- 1Laboratory of Brain Imaging, Nencki Institute of Experimental Biology of Polish Academy of Sciences, Warsaw, Poland
- 2Department of Psychiatry, Yale University School of Medicine, New Haven, CT, United States
- 3Child Study Center, Yale University School of Medicine, New Haven, CT, United States
- 4Department of Neuroscience, Yale University, New Haven, CT, United States
- 5Connecticut Mental Health Center, New Haven, CT, United States
- 6Connecticut Council on Problem Gambling, Wethersfield, CT, United States
Self-regulation (SR) is an important human function that relates to quality of life in multiple domains including mental health. Previous studies have found important correlates of low SR including impulsivity and poor emotional regulation; however, underpinnings of low SR are incompletely understood. Individuals low in SR frequently engage in maladaptive behaviors (substance abuse, procrastination, etc.) despite negative consequences. This phenomenon suggests that impaired learning from errors and punishments may be important mechanisms underlying low SR. Consistently, previous studies observed impaired error processing in a wide spectrum of individuals with low SR and impaired learning from errors and punishments in SR-related disorders. We also note a possible role for poor emotional regulation and refer to concepts suggesting that engaging in maladaptive behaviors may serve as short term emotion regulation strategies aimed at avoiding or alleviating negative affect. We speculate on transdiagnostic factors underlying poor SR. We propose that impaired error processing (possibly related to striatal functioning) may prevent subjects with low SR from learning from errors and punishments and thus learning better SR skills or tendencies. Additionally, impaired coping in emotionally challenging situations, possibly related to prefrontal-cortical functioning, may lead to maladaptive avoidance. Moreover, maladaptive behaviors may be reinforced by the temporary decreases in negative affect and rewarding values of behaviors. Given existing knowledge gaps, we call for more extensive research and describe possible directions and challenges for future studies.
Introduction
Self-regulation (SR) is an important human ability or tendency, with the former relating more to cognitive functioning and the latter more to behavioral traits. Levels of SR differ across individuals and correlate with quality of life in many domains including mental health (1). Thus, the topic is gaining attention among researchers in different fields of science. Previous studies have found multiple correlates of low SR including impulsivity and poor executive functioning and emotional regulation (2). Nonetheless, an incomplete understanding of underpinnings of low SR persists. Here we explore the notion that impaired learning from errors and punishments may underlie poor SR, and maladaptive avoidance may result from and perpetuate low SR.
People make many decisions regarding their actions, some of which are perceived at the time or later to be in error and may lead to negative consequences. For most people, such negative consequences serve as punishments and lead to learning and acting differently in similar future situations. However, people with low SR may frequently engage in maladaptive behaviors (e.g., substance abuse, procrastination, binge eating, gambling, etc.) despite negative consequences. Such data suggest that these individuals may have impaired mechanisms of learning from failures and punishments.
The topic of punishment and avoidance learning has arguably been receiving more attention recently (3, 4). While some human studies have focused on avoidance in relation to anxiety disorders (5) and relatively few experimental papers have investigated learning from punishments and/or avoidance learning in relation to broadly understood SR, some data suggest that subject groups low in SR may learn less from errors and punishment (6). These studies complement a more extensive behavioral and neuroimaging research in SR, impulsivity, substance use disorders and behavioral addictions (2, 7–12).
In the following sections, we first review studies suggesting error-processing may be impaired in a range of individuals with low SR. Next, we summarize data relating impaired error-processing to less effective learning from punishments. We then describe studies demonstrating impaired learning from errors and punishment in SR-related disorders and behaviors (in addictive disorders and procrastination). We also consider a role for poor emotional regulation and suggest that short-term avoidance of negative emotions may be a mechanism motivating people to engage in the maladaptive behaviors that may result in long-term negative consequences. Based on striatal and prefrontal systems, we propose a transdiagnostic model connecting impaired coping and learning from errors and punishments with tendencies to engage in maladaptive behaviors. Finally, we describe the need for more extensive research and suggest some possible future directions.
What Is Self-Regulation?
Following (2), we consider SR in a broad sense as, “the intrinsic processes aimed at adjusting mental and physiological state adaptively to context.” SR “encompasses cognitive control, emotion regulation, and top-down and bottom-up processes that alter emotion, behavior, or cognition to attempt to enhance adaptation (…) strategic/deliberative as well as reactive/automatized processes and their reciprocal influences.” This broad definition encompasses also self-control defined as “top-down aspects of SR” (2). From a clinical perspective, individuals with low SR often engage in maladaptive behaviors like substance abuse, problematic gambling, procrastination, excessive gaming, binge eating and other behaviors, and they will be a focus of the present considerations.
While punishment, negative motivation and negative affect may have different underpinnings and associations, they may also share features relevant to SR. As such, we will consider these processes generally, while noting some unique psychological and neurobiological underpinnings.
Error Processing
Error processing is often measured using specific tasks (e.g., Go/No-go, stop-signal or flanker) and assessment of error-related brain activity often employs EEG or fMRI approaches. Commission of an error results in activation of a network of brain regions including the anterior cingulate cortex (ACC); see (13) for a meta-analysis. Multiple studies of substance additions (involving cannabis, opioid, cocaine, or tobacco use) and behavioral addictions (involving gambling or gaming) suggest diminished error-related ACC activity measured with fMRI or as lower amplitude of error-related negativity (ERN) in EEG studies; see (14) for a meta-analysis. Interestingly, however, in people with alcohol dependence, increased ERN was found, possibly in relation to higher anxiety (15). Diminished error-related brain activity has also been found in people with criminal recidivism (16), procrastination (17, 18), and high impulsivity (19). These results suggest impaired error processing may link transdiagnostically to multiple groups with low SR.
Measurement of startle reactions after errors and correct trials suggests that errors are aversive (20). Amplitude of the ERN component related to degree of startle, consistent with a recent study in which assessments of error sensitivity (i.e., the fear of making mistakes) was correlated with ERN measures in children (21). These results suggest that errors may be less salient and arousing in individuals with lower ERNs. A study of impulsivity employing a flanker task with separated reward and punishment conditions found impulsive subjects to exhibit particularly low ERN components in punishment trials (22). Together these results suggest monitoring difficulties, especially in punishment contexts, and/or impaired processing of aversive values of errors and punishments in individuals with low SR.
Noteworthy errors can also be interpreted as conflicts between the assumed goals and the obtained situations [e.g., (23)], and conflicts have also been proposed to represent aversive signals (24). In light of the theory of expected value of control (25), the activity of ACC may be interpreted as a more general signal regulating cognitive control in demanding situations. This could suggest that individuals characterized with lower error-related ACC activity could also show cognitive control deficits in other situations. A recent review (26) has noted correlations between neural correlates of error processing (ERN amplitudes) and multiple measures of cognitive control. The measures, however, did not assess performance related to learning from errors. Taking the above-mentioned results together, one may hypothesize that lower ERNs and lower SR may concurrently relate to impaired learning from errors and punishments.
Learning From Errors and Punishments
Individual differences exist relating to tendencies to learn from rewards or punishments. A probabilistic cognitive reinforcement learning task used in one study (27) had two stages: learning and inference. In the learning phase, participants were presented with three pairs of stimuli (AB, CD, EF, one pair at a time), were to choose one of the stimuli from a pair, and were provided with feedback. Choosing A resulted in reward in 80% of trials and in punishment with 20% probability, while stimulus B had the opposite contingency. In the CD pair, the probabilities were 70 and 30%, and in the EF pair they were 60 and 40%. During the learning phase, participants learned to choose A over B; however, to check whether they had learned to pursue rewards (choose A) or to avoid punishments (avoid B), a second phase of the task was conducted. During the inference phase, participants again were to choose one of two stimuli, but the stimuli were mixed in different pairs (i.e., AC, AD, AE, AF and BC, BD, BE, BF) and no feedback was provided. Analysis of performance from the inference stage provides insight into whether participants show biases toward learning from rewards (more frequent choices of A) or punishments (more frequent avoidance of B). The authors recorded EEG during the task and found that learning from punishments correlated with ERN amplitudes. Unfortunately, no self-regulation-related questionnaires were employed in the study. However, together with information presented above, the results suggest that subjects with low SR, and thus low ERNs, would show decreased learning from punishments. Several recent studies support this possibility.
An fMRI study employing a spatial paired-associate learning task suggests impaired learning from errors in people who use cannabis (28). In this study, participants were instructed to remember and recall associations of numbers with spatial locations on the screen. After a first round of recall, subjects were presented with the correct numbers, and, if they were unsuccessful, they could improve their performance in a second round of recall. The proportion of corrected errors was significantly lower in individuals who used cannabis vs. those who did not. Neuroimaging results revealed significantly lower error-related brain activity in several regions including the ACC in individuals who used cannabis. Moreover, the ACC was implicated in a group-by-error-type interaction (group: cannabis use vs. non-use; error type: corrected vs. repeated). The interaction suggested higher ACC activity in the non-using group during processing of errors that were later corrected. These results suggest impaired error processing and learning from errors in relation to cannabis use, although disentangling whether impaired learning from errors could have led to cannabis abuse or vice versa would require further studies.
A monetary version of this task, allowing manipulation of the quantity of the monetary outcomes and to separate rewards and punishments, was used to investigate cigarette smoking (29). The study revealed that non-smoking subjects learned better than smoking subjects from small rewards and large punishments. fMRI results showed no error-related group differences in the ACC. The smoking group, however, showed higher activity of the right dorsolateral prefrontal cortex (DLPFC) during recall and during re-encoding of errors corrected in the second round. The authors noted that a “greater need for attentional control, or reduced efficiency in translating DLPFC activation into attentional control” may be evident in individuals who smoke cigarettes.
Impaired learning from punishments has been suggested in opioid addiction during an acquired equivalence task (30). During the first phase of the experiment, subjects were to learn by trial and error associations between antecedent stimuli and consequences. Importantly, learning of associations of two of four antecedent stimuli was reward-based (positive feedback and points gained for correct choices and no feedback for incorrect choices) and learning of the other two was punishment-based (no feedback for correct choices and negative feedback and points lost for incorrect choices). Relative to healthy comparison subjects, opioid-addicted participants needed more trials and committed more errors to reach a desired outcome while learning from punishments. However, no significant group difference was found in reward-based learning. The authors concluded that a “selective deficit in learning from punishment could contribute to processes by which addicted individuals continue to pursue drug use even at the cost of negative consequences.”
We have conducted a behavioral study where students high and low in procrastination performed probabilistic reversal learning tasks with separate reward and punishment conditions (31). During the reward condition, participants were to repeatedly choose between two stimuli, where one had higher (75%) and the other had lower (25%) probabilities of monetary reward. Participants were instructed to maximize gains. From time to time, the stimulus-reward contingencies reversed (the previously better stimulus became worse and vice versa). In the punishment condition, the situation was analogous, and participants were instructed to minimize losses. Analysis was based on the Rescorla-Wagner model (32) as applied to human data (33). The model permits calculation of learning rates (i.e., rates of change of conviction about stimuli-reward contingencies) and exploitation-exploration balance of each participant in each condition (reward/punishment). The analysis revealed significantly lower learning rates in students high in procrastination regardless of condition. This group also demonstrated less exploration (or more persistence). A group-by-condition interaction indicated greater persistence in highly procrastinating subjects during the punishment condition. These results suggest that individuals high in procrastination are less flexible in learning than those low in procrastination, especially in punishment contexts.
Taken together, the above-mentioned results suggest impaired learning from errors and punishments across diagnostic boundaries in individuals low in SR.
Maladaptive Avoidance and Impaired Emotional Regulation
The experiments described in the previous section confront subjects with choices and do not allow for or assess avoidance. Employing different experimental paradigms could assess avoidance behaviors that may better resemble real-life situations. Relatively few such studies have been conducted, with several described below.
In one study of heroin-dependent and non-dependent control subjects, participants were asked to perform an escape-avoidance task in the form of a computer game in which they controlled a spaceship with the goal of earning points by shooting enemy spaceships (34). During the first twelve “acquisition trials,” a warning signal announcing a bomb coming was periodically displayed for 5 s. Appearance of a bomb resulted in a reduction of points unless subjects hid their spaceship in a “safe box.” During the next twelve “extinction trials,” no bomb appeared after the warning signal. Women performed significantly worse on the task, and group differences were found only in male subjects. Heroin-dependent males scored significantly fewer points than control subjects because they spent significantly more time hiding in the safe box during the warning signal and a good while after the “bomb period,” during both acquisition and extinction trials. This study suggests that heroin-addicted vs. non-addicted males present exaggerated avoidance behavior that may result in reduced opportunities to obtain rewards.
A study employing the same task in alcohol-dependent and healthy men (35) led to similar and different results. Alcohol-dependent men escaped the bomb situation more often than healthy men. They also tended to spend more time hiding during the warning signal in the acquisition but not in the extinction trials. However, alcohol-dependent men scored more points in total as they shot significantly more enemy spaceships than healthy control men, especially during the extinction phase. The authors interpreted the results as, “supporting the idea that both positive and negative reinforcement are important components underlying addictive behaviors.”
Maladaptive avoiding may represent an important consideration in addictions. We use the term maladaptive avoidance as reflecting managing stressful situations by not addressing them directly, but by averting attention from them (c.f. “avoidance coping” in APA Dictionary) (36), and this may in turn eventually lead to negative consequences including more stress and negative emotions. Whether behaviors observed in the above-mentioned experimental studies are suitably modeling real-life maladaptive avoidance may be subject to interpretation and further investigation; however, a role for maladaptive avoidance in individuals characterized by poor SR (including those with addictions) was proposed decades ago (37, 38). In related models, engaging in maladapitve or addictive behaviors (e.g., substance abuse, gambling, proctrastination, binge eating) may serve as a short-term emotion-regulation strategy aimed at avoiding or alleviating negative affect [consistent with negative reinforcement motivations and self-medication models of addictions (39), among others (8, 40)]. Previous studies using self-report measures support the notion of engaging in potentially addictive behaviors like substance use (41), gambling (42), procrastination (43), and internet use and gaming (44, 45) for avoiding or alleviating negative mood states.
The described preference to obtain short-term rewards or relief over avoiding possibly larger long-term punishments in people with low SR may also may be interpreted in terms of steep delay discounting or a tendency to value immediate future events higher than more distant ones. Indeed, a recent meta-analysis of delay discounting in addictive behaviors found a small but highly significant correlation (46). Interestingly, delay discounting was correlated with procrastination when measured with questionnaires (47, 48), but not when measured with tasks (49). Most previous studies focused however on delayed reward discounting, and did not include punishments. Future studies should therefore examine delayed punishment discounting, and also consider whether there may be causal relationships between delay discounting and maladaptive avoidance.
The above-mentioned results suggest that maladaptive avoidance may represent a mechanism involved in disorders characterized by low SR. Maladaptive avoidance tendencies may reflect strategies developed to compensate for poor coping in response to challenging situations (for example, to decrease stress when exams approach, students could play computer games instead of studying). Alternatively, both maladaptive avoidance and impaired learning from errors and punishments may indicate impaired cognitive and behavioral control during emotionally challenging situations in which negative emotions and/or threat of punishment are experienced or anticipated.
Discussion and Possible Mechanisms
SR failures related to negative affect may result from imbalances between subcortical and frontal regions; e.g., insufficient frontal top-down control “either due to particularly strong impulses or when prefrontal function itself is impaired” (50). Indeed, both lack of reward and receipt of punishment have led to activation of the right DLPFC in healthy subjects (51). These findings suggest increased behavioral control in such situations. According to the theory of expected value of control (25), cognitive control is implemented by the lateral PFC in response to ACC signaling a potential need for it. Therefore, it may be predicted that activity of these prefrontal brain regions related to punishment or negative affect could differ in individuals high and low in SR. Indeed, abstinent cocaine-dependent participants showed hypoactivity of the ACC and right DLPFC in relation to punished errors in the Go/No-go task (52). We have found similar results (diminished ACC and right DLPFC activity during punishment condition in the Go/No-go task) in individuals high in procrastination (17). These findings are in line with prior theories (50) and suggest that impairment of prefrontal control by punishment threat or negative emotions may operate for many subject groups characterized by low SR. Note, however, that the previously mentioned study (29) showed higher DLPFC activity in tobacco-smoking individuals during re-encoding of later-corrected errors, possibly reflecting higher difficulty and/or effort needed to correctly perform in the task, or impaired mechanisms of regulation of intensity of implemented control [c.f. (25)].
Prefrontal-striatal mechanisms linked to SR may be amenable to interventions, with transdiagnostic implications. Both regulation of emotion and regulation of motivation (e.g., craving in addictive disorders) have been implicated in groups characterized by low SR. Among individuals who smoke tobacco, regulation of craving has been found to involve prefrontal control over striatal cue responsiveness (53). Prefrontal regions (PFC, ACC) and circuitry (a fronto-cingulo-parietal network) were less activated and engaged, respectively, in individuals with internet gaming disorder vs. those without during emotional regulation (54). Recently, both impaired regulation over responses to primary rewards and addictive cues have been reported in internet gaming disorder (55), and stimulation of the right DLPFC enhanced both regulation of craving and negative emotions in individuals with internet gaming disorder (56). Taken together and in line with prior theories of internet gaming disorder (57) and other addictive behaviors (8, 58), these findings suggest that interventions targeting increased prefrontal control over subcortical emotions/motivations may help multiple groups with low SR.
Concerning the balance between subcortical and frontal regions, dysregulation of dopaminergic circuits may be one mechanism underpinning impaired reward, punishment, and error processing, and thus underlie impulsivity, addictions and possibly low SR in general (59–62), although dopamine may play a more central role in some disorders than in others (63–65). At least two non-exclusive dopamine-related mechanisms may underlie aspects of low SR.
First, a “No-Go” pathway could be deficiently functioning in low SR as has been proposed as one element in some models of addiction (66) and could reflect higher striatal and lower prefrontal activity. However, addictive behaviors have also been linked to lower striatal activation [e.g., in reward anticipation in substance use and gambling disorders (67), with similar findings in binge eating disorder (68, 69) and internet gaming disorder (70)]. In several of these studies, blunted striatal activation was linked to increased impulsivity (71–73), suggesting a relationship to SR. These findings are in line with reward deficiency models of addiction (74) and suggest more complex etiologies relating to SR in addictive disorders. Blunted ventral striatal activation during a prospect phase of reward and loss processing has been linked to disadvantageous decision-making in people with and without gambling disorder, suggesting another route by which reward and loss processing may link to SR impairment (75). Although some models suggest that striatal dopamine may underlie aspects of human addictions (76), findings may be strongest for stimulant use disorders (63), and even in such disorders, dopamine receptor availability may show differential relationships with measures of disease severity (77).
When considering the involvement of the striatum in SR, one should be mindful of complexities involving regions of the striatum and how they may relate to SR, including changes within individuals over time both developmentally and from life experiences including psychopathology (78, 79). For example, data suggest that the ventral striatum may be more linked to impulsive behaviors and dorsal striatum to more habitual behaviors, with these regions components of different parallel cortico-striato-pallidal-thalamo-cortical circuits that are involved in different stages of disorders (e.g., addictions, impulse control disorders) characterized by impairments in SR (79–84). Given these data, a shift from more ventral to dorsal striatal involvement has been proposed for addictions as they become more instantiated (84). More recently, a study of healthy adults performing a naturalistic maze-navigation task identified functionally segregated regions of the ventral striatum that separately encode specific aspects of performance [effort activation, movement initiation and effort discounting of rewards (85)]. Furthermore, opposing patterns of activation related to effort activation and discounting were associated with striatal encoding of effort during effort-based decision-making. The authors suggested that the dorsomedial region of the striatum that has been previously understood as being linked to action may rather be involved in assessing cost of effort, raising questions regarding prior interpretations of striatal “reward” signals. Taken together, the findings indicate that more research is needed to examine involvement of specific striatal regions and circuits in studies of the neurochemical and neurocircuitry underpinnings of SR. Furthermore, additional circuitry should be considered, consistent with recent models of addiction (8, 40).
Second, considering the diminished error-related ACC activity, it could be hypothesized that the errors are less salient in low SR individuals and thus impede learning on errors. Early data (86) suggested that error-related ACC activity influenced learning not to repeat erroneous behaviors, and this may in part reflect dopamine-dependent reinforcement signals from the basal ganglia. This view was supported by data from patients with Parkinson's disease participating in a probabilistic reinforcement learning task (87) similar to the one described above (27). Unmedicated Parkinson's patients, considered characterized by low dopamine, learned better based on punishments than on rewards; application of dopamine-replacement medications reversed this pattern, suggesting that lower levels of dopamine may support D2-like-receptor-dependent “No-Go” learning based on errors and punishments, while high levels of dopamine may shift the balance toward D1-like-receptor-related “Go” behaviors (87). This interpretation is consistent with results of correlations between ERN amplitudes and learning from punishments in healthy subjects (27). This leads to speculation that people with lower ERNs and lower tendencies to learn from punishments may be characterized by higher levels of striatal dopamine, consistent with findings suggesting positive correlation between impulsivity and striatal dopamine measures (88, 89). Buckholtz and colleagues suggested that high levels of striatal dopamine in impulsive subjects may result from lower levels of D2/D3 autoreceptors in the substantia nigra and ventral tegmental area leading to stronger dopamine cell activity and enhanced release of dopamine which could in turn increase impulsivity and promote “Go” behaviors (88). However, given inconsistent findings and complications of synthesizing information across studies [e.g., from Parkinson and non-Parkinson populations that may show important dopamine-related differences given the pathophysiology of the disorder and other factors (90, 91)], additional research is necessary.
Summing up, in emotionally challenging situations prefrontal activity in individuals with low SR may be insufficient to effectively regulate emotional and motivational drives involving the striatum and other regions, and this may lead to difficulties in coping with negative situations. Additionally, individuals with low SR may not learn from errors and punishments, and this may relate to differences or impediments in error processing. Together, these mechanisms may impede coping in negative situations, hinder learning from such situations and impair learning SR (Figure 1).
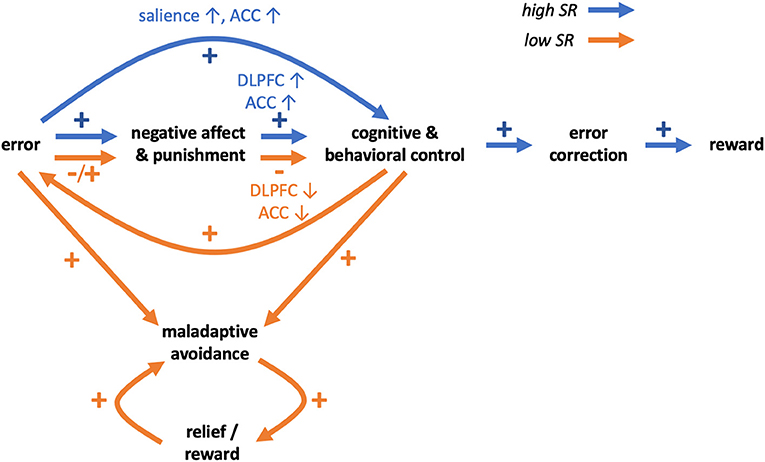
Figure 1. Schematic hypothetical transdiagnostic model of self-regulation behaviors and disorders in relation to impaired coping and learning from errors and punishments. Differences between high SR (blue arrows) and low SR (orange arrows) individuals may involve brain reactions to negative affect and punishments. In individuals high in SR, negative affect and punishments may lead to increased activity of prefrontal control regions (ACC, DLPFC) and increased cognitive and behavioral control. In individuals low in SR, ACC and DLPFC activity in emotionally challenging situations may be relatively lower and result in impairment of cognitive and behavioral control. In individuals high in SR, errors may be processed as more salient events and lead to increased monitoring and control, supporting correction of errors in the future, leading to more rewards and learning of self-regulation. In individuals low in SR, learning from errors and punishments may be impaired. Errors may be processed as less salient and aversive events and not result in increased monitoring and control, potentially leading to repeating of errors. Eventually however errors may result in punishments and negative affect and further decline of cognitive and behavioral control. Both because of error-processing and diminished control, individuals low in SR may preferentially engage in maladaptive behaviors. The behavioral pattern may be reinforced by the relief and rewarding value of substitute behaviors, eventually preventing individuals low in SR from learning self-regulation.
Such maladaptive behaviors may be reinforced over time. In an fMRI study employing a probabilistic reinforcement learning task with separated rewards and punishments (51), the authors showed that both obtaining rewards and avoiding punishments lead to activation of the medial orbitofrontal cortex (mOFC). Given that the mOFC is a component of the ventromedial PFC that has been implicated in processing rewarding outcomes (92), the findings suggest that avoidance of aversive outcome may be rewarding. Similar conclusions were made in a different study employing a repeated acquisition approach-avoidance learning task and showed, among other regions, activations in anterior and posterior cingulate and ventral striatum both for rewards and avoidance of aversive outcomes (93). These studies showed that reward and avoidance learning rely largely on common brain networks, consistent with meta-analytic findings (94). Therefore, negative reinforcement (i.e., avoidance of punishment) may lead to consolidation of avoidance behaviors including maladaptive avoidance. Moreover, engaging in maladapitve behaviors (e.g., substance abuse, gambling, gaming) may be rewarding in and of themselves and thus additionally reinforce such behavioral patterns (Figure 1).
Summary and Future Directions
In this manuscript, we discuss possible transdiagnostic mechanisms underlying poor SR. Impaired coping in emotionally challenging situations, related to decreased prefrontal activity, may promote avoidance of such situations in individuals with low SR. Moreover, impaired error processing may prevent individuals with low SR from learning from errors and punishments and impede effective correction of faulty behaviors and learning of SR itself. Additionally, maladaptive behaviors may be reinforced by short-term decreases in negative affect and by rewarding values of behaviors, leading to more persistent engagement (Figure 1).
Whether the model is valid and is transdiagnostic should be a subject of future studies, which may involve several challenges. First, regarding learning from errors and punishments, future studies may benefit from novel tasks (ideally applicable in neuroimaging studies) as many probabilistic reinforcement learning tasks often do not show between-group differences in task performance and require modeling approaches to show differences in learning strategies. Second, new experimental tasks, better mimicking real-life situations, should be developed to address learning of avoidance. Ideally, such tasks should allow participants a choice to avoid engagement in aspects of the task. They should also allow for distinguishing between learning of adaptive and maladaptive avoidance. Such human avoidance research tasks may encounter challenges that should be considered (95). Future studies should also check whether punishments, punishing contexts and negative affects evoked in different ways may lead to similar or different neuronal and behavioral effects. Future research could also refer to the theory of expected value of control and test which of the proposed components of cognitive control may be particularly impaired in individuals low in SR: estimation of expected outcomes, regulation of intensity of control, or monitoring of performance (25). Ideally, future research will address proposed mechanisms in a broad range of disorders characterized by low SR including substance use disorders, behavioral addictions, binge eating disorder, and other conditions, as well as in other relevant behavioral dimensions including procrastination and high impulsivity.
We believe that research addressing learning from negative consequences and coping in negative situations in disorders characterized by low SR may eventually contribute to the improvement of existing and/or development of new therapeutic approaches. Psychological interventions could possibly address emotional regulation, working with internal conflicts and/or training inhibitory control and error-awareness. Pharmacological therapies could aim at the regulation of function of neurochemical systems underlying motivational drives and cognitive control. Neuromodulatory approaches may be well-suited to increase prefrontal control over subcortical drives, particularly given the ability to target prefrontal cortical regions using transcranial direct current stimulation and repetitive transcranial magnetic stimulation.
Limitations
Our proposed model should be considered cautiously. The understanding of individual differences, especially concerning SR, in processes underlying learning based on errors and punishment and avoidance learning is still developing; therefore, the model may currently be considered speculative. Existing data do not typically permit dissection of causes from consequences (e.g., of substance abuse); nevertheless, we believe the model is relevant to SR impairments. In considering a transdiagnostic mechanism underlying low SR, we considered together many disorders and behaviors characterized by low SR. In doing so, the model does not incorporate all mechanisms that may contribute to low SR, for example delay discounting or motivational processes, neither does it include factors relating, for example, to effects of specific substances. Noteworthy, we relate the results of the reported studies to learning of SR, repetitive engagement in maladaptive behaviors, and their long-term negative consequences, while the studies investigated almost exclusively short-term processes. Thus, it is imaginable that the experimental situations do not fully reflect real-life processes, especially with respect to preferring short-term rewards over avoiding of possibly larger long-term punishments, and further research in this area would likely improve understanding of mechanisms and help to refine the model. We also simplified and limited brain considerations largely to the striatum and PFC, and we largely did not discuss roles for particular subregions and other parts of the brain. We also did not discuss the broader range of neurochemical/neurotransmitter systems that may contribute to SR. However, we believe that these simplifications permitted proposing a model for how impaired coping under negative affect and impaired learning from errors and punishments may operate as transdiagnostic mechanisms underlying poor SR. We hope that future studies will test the proposed model, lead to the development of more detailed models and facilitate clinical advances related to poor SR.
Data Availability Statement
The original contributions presented in the study are included in the article/supplementary material, further inquiries can be directed to the corresponding author/s.
Author Contributions
MW conceptualized the manuscript. Both authors reviewed literature, wrote, and approved the submitted version of the manuscript.
Funding
MW was supported by Polish National Science Center Grant No. 2014/13/D/HS6/03015. MP was supported by NIDA grant R01 DA039136 and the Connecticut Council on Problem Gambling.
Conflict of Interest
MP has consulted for and advised Game Day Data, the Addiction Policy Forum, AXA, Idorsia, and Opiant/Lakelight Therapeutics; received research support from the Veteran's Administration, Mohegan Sun Casino, and the National Center for Responsible Gaming (no the International Center for Responsible Gambling); participated in surveys, mailings, or telephone consultations related to drug addiction, impulse-control disorders or other health topics; consulted for law offices and the federal public defender's office in issues related to impulse-control and addictive disorders; provided clinical care in the Connecticut Department of Mental Health and Addiction Services Problem Gambling Services Program; performed grant reviews for the National Institutes of Health and other agencies; edited journals and journal sections; given academic lectures in grand rounds, CME events and other clinical/scientific venues; and generated books or chapters for publishers of mental health texts. The views presented in this manuscript represent those of the authors.
The remaining author declares that the research was conducted in the absence of any commercial or financial relationships that could be construed as a potential conflict of interest.
References
1. Tangney JP, Baumeister RF, Boone AL. High self-control predicts good adjustment, less pathology, better grades, and interpersonal success. J Pers. (2004) 72:271–324. doi: 10.1111/j.0022-3506.2004.00263.x
2. Nigg JT. Annual research review: on the relations among self-regulation, self-control, executive functioning, effortful control, cognitive control, impulsivity, risk-taking, and inhibition for developmental psychopathology. J Child Psychol Psychiatry All Discipl. (2017) 58:361–83. doi: 10.1111/jcpp.12675
3. Krypotos AM, Effting M, Kindt M, Beckers T. Avoidance learning: a review of theoretical models and recent developments. Front Behav Neurosci. (2015) 9:189. doi: 10.3389/fnbeh.2015.00189
4. LeDoux JE, Moscarello J, Sears R, Campese V. The birth, death and resurrection of avoidance: a reconceptualization of a troubled paradigm. Mol Psychiatry. (2017) 22:24–36. doi: 10.1038/mp.2016.166
5. Hofmann SG, Hay AC. Rethinking avoidance: toward a balanced approach to avoidance in treating anxiety disorders. J Anxiety Disord. (2018) 55:14–21. doi: 10.1016/j.janxdis.2018.03.004
6. Poulton A, Hester R. Transition to substance use disorders: impulsivity for reward and learning from reward. Soc Cogn Affect Neurosci. (2019) 15:1182–91. doi: 10.1093/scan/nsz077
7. Alvarez-Monjaras M, Mayes LC, Potenza MN, Rutherford HJV. A developmental model of addictions: integrating neurobiological and psychodynamic theories through the lens of attachment. Attach Hum Dev. (2019) 21:616–37. doi: 10.1080/14616734.2018.1498113
8. Brand M, Wegmann E, Stark R, Müller A, Wölfling K, Robbins TW, et al. The Interaction of Person-Affect-Cognition-Execution (I-PACE) model for addictive behaviors: update, generalization to addictive behaviors beyond internet-use disorders, and specification of the process character of addictive behaviors. Neurosci Biobehav Rev. (2019) 104:1–10. doi: 10.1016/j.neubiorev.2019.06.032
9. Fineberg NA, Chamberlain SR, Goudriaan AE, Stein DJ, Vanderschuren LJMJ, Gillan CM, et al. New developments in human neurocognition: clinical, genetic, and brain imaging correlates of impulsivity and compulsivity. CNS Spectr. (2014) 19:69–89. doi: 10.1017/S1092852913000801
10. Kuss DJ, Pontes HM, Griffiths MD. Neurobiological correlates in internet gaming disorder: a systematic literature review. Front Psychiatry. (2018) 9:166. doi: 10.3389/fpsyt.2018.00166
11. Volkow ND, Hampson AJ, Baler RD. Don't worry, be happy: endocannabinoids and cannabis at the intersection of stress and reward. Annu Rev Pharmacol Toxicol. (2017) 57:285–308. doi: 10.1146/annurev-pharmtox-010716-104615
12. Volkow ND, Wise RA, Baler R. The dopamine motive system: implications for drug and food addiction. Nat Rev Neurosci. (2017) 18:741–52. doi: 10.1038/nrn.2017.130
13. Klein TA, Endrass T, Kathmann N, Neumann J, von Cramon DY, Ullsperger M. Neural correlates of error awareness. Neuroimage. (2007) 34:1774–81. doi: 10.1016/j.neuroimage.2006.11.014
14. Luijten M, Machielsen MWJ, Veltman DJ, Hester R, de Haan L, Franken IHA. Systematic review of ERP and fMRI studies investigating inhibitory control and error processing in people with substance dependence and behavioural addictions. J Psychiatry Neurosci. (2014) 39:149–69. doi: 10.1503/jpn.130052
15. Schellekens AFA, De Bruijn ERA, Van Lankveld CAA, Hulstijn W, Buitelaar JK, De Jong CAJ, et al. Alcohol dependence and anxiety increase error-related brain activity. Addiction. (2010) 105:1928–34. doi: 10.1111/j.1360-0443.2010.03065.x
16. Aharoni E, Vincent GM, Harenski CL, Calhoun VD, Sinnott-Armstrong W, Gazzaniga MS, et al. Neuroprediction of future rearrest. Proc Natl Acad Sci USA. (2013) 110:6223–8. doi: 10.1073/pnas.1219302110
17. Wypych M, Michałowski JM, Droździel D, Borczykowska M, Szczepanik M, Marchewka A. Attenuated brain activity during error processing and punishment anticipation in procrastination – a monetary Go/No-go fMRI study. Sci Rep. (2019) 9:11492. doi: 10.1038/s41598-019-48008-4
18. Michałowski JM, Wiwatowska E, Weymar M. Brain potentials reveal reduced attention and error-processing during a monetary Go/No-Go task in procrastination. Sci Rep. (2020) 10:19678. doi: 10.1038/s41598-020-75311-2
19. Ruchsow M, Spitzer M, Grön G, Grothe J, Kiefer M. Error processing and impulsiveness in normals: evidence from event-related potentials. Cogn Brain Res. (2005) 24:317–25. doi: 10.1016/j.cogbrainres.2005.02.003
20. Hajcak G, Foti D. Errors are aversive. Psychol Sci. (2008) 19:103–8. doi: 10.1111/j.1467-9280.2008.02053.x
21. Chong LJ, Meyer A. Understanding the link between anxiety and a neural marker of anxiety (the error-related negativity) in 5 to 7 year-old children. Dev Neuropsychol. (2019) 44:71–87. doi: 10.1080/87565641.2018.1528264
22. Potts GF, George MRM, Martin LE, Barratt ES. Reduced punishment sensitivity in neural systems of behavior monitoring in impulsive individuals. Neurosci Lett. (2006) 397:130–4. doi: 10.1016/j.neulet.2005.12.003
23. Botvinick MM, Cohen JD, Carter CS. Conflict monitoring and anterior cingulate cortex: an update. Trends Cogn Sci. (2004) 8:539–46. doi: 10.1016/j.tics.2004.10.003
24. Dreisbach G, Fischer R. Conflicts as aversive signals. Brain Cogn. (2012) 78:94–8. doi: 10.1016/j.bandc.2011.12.003
25. Shenhav A, Botvinick MM, Cohen JD. The expected value of control: an integrative theory of anterior cingulate cortex function. Neuron. (2013) 79:217–40. doi: 10.1016/j.neuron.2013.07.007
26. Meyer A, Hajcak G. A review examining the relationship between individual differences in the error-related negativity and cognitive control. Int J Psychophysiol. (2019) 144:7–13. doi: 10.1016/j.ijpsycho.2019.07.005
27. Frank MJ, Woroch BS, Curran T. Error-related negativity predicts reinforcement learning and conflict biases. Neuron. (2005) 47:495–501. doi: 10.1016/j.neuron.2005.06.020
28. Carey SE, Nestor L, Jones J, Garavan H, Hester R. Impaired learning from errors in cannabis users: dorsal anterior cingulate cortex and hippocampus hypoactivity. Drug Alcohol Depend. (2015) 155:175–82. doi: 10.1016/j.drugalcdep.2015.07.671
29. Duehlmeyer L, Hester R. Impaired learning from punishment of errors in smokers: differences in dorsolateral prefrontal cortex and sensorimotor cortex blood-oxygen-level dependent responses. Neuroimage Clin. (2019) 23:101819. doi: 10.1016/j.nicl.2019.101819
30. Myers CE, Rego J, Haber P, Morley K, Beck KD, Hogarth L, et al. Learning and generalization from reward and punishment in opioid addiction. Behav Brain Res. (2017) 317:122–31. doi: 10.1016/j.bbr.2016.09.033
31. Przetacka J, Droździel D, Michałowski JM, Wypych M. Self-regulation and learning from failures: probabilistic reversal learning task reveals impaired learning on errors in procrastinators. (submitted and under review).
32. Rescorla AR, Wagner RA. A theory of classical conditioning: variations in the effectiveness of reinforcement and nonreinforcement. Class Condit II Curr Res Theory. (1972) 21:64–99.
33. Gläscher J, Hampton AN, O'Doherty JP. Determining a role for ventromedial prefrontal cortex in encoding action-based value signals during reward-related decision making. Cerebral Cortex. (2009) 19:483–95. doi: 10.1093/cercor/bhn098
34. Sheynin J, Moustafa AA, Beck KD, Servatius RJ, Casbolt PA, Haber P, et al. Exaggerated acquisition and resistance to extinction of avoidance behavior in treated heroin-dependent males. J Clin Psychiatry. (2016) 77:386–94. doi: 10.4088/JCP.14m09284
35. Sheynin J, Myers CE, Ghafar F, Morris AN, Morley KC, Haber PS, et al. A pilot study of escape, avoidance, and approach behaviors in treated alcohol-dependent males. J Clin Exp Neuropsychol. (2019) 41:601–14. doi: 10.1080/13803395.2019.1595530
36. American Psychological Association. APA Dictionary of Psychology. Available online at: https://dictionary.apa.org/avoidance-coping
37. Baker TB, Piper ME, Mccarthy DE, Majeskie MR, Fiore MC. Addiction motivation reformulated: an affective processing model of negative reinforcement. Psychol Rev. (2004) 111:33–51. doi: 10.1037/0033-295X.111.1.33
38. Tice DM, Bratslavsky E. Giving in to feel good: the place of emotion regulation in the context of general self-control. Psychol Inq. (2000) 11:149–59. doi: 10.1207/S15327965PLI1103_03
39. Khantzian EJ. The self-medication hypothesis of addictive disorders: focus on heroin and cocaine dependence. Cocaine Crisis. (1987) 142:65–74. doi: 10.1007/978-1-4613-1837-8_7
40. Koob GF, Volkow ND. Neurobiology of addiction: a neurocircuitry analysis. Lancet Psychiatry. (2016) 3:760–73. doi: 10.1016/S2215-0366(16)00104-8
41. Forsyth JP, Parker JD, Finlay CG. Anxiety sensitivity, controllability, and experiential avoidance and their relation to drug of choice and addiction severity in a residential sample of substance-abusing veterans. Addict Behav. (2003) 28:851–70. doi: 10.1016/S0306-4603(02)00216-2
42. Thomas AC, Allen FL, Phillips J, Karantzas G. Gaming machine addiction: the role of avoidance, accessibility and social support. Psychol Addict Behav. (2011) 25:738–44. doi: 10.1037/a0024865
43. Sirois FM, Pychyl TA. Procrastination and the priority of short-term mood regulation: consequences for future self. Soc Pers Psychol Compass. (2013) 7:115–27. doi: 10.1111/spc3.12011
44. Ang C-S, Chan N-N, Lee C-S. Shyness, loneliness avoidance, and internet addiction: what are the relationships? J Psychol. (2018) 152:25–35. doi: 10.1080/00223980.2017.1399854
45. Laier C, Wegmann E, Brand M. Personality and cognition in gamers: avoidance expectancies mediate the relationship between maladaptive personality traits and symptoms of Internet-gaming disorder. Front Psychiatry. (2018) 9:304. doi: 10.3389/fpsyt.2018.00304
46. Amlung M, Vedelago L, Acker J, Balodis I, MacKillop J. Steep delay discounting and addictive behavior: a meta-analysis of continuous associations. Addiction. (2017) 112:51–62. doi: 10.1111/add.13535
47. Steel P. A diagnostic measure of procrastination. Paper Presented at the 7th Procrastination Research Conference Biennial Meeting, Counseling the Procrastinator in Academic Settings. Amsterdam (2011).
48. Wypych M, Matuszewski J, Dragan WŁ. Roles of impulsivity, motivation, and emotion regulation in procrastination – path analysis and comparison between students and non-students. Front Psychol. (2018) 9:891. doi: 10.3389/fpsyg.2018.00891
49. Sutcliffe KR, Sedley B, Hunt MJ, Macaskill AC. Relationships among academic procrastination, psychological flexibility, and delay discounting. Behav Anal Res Pract. (2019) 19:315–26. doi: 10.1037/bar0000145
50. Heatherton TF, Wagner DD. Cognitive neuroscience of self-regulation failure. Trends Cogn Sci. (2011) 15:132–9. doi: 10.1016/j.tics.2010.12.005
51. Kim H, Shimojo S, O'Doherty JP. Is avoiding an aversive outcome rewarding? Neural substrates of avoidance learning in the human brain. PLoS Biol. (2006) 4:1453–61. doi: 10.1371/journal.pbio.0040233
52. Hester R, Bell RP, Foxe JJ, Garavan H. The influence of monetary punishment on cognitive control in abstinent cocaine-users. Drug Alcohol Depend. (2013) 133:86–93. doi: 10.1016/j.drugalcdep.2013.05.027
53. Kober H, Mende-Siedlecki P, Kross EF, Weber J, Mischel W, Hart CL, et al. Prefrontal-striatal pathway underlies cognitive regulation of craving. Proc Natl Acad Sci USA. (2010) 107:14811–6. doi: 10.1073/pnas.1007779107
54. Yip SW, Gross JJ, Chawla M, Ma SS, Shi XH, Liu L, et al. Is neural processing of negative stimuli altered in addiction independent of drug effects? Findings from drug-naïve youth with internet gaming disorder. Neuropsychopharmacology. (2018) 43:1364–72. doi: 10.1038/npp.2017.283
55. Wu LL, Zhu L, Shi XH, Zhou N, Wang R, Liu G, et al. Impaired regulation of both addiction-related and primary rewards in individuals with internet gaming disorder. Psychiatry Res. (2020) 286. doi: 10.1016/j.psychres.2020.112892
56. Wu LL, Potenza MN, Zhou N, Kober H, Shi XH, Yip SW, et al. A role for the right dorsolateral prefrontal cortex in enhancing regulation of both craving and negative emotions in internet gaming disorder: a randomized trial. Eur Neuropsychopharmacol. (2020) 36:29–37. doi: 10.1016/j.euroneuro.2020.04.003
57. Dong G, Potenza MN. A cognitive-behavioral model of Internet gaming disorder: theoretical underpinnings and clinical implications. J Psychiatr Res. (2014) 58:7–11. doi: 10.1016/j.jpsychires.2014.07.005
58. Brand M, Young KS, Laier C, Wölfling K, Potenza MN. Integrating psychological and neurobiological considerations regarding the development and maintenance of specific Internet-use disorders: an Interaction of Person-Affect-Cognition-Execution (I-PACE) model. Neurosci Biobehav Rev. (2016) 71:252–66. doi: 10.1016/j.neubiorev.2016.08.033
59. Berke JD. What does dopamine mean? Nat Neurosci. (2018) 21:787–93. doi: 10.1038/s41593-018-0152-y
60. Bissonette GB, Gentry RN, Padmala S, Pessoa L, Roesch MR. Impact of appetitive and aversive outcomes on brain responses: linking the animal and human literatures. Front Syst Neurosci. (2014) 8:24. doi: 10.3389/fnsys.2014.00024
61. Bromberg-Martin ES, Matsumoto M, Hikosaka O. Dopamine in motivational control: rewarding, aversive, and alerting. Neuron. (2010) 68:815–34. doi: 10.1016/j.neuron.2010.11.022
62. Hu H. Reward and aversion. Annu Rev Neurosci. (2016) 39:297–324. doi: 10.1146/annurev-neuro-070815-014106
63. Nutt DJ, Lingford-Hughes A, Erritzoe D, Stokes PRA. The dopamine theory of addiction: 40 years of highs and lows. Nat Rev Neurosci. (2015) 16:305–12. doi: 10.1038/nrn3939
64. Potenza MN. How central is dopamine to pathological gambling or gambling disorder? Front Behav Neurosci. (2013) 7:206. doi: 10.3389/fnbeh.2013.00206
65. Potenza MN. Searching for replicable dopamine-related findings in gambling disorder. Biol Psychiatry. (2018) 83:984–6. doi: 10.1016/j.biopsych.2018.04.011
66. Volkow ND, Wang GJ, Fowler JS, Tomasi D, Telang F. Addiction: beyond dopamine reward circuitry. Proc Natl Acad Sci USA. (2011) 108:15037–42. doi: 10.1073/pnas.1010654108
67. Luijten M, Schellekens AF, Kühn S, MacHielse MWJ, Sescousse G. Disruption of reward processing in addiction: an image-based meta-analysis of functional magnetic resonance imaging studies. JAMA Psychiatry. (2017) 74:387–98. doi: 10.1001/jamapsychiatry.2016.3084
68. Balodis IM, Kober H, Worhunsky PD, White MA, Stevens MC, Pearlson GD, et al. Monetary reward processing in obese individuals with and without binge eating disorder. Biol Psychiatry. (2013) 73:877–86. doi: 10.1016/j.biopsych.2013.01.014
69. Balodis IM, Grilo CM, Kober H, Worhunsky PD, White MA, Stevens MC, et al. A pilot study linking reduced fronto-Striatal recruitment during reward processing to persistent bingeing following treatment for binge-eating disorder. Int J Eat Disord. (2014) 47:376–84. doi: 10.1002/eat.22204
70. Dong G, Li H, Wang L, Potenza MN. Cognitive control and reward/loss processing in Internet gaming disorder: results from a comparison with recreational Internet game-users. Eur Psychiatry. (2017) 44:30–8. doi: 10.1016/j.eurpsy.2017.03.004
71. Andrews MM, Meda SA, Thomas AD, Potenza MN, Krystal JH, Worhunsky P, et al. Individuals family history positive for alcoholism show functional magnetic resonance imaging differences in reward sensitivity that are related to impulsivity factors. Biol Psychiatry. (2011) 69:675–83. doi: 10.1016/j.biopsych.2010.09.049
72. Balodis IM, Kober H, Worhunsky PD, Stevens MC, Pearlson GD, Potenza MN. Diminished frontostriatal activity during processing of monetary rewards and losses in pathological gambling. Biol Psychiatry. (2012) 71:749–57. doi: 10.1016/j.biopsych.2012.01.006
73. Beck A, Schlagenhauf F, Wüstenberg T, Hein J, Kienast T, Kahnt T, et al. Ventral striatal activation during reward anticipation correlates with impulsivity in alcoholics. Biol Psychiatry. (2009) 66:734–42. doi: 10.1016/j.biopsych.2009.04.035
74. Blum K, Cull JG, Braverman ER, Comings DE. Reward deficiency syndrome. Am Sci. (1996) 84:132–45.
75. Balodis IM, Linnet J, Arshad F, Worhunsky PD, Stevens MC, Pearlson GD, et al. Relating neural processing of reward and loss prospect to risky decision-making in individuals with and without gambling disorder. Int Gambling Stud. (2018) 18:269–85. doi: 10.1080/14459795.2018.1469658
76. Volkow ND, Wang G-J, Telang F, Fowler JS, Logan J, Jayne M, et al. Profound decreases in dopamine release in striatum in detoxified alcoholics: possible orbitofrontal involvement. J Neurosci. (2007) 27:12700–6. doi: 10.1523/JNEUROSCI.3371-07.2007
77. Worhunsky PD, Matuskey D, Gallezot JD, Gaiser EC, Nabulsi N, Angarita GA, et al. Regional and source-based patterns of [11C]-(+)-PHNO binding potential reveal concurrent alterations in dopamine D2 and D3 receptor availability in cocaine-use disorder. Neuroimage. (2017) 148:343–51. doi: 10.1016/j.neuroimage.2017.01.045
78. Chambers RA, Taylor JR, Potenza MN. Developmental neurocircuitry of motivation in adolescence: a critical period of addiction vulnerability. Am J Psychiatry. (2003) 160:1041–52. doi: 10.1176/appi.ajp.160.6.1041
79. Everitt BJ, Robbins TW. Neural systems of reinforcement for drug addiction: from actions to habits to compulsion. Nat Neurosci. (2005) 8:1481–9. doi: 10.1038/nn1579
80. Yin HH, Knowlton BJ. The role of the basal ganglia in habit formation. Nat Rev Neurosci. (2006) 7:464–76. doi: 10.1038/nrn1919
81. Brewer JA, Potenza MN. The neurobiology and genetics of impulse control disorders: relationships to drug addictions. Biochem Pharmacol. (2008) 75:63–75. doi: 10.1016/j.bcp.2007.06.043
82. Haber SN, Knutson B. The reward circuit: linking primate anatomy and human imaging. Neuropsychopharmacology. (2010) 35:4–26. doi: 10.1038/npp.2009.129
83. Cartoni E, Balleine B, Baldassarre G. Appetitive Pavlovian-instrumental Transfer: a review. Neurosci Biobehav Rev. (2016) 71:829–48. doi: 10.1016/j.neubiorev.2016.09.020
84. Everitt BJ, Robbins TW. Drug addiction: updating actions to habits to compulsions ten years on. Annu Rev Psychol. (2016) 67:23–50. doi: 10.1146/annurev-psych-122414-033457
85. Suzuki S, Lawlor VM, Cooper JA, Arulpragasam AR, Treadway MT. Distinct regions of the striatum underlying effort, movement initiation and effort discounting. Nat Hum Behav. (2020). doi: 10.1038/s41562-020-00972-y. Available online at: https://www.nature.com/articles/s41562-020-00972-y
86. Holroyd CB, Coles MGH. The neural basis of human error processing. Psychol Rev. (2002) 109:679–709. doi: 10.1037/0033-295X.109.4.679
87. Frank MJ, Seeberger LC, O'reilly RC. By carrot or by stick: cognitive reinforcement learning in parkinsonism. Science. (2004) 306:1940–3. doi: 10.1126/science.1102941
88. Buckholtz JW, Treadway MT, Cowan RL, Woodward ND, Li R, Ansari MS, et al. Dopaminergic network differences in human impulsivity. Science. (2010) 329:532. doi: 10.1126/science.1185778
89. Hofmans L, Papadopetraki D, Bosch R, van den Määttä JI, Froböse MI, Zandbelt BB, et al. Baseline dopamine predicts individual variation in methylphenidate's effects on cognitive motivation. BioRxiv. (2019) 859637. doi: 10.1101/859637. Available online at: https://www.biorxiv.org/content/10.1101/859637v3
90. Clark L, Stokes PR, Wu K, Michalczuk R, Benecke A, Watson BJ, et al. Striatal dopamine D2/D3 receptor binding in pathological gambling is correlated with mood-related impulsivity. Neuroimage. (2012) 63:40–6. doi: 10.1016/j.neuroimage.2012.06.067
91. Steeves TDL, Miyasaki J, Zurowski M, Lang AE, Pellecchia G, Van Eimeren T, et al. Increased striatal dopamine release in Parkinsonian patients with pathological gambling: a [11C] raclopride PET study. Brain. (2009) 132:1376–85. doi: 10.1093/brain/awp054
92. Knutson B, Greer SM. Review. Anticipatory affect: neural correlates and consequences for choice. Philos Transac R Soc B Biol Sci. (2008) 363:3771–86. doi: 10.1098/rstb.2008.0155
93. Schlund MW, Magee S, Hudgins CD. Human avoidance and approach learning: evidence for overlapping neural systems and experiential avoidance modulation of avoidance neurocircuitry. Behav Brain Res. (2011) 225:437–48. doi: 10.1016/j.bbr.2011.07.054
94. Oldham S, Murawski C, Fornito A, Youssef G, Yücel M, Lorenzetti V. The anticipation and outcome phases of reward and loss processing: a neuroimaging meta-analysis of the monetary incentive delay task. Hum Brain Mapp. (2018) 39:3398–418. doi: 10.1002/hbm.24184
Keywords: self-control, impulsive behavior, punishment, emotional regulation, avoidance learning, substance-related disorders, procrastination, addictive behaviors
Citation: Wypych M and Potenza MN (2021) Impaired Learning From Errors and Punishments and Maladaptive Avoidance—General Mechanisms Underlying Self-Regulation Disorders? Front. Psychiatry 11:609874. doi: 10.3389/fpsyt.2020.609874
Received: 24 September 2020; Accepted: 30 December 2020;
Published: 26 January 2021.
Edited by:
Boris B. Quednow, University of Zurich, SwitzerlandReviewed by:
Ann-Kathrin Stock, University Hospital Carl Gustav Carus, GermanyMauro Ceccanti, Sapienza University of Rome, Italy
Copyright © 2021 Wypych and Potenza. This is an open-access article distributed under the terms of the Creative Commons Attribution License (CC BY). The use, distribution or reproduction in other forums is permitted, provided the original author(s) and the copyright owner(s) are credited and that the original publication in this journal is cited, in accordance with accepted academic practice. No use, distribution or reproduction is permitted which does not comply with these terms.
*Correspondence: Marek Wypych, bS53eXB5Y2gmI3gwMDA0MDtuZW5ja2kuZWR1LnBs