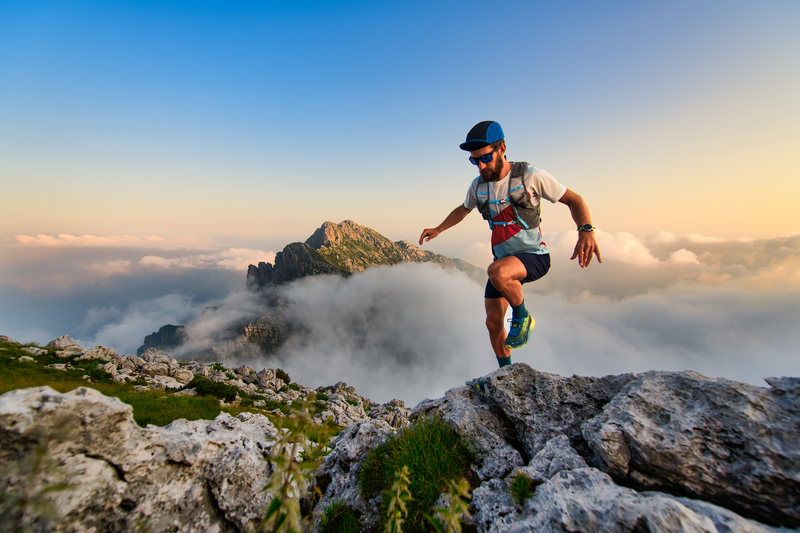
94% of researchers rate our articles as excellent or good
Learn more about the work of our research integrity team to safeguard the quality of each article we publish.
Find out more
METHODS article
Front. Psychiatry , 23 September 2021
Sec. Public Mental Health
Volume 12 - 2021 | https://doi.org/10.3389/fpsyt.2021.574440
This article is part of the Research Topic Digital Phenotyping/Digital Biomarkers to Monitor Psychiatric Disorders View all 15 articles
Artificial intelligence (AI) algorithms together with advances in data storage have recently made it possible to better characterize, predict, prevent, and treat a range of psychiatric illnesses. Amid the rapidly growing number of biological devices and the exponential accumulation of data in the mental health sector, the upcoming years are facing a need to homogenize research and development processes in academia as well as in the private sector and to centralize data into federalizing platforms. This has become even more important in light of the current global pandemic. Here, we propose an end-to-end methodology that optimizes and homogenizes digital research processes. Each step of the process is elaborated from project conception to knowledge extraction, with a focus on data analysis. The methodology is based on iterative processes, thus allowing an adaptation to the rate at which digital technologies evolve. The methodology also advocates for interdisciplinary (from mathematics to psychology) and intersectoral (from academia to the industry) collaborations to merge the gap between fundamental and applied research. We also pinpoint the ethical challenges and technical and human biases (from data recorded to the end user) associated with digital mental health. In conclusion, our work provides guidelines for upcoming digital mental health studies, which will accompany the translation of fundamental mental health research to digital technologies.
Digital health can be defined as the concept of healthcare meeting the Internet (1). It ranges from telehealth and telecare systems (2) to patient portals and personal health records (3, 4), mobile applications (5), and other online platforms and devices. However, and as opposed to digitized versions of traditional health approaches, digital health interventions (DHIs) (6) utilize artificial intelligence (AI) algorithms and other machine learning (ML) systems to monitor and predict symptoms of patients in an adaptive feedback loop (7). Improvements in ML over recent years have demonstrated potential within a variety of diseases and medical fields including neurological and mental health disorders (8) both at an individual-patient level and applied to larger populations for scalable understanding, management, and intervention of mental health conditions in different cohorts and various settings (7). In addition, and because to our knowledge, effective coverage does not exceed 50% in any country and is much lower in low- and middle-income countries, DHIs also address social problems in the healthcare system such as poor access, uncoordinated care, and increasingly heavy costs (9). Digital mental health interventions could thus give much needed attention to underresearched and undertreated populations (10).
The keywords “digital mental health” in PubMed's search engine (accessed April 2020) show that 2019 has the largest number of published articles compared to any prior year. The trend is also rising for the keywords “mental health mobile apps,” providing evidence that interest in both (i) publication of articles about digital health and (ii) technical advances is rising. Advances in digital health technologies in mental health are occurring at a rapid pace in research laboratories both in academic institutions and in the industry (11). The rapidly growing number of biological devices and the exponential accumulation of data in the mental health sector aim at facilitating the four purposes of healthcare: diagnosis, monitoring, treatment, and prevention (1).
Important digital health interventions for characterization or diagnosis include algorithms for illness detection and classification (11). One digital tool that is further revolutionizing mental healthcare is conversational AI (12). Although the clinician–AI collaborations have yet to be specified and the cognitive biases considered (see Designing digital health systems with human factors approach), a blended approach (in an AI-delivered human-supervised model) (12, 13) is alluring.
The use of data generated by personal electronic devices to monitor mental health parameters may result in useful biobehavioral markers that could in turn optimize diagnosis, treatment, and prevention and a global clinical improvement (14). This has led to the conception of all sorts of wearable devices and connected objects such as smart watches to collect data in healthy and pathological populations in a scalable unobtrusive way (15, 16), smart textiles to collect and monitor physiological outcome measure such as in athletes (17), or smart homes to monitor biophysiological measures of older people (18). This has also led to the development of various mobile applications (linked or not to a wearable device) that monitor given behaviors or cognitions in specific populations. This is the case of eMoods, a mood tracking app conceived for patients with bipolar disorders to follow their fluctuations. This is also the case of PROMIS, a mobile application to self-report different cognitive, emotional, and mood measures (19).
Beyond diagnosis and monitoring allowed mainly by data interpretation, some digital mental health interventions include assisting and treatment options (1). This is particularly timely as the Food & Drug Administration (FDA) has just approved its first prescription video game in mental health for kids with ADHD: EndeavorRx (20).
While digitized versions of classical clinical approaches propose digital conversational agents such as chatbots that provide coaching and cognitive behavioral therapies in a conceptually similar value than a human healthcare provider (7, 21), AI-based algorithms and data-driven digital health initiatives further aim at implementing more adaptive algorithms and flexible, personalized treatments via AI and ML (8, 21). Such is the case of Open Book, an assistive technology tool for adaptive, personalized text simplification for people with autism spectrum disorder (22). It is also the case of Entourage, a novel digital intervention that improves social connection for people with social anxiety symptoms (23), or Doppel, a device that helps people manage their daily stress by modulating physiological and emotional states through a heartbeat-like rhythm tactile sensation (24). Other digital mental health interventions for treatment purposes include virtual reality-based exposure [in the treatment of anxiety disorders for instance (25)] as well as the use of robotic technology [to improve social interactions in people with dementia for instance (26)].
By opening new modes of real-time assessment [through longitudinal data collection or through the presence of sensors in smartphones for instance, to track sleep, movement, speech… (27, 28)], digital mental health interventions enable catching new episodes of a given disorder at a very early stage. It is especially the case for suicide preventions (29).
In contrast, there is only scarce clinically significant outcomes of digitalized solutions. Although both advances in fundamental research and technical innovations are occurring rapidly, translation from one to the other has been slower (11). This can be explained by the lack of better-designed clinical trials and the loss of interest at the patient level in digital health products over time, both of which lead to poor long-term data and scarce information on whether new behavior facilitated by a digital health tool is long-lasting (30).
Another major problem at the time is the disparity of research and development processes across fields and sectors. One way of accelerating the potential benefits of digital mental health interventions and optimizing the transformation of fundamental discoveries into innovative digital technologies applied to routine clinical practice would be to propose a methodology that could be used across disciplines and sectors in the field of mental health. This would include homogenizing research and development processes in academia as well as in the private sector; improving technical methods that standardize, aggregate, and exchange data; centralizing data into federalizing platforms focusing on scalability; and establishing data repositories, common data standards, and collaborations (14, 31).
In March 2020, the WHO declared the novel coronavirus disease of 2019 also known as COVID-19 as a global pandemic. Today, a year later, the WHO counts 185,038,214 confirmed cases of COVID-19 globally, including 3,250,648 deaths. Amid this rapidly evolving sanitary crisis, digital innovation is being used to respond to the urgent needs of the pandemic. Actions in the field have been involving multiple stakeholders, from frontline healthcare to public health and governmental entities. They have also raised new challenges regarding the link between academia and the industry, the different velocities at which the two sectors evolve, the ethical questions of data collection, and the various geographical and socioeconomic inequalities due to limitations in capacity or resources (32).
Apart from the direct risks of COVID-19 on health and the healthcare system, the uncertainty of the context and the high death rate due to the virus also exacerbate the risk of mental health problems and worsen existing psychiatric symptoms, further impairing the daily functioning and cognition of patients (33).
While these illnesses do not all represent an immediate threat to life, they will have long-lasting serious effects on individuals and large populations. Emerging mental health issues should thus be addressed promptly. In addition, the multiple logistic changes imposed on us by the pandemic pose a unique challenge in mental health service delivery. For example, the restriction in freedom of movement and face-to-face therapies increases psychological distress (32). The limited knowledge on the virus and the overwhelming news that surround it also increase anxiety and fear in the public (33, 34). In addition, long quarantine durations are generating frustration, boredom, stigma, and stress, as well as financial loss that also affects mental health. This is without mentioning highly vulnerable populations such as healthcare providers (32), university students (35), children (36), and naturally anxious individuals (37) who are more prone to developing mental illnesses such as posttraumatic stress disorder or anxiety and mood disorders during this pandemic crisis. This is also without mentioning the already.
In this context and with the advent of AI, a digital methodology that optimizes and homogenizes research processes in an intersectoral and transdisciplinary approach makes more sense than ever, specifically in the field of mental health. Implementing such approaches could help detect and monitor mental health symptoms and their correlation to COVID-19 parameters (whether individuals are affected by the virus or know people affected by it, how political decisions impact mood and anxiety of general populations, etc.). Early detection and close monitoring would in turn allow adequate in-time treatment in the short term and prediction as well as prevention in the longer term.
Here, we propose an end-to-end methodology that highlights key priorities for optimal translational digital mental health research. Each step of the process is elaborated from brainstorming to product creation, with a focus on data analysis. Based on iterative processes, the methodology aims at being cross-sectorial, at the intersection between academia and the private industry. By formalizing the methodology around a mental health use case, the methodology also aims at being interdisciplinary, encompassing different fields (from computational neuroscience to psychology and well-being) all while stressing on the importance of human factors in the digitalization of health. An important goal of the methodology is thus to allow robust collaborations between experts from different fields and sectors (practicing clinicians, AI researchers in academic institutions, and R&D researchers in private industries) to pinpoint then advance foundational and translational research relevant to digital mental health and to create ultimately digital tools that satisfy various stakeholders (usability, clinical benefit, economic benefit, security, and safety). All in all, our methodology has the short-term ambition to propose guidelines for upcoming digital mental health studies and the ultimate ambition to transform the gap between fundamental and applied research into a federalizing platform.
All research begins with a question. Not all questions are testable though, and the scientific method only includes questions that can be empirically tested (observable/detectable/measurable) (38). Similarly, not all questions lead to the development of solutions. As a matter of fact, only few research projects directly reach practical solutions. However, in the digital health sector, research tends to have (or at least ought to have) a very pragmatic, concrete, and measurable outcome (39). The selection of ideas is therefore one of the most complex steps of the research process in the digital health sector since, in addition to verifying whether their idea can be transformed into a project, researchers must also evaluate whether the project can lead to practical often technical solutions, and when. As the world of technologies moves fast (11), by the time that an idea leads to a solution, the solution might lose some or all its value. It is thus crucial to assess whether the idea is feasible and realistic early in the process.
As a result of the COVID-19 pandemic context for instance, there has been an increase in the usage of telehealth medicine and alternative digital mental health options such as mobile applications and web-based platforms (40). Although the need is real and measurable, research projects must be cost-effective, and depending on the investment needed, they ought to be useful not only within a short-term period (i.e., to treat current psychiatric illnesses) but also in a longer time frame, to treat for instance the expected rise in symptoms of trauma among the general population (40).
In addition, according to Gartner (1), digital health research follows a hype cycle divided into five stages illustrated in Figure 1:
1. the innovation trigger,
2. a peak of inflated expectations,
3. a trough of disillusionment,
4. a slope of enlightenment, and
5. a plateau of productivity.
Figure 1. The five stages of the hype cycle of digital health research: (2) the innovation trigger, (3) a peak of inflated expectations, (4) a trough of disillusionment, (5) a slope of enlightenment, and (6) a plateau of productivity. Adapted from Gartner (41).
An ideal digital health research project predicts the failures that will occur at the third stage and the plateau that will be reached at the fifth stage in order to prepare for them and increase the chances of overcoming them. For instance, treating traumas through mobile applications might 1 day be the old-fashioned way of approaching such disorders. This is one major challenge as there are no generic methods describing a digital health project from research inception to solution development (1).
Evaluating an initial idea further faces more classical challenges such as finding the good mix between focused enough to be interesting yet broad enough to build on existing knowledge (42). A digital health research project should balance more than any other research project between ambitious but not overambitious as the competitive landscape is both wide and niche. Going back to our COVID-19 example, this would mean developing digital health technologies that are precise enough to treat specifically traumas in a pandemic context, but broad enough to be adaptable when traumas would not be the main mental health issue anymore, in a near enough future.
What is it that we want to put in light? Defining the goals and objectives of a digital health research project is essential as it keeps the project focused (11). The process of goal definition usually begins by writing down the broad and general goals of the study. As the process continues, the goals become more clearly defined and the research issues are narrowed to an extent that depends on the adopted approach. For instance, the general goal of a mental health mobile application could be to improve mental health conditions; this is the case of the 1,009 psychosocial wellness mobile apps that were found in a study looking to differentiate scientifically evidenced apps from the success stories due to a media buzz (43). A more palpable goal could be to promote behavioral change; this is the case of notOK, a suicide prevention application that alerts the support system of a patient when negative thoughts are too close to an acting out. This is also the case of Twenty-Four Hours A Day, an addiction app that offers 366 meditations (one per day) to help abstinent patients focus on sobriety. The goal ought, however, to be further narrowed as the design of the application might consider eliciting not only more engagement on the mobile app overall, but perhaps effective engagement defined by specific patterns (44). Twenty-Four Hours A Day could, for example, be used effectively during a year at the end of which users could lose interest, potentially resulting in a relapse. Narrowing the number of users could allow a deeper engagement of actual users; more is not always better in digital health (45).
Given the multistakeholder nature of healthcare and their varying incentives, the best approach to impactful and useful digital health research may differ depending on the project. The main challenge is to find the right balance that maximizes clinical impact all while utilizing efficient resources and at a rate that corresponds to the needs of the market in a globally very dynamic and rapidly changing digital health landscape. This brings us back to creating a requirement set broad enough to encapsulate concepts important to all products, but not too inclusive that the requirements are not relevant anymore (39).
This also allows us to emphasize on the importance of staying flexible and ready to change strategies depending on the number and the rate at which new technical solutions are deployed with time. Headspace for instance had started as an events company organizing mindfulness trainings and workshops; as they stayed open to opportunities, they later developed their mobile application that is currently being used in several clinical trials (46). In the case of this app, adopting a ready-to-change pivot strategy allowed them to seize an opportunity and scale drastically.
One way to stay flexible is to inject some agility in the research processes. Agility uses iterations (also called sprints) to create short loops of work (1–4 weeks) that start with planning and end with retrospection, favoring more frequent deliverables (such as quick posters or abstract publications, proof of concepts or minimum viable products) (47). If the concept of agility springs from the software development field, it has been more broadly applied in different fields and sectors recently, such as in mobile health technology (47). A clear step-by-step example applied to our use case, i.e., digital mental health, is the text-based coaching practical guidance provided by Lattie et al. (48).
All in all, it is crucial to define then narrow the goal progressively while balancing between clinical requirements and market realities by staying agile and considering the potential conceptions and misconceptions of all stakeholders.
Everyone is susceptible to the misconceptions of research, development, and innovation, including researchers and any other individual in academia or in the private industry (see Identifying cognitive biases in digital health to improve health outcomes). The what of research is challenging in itself and even more so in the digital health context that often includes translational application at the end of the process as well as the need to confront the views and requirements of academia and the industry. It is therefore critical to identify these misconceptions early in the research project to reduce them and promote alternative conceptions where necessary. Most common misconceptions include the following (49):
- Good research procedures necessarily yield positive results.
- Research becomes true when published.
- Properly conducted research never yields contradictory findings.
- It is acceptable to modify research data to make them look perfect.
- There is only one way of interpreting results.
Discussing conceptions and misconceptions of research can reduce cognitive biases (see Identifying cognitive biases in digital health to improve health outcomes) and improve research outcomes all while favoring a holistic approach to research (42):
- How would you describe research to your grandmother?
- What is the difference between academic (moving knowledge further, contributing to the development of the discipline, explaining, arguing, conceptualizing, theorizing, developing insights, being rigorous and methodical, situated within a theoretical or conceptual tradition) and industrial research (fact-finding, collecting and reporting, producing and developing)?
- How to combine different views and different approaches and methods of research into an R&D model that serves research and innovation in the digital health sector?
This “awakening” step is of particular importance in DHI as interdisciplinary and intersectoral collaborations increase by the day (see Identifying the team and potential partners or collaborators).
Reviewing the literature is an inevitable step of a research project (for further details, see Appendix 1). Nonetheless, it cannot factor in major advances in health technology if relying only on peer-reviewed sources (50). Given both the size (valued at 75 bn in 2017 by Technavio's Global Digital Health Market research report) and the evolution rate (projected to reach 223 bn in 2023 as predicted by Global Market Insights) of the digital health market, it seems crucial to complement the literature review with adequate market research also called gray literature.
Given the complexity that is characteristic of the digital health landscape of technologies, market research cannot be straightforward. For it to be as thorough as possible, it should include project reports, market research foresees, policy documents, and industry white papers (39). For instance, in the oversaturated market of mobile apps advocating for wellness and self-care, one approach would be to conduct a systematic review of publicly available apps on the stores using key words related to the topic (43).
In the context of digital mental health research, the market research would allow researchers not only to compare the potential outcome of their research to the state of current technology (51) but also to predict or at least speculate whether their solution will still have the same value by the time it reaches the market. Such market research could also provide researchers with an overview of the general landscape, i.e., of the unexplored new market areas (blue ocean strategy; 47).
Common benefits to collaboration including brainstorming, division of labor, and speed of execution are challenged by the difficulty of developing a shared vision and defining roles and responsibilities for the different collaborators (52). These challenges are exacerbated in the context of digital health as the field is essentially both interdisciplinary and intersectoral (53), bringing together academic researchers, private industries and their R&D departments, clinicians, patients, and other healthcare consumer groups (54). Indeed, while collaborations in the field are facilitated by complementary roles, authentic communication between partners, and clearly outlined goals or expectations prior to the collaboration, they can also be jeopardized by misaligned expectations, differences in productivity timelines, and balancing business outcomes vs. the generation of scientific evidence (53). It is thus crucial not only to identify the right fit for a collaboration but also to outline and communicate openly about goals, expectations, and timelines. This was done by X2AI, a US-based digital health company that developed in collaboration with experts (including clinical, ethical, technical, and research collaborators) an ethical code for startups, labs, and other entities delivering emotional AI services for mental health support (55). Once the project is developed, it moves to the commitment phase or project planning.
The rapid advancement of digital health technologies has produced a research and development approach characterized by rapid iteration, often at the expense of medical design, large cohort testing, and clinical trials (39, 43). According to the WHO's guidance for digital health research (56), digital research measures are too often evaluated in studies with varying samples and lack of or poor validation. Additional challenges with digital health research include a potentially unrepresentative sample (57). Consequently, insufficient sample sizes may make it difficult for these data to be interpreted through ML techniques (58) (see Data postprocessing: visualization and evaluation). Underestimation occurs when a learning algorithm is trained on insufficient data and fails to provide estimates for interesting or important cases, instead approximating mean trends to avoid overfitting (59).
It is, however, necessary to pursue the adequate amount of evaluation and verification to avoid dubious quality and ensure usefulness and adequacy of the solution (60). To do so, it is crucial to improve sampling strategies by including underrepresented groups in the recruitment, collecting and analyzing reasons for declining, analyzing the profiles of recurrent participants (61), and creating ultimately novel smart sampling approaches (62).
Research projects in the digital health sector can take the form of cohort studies, randomized trials, surveys, or secondary data analysis such as decision analyses, cost-effectiveness analyses, or meta-analyses. To sum things up, there are three basic methods of research:
1. Surveys by e-mail, via a web platform or via a mobile application. They usually involve a lengthy questionnaire that is either more in-depth (usually by email) or more cost-effective (web- and app-based surveys) (63).
2. Observation monitors subjects without directly interacting with them. This can be done either in the environment of the subject with different monitoring devices (ecological environment) or in a lab setting using one-way mirrors, sensors, and cameras to study biophysiological markers or behavior (controlled environment) (64). Faster digital tools now allow monitoring patients via their health insurance or via different health apps.
3. Experiments allow researchers to modify variables and explain changes observed in a dependent variable by a change observed in the independent variable. Experiments were mostly restricted to laboratory contexts as it is very difficult to control all the variables in an environment. This contextual limitation is, however, blurred with digital health research and the use of technologies in less controlled environments. In addition, and even within a laboratory, attention should be given to hardware and software variability between devices as it can affect stimulus presentation and perception of a stimulus as well as human–machine interaction (64).
Although there is no one best method for all digital health research projects, a well-defined problem usually hints at the most appropriate method of research. There also often are cost/quality trade-offs that urge the researcher to consider budget and time as part of the general design process.
A user-centered design (UCD) is an iterative design process in which designers focus on users and their needs in each phase of the design process. Design teams may include professionals from multiple disciplines (ethnographers, psychologists, engineers), as well as domain experts, stakeholders, and the users themselves. They also involve users throughout the design process via a variety of research and design techniques (surveys, interviews, brainstorming), to create highly usable and accessible products. Each iteration of the UCD approach involves four distinct phases illustrated in Figure 2 (65) [see norm ISO (9241-210, 2010)]:
1. understanding the context of use,
2. identifying and specifying user requirements,
3. designing solutions, and
4. evaluating the outcomes of the design to assess its performance.
Figure 2. The four phases of the user-centered design: (2) understanding the context of use, (3) identifying and specifying user requirements, (4) designing solutions, and (5) evaluating the outcomes of the design to assess its performance. Adapted from Nielsen (65).
Iterations are repeated until the evaluation phase is satisfactory.
The term “user-centered method” was first used in 1986 by Don Norman (66), who argued the “importance of design in our everyday lives, and the consequences of errors caused by bad designs.” Ambler later highlighted the efficiency of agility (47) by demonstrating that UCD reduces computing costs (67). UCD approaches further provide advantages in a digital change context (68), all of which can be distinguished in four ways (69):
- User involvement increases the likelihood for a product to meet expectations which in turn increases sales and reduces customer services costs.
- Tailored products reduce the risk of human error (29, 70).
- Designer–user reconciliation increases empathy and creates ethical designs that respect privacy.
- By focusing on specific product users, designers recognize the diversity of cultures and human values through UCD—a step in the right direction to create sustainable businesses.
Digital health asserts a translational vision of changed practices and care systems [new modes of assessment through virtual reality (71) and the presence of sensors in smartphones for instance (27, 28)] to drive better health outcomes. However, the human–technology interaction was only put in light recently (72): it took a decade to first develop and then apply a theoretical understanding of the scope for a substantial, human-centered “design-reality” gap in healthcare (73).
In terms of functionalities, the focus is on usability of parameters such as appearance, appeal, and ease of navigation, as well as various interventions that include quizzes, games, self-monitoring tools, progress reports, downloadable documents, and other similar features [e.g., for social anxiety disorder (74)]. On the other hand, numerous barriers potentially prevent people from participating in evaluations of DHIs such as being too busy, feeling incapable of using the technology, or disliking its impersonal nature (75, 76).
Increasing interest in human factors has underpinned key developments in digital health, spanning intervention development, implementation, and the quest for patient-centered care (77). The emergence of ML chatbots and other patient-centered designs within Internet-based cognitive behavioral therapy has proven to facilitate access and improve tailored treatments (78). This is mainly due to the digital removal of several barriers such as reduced perceptions of stigma (very present in face-to-face services) and a rapid response to the need of “in the moment” support for mental distress. All these reasons increase the demand for digital mental healthcare in formal healthcare settings (79).
To truly benefit from DHI, privacy and data governance, clinical safety (handling crisis in mental health apps for instance), and evidence for effectiveness must be at the core of the design (80, 81). This is unfortunately not always the case as shown by a smartphone app review revealing that, out of all health apps, only 11 were identified as “prescriptible” [meaning that they included randomized controlled trials (RCTs) reporting of effectiveness without clinical intervention] (82).
The UCD of digital health systems enables greater engagement and long-term use of digital tools (83). However, little attention is given to human factors such as ethnography of users or usability testing (77), or to the real-world difficulties that individuals face (84, 85) such as technology cost and privacy or security issues (86). These barriers reduce health outcomes with poor user engagement despite mobile health interventions (87–89). The decision-making power toward consumers is in turn insufficient (80), raising questions of access [namely in low- and middle-income countries (90)], equity, health literacy, privacy, and care continuity (14).
In their review of all barriers and facilitators for DHI engagement and recruitment, O'Connor et al. (91) distinguished four themes:
1. personal agency and motivation,
2. personal life and values,
3. the engagement and recruitment approach, and
4. DHI quality.
Education (91) and age (92, 93) were given particular attention as poor computer skills in both low-education individuals and old adults added to the enrollment struggle. In the same vein, literacy skills (94, 95) and the ability to pay for the technology (96) have impact on people's ability to interact with and use DHIs. All these factors ought to be further explored.
In summary, adopting a UCD of DHI would optimize long-term tool acceptance (6). Interdisciplinary collaborations could provide knowledge about “the context of use” (97), but it is crucial to further identify the technological and economic feasibility of the design (98, 99). In addition to the central role of human factors in DHI, attention should also be given to cognitive biases that come with ML strategy implementation and data interpretation.
Studies from the past decades point at the vulnerability of the human mind to cognitive biases, logical fallacies, false assumptions, and other reasoning failures (100). In the health system context, cognitive biases can be defined as faulty beliefs that affect decision-making and can result in the use of heuristics in the diagnostic process (101, 102). Kahneman and Tversky introduced a dual-system theoretical framework to explain judgments, decision under uncertainty, and cognitive biases (103, 104). In this model, illustrated in Figure 3, system 1 refers to an automatic, intuitive, unconscious, fast, and effortless decision process. Conversely, system 2 makes deliberate, non-programmed, conscious, slow, and effortful decisions. Most cognitive biases are likely due to the overuse of system 1 vs. system 2 (100, 105–107).
Figure 3. Properties of the dual-system theoretical framework of Kahneman (103) to explain judgments, decision under uncertainty, and cognitive biases: (2) system 1 refers to an automatic, intuitive, unconscious, fast, and effortless decision process; (3) system 2 makes deliberate, non-programmed, conscious, slow, and effortful decisions. Schema taken from Kahneman (103).
“Diagnostic reasoning is the complex cognitive process used by clinicians to ascertain a correct diagnosis and therefore prescribe appropriate treatment for patients” (108): the ultimate consequences of diagnostic errors include unnecessary hospitalizations, medication underuse and overuse, and wasted resources (109, 110).
Diagnostic reasoning and risk of errors can be explained by adapting the dual-system model to the health system context. For instance (99—see Appendix 2), system 2 overrides system 1 when physicians take a time-out to reflect on their thinking. System 1 also often irrationally overrides system 2 when physicians ignore evidence-based clinical decision rules that outperform them. Depending on what system overrides the other, the calibration (the degree to which the perceived and actual diagnostic accuracy corresponds) will differ.
The main cognitive biases affecting medical performance and diagnosis are the following (111, 112):
- Premature closure (113–117): an automatic process that occurs when the provider closes the diagnostic reasoning process by clinging to an early distractor/diagnosis without fully considering all the salient cues (106).
- Search satisficing (112, 118): a subtype of premature closure in which searches for further evidence are terminated after a diagnosis is reached. This is the case for medical students that do not initiate a search for a secondary diagnosis (118).
- Availability (106, 113, 118): falsely enhancing the probability of a diagnosis following the recent exposure of the physician to that diagnosis (106).
- Anchoring (112, 113, 115): a subtype of premature closure in which a provider stakes their claim on a diagnosis, minimizing information that do not support the diagnosis with which they have attached their proverbial anchor (115).
- Base rate neglect (119): predicting the diagnosis occurrence probability when two independent probabilities are erroneously combined, ignoring the base rate and leading to under- or overestimating the diagnosis possibility (120).
- Diagnostic momentum (112, 119, 121): a subtype of anchoring and premature closure in which the suggestion power of colleagues is taken at face value. For example, the diagnosis of anxiety disorder of the patient established from her family doctor through to the emergency department (ED), and although she might well have had hyperventilation due to anxiety, other possibilities were not ruled out earlier on in her care (112).
- Overconfidence and lower tolerance to risk/ambiguity (122, 123). Because of these two biases, misdiagnosis, mismanagement, and mistreatment are frequently associated with poorer outcomes, leading to patient dissatisfaction and medical complaints and eventually to a dropout of the digital health system (79, 124–126).
In the specific context of digital mental health, it is important to identify potential cognitive biases in patients as well in order to avoid misinterpretation and treatment misusage. In addition to the eight cognitive biases mentioned above, other cognitive factors such as coping strategies (127, 128) and the role of emotional stimuli (e.g., in depression, there is a lack of such a bias) (129) require particular attention in order to design tailored digital treatments and to drive ultimately an effective digital health strategy.
Early recognition of the cognitive biases of physicians is crucial to optimize medical decisions, prevent medical errors, provide realistic patient expectations, and decrease healthcare costs (107, 126, 130). Some debiasing strategies include the following:
1. Advocating for a view in which clinicians can change thinking patterns through awareness of bias and feedback (100). It consists of theories of reasoning and medical decision-making, bias inoculation, simulation training, computerized cognitive tutoring, metacognition, slow-down strategies, group decision strategy, and clinical decision support systems to force diagnostic reasoning out of bias-prone thought analytic processes.
2. Digital cognitive behavioral therapy [see, for review, 125] through which positive cognitive bias modification could be used as a potential treatment for depression (131), for anxiety disorders (25), for persecutory delusions (132), for improvement of social interaction in autism spectrum disorders and dementia (26), and for people with suicidal thoughts (133).
There is, however, no consensus regarding the efficacy of such debiasing approaches (118). In addition, other biases such as aggregation bias (the assumption that aggregated data from clinical guidelines do not apply to their patients) or hindsight bias (the tendency to view events as more predictable than they really are) also compromise a realistic clinical appraisal and could lead to medical errors (134, 135). This brings us to the urgent need for transparent and explicit data and strategy.
Cognitive biases exposed previously mainly concern physicians and their ability to analyze a digital diagnosis. Data scientists are also prone to specific cognitive biases given the strong interpretative component of data science and ML (136). Biases affecting data scientists in the digital mental health setting include but are not limited to the following (136):
- survivorship: a selection bias in which data scientists implicitly filter data based on some arbitrary criteria and then try to make sense out of it without realizing or acknowledging that they are working with incomplete data;
- retrospective cost: the tendency to make decisions based on how much of an investment they have already made, which leads to even more investment but no returns whatsoever;
- illusion of causality: the belief that there is a causal connection between two events that are unrelated;
- availability: the natural tendency to base decisions on information that is already available without looking at potentially useful alternatives that might be useful; and
- confirmation: the interpretation of new information in a way that makes it compatible with prior beliefs.
Despite these data science biases, a promise of ML in healthcare is precisely to avoid biases. The biases of scientists and clinicians would be circumvented by an algorithm that would objectively synthesize and interpret the data in the medical record and/or offer clinical decision support to guide diagnosis and treatment (58). In the digital health context, integration of ML to clinical decision support tools such as computerized alerts or diagnostic support could offer targeted and timely information that would in turn improve clinical decisions (58, 137–140). With the rise of ML in the DHI, data sources and data collection methods should be further examined to better understand their potential impact (141–143). Biases that could be introduced through reliance on data derived from the electronic health record include but are not limited to the following:
- Missing data: If communicated sources such as patient-reported data are incomplete (missing or inaccessible), algorithms (that only use available data) may correctly misinterpret available data (144). Algorithms could thus be a bad choice for people with missing data (145) [people with low socioeconomic status (146) or those with psychosocial issues (147) for instance].
- Misclassification and measurement errors: Misclassification of diseases and measurement errors are common sources of bias in observational studies and analyses based on electronic health record data. Care quality may be affected by implicit biases related to patient factors, such as sex and race, or practitioner factors [e.g., patient with low socioeconomic status (148) or women (149)]. If patients receive differential care or are differentially incorrectly diagnosed based on sociodemographic factors, algorithms may reflect practitioner biases and misclassify patients based on those factors (58).
We mostly identified and described biases that interfere once the data are already collected. It is important to note that biases can also interfere earlier in the process, at every step of it, from brainstorming to literature reviewing (143). The main recommendation is to stay alert to all different biases, whether they are mentioned in this paper or not.
As seen above, decision-making in the medical field often has far-reaching consequences. To better measure these consequences, it is essential to build certainties: certainties on the data used, their source, their format, and their update; certainties on the information put forward and their implications; and certainties on the tools exploiting these data as well as on the reliability of the algorithms and visual representations made available. These questions concern data in a broad way.
It thus seems important to start this technical part of the paper by defining the notions of data, information, and knowledge, as all three are involved in decision-making processes.
We will then focus our approach on the data and the different steps to structure, exploit, and enhance them.
Grazzini and Pantisano (150) defined each concept as follows:
Data can be considered as raw material given as input to an algorithm. Since it cannot be reproduced when lost, it must be carefully preserved and harvested. It can be of different forms: a continuous signal as in the recording of an electroencephalogram (EEG), an image representing a magnetic resonance imaging (MRI), a textual data, or a sequence of numerical values representing a series of physiological measurements or decisions taken via an application. Data can be complete, partial, or noisy. For example, if only a portion of an EEG recording is available, then the data are partial. Conversely, an EEG recording that has been completed but that has some parts unusable is said to be noisy because the noise alters the completeness of the recording. Two types of data can be distinguished: unstructured data, i.e., data directly after their collection or generation, and structured data, i.e., data that have been analyzed, worked on, and put in relation to each other to put them in a format suitable for the analysis considered afterwards. In the second case, it is considered information. Importantly, data by themselves are worthless.
Information is dependent on the original data and the context. If it is lost, it can be reproduced by analyzing the data. Depending on the data processed at time t, information must be accurate, relevant, complete, and available. Information is intelligible by a human operator and can be used in a decision-making process. It is therefore significant and valuable since it provides an answer to a question. It can take various forms such as a text message, a table of numerical values, graphs of all kinds, or even in the shape of a sound signal.
When semantics are added to a set of information, it becomes knowledge. Information, depending on the context, will not have the same impact. It is the context and the semantics brought by it and the human operator involved that will determine the value of that knowledge.
To illustrate these definitions in a mental health setting, in the case of a patient undergoing a follow-up with a psychiatrist: the psychiatrist can make his patient pass numerous tests in order to collect data: MRI, EEG, and textual answers to questionnaires. These data, once processed, formatted, and analyzed together, will represent a set of information on the condition of the patient. It is the combination of the knowledge and experience of the doctor, combined with his knowledge of the patient, his family context, and the current socioeconomic context, that will enable him to have a global knowledge of his patient and to provide him with the best possible support.
The passage from data to information thus requires a majority of digital processing to highlight correlations according to a given context. However, the passage to the knowledge stage requires considering individuals involved (see Project design). Figure 4 illustrates data transformation into information through digital processing and into knowledge through human evaluation.
Figure 4. Data transformation into information (from 1 to 5) then knowledge through KDD steps: (a) raw data can be collected to become target data, which can also be acquired or generated by an external process: it is the data generation, acquisition, or collection step. (b) Target data are submitted to a data preprocessing step according to the data mining techniques targeted: target data can thus be cleaned, filtered, completed, and anonymized if necessary. This second step allows to obtain preprocessed data. (c) Preprocessed data are submitted to data mining techniques to detect, identify, and extract patterns and relevant features. (d) Discovered patterns and features can thus be rearranged (e.g., with visual tools) for or within the phase of interpretation during the postprocessing step. The result is the generation of information. (e) Information evaluated by a human becomes knowledge: this step is an external one (i.e., not technical) and takes into account knowledge of the situation (context, issues, stakeholders, etc.).
There is thus an increasing complexity in this process of transforming data into information and then into knowledge which make it difficult to identify and extract. We will present a process dedicated to these tasks in the following section.
The process of Knowledge Discovery of Data (KDD) is defined as the process of discovering useful knowledge from data (151). As a three-step process, the KDD includes (2) a preprocessing step which consists of data preparation and selection, (3) a data mining step involving the application of one or many algorithms in order to extract information (i.e., patterns), and (4) a postprocessing step to analyze extracted information manually by a human operator and lead to knowledge discovery.
As an iterative and interactive process, KDD involves many steps and decisions of the users. Iterations can continue as long as extracted information does not satisfy the decision-maker (see Identifying cognitive biases in digital health to improve health outcomes).
Concretely, as illustrated in Figure 5, the KDD stages encompass the following: (2) understanding the scope of the application field; (3) creation of the target dataset; (4) data cleaning and preprocessing; (5) data reduction and projection: reducing the number of variables to be analyzed by reducing the dimensionality of the data, extracting invariant representations, or searching for relevant characteristics; (6) matching the goals of the KDD process with the right method(s) in data mining; (7) exploratory analysis and selection model and hypothesis: selection of the data mining algorithm and method that will be used for the pattern search; (8) data mining: searching for interesting patterns in a particular form of representation, which includes rule and tree classification, regression, and clustering; (9) data postprocessing and visualization: interpretation of the patterns found with possible return to any step from 1 to 7 for a new cycle; and (10) action on discovered knowledge.
Figure 5. The nine Knowledge Discovery of Data stages adapted from Fayyad (151): (2) understanding the scope of the application field, (3) creation of the target dataset, (4) data cleaning and preprocessing, (5) data reduction and projection: dimensionality reduction and extraction of invariant representations and relevant characteristics, (6) matching the KDD goals with the right data mining method(s), (7) exploratory analysis and selection model and hypothesis: selection of the data mining algorithm and method that will be used for the pattern search, (8) data mining: searching for interesting patterns in a particular form of representation (e.g., tree classification, regression, and clustering), (9) data postprocessing and visualization: interpretation of the patterns found with possible return to any step from 1 to 7 for a new cycle, and (10) action on discovered knowledge.
Here, we mainly focus our approach on the technical aspect, i.e., data and their transformation into information. We aim to present a complete and global approach by covering the KDD stages in the life cycle of a digital health product from the definition of the scientific question to data collection and analysis (for further details, see Appendix 3). We will reveal our approach in the following section.
We are aligned with the three-step approach of Fayyad (151) for information extraction:
- data preprocessing (data cleaning, data editing, data curation, and data wrangling),
- data mining with a special focus on biostatistics and AI and ML algorithms, and
- postprocessing focusing on data visualization.
Figure 4 proposes a representation of the global approach for information extraction for a specific question or product design.
Upstream of these activities, we would like to highlight two areas that are essential to good data management and that allow an optimization of the research of a team: data strategy, which aims at standardizing data management, and data governance, or the implementation of solutions to respond to the strategic issues defined beforehand.
Within a digital research project, technical and operational tasks are either managed by the same person (it is generally the case in a fundamental research team) or by distinct groups (it is the case for R&D groups in which technical teams focus on system architecture, development, quality, and testing, while operational teams handle experimental requirements and process definition). These concepts are classically and poorly applied to data (in fundamental and R&D teams), thus slowing down the improvement of accuracy, access, sharing, and reuse of data (152).
Data strategy applied to research aims at using, sharing, and moving data resources efficiently (adapted from 147) in order to manage projects easily, facilitate scientific collaboration, and accelerate decision-making regarding new project ideas.
Data strategy contains five core components that work together to comprehensively support an optimal data management:
- Identification: to set common data definition shared with the team, collaborators, and more broadly with the scientific community. In the mental health context for instance, it is crucial to define all biomarkers (whether they are genetic, molecular, anatomical, or environmental) to encompass the complexity of psychiatric disorders.
- Storage: to maintain data in information technology (IT) systems that allow easy access, sharing, and data processing.
- Provision: to anticipate and to prepare data in order to directly share or reuse it with adequate documentation explaining rules and definition.
- Processing: to aggregate data from different IT systems and obtain a centralized 360° data vision. In a mental health project, it could be pertinent to aggregate clinical, biological, and imaging data for instance.
- Governance: see dedicated chapter below (Data governance).
According to the Data Governance Institute, data governance is “a system of decision rights and accountabilities for information-related processes, executed according to agreed-upon models which describe who can take what actions with what information, and when, under what circumstances, using what methods.” Data governance is generally informal in fundamental research labs due to reduced (<15 people) and homogeneous (with the same background) teams in which processes, information, and tools are shared by everyone. This informal approach is less applicable with team expansion, different profile recruitment, scientific collaboration, or any operation that implies cross-functional activities. Initially designed for private industries, formal data governance approaches allow to frame cross-functional activities with a set of objectives adapted from the Data Governance Institute:
- to optimize decision-making: with a deeper knowledge of data assets and related documentation. This is helpful for instance when a choice has to be made between several scientific projects or strategies and the formal data governance approach estimates the ratio between investment and expected scientific value;
- to reduce operational friction: with defined and transparent roles and accountabilities regarding data and data use;
- to protect the needs of teams within a scientific collaboration framework;
- to train teams and collaborators to build common standards for approaching data issues;
- to reduce costs and increase effectiveness through effort coordination;
- to ensure transparency of processes;
- to accelerate and facilitate scientific collaboration;
- to allow scientific audit; and
- to respect compliance with the required documentation.
Data governance should not be applied as a theoretical concept but should rather be considered for its potential added value when it comes to pain points and the definition of use cases (e.g., to ensure data quality of a mental health digital project focused on schizophrenia). Good practices could anticipate value creation and changes triggered by the data governance framework (harmonious collaborations and their impact on data-related decisions for instance).
Regarding enterprise systems, research teams and/or scientific collaboration will require only a restricted number of rules and thus do not need a large organization assigned to data governance but more likely some clear and identified accountabilities and documentation for all scientific members (for further details, see Appendix 4).
In summary, data strategy and governance give a starting framework to structure the data management policy and strategy of a research team. Depending on the size of the team, the issues at stake, and the collaborations, these steps can have a real added value. As research teams do not rely on nor need large organizations for their data governance, it is also important to include other operational steps in a digital health data-centered project.
The preprocessing step consists in preparing the dataset to be mined (see Figure 4). This implies the following: (2) data cleaning, which consists in removing noise, corrupted data, and inaccurate records (3, 153, 154) data editing to control data quality by reviewing and adjusting it (155) and to anonymize data when needed with respect to data privacy standards (4, 156, 157) data curation to manage data maintainability over time for reuse and preservation (158); and 4) data wrangling or the process of mapping data from one type to another to fit the selected mining technique (e.g., from natural language to numerical vectors) (159). It is an important step in the KDD process (160) since the quality of the analysis of a data mining algorithm relies on the data available for the analysis. This step is inevitable as each dataset must be preprocessed before being mined. Alternatives (161) to preprocessing data exist but depend on the objective and the nature of available data, which makes it overwhelming to unexperienced users (162). It is thus essential to fix an explicit objective (i.e., a question to answer or a hypothesis to study) before preprocessing to choose the appropriate techniques.
Unlike ML, biostatistics are not used to establish predictions; hence, they do not require a large amount of data. Biostatistics study inferences between different populations by establishing a quantitative measure of confidence on a given sample of the population (163).
The frontiers between statistics and ML can be blurry as data analyses are often common to both [it is the case for the bootstrap method used for statistical inference and for the random forest (RF) algorithm]. It is thus important to differentiate statistics (that require us to choose a model incorporating our knowledge of the system) from ML (that requires us to choose a predictive algorithm by relying on its empirical capabilities) (163).
Data mining is characterized by the willingness to find any possible means in order to be able to answer the research question. It can thus be defined as the process of analyzing large amounts of data to uncover patterns, associations, anomalies, commonalities, and statically significant structures in data (164). The two main goals of data mining are thus prediction of future behavior according to discovered patterns and description or the presentation in human-understandable shapes of the patterns found. To do so, data mining focuses on the analysis and extraction of features (extractable measurements or attributes) and patterns (arrangements or ordering with an underlying structure). Subfields of data mining include pattern recognition domain (or the characterization of patterns) (165) and pattern detection and matching (mining data to characterize patterns).
Data mining also includes subsets of popular algorithms:
- Classification consists in learning a function that classifies data into one or more predefined classes. For example, to predict generalized anxiety disorder among women, it is possible to either use RF to implement featured selection of the data mining classifier on the mental health data (166), or to use decision tree-based classification (167) or Shapley value algorithm (168).
- Regression consists in learning a function that matches data with a real predictor variable. The purpose of these algorithms is to analyze the relationship of variables with respect to the others, one by one, and to make predictions according to these relationships. It can be a statistical method or a ML algorithm. For example, Yengil et al. (169) used regression algorithms to study depression and anxiety in patients with beta thalassemia major and to further evaluate the impact of the disorder on quality of life.
- Another type of algorithm is clustering that consists in detecting a finite set of categories to describe data. Categories can be mutually exclusive and exhaustive or consist of a richer representation such as hierarchical or overlapping categories. The k-mean algorithm for instance can describe a population of patients as a finite set of clusters, each one grouping individuals sharing same features (e.g., children vs. adults).
- Summarization methods are used to find a compact description for a subset of data.
- Dependency modeling consists in finding a model that describes significant dependencies between variables. This can be done at the structural level (specifying dependent variables) or the quantitative level (specifying the strength of a dependency using numerical scales).
All these methods aim at extracting features or patterns following the search method as previously discussed in Defining the goal and the approach.
To efficiently communicate scientific information, data visualization (or graphic representation) should be specifically designed for the targeted audience. This can involve exploratory and/or explanatory objectives (170):
- Pure exploratory: addressed to teammates and collaborators to highlight main results in order to make data memorable and to identify the next strategic steps of the project.
- Explanatory/exploratory mixed: addressed to the scientific community, to share information and provide reliable (accessible and intelligible) data that can be analyzed and challenged by others. It can also support the scientific story telling in a grant application.
- Pure explanatory: addressed to patients, to quickly and efficiently explain scientific information with an appropriate and tailored content.
As seen in Figures 4, 5, evaluating and interpreting mined patterns or extracted data through visualization can possibly induce returning to any previous step from preprocessing to data mining until discovered knowledge answers the fixed goal.
There is a growing number of mobile apps dedicated to mental health. Among them, “Moodfit” shapes up the mood, “Mood mission” teaches coping skills, “Talkspace” provides a virtual space for therapy, “Sanvello” acts as a stress relief, “Headspace” opens a virtual door to meditation, and “Shine” answers the specific mental health needs of BIPOC communities. However, there is no single guide for the development of evidence-based MHapps (171). An analysis of all apps dedicated to depression on the major marketplaces (Apple App and Google Play stores) shortlisted 293 apps that self-advertised as research-based (172). Among these apps, only 3.41% had published research that supports their claims of effectiveness, among which 20.48% were affiliated with an academic institution or medical facility. This analysis strongly indicates the need for mental health applications to be more rigorous (172), i.e., by following a strict method.
We have thus applied our end-to-end methodology to build a mobile application called i-decide (www.i-decide.fr) that facilitates decision processes under uncertainty. The application aims at complementing existing neuropsychological testing that take places punctually in a controlled setting by collecting longitudinal data on a daily basis. The data collected concern decision processes and all cognitive and emotional functions that impact decision-making (Boulos et al., in revision). All data are used to feed an algorithm that learns optimal choices (that reduce long hesitations and associated anxiety as well as the percentage of regret postdecision) under uncertain conditions. We tested the application on a population of 200 adult users with no diagnosed mental illness. Results revealed time slots during which decision-making was optimal as well as clusters of decision profiles according to stress, motivation, daily goals, support system, and the ratio of minor vs. major decisions (Boulos et al., in revision). More information can be found on the mobile application's website www.i-decide.fr.
AI algorithms together with advances in data storage have recently made it possible to better characterize, predict, prevent, and eventually cure a range of psychiatric illnesses. Amid the rapidly growing number of biological devices and the exponential accumulation of data in the mental health sector, the upcoming years are facing a need to homogenize research and development processes in academia as well as in the private sector and to centralize data into federalizing platforms. In this work, we describe an end-to-end methodology that optimizes and homogenizes digital biophysiological and behavioral monitoring with the ultimate ambition to bridge the gap between fundamental and applied research.
The first step described project conception and planning stages. We proposed approaches to evaluate the feasibility of a digital mental health project, to define its goal, and to design the research approach accordingly. We clarified digital mental health research conceptions and misconceptions and described the difficulties of combining academic literature and market research. We further underlined the importance of collaborations in the interdisciplinary and intersectoral field to better understand what digital mental health is. We finally focused on the concrete planning of such methodology, that is, how to inject agility every step of the way to create ultimately platforms that reconcile different stakeholders to provide the best assistance possible to patients with mental health issues.
The second step zoomed in on the specificities of project design in mental health. We explained the importance of digital health interventions, the necessity to have clear goals, and the importance of human factors in defining them (introducing the user-centered design). We finally described cognitive biases and their impact on both physicians and data scientists in digital mental health.
The third, last, and more technical step described the stages from data collection to data analysis and visualization. We differentiated the notions of data (raw element), information (transformed data), and knowledge (transformed data with semantic contextual value) to then focus on the key steps of data in a digital mental health research. We provided recommendations for data management, strategy, and governance depending on the size and type of research structure and further elaborated a KDD-based operational approach that can be especially useful for small research teams that wish to work from collection to processing.
Exploring the literature around digital mental health interventions leads us to question existing practices, that is, both their strengths and their issues. There are so many questions the scientific community and other stakeholders should consider when developing digital mental health solutions, and these include ethics and biases.
For a trial to be ethical, the assumption of equipoise (i.e., equilibrium) should be included in the design. While general designing and conducting RCT principles (173) are applicable to DHIs, specific DHI features deserve consideration when a trial is expected to provide evidence for rational decision-making: (2) the trial context, (3) the trade-off between external validity (the extent to which the results apply to a definable group of patients in a particular setting) and internal validity (how the design and conduct of the trial minimizes potential for bias) (174, 175) (e.g., of poor trade-off: recruiting highly motivated participants because of missing follow-up data) (174), (4) the specification of the intervention and delivery platform, (5) the choice of the comparator, and (6) establishing methods for separate data collection from the DHI itself.
Detailed specification of DHI is important, because it is required for the replication of trial results, the comparison between DHIs, and synthesizing data across trials in systematic reviews and meta-analyses (176). The relevant data to collect would then focus on usage, adherence, demographic access parameters, and user preferences (6, 177), even if participants are biased because they have access to a myriad of other DHIs. Indeed, someone who has sought help for a problem, entered a trial, and been randomized to the comparator arm, only to find the intervention unhelpful, may well search online until they find a better resource (178).
Finally, a well-designed RCT, especially for its ethical part, highlights the need to create interdisciplinarity. Researchers in digital mental health could learn from the multicycled iterative approach adopted in the industry for optimized development. Researchers from an engineering or computer science background may be surprised by the reliance on RCTs, whereas those from a biomedical or behavioral sciences background may consider that there is too much emphasis on methods other than RCTs. By enhancing critical thinking, interdisciplinarity in a team also tends to reduce cognitive biases. Although we have dedicated an entire part of this paper to cognitive biases (Identifying cognitive biases in digital health to improve health outcomes), there are several important points yet to be discussed. This includes the impact of biases in the decision-making process in digital mental health, the repercussion of the biases of practitioners on the data, and the biases of algorithms. One important message is that there are numerous cognitive biases across multiple domains (such as perception, statistics, logic, causality, social relations…) and that these biases are generally unconscious and effortless, making them hardly detectable and even less so controllable (179). Another important point is how AI and ML acceptability by the community on a social level can in turn affect the cognitive biases of physicians, researchers, and patients on digital mental health. In Appendix 5, we discuss these different issues and propose recommendations to better control the impact of cognitive biases in digital mental health research with the ultimate ambition to improve diagnostic reasoning and health outcomes.
In addition to the ethical considerations, working with data comes with technical challenges, three of which we wish to highlight: (2) interoperability that is defined as the property that facilitates rapid and unrestricted sharing and use of data or resources between disparate systems via networks (180), (3) the trade-off between anonymization (to respect data privacy standards) and anonymization willingness, and (4). ML interpretability and explainability issues in digital mental health and digital health in general.
The multiplicity of tools that needs to be functional all while operating easily with other tools rushed the need for a “plug-and-play” interoperability. This is particularly the case for the medical field and its daily clinical use of various medical devices (MRI, computed tomography, ultrasound…). Beyond the traditional interoperability between different healthcare infrastructures, the will of patients to consult and understand their own data is imposing a new infrastructure-to-individual interoperability (181). In the light of this context, we believe that interoperability should be considered by a research team for their data strategy, especially when the research involves collaborations that are wanted or already in place. Beyond optimizing the collaboration and facilitating patient contribution, this could avoid data manipulation mistakes, as well as security or confidentiality failure.
Beyond confidentiality, one of the most sensitive points is privacy. In the context of digital mental health, and given the fact that it is a relatively young field with little information regarding clinically relevant variables (157), the bigger the data volume, the easier it is to identify relevant variables. The need for large data volumes is, however, challenged by the difficulty to collect these data all while respecting the strict health ethics and laws. It is thus crucial to set up the right privacy strategy. We would like also to highlight the existence of other technical challenges such as anonymization of data and explainable AI that are growing research fields (for further details, see Appendix 6).
In conclusion, our interdisciplinary collaboration to provide an end-to-end methodology for digital mental health research using interpretable techniques and a human-centered design with a special attention to data management and respecting privacy is therefore (2) a moral subject because it is linked to the transparency of the algorithms and, by extension, to the deriving decisions; (3) an ethical subject because it requires taking into account all people involved, their cognitive biases, and their impact on trials, experiments, and algorithms; and (4) a lever of trust for the end user specially in the mental health field where personal privacy is a critical but essential part that has to be respected.
Beyond this work, we find through our review of the literature that the various approaches taken to address different facets of product conception and design from research to market are siloed. Advances are often made separately and little attention is given to interdisciplinary and intersectoral centralizing approaches like ours in an attempt to provide a complete end-to-end methodology. We cannot stress enough on the timely importance of collaborations in digital mental health to reduce the disciplinary and sectoral gap and create platforms that deliver solutions trusted both by scientists and end users.
The original contributions presented in the study are included in the article/Supplementary Material, further inquiries can be directed to the corresponding author/s.
All authors listed have made substantial, direct and intellectual contribution to the work and approved it for publication.
We express our gratitude to onepoint, especially Erwan Le Bronec, for the financial support to the R&D department, which permitted us to carry out this work. The funder was not involved in the study design, collection, analysis, interpretation of data, the writing of this article or the decision to submit it for publication. The only contribution of the funder is to pay the preliminary publishing fees.
AD, AM, and ICK were employed by the company onepoint when the research was conducted.
The remaining author declares that the research was conducted in the absence of any commercial or financial relationships that could be construed as a potential conflict of interest.
All claims expressed in this article are solely those of the authors and do not necessarily represent those of their affiliated organizations, or those of the publisher, the editors and the reviewers. Any product that may be evaluated in this article, or claim that may be made by its manufacturer, is not guaranteed or endorsed by the publisher.
First and foremost, we acknowledge all the contributing authors who participated equally in this work. We would also like to thank Coralie Vennin for her referral to the appropriate literature on the psychological impact of the health crisis due to COVID-19.
The Supplementary Material for this article can be found online at: https://www.frontiersin.org/articles/10.3389/fpsyt.2021.574440/full#supplementary-material
1. Klonoff DC, King F, Kerr D. New opportunities for digital health to thrive. J Diabetes Sci Technol. (2019) 13:159–63. doi: 10.1177/1932296818822215
2. May C, Mort M, Williams T, Mair F, Gask L. Health technology assessment in its local contexts: studies of telehealthcare. Soc Sci Med. (2003) 57:697–710. doi: 10.1016/S0277-9536(02)00419-7
3. Devlin AM, McGee-Lennon M, O'Donnell CA, Bouamrane M-M, Agbakoba R, et al. Delivering digital health and well-being at scale: lessons learned during the implementation of the dallas program in the United Kingdom. J Am Med Informat Assoc. (2016) 23:48–59. doi: 10.1093/jamia/ocv097
4. Pagliari C, Detmer D, Singleton P. Potential of electronic personal health records. BMJ. (2007) 335:330–3. doi: 10.1136/bmj.39279.482963.AD
5. Bailey SC, Belter LT, Pandit AU, Carpenter DM, Carlos E, Wolf MS. The availability, functionality, and quality of mobile applications supporting medication self-management. J Am Med Informat Assoc. (2014) 21:542–6. doi: 10.1136/amiajnl-2013-002232
6. Murray E, Hekler EB, Andersson G, Collins LM, Doherty A, Hollis C, et al. Evaluating digital health interventions: key questions and approaches. Am J Prev Med. (2016) 51:843–51. doi: 10.1016/j.amepre.2016.06.008
7. Palanica A, Docktor MJ, Lieberman M, Fossat Y. The need for artificial intelligence in digital therapeutics. Digital Biomarkers. (2020) 4:21–5. doi: 10.1159/000506861
8. Dunn J, Runge R, Snyder M. Wearables and the medical revolution. Per Med. (2018) 15:429–48. doi: 10.2217/pme-2018-0044
9. Reti SR, Feldman HJ, Ross SE, Safran C. Improving personal health records for patient-centered care. J Am Med Informat Assoc. (2010) 17:192–5. doi: 10.1136/jamia.2009.000927
10. Aboujaoude E, Gega L, Parish MB, Hilty DM. Editorial: digital interventions in mental health: current status and future directions. Front Psychiatry. (2020) 11:111. doi: 10.3389/fpsyt.2020.00111
11. Allen B, Seltzer SE, Langlotz CP, Dreyer KP, Summers RM, Petrick N, et al. A road map for translational research on artificial intelligence in medical imaging: from the 2018 national institutes of Health/RSNA/ACR/The academy workshop. J Am College Radiol. (2019) 16:1179–89. doi: 10.1016/j.jacr.2019.04.014
12. Miner AS, Shah N, Bullock KD, Arnow BA, Bailenson J, Hancock J. Key considerations for incorporating conversational AI in psychotherapy. Front Psychiatry. (2019) 10:746. doi: 10.3389/fpsyt.2019.00746
13. Wentzel J, van der Vaart R, Bohlmeijer ET, van Gemert-Pijnen JEWC. Mixing online and face-to-face therapy: how to benefit from blended care in mental health care. JMIR Mental Health. (2016) 3:e9. doi: 10.2196/mental.4534
14. Huckvale K, Venkatesh S, Christensen H. Toward clinical digital phenotyping: a timely opportunity to consider purpose, quality, and safety. NPJ Digital Med. (2019) 2:88. doi: 10.1038/s41746-019-0166-1
15. Kamdar MR, Wu MJ. PRISM: a data-driven platform for monitoring mental health. Pac Symp Biocomput. (2016) 21:333–44. doi: 10.1142/9789814749411_0031
16. Nelson BW, Allen NB. Accuracy of consumer wearable heart rate measurement during an ecologically valid 24-hour period: intraindividual validation study. JMIR MHealth UHealth. (2019) 7:e10828. doi: 10.2196/10828
17. Khundaqji H, Hing W, Furness J, Climstein M. Smart shirts for monitoring physiological parameters: scoping review. JMIR MHealth UHealth. (2020) 8:e18092. doi: 10.2196/18092
18. Chadborn NH, Blair K, Creswick H, Hughes N, Dowthwaite L, Adenekan O, et al. Citizens' juries: when older adults deliberate on the benefits and risks of smart health and smart homes. Healthcare. (2019) 7:54. doi: 10.3390/healthcare7020054
19. Reading Turchioe M, Grossman LV, Baik D, Lee CS, Maurer MS, Goyal P, et al. Older adults can successfully monitor symptoms using an inclusively designed mobile application. J Am Geriat Soc. (2020) 68:1313–8. doi: 10.1111/jgs.16403
20. Kollins SH, DeLoss DJ, Cañadas E, Lutz J, Findling RL, Keefe RSE, et al. A novel digital intervention for actively reducing severity of paediatric ADHD (STARS-ADHD): a randomised controlled trial. Lancet Digital Health. (2020) 2:e168–78. doi: 10.1016/S2589-7500(20)30017-0
21. Ginsburg GS, Phillips KA. Precision medicine: from science to value. Health Affairs. (2018) 37:694–701. doi: 10.1377/hlthaff.2017.1624
22. Cerga-Pashoja A, Gaete J, Shishkova A, Jordanova V. Improving reading in adolescents and adults with high-functioning autism through an assistive technology tool: a cross-over multinational study. Front Psychiatry. (2019) 10:546. doi: 10.3389/fpsyt.2019.00546
23. Rice S, O'Bree B, Wilson M, McEnery C, Lim MH, Hamilton M, et al. Leveraging the social network for treatment of social anxiety: pilot study of a youth-specific digital intervention with a focus on engagement of young men. Internet Interventions. (2020) 20:100323. doi: 10.1016/j.invent.2020.100323
24. Azevedo R, Bennett N, Bilicki A, Hooper J, Markopoulou F, et al. The calming effect of a new wearable device during the anticipation of public speech. Sci Rep. (2017) 7:2285. doi: 10.1038/s41598-017-02274-2
25. Valmaggia LR, Latif L, Kempton MJ, Rus-Calafell M. Virtual reality in the psychological treatment for mental health problems: an systematic review of recent evidence. Psychiatry Res. (2016) 236:189–195. doi: 10.1016/j.psychres.2016.01.015
26. Riek LD. Chapter 8—robotics technology in mental health care. In: DD Luxton, editor. Artificial Intelligence in Behavioral and Mental Health Care. Notre Dame, IN: Department of Computer Science and Engineering, University of Notre Dame. (2016). p. 185–203. doi: 10.1016/B978-0-12-420248-1.00008-8
27. Abdullah S, Matthews M, Frank E, Doherty G, Gay G, Choudhury T. Automatic detection of social rhythms in bipolar disorder. J Am Med Informat Assoc. (2016) 23:538–43. doi: 10.1093/jamia/ocv200
28. Saeb S, Zhang M, Karr CJ, Schueller SM, Corden ME, Kording KP, et al. Mobile phone sensor correlates of depressive symptom severity in daily-life behavior: an exploratory study. J Med Internet Res. (2015) 17:e175. doi: 10.2196/jmir.4273
29. Christensen H, Cuijpers P, Reynolds CF. Changing the direction of suicide prevention research: a necessity for true population impact. JAMA Psychiatry. (2016) 73:435–6. doi: 10.1001/jamapsychiatry.2016.0001
30. Lo B, Shi J, Hollenberg E, Abi-Jaoude A, Johnson A, Wiljer D. Surveying the role of analytics in evaluating digital mental health interventions for transition-aged youth: a scoping review. JMIR Mental Health. (2020) 7:e15942. doi: 10.2196/15942
31. Blumenthal D. Realizing the value (and Profitability) of digital health data. Ann Intern Med. (2017) 166:842–3. doi: 10.7326/M17-0511
32. Ping NPT, Shoesmith WD, James S, Hadi NMN, Yau EKB, Lin LJ. Ultra brief psychological interventions for covid-19 pandemic: introduction of a locally-adapted brief intervention for mental health and psychosocial support service. Malaysian J Med Sci. (2020) 27:51. doi: 10.21315/mjms2020.27.2.6
33. Yang Y, Li W, Zhang Q, Zhang L, Cheung T, Xiang YT. Mental health services for older adults in China during the COVID-19 outbreak. Lancet Psychiatry. (2020) 7:e19. doi: 10.1016/S2215-0366(20)30079-1
34. Shigemura J, Ursano RJ, Morganstein JC, Kurosawa M, Benedek DM. Public responses to the novel 2019 coronavirus (2019-nCoV) in Japan: Mental health consequences and target populations. Psychiatry Clin Neurosci. (2020) 74:281–2. doi: 10.1111/pcn.12988
35. Wang CJ, Car J, Zuckerman BS. The power of telehealth has been unleashed. Pediatr Clin North Am. (2020) 67:xvii–xviii. doi: 10.1016/j.pcl.2020.05.001
36. Ravens-Sieberer U, Kaman A, Erhart M, Devine J, Schlack R, Otto C. Impact of the COVID-19 pandemic on quality of life and mental health in children and adolescents in Germany. Euro Child Adolesc Psychiatry. (2021). doi: 10.1007/s00787-021-01726-5. [Epub ahead of print].
38. Eston RG, Rowlands AV. Stages in the development of a research project: putting the idea together. British J Sports Med. (2000) 34:59–64. doi: 10.1136/bjsm.34.1.59
39. Mathews SC, McShea MJ, Hanley CL, Ravitz A, Labrique AB, Cohen AB. Digital health: a path to validation. NPJ Digital Med. (2019) 2:38. doi: 10.1038/s41746-019-0111-3
40. Marshall JM, Dunstan DA, Bartik W. The role of digital mental health resources to treat trauma symptoms in australia during COVID-19. Psychol Trauma. (2020) 12:S269–71. doi: 10.1037/tra0000627
41. Hype Cycle Methodology. Available online at: https://www.gartner.com/en/research/methodologies/gartner-hype-cycle (accessed August 26, 2021).
42. Trigwell K, Dunbar-Goddet H. The Research Experience of Postgraduate Research Students at the University of Oxford. Oxford: Institute for the Advancement of University Learning, University of Oxford (2005).
43. Lau N, O'Daffer A, Colt S, Yi-Frazier JP, Palermo TM, McCauley E, et al. Android and iPhone mobile apps for psychosocial wellness and stress management: systematic search in app stores and literature review. JMIR MHealth UHealth. (2020) 8:e17798. doi: 10.2196/17798
44. Michie S, Yardley L, West R, Patrick K, Greaves F. Developing and evaluating digital interventions to promote behavior change in health and health care: recommendations resulting from an international workshop. J Med Internet Res. (2017) 19:e232. doi: 10.2196/jmir.7126
45. Alshurafa N, Jain J, Alharbi R, Iakovlev G, Spring B, Pfammatter A. Is more always better?: Discovering incentivized mhealth intervention engagement related to health behavior trends. Proc ACM Interact Mob Wearable Ubiquitous Technol. (2018) 2:153. doi: 10.1145/3287031
46. Champion L, Economides M, Chandler C. The efficacy of a brief app-based mindfulness intervention on psychosocial outcomes in healthy adults: a pilot randomised controlled trial. PLoS ONE. (2018) 13:e0209482. doi: 10.1371/journal.pone.0209482
47. Wilson K, Bell C, Wilson L, Witteman H. Agile research to complement agile development: a proposal for an mHealth research lifecycle. NPJ Digital Med. (2018) 1:46. doi: 10.1038/s41746-018-0053-1
48. Lattie EG, Graham AK, Hadjistavropoulos HD, Dear BF, Titov N, Mohr DC. Guidance on defining the scope and development of text-based coaching protocols for digital mental health interventions. Digital Health. (2019) 5:2055207619896145. doi: 10.1177/2055207619896145
49. Meyer JHF, Shanahan MP, Laugksch RC. Students' conceptions of research. I: A qualitative and quantitative analysis. Scand J Educ Res. (2005) 49:225–44. doi: 10.1080/00313830500109535
50. Loncar-Turukalo T, Zdravevski E, Machado da Silva J, Chouvarda I, Trajkovik V. Literature on wearable technology for connected health: scoping review of research trends, advances, and barriers. J Med Internet Res. (2019) 21:e14017. doi: 10.2196/14017
51. Urquhart C, Currell R. Systematic reviews and meta-analysis of health IT. Stud Health Technol Inform. (2016) 222:262–74. Available online at: https://www.nobelprize.org/prizes/economic-sciences/2002/kahneman/lecture
52. Murray E, Burns J, May C, Finch T, O'Donnell C, Wallace P, et al. Why is it difficult to implement e-health initiatives? A qualitative study. Implement Sci. (2011) 6:6. doi: 10.1186/1748-5908-6-6
53. Liu C, Shao S, Liu C, Bennett GG, Prvu Bettger J, Yan LL. Academia-industry digital health collaborations: a cross-cultural analysis of barriers and facilitators. Digital Health. (2019) 5:2055207619878627. doi: 10.1177/2055207619878627
54. Lupton D. Digital health now and in the future: findings from a participatory design stakeholder workshop. Digital Health. (2017) 3:2055207617740018. doi: 10.4324/9781315648835
55. Joerin A, Rauws M, Fulmer R, Black V. Ethical artificial intelligence for digital health organizations. Cureus. (2020) 12:e7202. doi: 10.7759/cureus.7202
56. Jandoo T. WHO guidance for digital health: What it means for researchers. Digit Health. (2020) 6:2055207619898984. doi: 10.1177/2055207619898984
57. Murray E, Khadjesari Z, White IR, Kalaitzaki E, Godfrey C, McCambridge J, et al. Methodological challenges in online trials. J Med Internet Res. (2009) 11:e9. doi: 10.2196/jmir.1052
58. Gianfrancesco MA, Tamang S, Yazdany J, Schmajuk G. Potential biases in machine learning algorithms using electronic health record data. JAMA Intern Med. (2018) 178:1544–7. doi: 10.1001/jamainternmed.2018.3763
59. d'Alessandro B, O'Neil C, LaGatta T. Conscientious classification: a data scientist's guide to discrimination-aware classification. Big Data. (2017) 5:120–34. doi: 10.1089/big.2016.0048
60. Plante TB, Urrea B, MacFarlane ZT, Blumenthal RS, Miller ER, Appel LJ, et al. Validation of the instant blood pressure smartphone app. JAMA Intern Med. (2016) 176:700–2. doi: 10.1001/jamainternmed.2016.0157
61. Poli A, Kelfve S, Motel-Klingebiel A. A research tool for measuring non-participation of older people in research on digital health. BMC Public Health. (2019) 19:1487. doi: 10.1186/s12889-019-7830-x
62. Dockendorf MF, Murthy G, Bateman KP, Kothare PA, Anderson M, Xie I, et al. Leveraging digital health technologies and outpatient sampling in clinical drug development: a phase i exploratory study. Clin Pharmacol Ther. (2019) 105:168–76. doi: 10.1002/cpt.1142
63. Liang J, He X, Jia Y, Zhu W, Lei J. Chinese mobile health apps for hypertension management: a systematic evaluation of usefulness. J Healthc Eng. (2018) 2018:7328274. doi: 10.1155/2018/7328274
64. Germine L, Reinecke K, Chaytor NS. Digital neuropsychology: challenges and opportunities at the intersection of science and software. Clin Neuropsychol. (2019) 33:271–86. doi: 10.1080/13854046.2018.1535662
66. Norman DA, Draper SW. User Centered System Design: New Perspectives on Human-computer Interaction. Boca Raton, FL: CRC Press (1986). doi: 10.1201/b15703
67. Ambler SW. Lessons in agility from Internet-based development. IEEE Software. (2002) 19:66–73. doi: 10.1109/52.991334
69. Benyon D. Designing Interactive Systems: A Comprehensive Guide to HCI, UX and Interaction Design. Pearson (2014). Available online at: https://www.pearson.com/uk/educators/higher-education-educators/program/Benyon-Designing-Interactive-Systems-A-comprehensive-guide-to-HCI-UX-and-interaction-design-3rd-Edition/PGM1047688.html (accessed August 26, 2021).
70. Faurholt-Jepsen M, Vinberg M, Frost M, Christensen EM, Bardram J, Kessing LV. Daily electronic monitoring of subjective and objective measures of illness activity in bipolar disorder using smartphones–the MONARCA II trial protocol: a randomized controlled single-blind parallel-group trial. BMC Psychiatry. (2014) 14:309. doi: 10.1186/s12888-014-0309-5
71. Freeman D. Studying and treating schizophrenia using virtual reality: a new paradigm. Schizophr Bull. (2008) 34:605–10. doi: 10.1093/schbul/sbn020
72. Granja C, Janssen W, Johansen MA. Factors determining the success and failure of ehealth interventions: systematic review of the literature. J Med Internet Res. (2018) 20:e10235. doi: 10.2196/10235
73. Heeks R. Health information systems: failure, success and improvisation. Int J Med Inform. (2006) 75:125–37. doi: 10.1016/j.ijmedinf.2005.07.024
74. Stott R, Wild J, Grey N, Liness S, Warnock-Parkes E, Commins S, et al. Internet-delivered cognitive therapy for social anxiety disorder: a development pilot series. Behav Cogn Psychother. (2013) 41:383–97. doi: 10.1017/S1352465813000404
75. Gorst SL, Armitage CJ, Brownsell S, Hawley MS. Home telehealth uptake and continued use among heart failure and chronic obstructive pulmonary disease patients: a systematic review. Ann Behav Med. (2014) 48:323–36. doi: 10.1007/s12160-014-9607-x
76. Sanders C, Rogers A, Bowen R, Bower P, Hirani S, Cartwright M, et al. Exploring barriers to participation and adoption of telehealth and telecare within the Whole System Demonstrator trial: a qualitative study. BMC Health Serv Res. (2012) 12:220. doi: 10.1186/1472-6963-12-220
77. Huckvale K, Wang CJ, Majeed A, Car J. Digital health at fifteen: More human (more needed). BMC Med. (2019) 17:62. doi: 10.1186/s12916-019-1302-0
78. Nutt AE. ‘The Woebot will see you now'—The Rise of Chatbot Therapy. Washington Post (2017). Available online at: https://www.washingtonpost.com/news/to-your-health/wp/2017/12/03/the-woebot-will-see-you-now-the-rise-of-chatbot-therapy/ (accessed August 26, 2021).
79. Fairburn CG, Patel V. The impact of digital technology on psychological treatments and their dissemination. Behav Res Ther. (2017) 88:19–5. doi: 10.1016/j.brat.2016.08.012
80. Berwick DM. What “patient-centered” should mean: confessions of an extremist. Health Affairs. (2009) 28:w555–65. doi: 10.1377/hlthaff.28.4.w555
81. Torous J. Mobile telephone apps first need data security and efficacy. BJPsych Bulletin. (2016) 40:106–7. doi: 10.1192/pb.40.2.106b
82. Byambasuren O, Sanders S, Beller E, Glasziou P. Prescribable mHealth apps identified from an overview of systematic reviews. Npj Digital Med. (2018) 1:1–12. doi: 10.1038/s41746-018-0021-9
83. Miyamoto S, Henderson S, Young HM, Ward D, Santillan V. Recruiting rural participants for a telehealth intervention on diabetes self-management. J Rural Health. (2013) 29:69–77. doi: 10.1111/j.1748-0361.2012.00443.x
84. Goel MS, Brown TL, Williams A, Cooper AJ, Hasnain-Wynia R, Baker DW. Patient reported barriers to enrolling in a patient portal. J Am Med Informat Assoc. (2011) 18(Suppl. 1):i8–12. doi: 10.1136/amiajnl-2011-000473
85. Lakerveld J, Ijzelenberg W, van Tulder MW, Hellemans IM, Rauwerda JA, van Rossum AC, et al. Motives for (not) participating in a lifestyle intervention trial. BMC Med Res Methodol. (2008) 8:17. doi: 10.1186/1471-2288-8-17
86. O'Connor S, Mair FS, McGee-Lennon M, Bouamrane M, O'Donnell K. Engaging in large-scale digital health technologies and services. What factors hinder recruitment? Stud Health Technol Inform. (2015) 210:306–10.
87. Dyrbye LN, Shanafelt TD, Sinsky CA, Cipriano PF, Bhatt J, Ommaya A, et al. Burnout Among Health Care Professionals: A Call to Explore and Address this Underrecognized Threat to Safe, High-Quality Care. NAM Perspectives. Discussion Paper, National Academy of Medicine, Washington, DC (2017). doi: 10.31478/201707b
88. Greenhalgh T, Procter R, Wherton J, Sugarhood P, Hinder S, Rouncefield M. What is quality in assisted living technology? The ARCHIE framework for effective telehealth and telecare services. BMC Med. (2015) 13:91. doi: 10.1186/s12916-015-0279-6
89. Torous J, Nicholas J, Larsen ME, Firth J, Christensen H. Clinical review of user engagement with mental health smartphone apps: evidence, theory and improvements. Evid Based Ment Health. (2018) 21:116–9. doi: 10.1136/eb-2018-102891
90. Opoku D, Stephani V, Quentin W. A realist review of mobile phone-based health interventions for non-communicable disease management in sub-Saharan Africa. BMC Med. (2017) 15:24. doi: 10.1186/s12916-017-0782-z
91. O'Connor S, Hanlon P, O'Donnell CA, Garcia S, Glanville J, Mair FS. Understanding factors affecting patient and public engagement and recruitment to digital health interventions: a systematic review of qualitative studies. BMC Med Inform Decis Mak. (2016) 16:120. doi: 10.1186/s12911-016-0359-3
92. Choi NG, DiNitto DM. The digital divide among low-income homebound older adults: internet use patterns, ehealth literacy, and attitudes toward computer/internet use. J Med Internet Res. (2013) 15:e93. doi: 10.2196/jmir.2645
93. Selwyn N, Gorard S, Furlong J, Madden L. Older adults' use of information and communications technology in everyday life. Ageing Soc. (2003) 23:561–82. doi: 10.1017/S0144686X03001302
94. Cashen MS, Dykes P, Gerber B. eHealth technology and Internet resources: Barriers for vulnerable populations. J Cardiovasc Nursing. (2004) 19:209–14; quiz 215–216. doi: 10.1097/00005082-200405000-00010
95. Kontos E, Blake KD, Chou WYS, Prestin A. Predictors of eHealth usage: Insights on the digital divide from the Health Information National Trends Survey 2012. J Med Internet Res. (2014) 16:e172. doi: 10.2196/jmir.3117
96. Neter E, Brainin E. eHealth literacy: extending the digital divide to the realm of health information. J Med Internet Res. (2012) 14:e19. doi: 10.2196/jmir.1619
97. de Ruyter B. User centred design. In Aarts E, Marzano S, editors. In: The New Everyday: Views on Ambient Intelligence. 010 Publishers (2003).
98. Bowen DJ, Kreuter M, Spring B, Cofta-Woerpel L, Linnan L, Weiner D, et al. How we design feasibility studies. Am J Prev Med. (2009) 36:452–7. doi: 10.1016/j.amepre.2009.02.002
99. Steen M. Human-centered design as a fragile encounter. Design Issues. (2012) 28:72–80. JSTOR. doi: 10.1162/DESI_a_00125
100. Croskerry P. From mindless to mindful practice—Cognitive bias and clinical decision making. N Engl J Med. (2013) 368:2445–8. doi: 10.1056/NEJMp1303712
101. Croskerry P, Singhal G, Mamede S. Cognitive debiasing 1: origins of bias and theory of debiasing. BMJ Qual Safety. (2013) 22(Suppl. 2):ii58–64. doi: 10.1136/bmjqs-2012-001712
102. Zwaan L, Thijs A, Wagner C, Timmermans DRM. Does inappropriate selectivity in information use relate to diagnostic errors and patient harm? The diagnosis of patients with dyspnea. Soc Sci Med. (2013) 91:32–8. doi: 10.1016/j.socscimed.2013.05.001
103. Kahneman D. 'Maps of bounded rationality: a perspective on intuitive judgment and choice'. Nobel Prize Lecture. (2002) 8, 351–401. Available online at: https://www.nobelprize.org/prizes/economic-sciences/2002/kahneman/lecture/
104. Tversky A, Kahneman D. Judgment under uncertainty: heuristics and biases. Science. (1974) 185:1124–31. doi: 10.1126/science.185.4157.1124
105. Ely JW, Graber ML, Croskerry P. Checklists to reduce diagnostic errors. Acad Med. (2011) 86:307–13. doi: 10.1097/ACM.0b013e31820824cd
106. Mamede S, van Gog T, van den Berge K, van Saase JLCM, Schmidt HG. Why do doctors make mistakes? A study of the role of salient distracting clinical features. Acad Med. (2014) 89:114–20. doi: 10.1097/ACM.0000000000000077
107. van den Berge K, Mamede S. Cognitive diagnostic error in internal medicine. Eur J Intern Med. (2013) 24:525–9. doi: 10.1016/j.ejim.2013.03.006
108. Appel S, Wadas T, Talley M, Williams A. Teaching diagnostic reasoning: transitioning from a live to a distance accessible online classroom in an Adult Acute Care Nurse Practitioner Program. J Nursing Educ Practice. (2014) 3. doi: 10.5430/jnep.v3n12p125
109. Ioannidis JP, Lau J. Evidence on interventions to reduce medical errors: an overview and recommendations for future research. J Gen Intern Med. (2001) 16:325–34. doi: 10.1046/j.1525-1497.2001.00714.x
110. OECD. Health at a Glance 2013. (2013). Available online at: https://www.oecd-ilibrary.org/content/publication/health_glance-2013-en (accessed August 26, 2021).
111. Lawson TN. Diagnostic reasoning and cognitive biases of nurse practitioners. J Nurs Educ. (2018) 57:203–8. doi: 10.3928/01484834-20180322-03
112. Saposnik G, Redelmeier D, Ruff CC, Tobler PN. Cognitive biases associated with medical decisions: a systematic review. BMC Med Inform Decision Making. (2016) 16:138. doi: 10.1186/s12911-016-0377-1
113. Brosinski CM. Implementing diagnostic reasoning to differentiate Todd's paralysis from acute ischemic stroke. Adv Emerg Nurs J. (2014) 36:78–86. doi: 10.1097/TME.0000000000000007
114. Elstein AS. Thinking about diagnostic thinking: a 30-year perspective. Adv Health Sci Educ. (2009) 14(Suppl).1:7–18. doi: 10.1007/s10459-009-9184-0
115. Ilgen JS, Eva KW, Regehr G. What's in a label? Is diagnosis the start or the end of clinical reasoning? J General Internal Med. (2016) 31:435–7. doi: 10.1007/s11606-016-3592-7
116. Monteiro SM, Norman G. Diagnostic reasoning: where we've been, where we're going. Teach Learn Med. (2013) 25(Suppl. 1):S26–32. doi: 10.1080/10401334.2013.842911
117. Pirret AM, Neville SJ, La Grow SJ. Nurse practitioners versus doctors diagnostic reasoning in a complex case presentation to an acute tertiary hospital: A comparative study. Int J Nurs Stud. (2015) 52:716–26. doi: 10.1016/j.ijnurstu.2014.08.009
118. Sherbino J, Kulasegaram K, Howey E, Norman G. Ineffectiveness of cognitive forcing strategies to reduce biases in diagnostic reasoning: a controlled trial. CJEM. (2014) 16:34–40. doi: 10.2310/8000.2013.130860
119. Thammasitboon S, Cutrer WB. Diagnostic decision-making and strategies to improve diagnosis. Curr Probl Pediatr Adolesc Health Care. (2013) 43:232–41. doi: 10.1016/j.cppeds.2013.07.003
120. Thompson C. Clinical experience as evidence in evidence-based practice. J Adv Nurs. (2003) 43:230–7. doi: 10.1046/j.1365-2648.2003.02705.x
121. Thammasitboon S, Thammasitboon S, Singhal G. Diagnosing diagnostic error. Curr Probl Pediatr Adolesc Health Care. (2013) 43:227–31. doi: 10.1016/j.cppeds.2013.07.002
122. Baldwin RL, Green JW, Shaw JL, Simpson DD, Bird TM, Cleves MA, et al. Physician risk attitudes and hospitalization of infants with bronchiolitis. Acad Emergency Med. (2005) 12:142–6. doi: 10.1197/j.aem.2004.10.002
123. Yee LM, Liu LY, Grobman WA. The relationship between obstetricians' cognitive and affective traits and their patients' delivery outcomes. Am J Obstetr Gynecol.(2014) 211:692.e1–6. doi: 10.1016/j.ajog.2014.06.003
124. Källberg A-S, Göransson KE, Östergren J, Florin J, Ehrenberg A. Medical errors and complaints in emergency department care in Sweden as reported by care providers, healthcare staff, and patients—a national review. Europ J Emergency Med. (2013) 20:33–8. doi: 10.1097/MEJ.0b013e32834fe917
125. Studdert DM, Mello MM, Gawande AA, Gandhi TK, Kachalia A, Yoon C, et al. Claims, errors, and compensation payments in medical malpractice litigation. New Engl J Med. (2006) 354:2024–33. doi: 10.1056/NEJMsa054479
126. Zwaan L, Thijs A, Wagner C, van der Wal G, Timmermans DRM. Relating faults in diagnostic reasoning with diagnostic errors and patient harm. Acad Med. (2012) 87:149–56. doi: 10.1097/ACM.0b013e31823f71e6
127. Lazarus RS, Folkman S. Stress, Appraisal, and Coping. San Francisco, CA: Department of Medicine, School of Medicine University of California (1984).
128. Miller SM. Monitoring and blunting: validation of a questionnaire to assess styles of information seeking under threat. J Pers Soc Psychol. (1987) 52:345–53. doi: 10.1037/0022-3514.52.2.345
129. Deldin PJ, Keller J, Gergen JA, Miller GA. Cognitive bias and emotion in neuropsychological models of depression. Cogn Emot. (2001) 15:787–802. doi: 10.1080/02699930143000248
130. Andel C, Davidow SL, Hollander M, Moreno DA. The economics of health care quality and medical errors. J Health Care Finance. (2012) 39:39–50.
131. Blackwell SE, Browning M, Mathews A, Pictet A, Welch J, Davies J, et al. Positive imagery-based cognitive bias modification as a web-based treatment tool for depressed adults: a randomized controlled trial. Clin Psychol Sci. (2015) 3:91–111. doi: 10.1177/2167702614560746
132. Freeman D, Bradley J, Antley A, Bourke E, DeWeever N, Evans N, et al. Virtual reality in the treatment of persecutory delusions: randomised controlled experimental study testing how to reduce delusional conviction. Br J Psychiatry. (2016) 209:62–7. doi: 10.1192/bjp.bp.115.176438
133. van Spijker BAJ, van Straten A, Kerkhof AJFM. Effectiveness of online self-help for suicidal thoughts: results of a randomised controlled trial. PLoS ONE. (2014) 9:e90118. doi: 10.1371/journal.pone.0090118
134. Croskerry P. The importance of cognitive errors in diagnosis and strategies to minimize them. Acad Med. (2003) 78:775–80. doi: 10.1097/00001888-200308000-00003
135. Michaels AD, Spinler SA, Leeper B, Ohman EM, Alexander KP, Newby LK, et al. Medication errors in acute cardiovascular and stroke patients: a scientific statement from the American Heart Association. Circulation. (2010) 121:1664–82. doi: 10.1161/CIR.0b013e3181d4b43e
136. Agarwal R. Five Cognitive Biases in Data Science (and How to Avoid Them). Built In (2020). Available online at: https://builtin.com/data-science/cognitive-biases-data-science (accessed August 26, 2021).
137. Caliskan A, Bryson JJ, Narayanan A. Semantics derived automatically from language corpora contain human-like biases. Science. (2017) 356:183–6. doi: 10.1126/science.aal4230
138. Eubanks V. Automating Inequality: How High-tech Tools Profile, Police, and Punish the Poor. St Martin's Press (2018).
139. Noble SU. Algorithms of Oppression: How Search Engines Reinforce Racism. New York, NY: New York University Press (2018). doi: 10.2307/j.ctt1pwt9w5
140. O'Neil C. Weapons of Math Destruction: How Big Data Increases Inequality and Threatens Democracy. Crown (2016).
141. Çalikli G, Bener AB. Influence of confirmation biases of developers on software quality: an empirical study. Software Qual J. (2013) 21:377–416. doi: 10.1007/s11219-012-9180-0
142. Nelson GS. Bias in artificial intelligence. N C Med J. (2019) 80:220–2. doi: 10.18043/ncm.80.4.220
143. Ntoutsi E, Fafalios P, Gadiraju U, Iosifidis V, Nejdl W, Vidal ME, et al. Bias in data-driven artificial intelligence systems—an introductory survey. Wiley Interdisciplin Rev. (2020) 10:e1356. doi: 10.1002/widm.1356
144. Cabitza F, Rasoini R, Gensini GF. Unintended consequences of machine learning in medicine. JAMA. (2017) 318:517–8. doi: 10.1001/jama.2017.7797
145. Char DS, Shah NH, Magnus D. Implementing machine learning in health care—addressing ethical challenges. N Engl J Med. (2018) 378:981–3. doi: 10.1056/NEJMp1714229
146. Arpey NC, Gaglioti AH, Rosenbaum ME. How socioeconomic status affects patient perceptions of health care: a qualitative study. J Prim Care Community Health. (2017) 8:169–75. doi: 10.1177/2150131917697439
147. Ng JH, Ye F, Ward LM, Haffer SCC, Scholle SH. Data on race, ethnicity, and language largely incomplete for managed care plan members. Health Affairs. (2017) 36:548–52. doi: 10.1377/hlthaff.2016.1044
148. Rauscher GH, Khan JA, Berbaum ML, Conant EF. Potentially missed detection with screening mammography: does the quality of radiologist's interpretation vary by patient socioeconomic advantage/disadvantage? Ann Epidemiol. (2013) 23:210–4. doi: 10.1016/j.annepidem.2013.01.006
149. Li S, Fonarow GC, Mukamal KJ, Liang L, Schulte PJ, Smith EE, et al. Sex and race/ethnicity-related disparities in care and outcomes after hospitalization for coronary artery disease among older adults. Circul Cardiovasc Qual Outcomes. (2016) 9(2 Suppl. 1):S36–44. doi: 10.1161/CIRCOUTCOMES.115.002621
150. Grazzini J, Pantisano F. Guidelines for Scientific Evidence Provision for Policy Support Based on Big Data and Open Technologies (2015).
151. Fayyad U, Piatetsky-Shapiro G, Smyth P. From data mining to knowledge discovery in databases. AI Magazine. (1996) 17:37.
152. SAS Institute Inc. SAS Institute Whitepaper. “The 5 Essential Components of a Data Strategy. (2016).” Available online at: https://www.sas.com/content/dam/SAS/en_au/doc/whitepaper1/five-essential-components-data-strategy.pdf (accessed August 26, 2021).
153. Rahm E, Do HH. Data cleaning: problems and current approaches. IEEE Data Eng Bull. (2000) 23:3–13.
154. Müller H, Freytag JC. Problems, methods, and challenges in comprehensive data cleansing. Humboldt-Univ. zu Berlin. (2005).
155. Famili A, Shen WM, Weber R, Simoudis E. Data preprocessing and intelligent data analysis. Intelligent Data Analy. (1997) 1:3–23. doi: 10.3233/IDA-1997-1102
156. Mittelstadt B, Floridi L. The Ethics of Biomedical Big Data. Vol. 29. Springer(2016). doi: 10.1007/978-3-319-33525-4
157. Aledavood T, Triana Hoyos AM, Alakörkkö T, Kaski K, Saramäki J, Isometsä E, et al. Data collection for mental health studies through digital platforms: requirements and design of a prototype. JMIR Res Protoc. (2017) 6:e110. doi: 10.2196/resprot.6919
159. Furche T, Gottlob G, Libkin L, Orsi G, Paton NW. Data wrangling for big data: challenges and opportunities. EDBT. (2016) 16:473–8.
161. Alasadi SA, Bhaya WS. Review of data preprocessing techniques in data mining. J Eng Appl Sci. (2017) 12:4102–7. doi: 10.36478/jeasci.2017.4102.4107
162. Bilalli B, Abelló A, Aluja-Banet T, Wrembel R. Intelligent assistance for data pre-processing. Computer Standards Interfaces. (2018) 57:101–9. doi: 10.1016/j.csi.2017.05.004
163. Bzdok D, Altman N, Krzywinski M. Statistics versus machine learning. Nat Methods. (2018) 15:233–4. doi: 10.1038/nmeth.4642
164. Tomar D, Agarwal S. A survey on Data Mining approaches for Healthcare. Int J Bio-Sci Bio-Technol. (2013) 5:241–66. doi: 10.14257/ijbsbt.2013.5.5.25
165. Bishop C. Pattern Recognition and Machine Learning. Springer-Verlag (2006). Available online at: https://www.springer.com/gp/book/9780387310732 (accessed August 26, 2021).
166. Husain W, Xin LK, Rashid NA, Jothi N. Predicting Generalized Anxiety Disorder among women using random forest approach. 2016 3rd International Conference on Computer and Information Sciences (ICCOINS). (2016). p. 37–42. doi: 10.1109/ICCOINS.2016.7783185
167. Jothi N, Husain W, Rashid NA, Xin LK. Predicting generalised anxiety disorder among women using decision tree-based classification. Int J Business Informat Syst. (2018) 29:75–91. doi: 10.1504/IJBIS.2018.093998
168. Jothi N, Husain W, Rashid NA. Predicting generalized anxiety disorder among women using Shapley value. J Infect Public Health. (2020) 14:103–8. doi: 10.1016/j.jiph.2020.02.042
169. Yengil E, Acipayam C, Kokacya MH, Kurhan F, Oktay G, Ozer C. Anxiety, depression and quality of life in patients with beta thalassemia major and their caregivers. Int J Clin Exp Med. (2014) 7:2165–72.
170. Goodman AA, Borkin MA, Robitaille TP. New thinking on, and with, data visualization. ArXiv. (2018).
171. Bakker D, Kazantzis N, Rickwood D, Rickard N. Mental health smartphone apps: review and evidence-based recommendations for future developments. JMIR Mental Health. (2016) 3:e7. doi: 10.2196/mental.4984
172. Marshall JM, Dunstan DA, Bartik W. The digital psychiatrist: in search of evidence-based apps for anxiety and depression. Front Psychiatry. (2019) 10:831. doi: 10.3389/fpsyt.2019.00831
173. Craig P, Dieppe P, Macintyre S, Michie S, Nazareth I, Petticrew M. Developing and evaluating complex interventions: The new Medical Research Council guidance. BMJ. (2008) 337:a1655. doi: 10.1136/bmj.a1655
174. Kennedy-Martin T, Curtis S, Faries D, Robinson S, Johnston J. A literature review on the representativeness of randomized controlled trial samples and implications for the external validity of trial results. Trials. (2015) 16:495. doi: 10.1186/s13063-015-1023-4
175. Rothwell PM. External validity of randomised controlled trials: “to whom do the results of this trial apply?” Lancet. (2005) 365:82–93. doi: 10.1016/S0140-6736(04)17670-8
176. Hoffmann TC, Glasziou PP, Boutron I, Milne R, Perera R, Moher D, et al. Better reporting of interventions: template for intervention description and replication (TIDieR) checklist and guide. BMJ. (2014) 348:g1687. doi: 10.1136/bmj.g1687
177. Muris P, Roodenrijs D, Kelgtermans L, Sliwinski S, Berlage U, Baillieux H, et al. No Medication for My Child! A naturalistic study on the treatment preferences for and effects of cogmed working memory training versus psychostimulant medication in clinically referred youth with ADHD. Child Psychiatry Human Dev. (2018) 49:974–92. doi: 10.1007/s10578-018-0812-x
178. Khadjesari Z, Stevenson F, Godfrey C, Murray E. Negotiating the ‘grey area between normal social drinking and being a smelly tramp': a qualitative study of people searching for help online to reduce their drinking. Health Expectat. (2015) 18:2011–20. doi: 10.1111/hex.12351
179. Marewski JN, Gigerenzer G. Heuristic decision making in medicine. Dialogues Clin Neurosci. (2012) 14:77–89. doi: 10.31887/DCNS.2012.14.1/jmarewski
180. Veltman KH. Syntactic and semantic interoperability: new approaches to knowledge and the semantic web. New Rev Informat Networking. (2001) 7:159–83. doi: 10.1080/13614570109516975
181. Gordon WJ, Catalini C. Blockchain technology for healthcare: facilitating the transition to patient-driven interoperability. Comput Struct Biotechnol J. (2018) 16:224–30. doi: 10.1016/j.csbj.2018.06.003
182. Gilpin LH, Bau D, Yuan BZ, Bajwa A, Specter M, Kagal L. Explaining explanations: an overview of interpretability of machine learning. In: 2018 IEEE 5th International Conference on Data Science and Advanced Analytics (DSAA). (2018). p. 80–9. doi: 10.1109/DSAA.2018.00018
183. Angwin J, Larson J, Mattu S, Kirchner L. Machine Bias. ProPublica. (2016). Available online at: https://www.propublica.org/article/machine-bias-risk-assessments-in-criminal-sentencing
184. Kaadoud IC, Rougier NP, Alexandre F. Knowledge extraction from the learning of sequences in a long short term memory (LSTM) architecture. ArXiv. (2019).
185. Kim WC, Mauborgne R. Blue ocean leadership. Harvard Business Rev. (2014) 92:60–8, 70, 72 passim.
188. Guise J-M, Chang C, Viswanathan M, Glick S, Treadwell J, et al. Systematic Reviews of Complex Multicomponent Health Care Interventions. Agency for Healthcare Research and Quality (US) (2014). Available online at: http://www.ncbi.nlm.nih.gov/books/NBK194846/
189. Arrieta AB, Díaz-Rodríguez N, Del Ser J, Bennetot A, Tabik S, Barbado A, et al. Explainable Artificial Intelligence (XAI): concepts, taxonomies, opportunities and challenges toward responsible AI. Informat Fusion. (2020) 58:82–115. doi: 10.1016/j.inffus.2019.12.012
190. Kamishima T, Akaho S, Asoh H, Sakuma J. Fairness-aware classifier with prejudice remover regularizer. In Flach PA. De Bie T, Cristianini N, editors. Machine Learning and Knowledge Discovery in Databases. Springer(2012). p. 35–50. doi: 10.1007/978-3-642-33486-3_3
191. Bourne KC. Chapter 19—your organization. In: Bourne KC, editor. Application Administrators Handbook. Morgan Kaufmann (2014). p. 329–43. doi: 10.1016/B978-0-12-398545-3.00019-4
192. Rodiya K, Gill P. A review on anonymization techniques for privacy preserving data publishing. Int J Eng Res Technol. (2015) 4:228–231. doi: 10.15623/ijret.2015.0411039
193. Kido T, Takadama K. The Challenges for Interpretable AI for Well-being -Understanding Cognitive Bias and Social Embeddedness. CEUR-WS 2448 (2019).
194. Guidotti R, Monreale A, Ruggieri S, Turini F, Pedreschi D, Giannotti F. A survey of methods for explaining black box models. ArXiv. (2018). doi: 10.1145/3236009
195. Wathelet M, Duhem S, Vaiva G, Baubet T, Habran E, Veerapa E, et al. Factors associated with mental health disorders among university students in France confined during the COVID-19 pandemic. JAMA Network Open. (2020) 3:e2025591. doi: 10.1001/jamanetworkopen.2020.25591
196. Goals and Principles for Data Governance. Available online at: http://www.datagovernance.com/adg_data_governance_goals/
197. Bengio Y, Courville A, Vincent P. Representation learning: a review and new perspectives. IEEE Trans Pattern Anal Mach Intell. (2013) 35:1798–828. doi: 10.1109/TPAMI.2013.50
198. Alasadi SA, Bhaya WS. Review of data preprocessing techniques in data mining. J Eng Appl Sci. (2017) 12:4102–7.
199. Nguyen A, Yosinski J, Clune J. Deep neural networks are easily fooled: High confidence predictions for unrecognizable images. 2015 IEEE Conference on Computer Vision and Pattern Recognition (CVPR). (2015). p. 427–36. doi: 10.1109/CVPR.2015.7298640
200. Li W, Yang Y, Liu ZH, Zhao YJ, Zhang Q, Zhang L, et al. Progression of mental health services during the COVID-19 outbreak in China. Int J Biol Sci. (2020) 16:1732. doi: 10.7150/ijbs.45120
201. Sebastian-Coleman L. Measuring Data Quality for Ongoing Improvement: A Data Quality Assessment Framework. Newnes. (2012). doi: 10.1016/B978-0-12-397033-6.00020-1
202. Taylor J, Pagliari C. Comprehensive scoping review of health research using social media data. BMJ Open. (2018) 8:e022931. doi: 10.1136/bmjopen-2018-022931
203. Selvi U, Pushpa S. A review of big data and anonymization algorithms. Int J Appl Eng Res. (2015) 10.
204. Huckvale K, Prieto JT, Tilney M, Benghozi PJ, Car J. Unaddressed privacy risks in accredited health and wellness apps: a cross-sectional systematic assessment. BMC Med. (2015) 13:214. doi: 10.1186/s12916-015-0444-y
205. Eysenbach Group C.-E. CONSORT-EHEALTH: improving and standardizing evaluation reports of web-based and mobile health interventions. J Med Internet Res. (2011) 13:e126. doi: 10.2196/jmir.1923
206. Ayache S, Eyraud R, Goudian N. Explaining black boxes on sequential data using weighted automata. ArXiv. (2018).
207. Ma H, Miller C. Trapped in a double bind: Chinese overseas student anxiety during the COVID-19 pandemic. Health Commun.(2020) 1–8. doi: 10.1080/10410236.2020.1775439
208. Deshazo JP, Lavallie DL, Wolf FM. Publication trends in the medical informatics literature: 20 years of “Medical Informatics” in MeSH. BMC Med Inform Decis Mak. (2009) 9:7. doi: 10.1186/1472-6947-9-7
Keywords: digital mental health, an end-to-end methodology, human factors, cognitive biases, machine learning, knowledge discovery data base (KDD), interdisciplinar intersectoral collaborations, ethics
Citation: Boulos LJ, Mendes A, Delmas A and Chraibi Kaadoud I (2021) An Iterative and Collaborative End-to-End Methodology Applied to Digital Mental Health. Front. Psychiatry 12:574440. doi: 10.3389/fpsyt.2021.574440
Received: 19 June 2020; Accepted: 12 August 2021;
Published: 23 September 2021.
Edited by:
Jennifer H. Barnett, Cambridge Cognition, United KingdomReviewed by:
Erping Long, National Institutes of Health (NIH), United StatesCopyright © 2021 Boulos, Mendes, Delmas and Chraibi Kaadoud. This is an open-access article distributed under the terms of the Creative Commons Attribution License (CC BY). The use, distribution or reproduction in other forums is permitted, provided the original author(s) and the copyright owner(s) are credited and that the original publication in this journal is cited, in accordance with accepted academic practice. No use, distribution or reproduction is permitted which does not comply with these terms.
*Correspondence: Laura Joy Boulos, bGF1cmFqb3lib3Vsb3NAZ21haWwuY29t; Alexandre Mendes, YS5tZW5kZXNAZ3JvdXBlb25lcG9pbnQuY29t; Alexandra Delmas, YS5kZWxtYXNAZ3JvdXBlb25lcG9pbnQuY29t; Ikram Chraibi Kaadoud, aWNocmFpYmlrQG91dGxvb2suZnI=
Disclaimer: All claims expressed in this article are solely those of the authors and do not necessarily represent those of their affiliated organizations, or those of the publisher, the editors and the reviewers. Any product that may be evaluated in this article or claim that may be made by its manufacturer is not guaranteed or endorsed by the publisher.
Research integrity at Frontiers
Learn more about the work of our research integrity team to safeguard the quality of each article we publish.