiser Family Foundation (2010).
34. Gentile DA, Choo H, Liau A, Sim T, Li D, Fung D, et al. Pathological video game use among youths: a two-year longitudinal study. Pediatrics. (2011) 127:e319–29. doi: 10.1542/peds2010-1353
35. American Psychiatric Association (2013). Diagnostic and Statistical Manual of Mental Disorders. 5th Edn. Washington: American Psychiatric Association. doi: 10.1176/appibooks9780890425596
36. World Health Organization (2017). International Classification of Diseases 11th Revision ICD-11 Beta Draft. Available online at: https://icd.who.int/dev11/l-m/en (accessed 23 September 2020).
37. Gentile DA. Pathological video-game use among youth ages 8 to 18. Psychol Sci. (2009) 20:5. doi: 10.1111/j.1467-9280.200902340x
38. Mihara S, Higuchi S. Cross-sectional and longitudinal epidemiological studies of internet gaming disorder: a systematic review of literature. Psychiatry Clin Neurosci. (2017) 71:425–44. doi: 10.1111/pcn12532
39. Casale S, Caplan SE, Fioravanti G. Positive metacognitions about Internet use: the mediating role in the relationship between emotional dysregulation and problematic use. Addict Behav. (2016) 59:84–8. doi: 10.1016/j.addbeh.201603014
40. Hollett KB, Harris N. Dimensions of emotion dysregulation associated with problem video gaming. Addict Res Theory. (2019) 28:38–45. doi: 10.1080/1606635920191579801
41. Mo PKH, Chan VWY, Chan SW, Lau JTF. The role of social support on emotion dysregulation and internet addiction among Chinese adolescents: a structural equation model. Addict Behav. (2018) 82:86–93. doi: 10.1016/j.addbeh.201801027
42. Hormes JM, Kearns B, Timko CA. Craving Facebook? Behavioral addiction to online social networking and its association with emotion regulation deficits. Addiction. (2014) 109:2079–88. doi: 10.1111/add12713
43. Achenbach TM, Rescorla LA. (2000) Child Behavior Checklist for Ages 1 1/2-5. Burlington, VT: ASEBA, University of Vermont. doi: 10.1037/10517-028
44. Biederman J, Petty CR, Day H, Goldin RL, Spencer T, Faraone SV, et al. Severity of the aggression/anxiety-depression/attention (AAA) CBCL profile discriminates between different levels of deficits in emotional regulation in youth with ADHD. J Dev Behav Pediatr. (2012) 33:236. doi: 10.1097/DBP0b013e3182475267
45. Dölitzsch C, Kölch M, Fegert JM, Schmeck K, Schmid M. Ability of child behavior checklist-dysregulation profile and the youth self report-dysregulation profile to identify serious psychopathology and association with correlated problems in high-risk children and adolescents. J Affect Disord. (2016) 205:327–34. doi: 10.1016/j.jad.201608010
46. Holtmann M, Buchmann AF, Esser G, Schmidt MH, Banaschewski T, Laucht M. The Child Behavior Checklist-Dysregulation Profile predicts substance use, suicidality, and functional impairment: a longitudinal analysis. J Child Psychol Psychiatry. (2011) 52:139–47. doi: 10.1111/j.1469-7610.201002309x
47. Papachristou E, Schulz K, Newcorn J, Bédard ACV, Halperin JM, Frangou S. Comparative evaluation of child behavior checklist-derived scales in children clinically referred for emotional and behavioral dysregulation. Front Psychiatry. (2016) 7:146. doi: 10.3389/fpsyt201600146
48. Hudziak JJ, Althoff RR, Derks EM, Faraone SV, Boomsma DI. Prevalence and genetic architecture of Child Behavior Checklist–juvenile bipolar disorder. Biol Psychiatry. (2005) 58:562–8. doi: 10.1016/j.biopsych.200503024
49. Masi G, Muratori P, Manfredi A, Pisano S, Milone A. Child behaviour checklist emotional dysregulation profiles in youth with disruptive behaviour disorders: clinical correlates and treatment implications. Psychiatry Res. (2015) 225:191–6. doi: 10.1016/j.psychres.201411019
50. Althoff RR, Verhulst F, Rettew DC, Hudziak JJ, van der Ende J. Adult outcome of childhood dysregulation: a 14-year follow-up study. J Am Acad Child Adolesc Psychiatry. (2010) 49:1105–16. doi: 10.1016/j.jaac.201008006
51. Kim J, Carlson GA, Meyer SE, Bufferd SJ, Dougherty LR, Dysin MW, et al. Correlates of the CBCL-dysregulation profile in preschool-aged children. J Child Psychol Psychiatry. (2012) 53:918–26. doi: 10.1111/j.1469-7610.201202546x
52. Bellani M, Negri GAL, Brambilla P. The dysregulation profile in children and adolescents: a potential index for major psychopathology? Epidemiol Psychiatr Sci. (2012) 21:155–9. doi: 10.1017/S2045796011000849
53. Biederman J, Chan J, Faraone SV, Woodworth KY, Spencer TJ, Wozniak JR. A familial risk analysis of emotional dysregulation: a controlled study. J Atten Disord. (2018) 22:848–54. doi: 10.1177/1087054715596576
54. De Caluwe E, Decuyper M, De Clercq B. The child behavior checklist dysregulation profile predicts adolescents DSM-5 pathological personality traits 4 years later. Eur Child Adolesc Psychiatry. (2013) 22:401–11. doi: 10.1007/s00787-013-0379-9
55. Deutz MHF, Geeraerts SB, Belsky J, Dekovic M, van Baar AL, Prinzie P, et al. General psychopathology and dysregulation profile in a longitudinal community sample: stability, antecedents and outcomes. Child Psychiatry Hum Dev. (2019) 51:114–26. doi: 10.1007/s10578-019-00916-2
56. Petry NM, Rehbein F, Gentile DA, Lemmens JS, Rumpf HJ., Mößle T, et al. An international consensus for assessing internet gaming disorder using the new DSM-5 approach. Addiction. (2014) 109:1399–406. doi: 10.1111/add12457
57. Hudziak JJ, Achenbach TM, Althoff RR, Pine DS. A dimensional approach to developmental psychopathology. Int J Methods Psychiatr Res. (2007) 16:S16–23. doi: 10.1002/mpr217
58. Krueger RF, Piasecki TM. Toward a dimensional and psychometrically-informed approach to conceptualizing psychopathology. Behav Res Ther. (2002) 40:485–99. doi: 10.1016/S0005-7967(02)00016-5
59. Moreland AD, Dumas JE. Categorical and dimensional approaches to the measurement of disruptive behavior in the pre-school years: a meta-analysis. Clin Psychol Rev. (2008) 28:1059–70. doi: 10.1016/j.cpr.200803001
60. Bear S, Bogusz E, Green DA. Stuck on screens: patterns of computer and gaming station use in youth seen in a psychiatric clinic. J Can Acad Child Adolesc Psychiatry. (2011) 20:86–94.
61. Donald JN, Ciarrochi J, Sahdra BK. The consequences of compulsion: A 4-year longitudinal study of compulsive internet use and emotion regulation difficulties. Emotion. (2020). doi: 10.1037/emo0000769. [Epub ahead of print].
62. Coeffec A, Romo L, Cheze N, Riazuelo H, Plantex S, Kotbagi G, et al. Early substance consumption and problematic use of video games in adolescence. Front Psychol. (2015) 6:501. doi: 10.3389/fpsyg.2015.00501
63. Hussain Z, Griffiths MD, Baguley T. Online gaming addiction: classification, prediction and associated risk factors. Addict Res Theory. (2012) 20:359–71. doi: 10.3109/160663592011640442
64. Kuss DJ, Griffiths MD. Internet gaming addiction: a systematic review of empirical research. Int J Ment Health Addict. (2012) 10:278–96. doi: 10.1007/s11469-011-9318-5
65. Peters CS, Malesky LA. Problematic usage among highly-engaged players of massively multiplayer online role playing games. CyberPsychol Behav. (2008) 11:140. doi: 10.1089/cpb.2007.0140
66. Paulus FW, Sinzig J, Mayer H, Weber M., von Gontard. A. Computer gaming disorder and ADHD in young children – a population-based study. Int J Ment Health Addict. (2018) 16:1193–207. doi: 10.1007/s11469-017-9841-0
67. Vandewater EA, Rideout VJ, Wartella EA, Huang X, Lee JH, Shim MS. Digital childhood: electronic media and technology use among infants, toddlers, and preschoolers. Pediatrics. (2007) 119:e1006–15. doi: 10.1542/peds2006-1804
Keywords: Emotional Dysregulation, Gaming Disorder, media use, preschool age, school age
Citation: Paulus FW, Hübler K, Mink F and Möhler E (2021) Emotional Dysregulation in Preschool Age Predicts Later Media Use and Gaming Disorder Symptoms in Childhood. Front. Psychiatry 12:626387. doi: 10.3389/fpsyt.2021.626387
Received: 05 November 2020; Accepted: 14 April 2021;
Published: 17 June 2021.
Edited by:
Li Yang, Peking University Sixth Hospital, ChinaReviewed by:
Isabelle Esther Bauer, University of Texas Health Science Center at Houston, United StatesLi Lu, INSERM U1219 Bordeaux Population Health Centre Recherche (BPH), France
Copyright © 2021 Paulus, Hübler, Mink and Möhler. This is an open-access article distributed under the terms of the Creative Commons Attribution License (CC BY). The use, distribution or reproduction in other forums is permitted, provided the original author(s) and the copyright owner(s) are credited and that the original publication in this journal is cited, in accordance with accepted academic practice. No use, distribution or reproduction is permitted which does not comply with these terms.
*Correspondence: Frank W. Paulus, frank.paulus@uks.eu