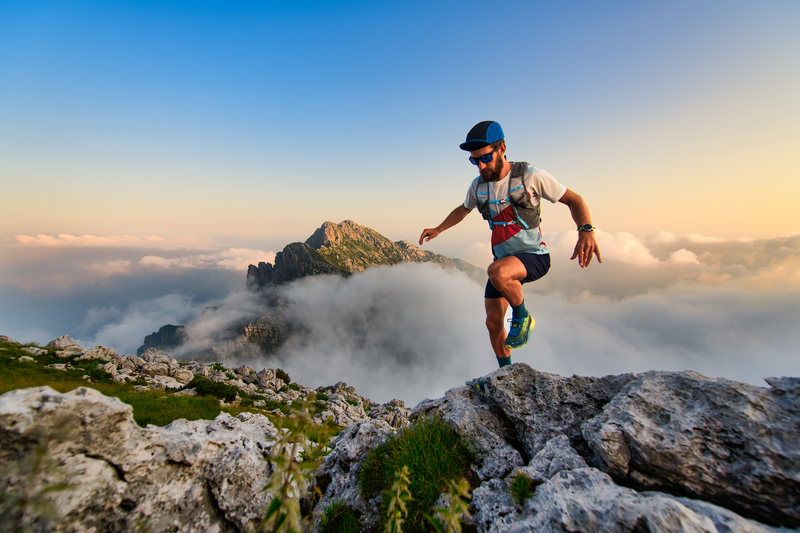
94% of researchers rate our articles as excellent or good
Learn more about the work of our research integrity team to safeguard the quality of each article we publish.
Find out more
ORIGINAL RESEARCH article
Front. Psychiatry , 04 December 2020
Sec. Addictive Disorders
Volume 11 - 2020 | https://doi.org/10.3389/fpsyt.2020.599141
This article is part of the Research Topic Cognitive Control and Reward Processing in Addiction View all 10 articles
Converging evidence indicates that addiction involves impairment in reward processing systems. However, the patterns of dysfunction in different stages of reward processing in internet gaming addiction remain unclear. In previous studies, individuals with internet gaming disorder were found to be impulsive and risk taking, but there is no general consensus on the relation between impulsivity and risk-taking tendencies in these individuals. The current study explored behavioral and electrophysiological responses associated with different stages of reward processing among individuals with internet gaming disorders (IGDs) with a delayed discounting task and simple gambling tasks. Compared to the healthy control (HC) group, the IGD group discounted delays more steeply and made more risky choices, irrespective of the outcome. As for the event-related potential (ERP) results, during the reward anticipation stage, IGDs had the same stimulus-preceding negativity (SPN) for both large and small choices, whereas HCs exhibited a higher SPN in large vs. small choices. During the outcome evaluation stage, IGDs exhibited a blunted feedback-related negativity for losses vs. gains. The results indicate impairment across different stages of reward processing among IGDs. Moreover, we found negative correlation between impulsivity indexed by BIS-11 and reward sensitivity indexed by SPN amplitude during anticipation stage only, indicating different neural mechanisms at different stages of reward processing. The current study helps to elucidate the behavioral and neural mechanisms of reward processing in internet gaming addiction.
Internet gaming disorder is a rapidly increasing concern in today's world. It is a preoccupation and obsession with internet games that interferes with one's social, personal, or occupational life, with typical symptoms of dependence being tolerance, withdrawal, and failed attempts to quit the habit (1). As one of the most common behavioral addictions, it is an emerging health concern. It has been included as Internet Gaming Disorder in ICD-11 and as a “Condition for Further Study” in DSM-5. Internet gaming disorders (IGDs) often struggle in their day-to-day activities, relationships, and jobs because of prolonged game play. They are more likely to have poor sleep quality (2), tend to suffer from emotional problems such as depression and anxiety (2, 3), have poor coping skills (4) and are more prone to developing psychopathology or psychopathological symptoms in the long run (5–7). IGDs often use internet games as an escape from negative moods and feelings, such as hopelessness and guilt (1), allowing them to feel relaxed (6) and in control of the situation (8).
Reward processing is an important aspect of human functioning affecting daily life, and has also been regarded as a key neural mechanism involved in behavioral and cognitive processes related to addiction (9, 10). Impairments in reward processing is the core symptom of many kinds of mental and neurological diseases (11, 12), including drug addiction. It can be classified into two stages: reward anticipation and outcome evaluation (13).
Reward anticipation refers to the incentive salience of a reward. Incentive salience is a psychological process that imbues the perception of stimuli with salience and transforms them into incentive stimuli. Previous addiction studies conducted on substance addiction and internet gaming disorder indicated altered reward processing system among the addicts during the reward anticipation stage. They found less activations in ventral stratum and decreased prefrontal cortical sensitivity to monetary rewards (14–17).
Outcome evaluation refers to the hedonic enjoyment received from reward consumption. IGDs have been found to have alterations in the reward processing system (18–20). The addiction studies conducted on substance addiction and behavioral addiction (i.e., internet gaming disorder) have found addicts to be driven toward high rewards and tend to ignore negative consequences, thus resulting in impaired decision making process and risk taking tendencies (21–26).
Monetary rewards are frequently used to study neural mechanisms involved in reward processing among healthy and addicted individuals (27, 28). However, the findings have been inconsistent about whether individuals with addiction have enhanced or blunted responses to monetary rewards.
The inhibitory control dysfunction theory (29) attempts to explain the alterations in the reward processing systems underlying addiction. It proposes impulsivity and reward processing as the underlying factors of addiction that play a role in promoting or limiting drug use at each of the three stages of addiction: (i) initiation of use, (ii) maintenance of use, and (iii) relapse. According to this theory, impulsivity is a personality trait while impairment in reward processing refers to sensitivity to rewards (positive effects of the drug) paired with insensitivity to punishment (negative outcomes of the drug). This theory has often found support from the research studies (30–34) that found addicts to be impulsive and indulge in risky decision making.
Currently, there is no conclusive evidence from previous studies providing a consensus about the neural correlates of the reward processing system at different stages of reward processing. In the current study, we focused on three key ERP components: stimulus-preceding negativity (SPN), feedback-related negativity (FRN), and P300. SPN is a negative-going slow wave. It is considered an electrophysiological index of reward expectation. Previous research has shown that people with substance dependence had larger SPN while anticipating substance related cues than controls (35, 36). The two other ERP components, FRN and P300, play important roles in outcome evaluation. FRN is usually a negative deflection following feedback onset that typically peaks around 250 ms. Previous studies have found that people with substance dependence have a larger FRN peak, indicating impairment in outcome evaluation processes (23, 37). P300 is a positive deflection typically peaking around 300–500 ms after feedback onset. Previous studies have found that people with substance dependence have larger P300 amplitudes than controls, indicating their poor attentional control.
IGDs have been found to have high impulsivity (38) and high sensation seeking (5). These personality traits are associated with inability to delay gratification, leading to steep delay discounting. Delay discounting refers to the subjective devaluation of an outcome with an increase in delay of its attainment (39, 40). Previous studies have found IGDs to be highly impulsive, which is reflected by a dysfunctional prefrontal cortex (41) and decreased frontostriatal connectivity (42), leading to risky decisions.
IGDs have altered risk evaluation, high risk taking tendencies, and tend to indulge in risky decision making (30, 32, 43–45). They were found to have enhanced reward sensitivity and decreased loss sensitivity compared to control counterparts. A similar study conducted on IGD adolescents (46) testing the dual-system model found that individuals with internet gaming addiction have altered reward processing and inhibitory control in a gambling task and a Go/No Go task, respectively. These impairments in reward processing system make it difficult for IGDs to quit playing internet games despite negative effects on their daily life, such as poor grades and deterioration of relationships (5, 7). Their altered reward processing system also makes them prone to developing psychopathology (47, 48).
A few fMRI studies (32, 34, 49) have examined the neural basis of reward processing among IGDs. However, the low temporal resolution made fMRI a less powerful technique to answer the question about different processing stages. Instead, ERP technique has fine-grained temporal resolution, and is uniquely suitable to investigate in detail the time course of reward processing in internet gaming addiction. To our knowledge, no ERP study on internet gaming disorder to date has explored the neural correlates of behavioral addiction across different stages of reward processing. The P300 and FRN components have frequently been studied among IGDs (19, 37). However, the SPN component occurring at the early stages of reward processing often remains a neglected ERP component in these studies. In light of previous work indicating possible abnormal reward system in IGDs, the current study aimed to bridge this gap by exploring alterations in the reward processing system during different stages of reward processing. In the current study, we examined the reward processing systems in IGDs as compared to the HCs while they expected and received rewards during the delayed discounting and a simple gambling task. Behaviorally, we anticipated the IGDs to make more risky choices and discount delays more steeply than the HCs. Neurally, we expected decreased risk sensitivity, indexed by smaller magnitude effect on SPN during the anticipation stage and reduced FRN magnitude during the outcome-appraisal stage of reward processing. Moreover, based on inhibitory control dysfunction theory, we predicted that larger P300 amplitude would be observed on gain trials than loss trials. We hypothesized that IGDs discount delays more steeply on a delayed discounting task and make more risky decisions, irrespective of whether they were in a gain or loss condition. With a delay discounting task, we further explored and established the relationship between delay gratification and risky decision making among IGDs.
Thirty-five male adults (age 22.06 ± 3.65) with internet gaming disorder and another 39 age-matched healthy male adults (age 21.95 ± 3.47) in total were recruited in this study. They had either normal or corrected-to-normal vision and self-reported no history of physical disability, chronic physical illness, or neurological or psychiatric problems. The inclusion criteria for IGDs required minimum scores of 50 on the Internet Addiction Test (IAT) (50), and 5 on the DSM Test for Internet Gaming Disorder (51), while for the control group, the scores on both the tests were required to be lower than these thresholds. The IAT (Cronbach's α = 0.93; r = 0.46) and DSM Test (Cronbach's α = 0.91; r = 0.44) were used to screen the IGDs from the control group. These two tests were intended to measure the effect of Internet use on the individual's daily life and the extent of problems caused by it on daily routine, work, social life, sleep routine, and feelings, in accordance with DSM-5 criteria. In addition, the Alcohol Use Disorder Identification Test [AUDIT, (52)], Beck Depression Inventory [BDI-II, (53)], State-Trait Anxiety Inventory-Trait [STAI-T, (54)], and State-Trait Anxiety Inventory-State (STAI-S) were used to exclude those with alcohol use disorder, depression, and anxiety disorders. Moreover, we used the Barratt Impulsiveness Scale-Version 11 [BIS-11, (55)], Behavioral Inhibition System/Behavioral Activation System [BAS/BIS, (56)], and Sensation Seeking Scale (57) to explore impulsivity, reward systems, and sensation seeking in relation to decision making in IGDs. The two groups were counterbalanced on years of education, with high school as the minimum education level (see Table 1).
Thirty-three IGDs and 35 HCs completed the delay discounting task, among which 24 IGDs and 26 HCs participated in the ERP study with a simple gambling task. In the gambling task analysis, five subjects (one IGD and four HCs) were excluded from further analysis because they mostly chose one option (high or low, over 90%). With this, we sought to ensure participants' conscious attention on the trials, while employing the excluding criteria comparable to that reported by Dewitt et al. (58). In the ERP analysis, another three subjects (two IGDs and one HC) were excluded from the SPN analysis, and four subjects (two IGDs and two HCs) were excluded from the FRN and P300 analysis, respectively, because too few effective epochs were left after removing artifacts.
The research was approved by the Institutional Review Board of the Institute of Psychology, Chinese Academy of Science before the commencement of the experiments. All participants signed a consent form before participating in the experiment.
In this task, participants were required to choose between a small gain that was available immediately and a fixed larger gain (¥ 1,000) that was delayed by one of five periods of time (1 week, 1 month, 6 months, 3 years, and 15 years). The participants practiced by making choices between 2-week delayed periods and varied amounts available immediately, to familiarize themselves with the procedure before the formal experiment procedures. During the formal experiment blocks, there were seven choices for the immediate gain amount with five delayed periods: 1 week, 1 month, 6 months, 1 year, and 15 years. An algorithm was used to adjust the amount of the immediate gain across the seven to estimate the subjective values of delayed gains. The participants were given the opportunity to restart the procedure after each trial to avoid errors that could lead to inaccurate estimates of their subjective values, in case they wanted to modify or change their choice.
In the simple gambling task, the participants were instructed in the beginning that their remuneration for the experiment would be dependent on the amount they won or lost in this task (see Figure 1). The main procedure consisted of one practice block and six main blocks. The practice block consisted of 10 trials, and each main block comprised 80 trials with a short break between two consecutive blocks.
The trial contained two options (9 for low risk and 99 for high risk) appearing on either side of a fixation cross, which disappeared until responses were made. The participants were required to choose one option by pressing “f” (for the left option) or “j” (for the right option). After a response was made, only the fixation cross remained on the screen for 2,000 ms followed by a feedback slide for 1,000 ms. The feedback slide contained points with a “+” or “-” sign to indicate the points they had won or lost for their response.
We used Brain Products System (64 channel amplifier, Brain Vision Recorder Version 2.0; Brain Products, GmbH, Germany) for EEG data recording. The Easy Cap electrode system (EASY-CAP, Herrsching) was used to place electrodes in accordance with the 10–20 system on 64 positions. Vertical eye movements were recorded by placing one electrode below the right eye (VEOG). The channel FCz was set as the reference channel during data recording. Chloride free-electrolyte gel was used to gently abrade the scalp to keep impedances in electrodes below 5 kΩ. EEG data were recorded at a sampling rate of 500 Hz with a pass band of 0.01–100 Hz.
We adopted the analysis approaches from previous studies (26, 59). EEGLAB toolbox (60) running under MATLAB software was used for the raw data analysis. The data were re-referenced to the average of channels TP9 and TP10. The reference channel FCz was then added back to the data. A low-pass filter of 20 Hz was used to determine the SPN for pre-feedback epochs (2,000 ms pre-stimulus, 500 ms post-stimulus), while a band-pass filter of 0.1–20 Hz was applied for FRN and P300 for post-feedback epochs (200 ms pre-stimulus, 800 ms post-stimulus). The independent component analysis ocular correction method was used to remove any artifacts present due to eye movements and eye blinks in the epochs after visual inspection. We set the activity from −200 to 0 ms, and from −2,000 to −1,800 ms as baseline correction for post-feedback components (FRN and P300), and the pre-feedback component (SPN), respectively.
For the delay discounting task, the area under the discounting curves (AUCs) for each subject were calculated with the method in line with previous studies (61–63). The AUC values were used since they are not affected by the quality of fit of the discounting models, and are usually more normally distributed than other discounting function parameters (e.g., k or h values) (64).
For the gambling task, we calculated the effects of valence (gain or loss) on the basic risky choice proportion and conditional risky choice proportions (choice following the previous outcome) and reaction times.
At the EEG level, we recorded the peak amplitudes of the four conditions: high gain, low gain, high loss, and low loss on SPN, FRN, and P300 components. The component values for SPN were measured with four electrodes in the left-hemisphere (C3, C5, FC3, and FC5), and four electrodes in the right-hemisphere (C4, C6, FC4, and FC6) electrodes according to the topographic maps and grand average waveforms. The time window for SPN was observed at −200 to 0 ms (before feedback). The FRN was extracted from 250 to 350 ms after the feedback onset at FCz, Fz, and Cz, where it was observed to be maximal. P300 was measured with CPz and Pz from 350 to 450 ms (after the feedback). The channels and time windows for each component were selected according to the activations on the topographic maps and the peak of the waveform, respectively.
Two-sample t-tests were applied to the AUC values in the delay-discounting task and the basic choice risk proportion. The basic choice reaction time in the gambling task was analyzed with an analysis of variance (ANOVA) of Group (IA group vs. HC group) × Risk (high risk vs. low risk). The conditional analysis was achieved with a three-way ANOVA of Group (IA group vs. HC group) × Risk (high risk vs. low risk) × Previous Outcome (win vs. loss).
The ERP data for the simple gambling task were analyzed twice, one pre-feedback condition for determining the SPN component and one post-feedback stimuli for FRN and P300 components. Repeated-measure ANOVAs were used for the SPN component with the between-subject factor GROUP (HC group vs. IGD group) and within-subject factor Magnitude (high vs. low) and Hemisphere (right vs. left). Repeated-measure ANOVAs were used for FRN and P300 component, with the between-subjects factor Group (HC group vs. IA group) and within-subject factor Magnitude (high vs. low) and another within-subject factor Valence (gain vs. loss). Greenhouse-Geisser correction was used for two or more factors with major effects. Post-hoc analysis was conducted using Bonferroni corrections.
Table 1 shows the demographic data for the HC and IGD groups. The groups did not differ in age and educational level. As expected, the groups differed significantly on the IAT and DSM Test for Internet Gaming. Moreover, the IGD group scored higher on BDI, STAI, the Motor subscale, Non-Planning in the BIS-11, and the BAS-Fun-Seeking subscale in the BAS/BIS.
For the delay discounting task, there was a significant group effect, t (66) = 2.57, p = 0.012, indicating that the IGD group discounted delayed outcomes (MAUC = 0.17, SDAUC = 0.02) more steeply than the HC group (MAUC = 0.26, SDAUC = 0.03) (see Figures 2A,B).
Figure 2. Behavioral Results in delay discounting task and Gambling Task. (A) Slope for area under the curve (AUC) and (B) the distribution of mean value of area under the curve on Delayed Discounting task. (C) Proportion of Basic Choice and Reaction Times on Simple Gambling Task. (D) Proportion of Risky Choice on Simple Gambling Task.
For decision making time, there was no significant main effect of group, F(1,43) = 0.82, p = 0.371, ηp2 = 0.019, or condition effect, F(1,43) = 1.60, p = 0.201, ηp2 = 0.038, nor a significant condition × group interaction effect, F(1,43) = 0.14, p = 0.707, ηp2 = 0.003.
There was a marginally significant group effect, t (43) = 1.82, p = 0.076, indicating that a higher proportion (56.5%) of the IGD group preferred risky choices than the HC group (48.2%). Specifically, IGDs tended to make more risky decisions than the chance level (50%), t (22) = 2.020, p = 0.056. In contrast, HCs exhibited a risk-neutral pattern, t (21) = −0.563, p = 0.579 (see Figure 2C).
Both groups tended to risk larger amounts after facing a loss in the previous trial than when they had a gain in the previous trial, F(1,43) = 9.59, p = 0.003, ηp2 = 0.182, and after making a high-risk choice in the previous trial than when they made a low-risk choice in the previous trial, F(1,43) = 21.38, p = 0.000, ηp2 = 0.332. The IGD group made more risky choices than the HC group, irrespective of the previous outcome, F(1,43) = 6.12, p = 0.017, ηp2 = 0.125 (see Figure 2D).
Figure 3 presents the grand average ERP waveforms at FCz elicited by gains and losses and their differences, and the topographic map for these two groups. Repeated-measures ANOVA revealed a significant magnitude effect on the FRN component, F(1,41) = 12.33, p = 0.001, ηp2 = 0.231, indicating that the FRN amplitude was higher in high-risk than in low-risk outcomes (−2.11 μV vs. −0.67 μV). The interaction between magnitude and group was also statistically significant, F(1,41) = 5.17, p = 0.028, ηp2 = 0.112. Simple effect analysis revealed that the FRN amplitude was greater in high-risk outcomes compared to low-risk outcomes only in the HC group (−2.74 μV vs. −0.36 μV, p = 0.000), but not in the IGD group (−1.48 μV vs. −0.97 μV, p = 0.392).
Figure 3. Grand average ERP waveforms following low- and high-risk decisions for HCs and IGDs at FCz. The upper figure shows the ERP waveforms for HCs and IGDs at FCz. FRN is calculated as the difference between loss and gain waveforms after feedback, and the time window was depicted as the shaded areas. The lower figure shows the topographic maps on time window 250–350 ms.
Figure 4 presents the grand average ERP waveforms at Pz elicited by gains and losses, and the topographic map for these two groups. Repeated-measures ANOVA revealed significant magnitude effect, F(1,41) = 74.47, p = 0.000, ηp2 = 0.645, indicating that the P300 amplitude was higher in high-risk outcomes than in low-risk outcomes (12.74 μV vs. 7.39 μV); and significant valence effect, F(1,41) = 7.51, p = 0.009, ηp2 = 0.155, indicating that the P300 amplitude was higher in a gain context than in a loss context (10.44 μV vs. 9.70 μV).
Figure 4. Grand average ERP waveforms following low- and high-risk decisions for HCs and IGDs at Pz. The upper figure shows the ERP waveforms for controls and IGDs at Pz. The time window was depicted as the shaded areas. The lower figure shows the topographic maps on time window 350–450 ms.
Figure 5 presents the grand average ERP waveforms at C3 and C4 and topographic maps of the SPN (−200 to 0 ms) for these two groups. Repeated-measures ANOVA revealed a significant magnitude effect on the SPN component, F(1,42) = 5.06, p = 0.030, ηp2 = 0.108, indicating that the SPN amplitude was higher for high-risk choices than for low-risk choices (−2.26 μV vs. −1.69 μV). The interaction between magnitude and group was marginally significant, F(1,42) = 3.03, p = 0.089, ηp2 = 0.067. Simple effect revealed that the SPN amplitude was greater in high-risk decision making compared to low-risk decision making only in the HC group (−2.41 μV vs. −1.39 μV, p = 0.006), but not in the IGD group (−2.12 μV vs. −1.97 μV, p = 0.727).
Figure 5. Grand average ERP waveforms following low- and high-risk decisions for HCs and IGDs at C3 and C4. The upper figure shows the ERP waveforms for controls and internet gamer at C3 and C4. The time window was depicted as the shaded areas. The lower figure shows the topographic maps on time window −200 to 0 ms.
To examine the potential relationship between impulsivity and risk-taking tendencies at the individual difference level, we calculated the correlation between the impulsivity indices (i.e., AUC and BIS score) and basic choice, risky choice, and three ERP amplitudes within each group independently. Although none of these indices significantly correlated with AUC results, we found significant negative correlation between the BIS score and the SPN amplitude in the left hemisphere when choosing low risk choice, r = −0.41, p = 0.031, as well as when choosing high risk choice, r = −0.38, p = 0.044 (see Figure 6).
Figure 6. Correlation between BIS score and the SPN amplitude. The left panel shows the result when choosing low risk choices, and the right panel shows the result when choosing high risk choices.
In our sample of participants, we found that IGDs had relatively higher impulsivity, higher proneness to risky decision making, reduced ability to delay gratification, reduced ability to evaluate risk, and different outcome expectancies in risky situations. The behavior of IGDs, that is, making more risky choices, is supported by neural patterns indicating higher sensitivity to rewards and lower sensitivity to punishment among IGDs.
Previous research on substance addiction found that people with substance dependence discounted delayed gains more steeply than non-dependent people on a delay discounting task (65–68). This effect has been found to hold true for IGDs, which were shown to be unable to delay gratification, as indicated by their steep pattern of discounting delayed gains on a delayed discounting task (41, 61, 69). The results of the delay discounting task are supported by the high impulsivity scores on the BIS among the IGD group compared to the HC group. The IGD group were more impulsive on the motor impulsiveness and non-planning subscales than the HC group, in accordance with previous studies (70–73). However, there were no differences in attention impulsivity subscale of BIS-11 between the two groups. This may be attributed to the positive effects of online gaming or video gaming on individuals in increasing sustained attention (74, 75). IGDs were revealed to be more thrill and adventure seeking and more sensitive to rewards than HCs. These observations are in line with the findings of previous studies on problematic internet gaming (76–78).
On the behavioral level, risk-taking tendencies were more pronounced in the IGD group. They were also found to be more prone to make risky choices, irrespective of whether the previous outcome was a win or a loss. These results are in accordance with previous literature that found problematic IGDs to be more focused on and sensitive to wins and less focused on and less sensitive to losses (79, 80). The enhanced reward sensitivity and decreased sensitivity to losses lead them to risky decision making. Previous studies have indicated an association between risky choices and personality factors as impulsivity, sensation seeking, thrill seeking behaviors among addicts. They found that addicts tend to ignore the negative consequences of the situation and focus only on positive rewards (5, 43, 44, 46). Consistently, the IGD group in our current study were also found to have enhanced sensitivity toward rewards as indicated by their higher scores on the BAS/BIS and thrill seeking than the HC group, as well as their less sensitivity to loss, indexed by behavioral choices, SPN and FRN amplitudes in simple gambling task.
At the early stage of reward processing, the SPN was more negative for the larger risk than the smaller risk for the HC group, while no significant differences were found in the IGD group. These results indicated that IGDs expected the same reward outcome whether the risk was high or low, but HCs expected more on a larger risk. Furthermore, the IGDs were less concerned about the outcome, indicating their high risk-taking tendencies. These results are consistent with previous findings that found an altered ability to evaluate risk among IGDs (22, 32, 81).
At the later stages of reward processing, the more prominent FRN amplitude for high-risk choices in comparison with low risk choices was observed in the HC group. However, such results diminished in the IGD group, indicating their increased risk-taking tendencies and decreased sensitivity toward high risk situations. FRN is associated with the binary evaluation of positive vs. negative outcomes (gains vs. losses in our study) based on external feedback that outcome is worse than expected (82). The amplitude of FRN increases when external feedback indicates a negative outcome (i.e., loss). However, the previous studies found that IGDs are less sensitive to negative outcomes. Our results of FRN are consistent with previous studies on individuals with internet gaming disorder (23, 37) that found IGDs to have reduced amplitudes on FRN than healthy controls. Similar to our results of the FRN component, these studies also found a blunted risk effect among IGDs irrespective of the feedback response. Our results on FRN seems to be comparable to the previous studies have found blunted FRN to be associated with unplanned impulsivity and high scores on BIS/BAS system (83, 84). In our sample of participants, the amplitude of the P300 component was significantly larger for the gain than the loss condition indicating their attentional allocation to gains more than the losses. This is consistent with previous studies on decision making and risk-taking (85–87) that found an increase in the amplitudes of the P300 component on gain than loss conditions in a gambling task. The P300 component is often regarded as an index of attentional allocation to task relevant stimuli (88). In contrast to previous studies (37, 89), we did not find a significant group effect on P300 amplitude. A possible explanation for this inconsistency that monetary rewards may not be a strong reinforcer for our IGD group. The enhanced preference toward rewards and less sensitivity toward punishment is indicated but monetary rewards may not be a stronger reinforcer for the IGDs than the HCs, resulting in this inconsistency. Previous studies have also indicated mixed findings while using monetary rewards. In severely addicted individuals, such as cocaine addicts, monetary rewards may not elicit the same sensitivity as using the substance reward they are addicted to (14). This may also be explained considering previous studies (90) that reported an improvement in attentional control as a result of playing computer games.
The results of the current study on IGD are very similar to the previous study conducted on IGD of adolescents (46) that also found IGD to depict high impulsivity on BIS-11, greater tendency to novelty seeking experience on BAS-F subscale of BAS/BIS and significantly greater tendency to indulge in thrill and sensation seeking activities than the non-IGDs. They also found IGD to be more prone to making risky choices but could not find any interaction effect between high risky choices and reaction times, in line with the current study. Although we could not find enhanced FRN for the controls directly, we did find the peak amplitude of the difference between loss and gain to be significantly enhanced on FRN, similar to this study. However, they also only found significant magnitude and valence effect on P300 with no interaction effect between group and the peak amplitude on P300, similar to our study. These similarities give an indication that impairment in reward processing is extended to the adulthood following the same pattern as observed among the adolescents suffering from internet gaming disorder.
We found SPN component and subjective impulsivity, as indicated by BIS-11 scores, to be negatively correlated with each other in the IGD group. It indicates that risk sensitivity during the anticipation stage decreases as the impulsivity level increases among IGDs. This is in line with the addiction studies that found high risk taking tendencies to be associated with high impulsivity (91, 92). However, the similar pattern could not be found in the outcome-appraisal stage, nor any correlation pattern could be determined between impulsivity and delayed discounting and risk-taking strategies on the behavioral level. In agreement with studies (93, 94) that indicated that impulsivity and risky decision making are distinct constructs, our results are consistent with the notion that the relation between impulsivity and risk taking is more complex and these personality measures may function as distinct constructs among IGDs (93). The dominant construct in each internet gamer may vary from individual to individual, for example, some IGDs may be impulsive but not risk-taking and vice versa. In a recent study, researchers found impulsivity and risk-taking tendencies to be distinct constructs associated with separate moods. Risky decision making and high-risk behaviors were found to be influenced by the positive emotions while high impulsivity was found to be associated with negative emotions (94). These results indicate the contrast between anticipation and outcome-appraisal stage, in relation to association between decreased risk sensitivity and impulsivity traits. Future studies may explore the effect of each dominant construct on reward processing among IGDs with different degrees of dependence with larger sample sizes.
This study explored the neural correlates of internet gaming disorder across different stages of reward processing. The results strengthen the notion that IGDs share common patterns of reward processing impairments with people with substance dependence. It also gives an insight into the distinct attentional allocation patterns found among IGDs, owing to their gaming addiction. The common and distinct patterns provide useful behavioral and neurological markers for subsequent prevention and intervention.
Due to the high prevalence of internet gaming addiction among young male adults, we selected only young male adults under 30 years old, which may also be one of the limitations of our study. Another limitation is the relatively small sample size. Although our study has indicated the patterns of impairment across different stages of reward processing, larger sample size studies may be required to confirm this impairment pattern, as well as across different age groups. An additional concern is the possible confounding effect of the relatively higher BDI and STAI-S scores of IGDs than HCs, which may bias the results of this study. Here, we would like to mention that the cognitive effects of internet gaming may be manifold and might also be linked to certain factors affecting the decision of the participants, such as mood, emotion and attention. Nevertheless, the results of the two tasks (delayed discounting task and simple gambling task) provide insight into some behavioral and neural patterns of reward processing among IGDs. Similar to people with substance dependence, the internet addiction group showed high impulsivity, reduced ability to delay gratification, altered ability to evaluate risk, altered outcome expectancies from risky situations and decisions, and risk-taking tendencies. However, IGDs in our sample were found to use an avoidance system in response to punishment, giving us an insight into a distinct pattern of reward processing among IGDs. They were found to be much more focused on larger gains and demonstrated less sensitivity to losses.
The raw data supporting the conclusions of this article will be made available by the authors, without undue reservation.
The studies involving human participants were reviewed and approved by the Review Board of Institute of Psychology, CAS. The patients/participants provided their written informed consent to participate in this study.
SR: data curation, writing—original draft, and writing—editing. GY: data curation, writing—original draft, and writing—editing. LW: writing—review and editing. WD: writing—review and editing. HW: writing—review and editing. GM: writing—review and editing. BZ: writing—review and editing. XL: conceptualization, writing—review and editing, supervision, project administration, and funding acquisition. All authors contributed to the article and approved the submitted version.
This research was supported by the National Key R&D Program of China (2016YFC0800901-Z03), the National Natural Science Foundation of China (Grants 31271194 and 61621136008/DFG TRR-169).
The authors declare that the research was conducted in the absence of any commercial or financial relationships that could be construed as a potential conflict of interest.
We thank Ya Zheng for providing invaluable comments on the manuscript, Moqian Tian and Zhenghan Li for providing help in data analysis, Honghui Xu, Hao Yu, and Ziwei Chen for helping in data collection.
1. American Psychiatric Association. Diagnostic and Statistical Manual of Mental Disorders (DSM-5®) (2013).
2. Bhandari PM, Neupane D, Rijal S, Thapa K, Mishra SR, Poudyal AK. Sleep quality, internet addiction and depressive symptoms among undergraduate students in Nepal. BMC Psychiatry. (2017) 17:106. doi: 10.1186/s12888-017-1275-5
3. Coventry KR. Mood state, arousal, decision making, and persistence at gaming. Addiction. (2001) 96:1860–1.
4. Brand M, Laier C, Young KS. Internet addiction: coping styles, expectancies, and treatment implications. Front. Psychol. (2014) 5:1256. doi: 10.3389/fpsyg.2014.01256
5. Floros G, Siomos K, Stogiannidou A, Giouzepas I, Garyfallos G. The relationship between personality, defense styles, internet addiction disorder, and psychopathology in college students. Cyberpsychol. Behav. Soc. Netw. (2014) 17:672–6. doi: 10.1089/cyber.2014.0182
6. Yan W, Li Y, Sui N. The relationship between recent stressful life events, personality traits, perceived family functioning and internet addiction among college students. Stress Health. (2014) 30:3–11. doi: 10.1002/smi.2490
7. Kim E, Yim HW, Jeong H, Jo SJ, Lee HK, Son HJ, et al. The association between aggression and risk of Internet gaming disorder in Korean adolescents: the mediation effect of father-adolescent communication style. Epidemiol. Health. (2018) 40:e2018039. doi: 10.4178/epih.e2018039
8. Bailey K, West R, Kuffel J. What would my avatar do? Gaming, pathology, and risky decision making. Front. Psychol. (2013) 4:609. doi: 10.3389/fpsyg.2013.00609
9. Trafton JA, Gifford EV. Behavioral reactivity and addiction: the adaptation of behavioral response to reward opportunities. J. Neuropsychiatry Clin. Neurosci. (2008) 20:23–35. doi: 10.1176/jnp.2008.20.1.23
10. Flagel SB, Akil H, Robinson TE. Individual differences in the attribution of incentive salience to reward-related cues: implications for addiction. Neuropharmacology. (2009) 56(Suppl. 1):139–48. doi: 10.1016/j.neuropharm.2008.06.027
11. Weiss F, Koob GF. Drug addiction: functional neurotoxicity of the brain reward systems. Neurotoxicol. Res. (2001) 3:145–56. doi: 10.1007/BF03033235
12. Ahmed SH. Neuroscience. Addiction as compulsive reward prediction. Science. (2004) 306:1901–2. doi: 10.1126/science.1107071
13. Robinson TE, Berridge KC. The neural basis of drug craving: an incentive-sensitization theory of addiction. Brain Res. Brain Res. Rev. (1993) 18:247–91. doi: 10.1016/0165-0173(93)90013-P
14. Goldstein RZ, Alia-Klein N, Tomasi D, Zhang L, Cottone LA, Maloney T, et al. Is decreased prefrontal cortical sensitivity to monetary reward associated with impaired motivation and self-control in cocaine addiction? Am. J. Psychiatry. (2007) 164:43–51. doi: 10.1176/ajp.2007.164.1.43
15. Beck A, Schlagenhauf F, Wustenberg T, Hein J, Kienast T, Kahnt T, et al. Ventral striatal activation during reward anticipation correlates with impulsivity in alcoholics. Biol. Psychiatry. (2009) 66:734–42. doi: 10.1016/j.biopsych.2009.04.035
16. Peters J, Bromberg U, Schneider S, Brassen S, Menz M, Banaschewski T, et al. Lower ventral striatal activation during reward anticipation in adolescent smokers. Am. J. Psychiatry. (2011) 168:540–9. doi: 10.1176/appi.ajp.2010.10071024
17. Hahn T, Notebaert KH, Dresler T, Kowarsch L, Reif A, Fallgatter AJ. Linking online gaming and addictive behavior: converging evidence for a general reward deficiency in frequent online gamers. Front. Behav. Neurosci. (2014) 8:385. doi: 10.3389/fnbeh.2014.00385
18. Dong G, Lin X, Hu Y, Xie C, Du X. Imbalanced functional link between executive control network and reward network explain the online-game seeking behaviors in Internet gaming disorder. Sci. Rep. (2015) 5:9197. doi: 10.1038/srep09197
19. Duven EC, Muller KW, Beutel ME, Wolfling K. Altered reward processing in pathological computer gamers–ERP-results from a semi-natural gaming-design. Brain Behav. (2015) 5:13–23. doi: 10.1002/brb3.293
20. Gleich T, Lorenz RC, Gallinat J, Kuhn S. Functional changes in the reward circuit in response to gaming-related cues after training with a commercial video game. Neuroimage. (2017) 152:467–75. doi: 10.1016/j.neuroimage.2017.03.032
21. Powell J, Dawkins L, Davis RE. Smoking, reward responsiveness, and response inhibition: tests of an incentive motivational model. Biol. Psychiatry. (2002) 51:151–63. doi: 10.1016/S0006-3223(01)01208-2
22. Lin X, Zhou H, Dong G, Du X. Impaired risk evaluation in people with Internet gaming disorder: fMRI evidence from a probability discounting task. Prog. Neuropsychopharmacol. Biol. Psychiatry. (2015) 56:142–8. doi: 10.1016/j.pnpbp.2014.08.016
23. Yau YH, Potenza MN, Mayes LC, Crowley MJ. Blunted feedback processing during risk-taking in adolescents with features of problematic Internet use. Addict. Behav. (2015) 45:156–63. doi: 10.1016/j.addbeh.2015.01.008
24. Weinstein A, Abu HB, Timor A, Mama Y. Delay discounting, risk-taking, and rejection sensitivity among individuals with internet and video gaming disorders. J. Behav. Addict. (2016) 5:674–82. doi: 10.1556/2006.5.2016.081
25. Hing N, Russell AM, Browne M. Risk factors for gambling problems on online electronic gaming machines, race betting and sports betting. Front. Psychol. (2017) 8:779. doi: 10.3389/fpsyg.2017.00779
26. Wei S, Zheng Y, Li Q, Dai W, Sun J, Wu H, et al. Enhanced neural responses to monetary rewards in methamphetamine use disordered individuals compared to healthy controls. Physiol. Behav. (2018) 195:118–27. doi: 10.1016/j.physbeh.2018.08.003
27. Balodis IM, Potenza MN. Anticipatory reward processing in addicted populations: a focus on the monetary incentive delay task. Biol. Psychiatry. (2015) 77:434–44. doi: 10.1016/j.biopsych.2014.08.020
28. Knutson B, Heinz A. Probing psychiatric symptoms with the monetary incentive delay task. Biol. Psychiatry. (2015) 77:418–20. doi: 10.1016/j.biopsych.2014.12.022
29. de Wit H, Richards JB. Dual determinants of drug use in humans: reward and impulsivity. In Bevins RA, Bardo MT, editors. Motivational Factors in the Etiology of Drug Abuse. Vol. 50 of the Nebraska Symposium on Motivation. University of Nebraska Press. (2004). p. 19–55.
30. Sun DL, Chen ZJ, Ma N, Zhang XC, Fu XM, Zhang DR. Decision-making and prepotent response inhibition functions in excessive internet users. CNS Spectr. (2009) 14:75–81. doi: 10.1017/S1092852900000225
31. Seok JW, Lee KH, Sohn S, Sohn JH. Neural substrates of risky decision making in individuals with Internet addiction. Aust. N. Z. J. Psychiatry. (2015) 49:923–32. doi: 10.1177/0004867415598009
32. Yao YW, Chen PR, Li S, Wang LJ, Zhang JT, Yip SW, et al. Decision-making for risky gains and losses among college students with Internet gaming disorder. PLoS ONE. (2015) 10:e0116471. doi: 10.1371/journal.pone.0116471
33. Yao YW, Wang LJ, Yip SW, Chen PR, Li S, Xu J, et al. Impaired decision-making under risk is associated with gaming-specific inhibition deficits among college students with Internet gaming disorder. Psychiatry Res. (2015) 229:302–9. doi: 10.1016/j.psychres.2015.07.004
34. Wang Y, Wu L, Wang L, Zhang Y, Du X, Dong G. Impaired decision-making and impulse control in internet gaming addicts: evidence from the comparison with recreational internet game users. Addict. Biol. (2017) 22:1610–21. doi: 10.1111/adb.12458
35. Dean AC, Sugar CA, Hellemann G, London ED. Is all risk bad? Young adult cigarette smokers fail to take adaptive risk in a laboratory decision-making test. Psychopharmacology. (2011) 215:801–11. doi: 10.1007/s00213-011-2182-y
36. Briggs Z, O'Connor M, Jollans EK, O'Halloran L, Dymond S, Whelan R. Flexible emotion-based decision-making behavior varies in current and former smokers. Addict. Behav. (2015) 45:269–75. doi: 10.1016/j.addbeh.2015.02.011
37. Balconi M, Venturella I, Finocchiaro R. Evidences from rewarding system, FRN and P300 effect in Internet-addiction in young people. Brain Sci. (2017) 7:81. doi: 10.3390/brainsci7070081
38. Kim J, Kim H, Kang E. Impaired feedback processing for symbolic reward in individuals with internet game overuse. Front. Psychiatry. (2017) 8:195. doi: 10.3389/fpsyt.2017.00195
39. Rachlin H. Diminishing marginal value as delay discounting. J. Exp. Anal. Behav. (1992) 57:407–15. doi: 10.1901/jeab.1992.57-407
40. Jones BA, Rachlin H. Delay, probability, and social discounting in a public goods game. J. Exp. Anal. Behav. (2009) 91:61–73. doi: 10.1901/jeab.2009.91-61
41. Wang Y, Wu L, Zhou H, Lin X, Zhang Y, Du X, et al. Impaired executive control and reward circuit in Internet gaming addicts under a delay discounting task: independent component analysis. Eur. Arch. Psychiatry Clin. Neurosci. (2017) 267:245–55. doi: 10.1007/s00406-016-0721-6
42. Kim JY, Chun JW, Park CH, Cho H, Choi J, Yang S, et al. The correlation between the frontostriatal network and impulsivity in internet gaming disorder. Sci. Rep. (2019) 9:1191. doi: 10.1038/s41598-018-37702-4
43. Hills AM, Dickerson M. Emotion, implicit decision making and persistence at gaming. Addiction. (2002) 97:598–9. doi: 10.1046/j.1360-0443.2002.t01-4-00134.x
44. Dong G, Wang J, Yang X, Zhou H. Risk personality traits of Internet addiction: a longitudinal study of internet-addicted Chinese university students. Asia Pac. Psychiatry. (2013) 5:316–21. doi: 10.1111/j.1758-5872.2012.00185.x
45. Dong G, Potenza MN. Risk-taking and risky decision-making in internet gaming disorder: implications regarding online gaming in the setting of negative consequences. J. Psychiatr. Res. (2016) 73:1–8. doi: 10.1016/j.jpsychires.2015.11.011
46. Li Q, Wang Y, Yang Z, Dai W, Zheng Y, Sun Y, et al. Dysfunctional cognitive control and reward processing in adolescents with Internet gaming disorder. Psychophysiology. (2020) 57:e13469. doi: 10.1111/psyp.13469
47. Ko CH, Hsiao S, Liu GC, Yen JY, Yang MJ, Yen CF. The characteristics of decision making, potential to take risks, and personality of college students with Internet addiction. Psychiatry Res. (2010) 175:121–5. doi: 10.1016/j.psychres.2008.10.004
48. Ding YJ, Lau CH, Sou KL, Abraham AA, Griffiths SM, Kim JH. Association between internet addiction and high-risk sexual attitudes in Chinese university students from Hong Kong and Macau. Public Health. (2016) 132:60–3. doi: 10.1016/j.puhe.2015.11.009
49. Starcevic V. Problematic internet use, reward sensitivity and decision making. Aust. N. Z. J. Psychiatry. (2015) 49:937–8. doi: 10.1177/0004867415600077
50. Lai CM, Mak KK, Watanabe H, Ang RP, Pang JS, Ho RC. Psychometric properties of the internet addiction test in Chinese adolescents. J. Pediatr. Psychol. (2013) 38:794–807. doi: 10.1093/jpepsy/jst022
51. Koo HJ, Han DH, Park SY, Kwon JH. The structured clinical interview for DSM-5 internet gaming disorder: development and validation for diagnosing IGD in Adolescents. Psychiatry Investig. (2017) 14:21–9. doi: 10.4306/pi.2017.14.1.21
52. Babor TF, de la Fuente JR, Saunders J, Grant M. AUDIT - the Alcohol-Use Disorders Identification Test: Guidelines for Use in Primary Health Care. Geneva: World Health Organization, Division of Mental Health (1989).
53. Beck AT, Steer RA, Ball R, Ranieri W. Comparison of beck depression inventories -IA and -II in psychiatric outpatients. J. Pers. Assess. (1996) 67:588–97. doi: 10.1207/s15327752jpa6703_13
54. Shek DT. The Chinese version of the state-trait anxiety inventory: its relationship to different measures of psychological well-being. J. Clin. Psychol. (1993) 49:349–58. doi: 10.1002/1097-4679(199305)49:3%3c349::aid-jclp2270490308%3e3.0.co;2-j
55. Li X, Phillips M, Xu D, Zhang Y-l, Yang S, Tong Y, et al. Reliability and validity of an adapted Chinese version of Barratt impulsiveness scale. Chinese Mental Health. (2011) 25:610–5.
56. Li YZ, Zhang Y, Jiang Y, Li H. The Chinese version of the BIS/BAS Scale: Reliability and Validity (2008).
57. Wang W, Wu YX, Peng ZG, Lu SW, Wang GP, Fu XM, et al. (2000). Test of sensation seeking in a Chinese sample. Pers. Individ. Dif. 28:169–79. doi: 10.1016/S0191-8869(99)00092-6
58. Dewitt B, Fischhoff B, Davis AL, Broomell SB, Roberts MS, Hanmer J. Exclusion criteria as measurements I: identifying invalid responses. Med. Decis. Making. (2019) 39:693–703. doi: 10.1177/0272989X19856617
59. Zheng Y, Liu X. Blunted neural responses to monetary risk in high sensation seekers. Neuropsychologia. (2015) 71:173–80. doi: 10.1016/j.neuropsychologia.2015.04.002
60. Delorme A, Makeig S. EEGLAB: an open source toolbox for analysis of single-trial EEG dynamics including independent component analysis. J. Neurosci. Methods. (2004) 134:9–21. doi: 10.1016/j.jneumeth.2003.10.009
61. Li Q, Tian M, Taxer J, Zheng Y, Wu H, Sun S, et al. Problematic internet users' discounting behaviors reflect an inability to delay gratification, not risk taking. Cyberpsychol. Behav. Soc. Netw. (2016) 19:172–8. doi: 10.1089/cyber.2015.0295
62. Tian M, Tao R, Zheng Y, Zhang H, Yang G, Li Q, et al. Internet gaming disorder in adolescents is linked to delay discounting but not probability discounting. Comput. Hum. Behav. (2018) 80:59–66. doi: 10.1016/j.chb.2017.10.018
63. Zheng Y, Tian M, Li Q, Liu X. Greater tolerance to losses in sensation seeking: evidence from probability and delay discounting. Drug Alcohol. Depend. (2018) 194:159–65. doi: 10.1016/j.drugalcdep.2018.09.027
64. Myerson J, Green L, Warusawitharana M. Area under the curve as a measure of discounting. J. Exp. Anal. Behav. (2001) 76:235–43. doi: 10.1901/jeab.2001.76-235
65. Madden GJ, Bickel WK, Jacobs EA. Discounting of delayed rewards in opioid-dependent outpatients: exponential or hyperbolic discounting functions? Exp. Clin. Psychopharmacol. (1999) 7:284–93. doi: 10.1037/1064-1297.7.3.284
66. Dallery J, Raiff BR. Delay discounting predicts cigarette smoking in a laboratory model of abstinence reinforcement. Psychopharmacology. (2007) 190:485–96. doi: 10.1007/s00213-006-0627-5
67. Field M, Rush M, Cole J, Goudie A. The smoking stroop and delay discounting in smokers: effects of environmental smoking cues. J. Psychopharmacol. (2007) 21:603–10. doi: 10.1177/0269881106070995
68. Andrade LF, Alessi SM, Petry NM. The effects of alcohol problems and smoking on delay discounting in individuals with gambling problems. J. Psychoactive Drugs. (2013) 45:241–8. doi: 10.1080/02791072.2013.803645
69. Buono FD, Sprong ME, Lloyd DP, Cutter CJ, Printz DM, Sullivan RM, et al. Delay discounting of video game players: comparison of time duration among gamers. Cyberpsychol. Behav. Soc. Netw. (2017) 20:104–8. doi: 10.1089/cyber.2016.0451
70. Skinner MD, Aubin HJ, Berlin I. Impulsivity in smoking, nonsmoking, and ex-smoking alcoholics. Addict. Behav. (2004) 29:973–8. doi: 10.1016/j.addbeh.2004.02.045
71. Doran N, McChargue D, Cohen L. Impulsivity and the reinforcing value of cigarette smoking. Addict. Behav. (2007) 32:90–8. doi: 10.1016/j.addbeh.2006.03.023
72. VanderVeen JW, Cohen LM, Cukrowicz KC, Trotter DR. The role of impulsivity on smoking maintenance. Nicotine Tob. Res. (2008) 10:1397–404. doi: 10.1080/14622200802239330
73. Spillane NS, Smith GT, Kahler CW. Impulsivity-like traits and smoking behavior in college students. Addict. Behav. (2010) 35:700–5. doi: 10.1016/j.addbeh.2010.03.008
74. Mishra J, Bavelier D, Gazzaley A. How to assess gaming-induced benefits on attention and working memory. Games Health J. (2012) 1:192–8. doi: 10.1089/g4h.2011.0033
75. Trisolini DC, Petilli MA, Daini R. Is action video gaming related to sustained attention of adolescents? Q. J. Exp. Psychol. (2018) 71:1033–9. doi: 10.1080/17470218.2017.1310912
76. Hu J, Zhen S, Yu C, Zhang Q, Zhang W. Sensation seeking and online gaming addiction in adolescents: a moderated mediation model of positive affective associations and impulsivity. Front. Psychol. (2017) 8:699. doi: 10.3389/fpsyg.2017.00699
77. Yen JY, Liu TL, Wang PW, Chen CS, Yen CF, Ko CH. Association between Internet gaming disorder and adult attention deficit and hyperactivity disorder and their correlates: impulsivity and hostility. Addict. Behav. (2017) 64:308–13. doi: 10.1016/j.addbeh.2016.04.024
78. Tian Y, Yu C, Lin S, Lu J, Liu Y, Zhang W. Sensation seeking, deviant peer affiliation, and internet gaming addiction among Chinese adolescents: the moderating effect of parental knowledge. Front. Psychol. (2018) 9:2727. doi: 10.3389/fpsyg.2018.02727
79. Dong G, Hu Y, Lin X. Reward/punishment sensitivities among internet addicts: implications for their addictive behaviors. Prog. Neuropsychopharmacol. Biol. Psychiatry. (2013) 46:139–45. doi: 10.1016/j.pnpbp.2013.07.007
80. Altbacker A, Plozer E, Darnai G, Perlaki G, Horvath R, Orsi G, et al. Problematic internet use is associated with structural alterations in the brain reward system in females. Brain Imaging Behav. (2016) 10:953–9. doi: 10.1007/s11682-015-9454-9
81. Qi X, Du X, Yang Y, Du G, Gao P, Zhang Y, et al. Decreased modulation by the risk level on the brain activation during decision making in adolescents with internet gaming disorder. Front. Behav. Neurosci. (2015) 9:296. doi: 10.3389/fnbeh.2015.00296
82. Hajcak G, Moser JS, Holroyd CB, Simons RF. The feedback-related negativity reflects the binary evaluation of good versus bad outcomes. Biol. Psychol. (2006) 71:148–54. doi: 10.1016/j.biopsycho.2005.04.001
83. Balconi M, Crivelli D. FRN and P300 ERP effect modulation in response to feedback sensitivity: the contribution of punishment-reward system (BIS/BAS) and behaviour identification of action. Neurosci. Res. (2010) 66:162–72. doi: 10.1016/j.neures.2009.10.011
84. Onoda K, Abe S, Yamaguchi S. Feedback-related negativity is correlated with unplanned impulsivity. Neuroreport. (2010) 21:736–9. doi: 10.1097/WNR.0b013e32833bfd36
85. Nieuwenhuis S, Aston-Jones G, Cohen JD. Decision making, the P3, and the locus coeruleus-norepinephrine system. Psychol. Bull. (2005) 131:510–32. doi: 10.1037/0033-2909.131.4.510
86. Kamarajan C, Pandey AK, Chorlian DB, Manz N, Stimus AT, Bauer LO, et al. Reward processing deficits and impulsivity in high-risk offspring of alcoholics: a study of event-related potentials during a monetary gambling task. Int. J. Psychophysiol. (2015) 98(2 Pt 1):182–200. doi: 10.1016/j.ijpsycho.2015.09.005
87. Pornpattananangkul N, Nadig A, Heidinger S, Walden K, Nusslock R. Elevated outcome-anticipation and outcome-evaluation ERPs associated with a greater preference for larger-but-delayed rewards. Cogn. Affect. Behav. Neurosci. (2017) 17:625–41. doi: 10.3758/s13415-017-0501-4
88. Kok A. On the utility of P3 amplitude as a measure of processing capacity. Psychophysiology. (2001) 38:557–77. doi: 10.1017/S0048577201990559
89. Grose-Fifer J, Migliaccio R, Zottoli TM. Feedback processing in adolescence: an event-related potential study of age and gender differences. Dev. Neurosci. (2014) 36:228–38. doi: 10.1159/000358917
90. Colzato LS, van den Wildenberg WP, Zmigrod S, Hommel B. Action video gaming and cognitive control: playing first person shooter games is associated with improvement in working memory but not action inhibition. Psychol. Res. (2013) 77:234–9. doi: 10.1007/s00426-012-0415-2
91. Bakhshani NM. Impulsivity: a predisposition toward risky behaviors. Int. J. High Risk Behav. Addict. (2014) 3:e20428. doi: 10.5812/ijhrba.20428
92. Rieser NM, Shaul L, Blankers M, Koeter MWJ, Schippers GM, Goudriaan AE. The predictive value of impulsivity and risk-taking measures for substance use in substance dependent offenders. Front. Behav. Neurosci. (2019) 13:192. doi: 10.3389/fnbeh.2019.00192
93. Ryan KK, Mackillop J, Carpenter MJ. The relationship between impulsivity, risk-taking propensity and nicotine dependence among older adolescent smokers. Addict. Behav. (2013) 38:1431–4. doi: 10.1016/j.addbeh.2012.08.013
Keywords: internet gaming, addiction, reward processing, stimulus-preceding negativity, feedback-related negativity, impulsivity, ERP, gaming addiction
Citation: Raiha S, Yang G, Wang L, Dai W, Wu H, Meng G, Zhong B and Liu X (2020) Altered Reward Processing System in Internet Gaming Disorder. Front. Psychiatry 11:599141. doi: 10.3389/fpsyt.2020.599141
Received: 26 August 2020; Accepted: 10 November 2020;
Published: 04 December 2020.
Edited by:
Xingchao Wang, Shanxi University, ChinaReviewed by:
Guangheng Dong, Zhejiang Normal University, ChinaCopyright © 2020 Raiha, Yang, Wang, Dai, Wu, Meng, Zhong and Liu. This is an open-access article distributed under the terms of the Creative Commons Attribution License (CC BY). The use, distribution or reproduction in other forums is permitted, provided the original author(s) and the copyright owner(s) are credited and that the original publication in this journal is cited, in accordance with accepted academic practice. No use, distribution or reproduction is permitted which does not comply with these terms.
*Correspondence: Xun Liu, bGl1eEBwc3ljaC5hYy5jbg==
†These authors have contributed equally to this work
Disclaimer: All claims expressed in this article are solely those of the authors and do not necessarily represent those of their affiliated organizations, or those of the publisher, the editors and the reviewers. Any product that may be evaluated in this article or claim that may be made by its manufacturer is not guaranteed or endorsed by the publisher.
Research integrity at Frontiers
Learn more about the work of our research integrity team to safeguard the quality of each article we publish.