- 1Center for Evolutionary Cognitive Sciences, Graduate School of Art and Sciences, The University of Tokyo, Meguro-ku, Japan
- 2Department of Integrative Physiology, Graduate School of Medicine, University of Yamanashi, Chuo, Yamanashi, Japan
- 3University of Tokyo Institute for Diversity & Adaptation of Human Mind (UTIDAHM), Meguro-ku, Japan
- 4University of Tokyo Center for Integrative Science of Human Behavior (CiSHuB), 3-8-1 Komaba, Meguro-ku, Japan
- 5The International Research Center for Neurointelligence (WPI-IRCN), Institutes for Advanced Study (UTIAS), University of Tokyo, Bunkyo-ku, Japan
Traditional neuroscience sees sensory perception as a simple feedforward process. This view is challenged by the predictive coding model in recent years due to the robust evidence researchers had found on how our prediction could influence perception. In the first half of this article, we reviewed the concept of predictive brain and some empirical evidence of sensory prediction in visual and auditory processing. The predictive function along the auditory pathway was mainly studied by mismatch negativity (MMN)—a brain response to an unexpected disruption of regularity. We summarized a range of MMN paradigms and discussed how they could contribute to the theoretical development of the predictive coding neural network by the mechanism of adaptation and deviance detection. Such methodological and conceptual evolution sharpen MMN as a tool to better understand the structural and functional brain abnormality for neuropsychiatric disorder such as schizophrenia.
Introduction
From an evolutionary perspective, the nervous system of animals needs to be highly adaptive to the constant changes of the physical world to increase their survivability. To achieve this, an accurately perceived sensory input and the respective cognitive processing, as well as an effective interaction between them are needed. The animal brain, therefore, forms a dynamic network linking its own body and the external environment (1). This perspective becomes fundamental to many contemporary brain-behavioral models. Perceptual learning, for instance, highlights how our sensory system is shaped and tuned by past experiences. Such exceptional ability allows animals to filter off unwanted sensation (noise) and concentrate on the unexpected information, based on their prior knowledge. This function is termed as prediction in predictive coding theory of the brain, serving as one of its foundational concepts (2–4).
Predictive coding has gained increasing interest in recent decades and appears as a robust underlying mechanism of perceptual learning (3, 5). The notion of predictive coding suggested that the brain continuously generates and updates its predictions to the physical world by integrating the sensory information. In this manner, perception is not a uni-directional projection, but a bi-directional and hierarchical process (see Figure 1). In sensory processing, sensory “prediction” is actively generated by an internal model at the higher-order area which incorporates information from the past (memory), produces and sends information regarding the future to the lower hierarchical cascade. This inferential process naturally allows the implementation of the Bayesian theories, where prior sensory experiences and current sensory input were used to compute the posterior perceptual estimation, that is, the prediction (6–8). At each processing layer, neurons subtract this prediction from the sensory data and register the residual by a “prediction error” signal. In this sense, it is not necessary for an expected event to transmit to the top of the cortical hierarchy (9). Instead, only the information that deviates from predictions is further processed and passed upward to the higher-order cortical area in the form of “error”. The greater the prediction error, the larger the neural response evoked. This prediction error signal propagates through an ascending pathway and updates the subsequent prediction. When the prediction eventually matched with the sensory input, the neural activity induced by prediction error would be suppressed.
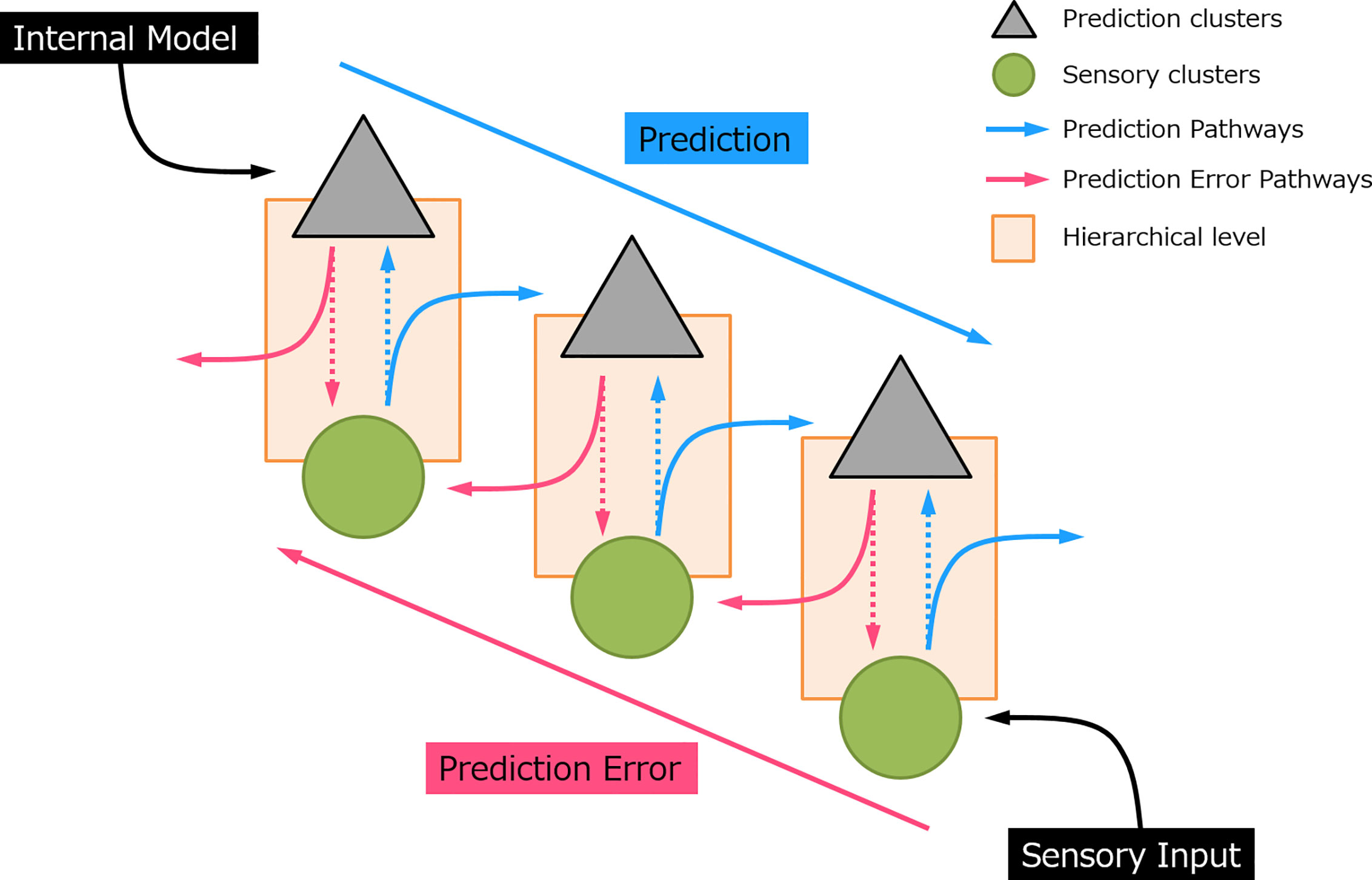
Figure 1 A simplified sensory prediction model. The blue arrow indicates how prediction is updated on the prediction neurons and pass to a lower hierarchical level. The red arrow indicates how prediction error is generated and feedforward to a higher hierarchical level. Each hierarchical level could represent a processing layer, for example, in auditory processing, i.e., auditory cortex, thalamus (medial geniculate body), and midbrain (inferior colliculus), respectively (from top to bottom).
While it may seem easy to assume that one is consciously aware of the role of prediction in everyday decision making (10), it is neither fair nor true. There are many “less obvious” predictions hidden along the sensory system in one’s daily life that are likely to go unnoticed. For example, a goal-directed behavior as simple as grabbing an object first requires the integration of predictions of the visual and interoceptive inputs to ensure the precision of the sensory information, such as the locations of the object and our hand [see (11)]. With these accurate sensory inputs, the brain can produce the corresponding muscle movements via the corticomuscular channel as an end-result. Some literature showed that this succession of neuronal activities can be modulated by the dopaminergic reward system (12, 13).
With such a fascinating theory on sensory perception, scientists put effort into “visualizing” or quantifying prediction and prediction error in order to justify their proposed neural mechanism. This is later achieved by experiments that initiate prediction by the repeated presentation of certain stimuli at a given rhythmic time interval and subsequently stop delivering the predicted stimulus. This paradigm generates a transient neural response in the absence of sensory input, which becomes strong evidence of sensory prediction. For example, by repetitively presenting auditory or visual cues followed by a button press (motor response), the enhanced event-related potential (ERP) could be evoked by the button press even without the actual feedback stimuli (14–16). Using a similar technique of attributing sensory feedback with another sensory cue, pre-activation of the sensory cortex was also observed via functional imaging which is in support of the subjective prediction in sensory input (17).
The notion of predictive coding has been adopted and confirmed by a wide range of neurocognitive computational models (2, 7, 18–21). Its extensive application also provides valuable insights into the investigation of many psychiatric and developmental disorders such as schizophrenia and autism (22–24). For instance, abnormalities (hyper/hypo-responsivity) in sensory processing were hypothesized to be responsible for several symptoms of psychiatric disorders (25). Disruption of the exteroceptive prediction may lead to visual and auditory hallucinations while the disturbances of interoception might also give rise to emotional disorders (20, 26, 27). In this paper, we seek to review the neural basis and empirical findings of sensory prediction under the predictive coding framework. The majority of experimental and clinical evidence for sensory prediction was provided by the mismatch negativity (MMN) paradigm in which the prediction error fluctuation underlies the breaking of a stream of acoustic sounds. Therefore, we seek to give an extensive review to such task and discuss its application in neuropsychiatric disorders using the example of schizophrenia.
Sensory Prediction in the Visual System
If we look around the living room where we are staying in, we would not be surprised to see objects such as chairs, desks, lamps, or windows. However, the presence of a wild animal or a stranger in the room would undoubtedly shock and alert us. Predictive coding theory suggested that the higher cortical area would constantly produce internal models of the surroundings and make expectations on what objects we would see. This information would be passed to the lower order neural structure, forming predictions on visual features as simple as colors and shapes (28). In this example, assuming that we live alone, the prediction error is generated at the hierarchical level where we identify the objects we see as human or animal (which are objects that we do not expect to see in our living room). On the other hand, if we live with our family and pets rather than alone, the upward propagated prediction error might appear later at a higher level when our brain successfully identifies those objects as “intruders”. This suggested visual perception is not only driven by the bottom-up sensory input but also influenced by the top-down prediction model (29).
The predictive ability in the visual system can be observed as early as in the retina. Previous studies showed that retinal ganglion cell had enhanced sensitivity to unpredicted changes and suppression for predicted stimulations in various domains of visual stimuli including orientation, spatial frequency, and temporal structure (30). This property of receptive fields is consistent with the notion of predictive coding. At the lower hierarchical level, prediction of the visual system can also be seen from salience detection, which is guided by a “centred-surround prediction” mechanism (3, 31). In a simple visual search task, a salient visual feature (e.g., color, motion, and orientation) can be spotted irrespective to the number of surrounded visual distractors (32). Since a pixel in the natural scene tends to correlate with the neighboring pixels, the brain is able to form a spatial prediction on the centred pixel by the weighted linear sum of the surroundings (3). A salient stimulus is inconsistent with the internal computational model, which produces an error detection signal that increases along the visual hierarchy via retina (33, 34), lateral geniculate nucleus [LGN; (35)], striate and extrastriate cortex (3), and lateral intra-parietal cortex (36). This signal passes along the visual hierarchy, reaches the oculomotor system, and re-orients the eye-gaze to the location with high salience (37). Such hierarchical communications are well-supported by the feedforward and feedback connections in the cortico-cortical and subcortical-cortical network which have been described in detail in previous literatures (38–40).
The predictive power of the visual system also compensates the limited capabilities per se. For example, human retina can only encode two-dimensional information. In addition to the retinal disparity produced by our two visual fields, visual experiences such as an object’s relative size and aerial perspective also contribute to our 3-D vision by providing the perception of depth. Besides, the number of neurons and energy required for metabolic activities restrict the computational power (e.g., sampling rate and speed of signal transmission) of the visual system. Visual prediction, therefore, becomes crucial in motion perception. By sampling an object’s movement within hundred milliseconds, the visual system is capable to predict the motion pathway of the object which provides the visual ability to pursue an object moving in constant velocity (41–43).
A more active form of visual prediction happens when we voluntarily focus on a location in space and enhance our visual sensitivities on that specific location. This ability to guide our spatial attention is based on one’s prediction on where a task-relevant cue might be presented. Eye movement signals in frontal eye field (FEF) can also enhance the sensory gain in the visual cortex [for example, area V4; (44, 45)]. Previous studies have shown that neuronal activity can be modulated by the prediction of stimulus onset (46, 47). By conditioning a visual target on a certain location with a sensory cue after a period of time, a monkey could learn the conditioned patterns of the visual events by making eye movements to the target with a precise temporal pattern (47). An increased neuronal response could be observed from their parietal cortex when the visual target was absent from the conditioned location unexpectedly. This paradigm is similar to the terminology of the oddball experiment where a “deviant” event elicits an elevated neural response, which is also known as MMN, compared to the “standard” (48). This visual MMN signal can be suppressed by silencing the somatostatin-containing GABAergic interneurons (SOMs) pharmacogenetically (49).
Overall, the empirical evidence of the visual system appears to support the notion of predictive coding. In the following sections, the sensory prediction in the auditory system would be described in more detail.
Sensory Prediction in the Auditory System and Mismatch Negativity
Similar to the visual counterpart, the auditory prediction is vital to cognitive functions, including language, and speech processing (50). In spoken word recognition, it has been demonstrated that there are top-down predictions in the lexical-semantic system. For example, studies showed that replacing the last syllable of a word such as “formula” to “formubo” can facilitate an enhanced evoked response at superior temporal gyrus (STG) on the listeners (51). Another experiment showed that a non-Finnish language vowel presented in a train of Finnish vowels could elicit a pre-attentive neural response at the left auditory cortex (AC) (52). Predictive coding hypothesized that these neural responses originated from the prediction error signal when the expected vowels unmatched with the actual acoustic signal somewhere in a higher cortical area of the linguistic hierarchy. Music perception was also hypothesized to be inferred by our experience and knowledge on music (e.g., melodic, temporal, and harmonic structure) while we are listening to a stream of musical tones (53).
The neural basis of prediction and prediction error, as well as the evidence of the predictive coding hierarchy in the auditory domain, was explored most extensively by the studies of auditory MMN. The MMN is a negative deflection of the ERP elicited by an abrupt change of auditory stimulus after the repetition of the same sound, indexing the pre-attentive sensory processing (54). MMN is not a manifestation unique to the auditory modality. It can also be elicited by visual stimuli using a similar experimental setup [for more detail, please see the reviews of vMMN from (55) and (56)]. MMN reduction is one of the most robust biomarkers in patients with schizophrenia (57). Therefore, the neural mechanism underlying MMN has raised significant interest over the years.
The MMN in a classical oddball paradigm was measured by electroencephalogram (EEG) when an infrequent deviant (d) sound was interspersed among a train of repetitive standard (s) sounds (e.g., s s s s s s d s s s s s d s s s …) (58). The deviant tone can be different from the standard in terms of frequency, duration, or intensity. Traditionally, the MMN is obtained by subtracting the event-related response evoked by the standard tone from the response of deviant tone. MMN experiment can be carried out in the absence of attention, though some studies showed that the MMN response is attenuated without the involvement of attention (59).
Neural Basis of Mismatch Negativity Under the Predictive Coding Framework
The auditory MMN often peaked at the frontocentral scalp that inverts polarity at the posterior-temporal regions (60). Dipole analyses on EEG and magnetoencephalogram patterns revealed that the neural generator of this response was located at the AC which lies along the STG in each hemisphere (61, 62). Whether the deviant was presented as a mismatch in frequency, duration or intensity shifted the dipole source over the supratemporal cortex (63–66). Other regions have also been reported to contribute to MMN in an auditory oddball paradigm, for example, frontal cortex [FC; (67–71)], subcortical structures such as thalamus (21, 72, 73) and hippocampus (74, 75). Despite decades of clinical and experimental research, the role of different neural structures and the actual neural mechanism underlying MMN remained controversial. Two main hypotheses have been most extensively studied, namely, “adaptation” and “sensory memory/predictive coding” (76).
Adaptation, also known as stimulus-specific adaptation (SSA), was interpreted as a lower-level inhibitory neural mechanism in response to repetitive stimulations (77). This property was observed at cortical and subcortical structures such as the AC, thalamus (medial geniculate body, MGB), and midbrain (inferior colliculus, IC) [see (72, 76–78)]. Some researchers argued that there is a bottom-up thalamocortical network where the AC is modulated by a synaptic depressive input projected from the thalamus (77, 79). In this case, MMN is a derivative of the N1 suppression when we subtracted the standard-evoked potential form the deviant-evoked potential. The subsequent negative deflection of the deviant signal simply represented a non-adapted evoked response, highlighting a “release” from the suppression (see Figure 2). However, this hypothesis failed to explain the MMN findings in some oddball experiments. For example, if the deviant in an oddball paradigm is replaced by an omission stimulus, the absence of the expected tone is still able to induce an MMN when no adaptation could have occurred (80, 81). Additionally, using a local/global paradigm, studies showed that the violation of local and global rules would elicit two different MMN signals at the AC and FC, respectively (82). These findings suggested that there must be a higher-order process encoding the patterns of sequence and producing an enhanced response when the encoding rule is violated (83).
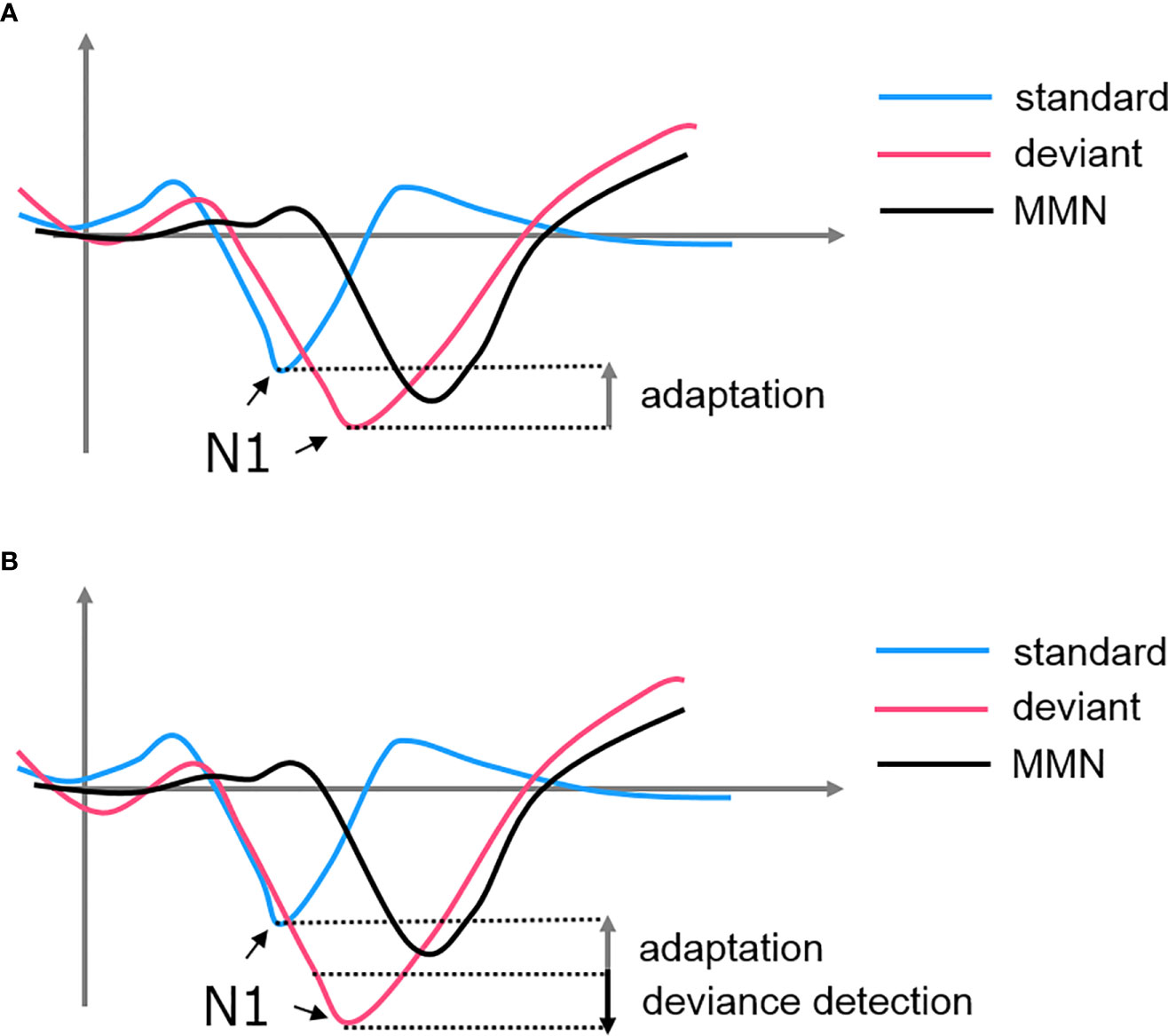
Figure 2 The formation of MMN from the subtraction of standard-evoked potential from deviant-evoked potential. (A) SSA hypothesized that the MMN is simply a derivative of the attenuated N1 of the standard. (B) Predictive coding hypothesized that both adaptation of the standard and deviance detection of the deviant contributes to the MMN.
In contrast, predictive coding suggested that neurons in the thalamocortical network have a more sophisticated cognitive processing to gather sensory information. The standard stimulus, which is a series of repetitive sounds/tones, would be encoded as a sequence of patterns by the brain, forming a “prediction” for the upcoming stimuli. When the sensory data is matched with the prediction, the prediction error is minimized, leading to the neural adaptation. Since the prediction has been shaped by the standard stimuli, the deviant as a distinctive sensory input would produce a considerable amount of prediction error. The MMN is, therefore, an electrophysiological marker reflecting the level of prediction error when an unexpected stimulus (deviant) is presented (see Figure 3). As a limitation, this simplified model did not address the firing patterns of the prediction neurons. Therefore, the “prediction error signal” might indeed represent the ratio between prediction error and prediction signal or the net electrophysiological activities of these two clusters of neurons.
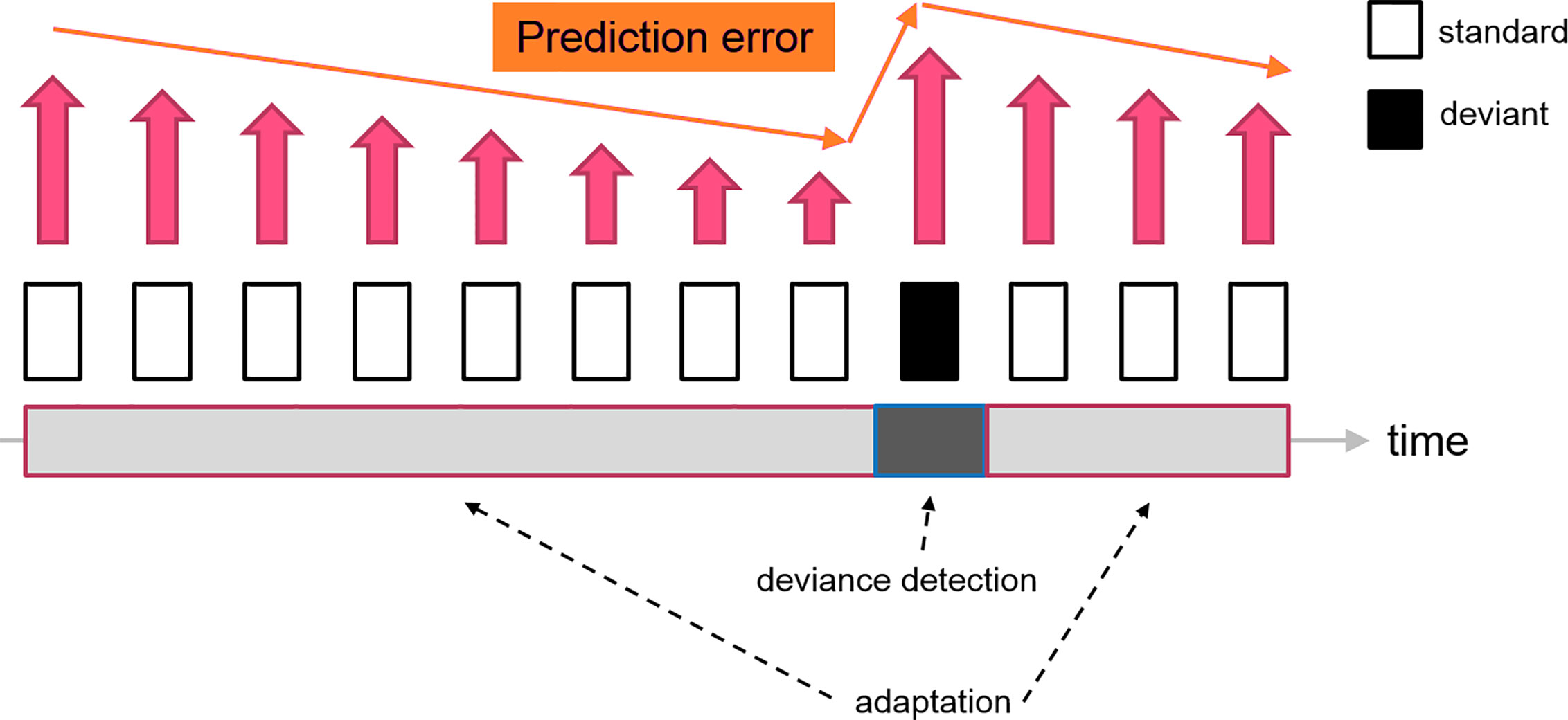
Figure 3 A diagram indicating the change of prediction error signal during a classical oddball experiment. The repetitive presentations of the standard stimuli reduce the prediction error signal (decrease neuron firing) feeding to the higher-order area, which leads to the “adaptation” effect. The subsequent deviant tone elevates the prediction error, which generates the deviance detection input (increase neuron firing). Therefore, the resultant MMN (deviant - standard) consisted of two neural mechanisms driven by the fluctuation of prediction error signals.
The prediction signal might only be visible as N1 during the adaptation period when the prediction error is theoretically minimized. With an early latency (peaked around 100 ms), adaptation is expected to be localized at the lower level of the prediction hierarchy formed by the subcortical-cortical network, involving mainly the belt area of MGB and IC with both the belt and core areas of the primary AC (A1) (84, 85). Previous studies revealed that the AC can have both excitatory and inhibitory effects on the MGB (86) and IC (87). Thus, the AC can suppress the predicted information from approaching the cerebral cortex by inhibiting the activities of the thalamus and midbrain when prediction error input is reduced (84, 88).
In addition, neurons at the IC with strong SSA properties appeared to receive extensive inputs from the AC while those with no/less SSA properties mainly receive input from the brainstem nuclei (89). Studies using a cooling technique to deactivate the cortical activity on animals also discovered that the SSA properties of certain neurons at the IC and MGB would be reduced while certain proportions of neurons still retained their SSA sensitivity (72, 84). Collectively, adaptation as a key component of predictive coding and MMN, appeared to be modulated by a lower order AC-MGB-IC network which continuously interacts with each other, maintaining the efficiency of sensory processing.
Deviant detection (DD) is another core component of the MMN signal. Using single-neuron recording, Parras, and colleagues demonstrated (90) on anaesthetized rats that the AC-MGB-IC network contributes to both the adaptation and deviance detection of the MMN signal. The prediction error activities elevated from IC to MGB to AC and from lemniscal to non-lemniscal regions. Other subcortical regions such as the cerebellum (91) and basal ganglia (92) were also known to be associated with the sensory prediction processing. However, DD has mainly been linked with the higher-order processing in predictive coding based on the temporal profile of the MMN, where the lower order response activates earlier (~100 ms) at the AC while the higher-order DD signal becomes visible in a later time window at the frontal area [100–200 ms after the stimulus onset; (93–95)]. The P3a followed by the frontal MMN also suggested that there could be a switch of attention toward the deviance (96).
Additional studies revealed that deviance detection was associated with the higher cortical area. An electrocorticographic (ECoG) study showed that deviance detection signals in the cerebral cortex are localized to the lateral STG and FC in humans (97). Other studies argued that the PFC (e.g., ventral prefrontal regions) contributed to DD when the sensory input projected from the AC (anterior belt) does not match the existing memory (98). The role of the frontal region in this sort of feedback mechanism is well-supported by the studies of non-conscious patients. For instance, patients in the vegetative state showed a reduction in feedback modulation with the sensory cortex while their feedforward functioning was still maintained (99, 100).
To sum up, predictive coding provided a comprehensive explanation to the temporal and spatial profile of MMN by the fluctuation of the prediction error signal, which was modulated by the cortical and subcortical network in a hierarchical manner. Associating adaptation and DD with lower-order regions and higher-order regions respectively were tentative, with no strict boundary between each neural hierarchy. Intuitively speaking, it seems logical to think that adaptation serves the function of gatekeeping at the early sensory pathway to prevent information overloading. Without excessive data input, the higher-order sensory cortex should perform the DD more efficiently. Since predictive coding assumes the same communication mechanism, formed by prediction and prediction error, for each neural structure, the functional specification of adaptation and DD might be achieved by the differences in neural properties and possibly the proportion of each type of the neurons along the neural hierarchy.
Various Forms of Oddball Paradigm
The deviant evoked response in a classical oddball paradigm is known to be influenced by both adaptation and deviance detection. By introducing various forms of oddball paradigm and control task, literatures found that the evoked response, more precisely speaking, could reflect the cognitive process of adaptation and deviance detection separately. In the following section, we briefly describe 7 major MMN paradigms and compare their effectiveness in identifying the underlying mechanism (see Figure 4).
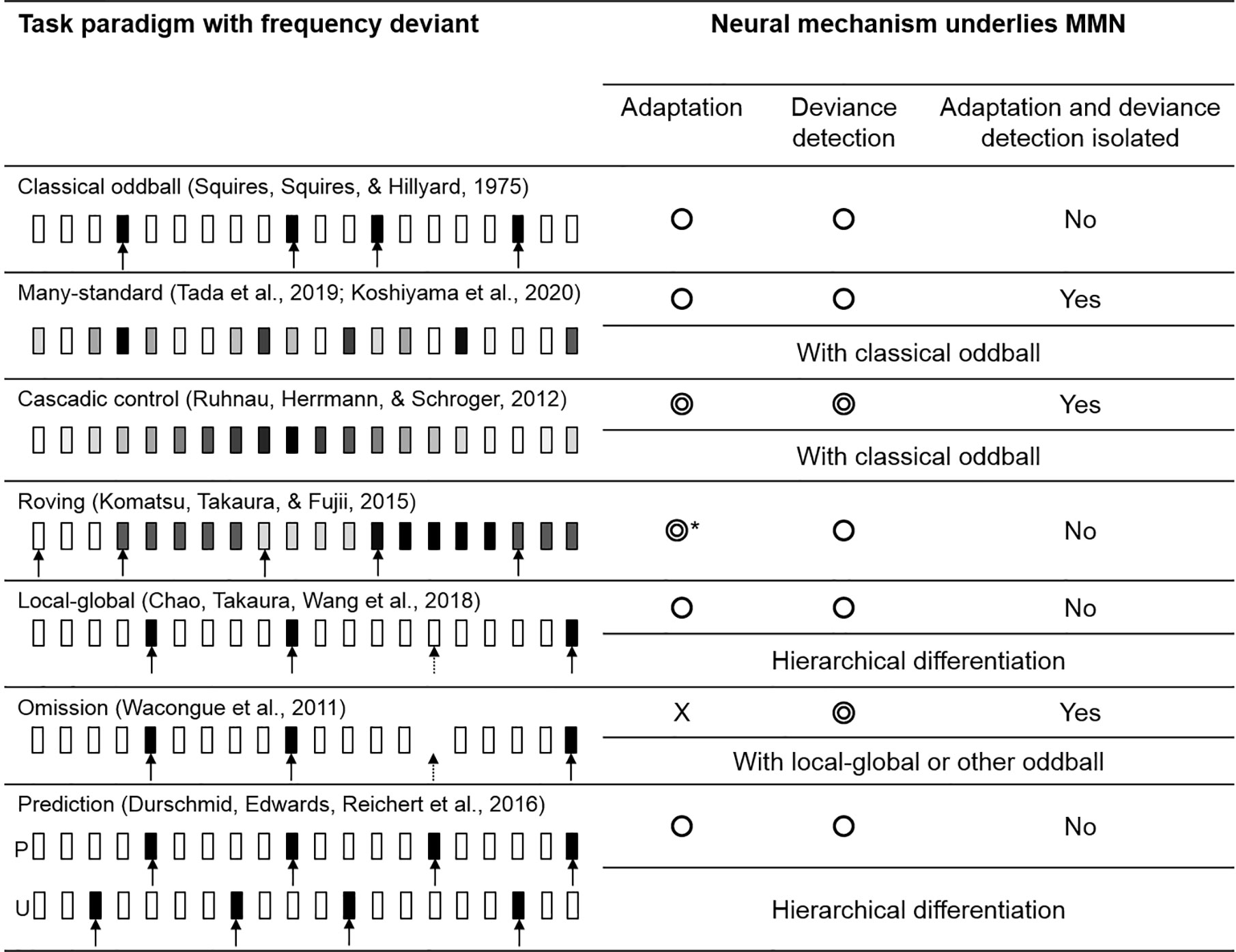
Figure 4 The variations of oddball paradigm. The circle symbols indicated whether the MMN would be influenced by adaptation or deviance detection. The double circle indicated that the MMN would give a cleaner representation of adaptation/deviance detection. Classical oddball coupling with many-standard and cascadic-control allows the isolation of deviance detection from adaptation. Without an actual auditory input, an omission deviant would elicit a signal that “free” from adaptation, while MMN in other paradigms would be influenced by both mechanisms. *Roving paradigm allows the comparison of MMNs evoked by different length of standards in which adaptation effect could be investigated. The greyscale indexed the pitch of the stimulus; the arrow indicated the deviant tone (the dashed arrow indicated the global deviant).
Classical Oddball
Traditionally, the MMN in the classical oddball paradigm is obtained by subtracting the standard-evoked potential from the deviant-evoked potential. However, the adaptation effect induced by the standard tone was known to cause a reduced auditory N1. By subtracting a standard evoked response with a reduced N1 (peaked at 100 ms) from the deviant evoked response (peaked at around 100–200 ms), the resultant MMN could be contaminated by the N1 and hence overestimated.
Many-Standard Control
The many-standard paradigm was developed as a control paradigm to eliminate the adaptation effect (N1) from MMN. A simple “many-standard” control consisted of a sequence of tones [e.g., varied in pitch but fixed duration, loudness and onset-to-onset intervals (78, 101–104)]. Two of the tones were matched with the pitch of the standard and deviant tone using in a classical oddball respectively, while the rest of tones have a random pitch. A many-standard control presents the tones in pseudo-random order, creating a sequence with no known regularity which prevents the formation of adaptation among the participants.
Therefore, by comparing the event-related response evoked by the deviant tone in the classical oddball paradigm with the response elicited from the tone with the same pitch but presented in the many-standard sequence (without the reduced N1), researchers should be able to obtain the “adaptation-free” MMN that represents the deviance detection process. On the other hand, the comparison between the neural response evoked by the “standard” in classical oddball and the many-standard paradigm should identify neural signals that indicate the adaptation effect. This also allows the comparison on two identical tones, eliminating any confounding effect produced by the acoustic features (i.e., pitch for a frequency deviant) rather than the abstract rule of the sequence.
The disadvantage of the many-standard control is that the randomness of the sequence would generate a considerable amount of prediction error according to the predicting coding theory. Therefore, the comparison with the oddball sequence may give an underestimated deviance detection effect and an overestimated adaptation effect.
Cascadic Control
With the limitation of a many-standard control, an additional sequence coupling with the classical oddball and many-standard paradigm, known as “cascadic-control” was introduced (105). The cascade sequence consisted of a regular train of tones in increasing or decreasing frequencies. The target control tone is hidden in a predictable sequence which suppresses the generation of prediction error from the control sequence. The descending or ascending cascade control corresponds to the oddball deviant that has a decreased or increased frequency respectively. The preceding tones of the control and oddball sequence are also identical, which makes the cascade a better control compared to many-standard to identify the deviance detection process.
Roving
The roving paradigm contains multiple trains of tones in various frequencies (106–108). Each train consists of a random amount of identical tones separated by regular inter-stimulus-intervals. The repetition within each train of tones eventually leads to an adaptation effect; thus, the last tone of the block can be viewed as a “standard” as in the oddball paradigm. The first tone of a train, on the other hand, indicates a switch of frequency, making it a natural deviant tone with the same physical properties as the standard. Similar to standard oddball, the MMN is indicated by the comparison of standard and deviant evoked response. In addition, the roving paradigm can examine the build-up of the adaptation by comparing the MMN caused by the different numbers of repetitions between the deviant and standard tone (109).
Local-Global
The local-global paradigm typically consists of two sets of auditory stimuli (82, 110). A set of tones, named as local standard, contains a series of identical tones (e.g., AAAAA). The repetition of tone A is the local rule of this sequence which makes the sequence AAAAA fitting the internal rule. Another set of tone, named as local deviant, also contains a train of identical tones but the last one is replaced by a different tone (e.g., AAAAB). Therefore, the internal rule of repeating tone A in this sequence is violated by the last tone. By presenting the local deviant repeatedly (e.g., AAAAB AAAAB AAAAB…), our brain can identify such sequence as a global-rule, which makes the local deviant a global-standard. If a rare number of local standard (AAAAA) is mixed randomly into the train of local deviant (e.g., AAAAB AAAAB AAAAB AAAAA AAAAB AAAAB AAAAB AAAAB AAAAB AAAAB AAAAB AAAAA AAAAB …), the local standard here then appears as violating the global rule, which turns the local-standard as a global-deviant. By interchanging the proportion (probability) of the local-deviant and local-standard, their roles in the global-rule can be swapped.
This paradigm introduced two types of temporal regularities, that is, the local rule (tone-to-tone transition) and the global rule (sequence-to-sequence transition). The detection of violating a local-rule was hypothesized as lower-order processing while the global-rule was hypothesized to be processed at the higher-order cortical area. This fits the paradigm into the predictive coding manner which proposes a hierarchical communication network. Chao and colleagues showed that the local prediction errors were generated in the AC while the global prediction errors were generated in the FC (82). Their ECoG connectivity analyses revealed that local prediction error signals were fed forward to the AC and that global prediction error signals were fed forward to the FC. A feedback component from FC to AC for global prediction errors was found as well.
Omission
All oddball paradigms described above have both standard and deviant tones. An omission paradigm often couples with other oddball paradigms as an additional sequence where an omission deviant replaces the typical frequency deviant (110). In other words, the expected event is omitted (no tone) followed by a train of standard tones. Since no actual acoustic input is presented, the evoked response cannot be sensitive to the adaptation effect, which allows the isolation of deviance detection signal.
Prediction
The prediction task used by Durschmid and colleagues (111) is a variation of the local-global paradigm, formed by two types of blocks, the predictable (P) and the unpredictable (U). The fully predictable blocks consist of the regular repetitions of the local-deviant (i.e., AAAAB AAAAB AAAAB …), where the location of the deviant tone (B) can be fully predicted (after the presentation of 4 As). In contrast, the local-deviant tone (B) does not follow any global rules in the unpredictable blocks. It appears between 3 and 7 repetitions of the local-standard tone (A). This design also allows the investigation of local and global rules hierarchically, fitting the scope of predictive coding.
Recent advancement of methodological (oddball paradigms) and conceptual (predictive coding) development in MMN has enabled us to better understand the functional and anatomical mechanism underlying it, namely, adaptation/deviance detection or hierarchical segmentation. This provides a valuable new perspective to the interpretation of existing neurological data on MMN and in generating hypotheses about the pathology of certain psychiatric disorders where MMN has long been used as a biomarker. Considered the widely diverse MMN findings and different pathologies associated with each psychiatric disorder, we decided to use one clinical condition as an example to illustrate how such new perspective could be applied to the existing literature and future studies. Schizophrenia has been chosen because the classic MMN, which is said to index the pre-attentive perceptual deficit, is perceived as a valid biomarker in schizophrenia.
Mismatch Negativity in Schizophrenia
Reduced auditory MMN (104, 112–115), magnetic auditory MMN (116, 117) and visual MMN (118, 119) in a classic oddball paradigm is commonly observed on patients suffered from psychotic disorders. MMN also has the greatest effect size as a biomarker for schizophrenia amongst P50, N100, and P300 (120). Remarkably, there were significant associations between MMN amplitudes and psychotic symptom severity in cross-sectional comparisons (121–124), which inspired the possibility of using MMN amplitudes for clinical prognosis. Some research groups showed that an increase in MMN amplitude predicted the clinical remission of people with ultra-high risk for psychosis (UHR) (125, 126). Another longitudinal study revealed that MMN deficits might be associated with the negative symptoms and functional outcomes of the patients with schizophrenia (127). Unfortunately, these studies did not control medication dosage as well as other medical care and treatment as confounders, therefore the actual contribution of MMN can only be evaluated in larger longitudinal samples with appropriate confounding control.
Several studies have attempted to investigate the association between MMN amplitude and the trajectory of psychosis development: adolescent preclinical psychotic-like experiences (PLEs), UHR, first-episode psychosis (FEP), first-episode schizophrenia (FESZ), and chronic schizophrenia. Cross-sectional studies have shown that MMN amplitude evoked by duration deviants (dMMN) was significantly reduced in earlier clinical stages of psychosis - UHR and FEP, compared to healthy controls, while such differences were not observed using frequency deviants (fMMN) (113, 121, 128–134). Reduced dMMN amplitude can also be observed on adolescents having PLEs (135) and people with UHR who later converted to psychosis (130). The attenuation of a double (duration + frequency) deviants evoked MMN also predicted the conversion of UHR to FEP (136). Collectively, dMMN attenuation can be observed across different stages of psychosis, making it a valuable trait marker.
However, the association between MMN and the development of psychosis are still lacking in support from longitudinal investigations. Some longitudinal findings revealed a progressive reduction of MMN amplitude in FESZ (137, 138) but not in chronic schizophrenia (139). Salisbury and colleagues also found a progressive reduction of fMMN alongside the volume decrease at the left Heschl’s gyrus for patients with schizophrenia instead of bipolar disorder and healthy controls (138). However, these findings are somewhat controversial with no group difference nor progressive reduction being seen between UHR, FEP, and control groups (115). A similar trend has been reported for dMMN (140) but not in others (115, 141). Whether this controversy originates from the difference in measurement procedure, recruitment, the definition of clinical stages or follow-up period remained unanswered.
Compared to schizophrenia, there were fewer examinations on MMN for other psychiatric disorders. Cross-disease comparisons have shown that patients with bipolar disorder had a decreased dMMN amplitude similar to those with schizophrenia compared to healthy controls (123). Another studied showed a contradictory finding in which their patients with bipolar disorder and major depressive disorder had no significant dMMN amplitude reduction (142). A review of the MMN study on the bipolar spectrum has proposed that bipolar disease could lead to an intermediate level reduction on MMN in-between those with schizophrenia and the healthy controls (143) although a systematic model is yet to be developed due to the unclear relationship between MMN and the subtypes (as well as medication status) of bipolar disorder (144, 145). MMN for the major depressive disorder had also been investigated, yet again, the results were inconsistent across the literatures (146–148).
Recent cross-disease multi-modal studies have proposed that the deficits in MMN amplitude could be driven by the structural change at specific brain regions rather than the disease pathology. This idea is supported by the intermediate dMMN amplitude decrease of patients with bipolar disorder compared to patients with schizophrenia and healthy controls, while the amplitude was associated with the cortical thickness of the right STG (149), suggesting that the MMN amplitude could rather indicate the deficit of higher-order auditory functions in the STG irrespective of the spectrum of the psychiatric disorders. A functional MRI study showed that the deviant-induced BOLD signal for patients with schizophrenia was reduced in a few cortical areas and subcortical areas (IC, thalamus, AC, and PFC) and those with major depressive disorder only had the reduced BOLD on the PFC (73). Some researchers argued that there could be broader pathophysiology for MMN (143), and therefore, MMN should not be used as a biomarker exclusively for schizophrenia. With our understanding of the formation of MMN using predictive coding, it appears that MMN can index different psychopathology that are shared across different psychotic and related disorders.
Pathology of Schizophrenia From a Predictive Coding Perspective
The classification of most psychiatric disorders nowadays such as the Diagnostic and Statistical Manual (DSM-V) and International Classification of Diseases (ICD-10) diagnoses the presence or absence of a disorder based on a list of categorical symptoms. For example, schizophrenia is characterized by positive symptoms such as delusions and hallucinations.
Based on predictive coding theory, some researchers argued that exaggerating or overly precise prior beliefs renders a sensory noise into an organized and reasoned percept (150). Since the precision of the sensory data and the prior belief (memory) would both be inferred to the posterior (updated perception), a relatively high prior belief would reduce the influence of the sensory data on the resultant perception. A recent study showed support to this notion by building prior belief through the repeated presentation of a visual cue concurrently with an auditory tone (151). They demonstrated that it was easier for the visual cue (in the absence of the actual tone) to induce hallucinatory tones as well as activations in certain cortical areas, such as AC, posterior superior temporal sulcus and anterior cingulate cortex, on psychotic patients who have hallucination symptoms. However, this appears as contradictory to some early studies of schizophrenia, where patients are less susceptible to hollow-mask illusion (a concave mask creating an illusion of convex face) (152, 153). Using a dynamic causal model, the authors showed that patients with schizophrenia had a stronger feedforward modulation from the primary visual cortex to the lateral occipital complex, implying a weak inference from learned prior beliefs compared to the sensory input. Although the above two examples may highlight two completely different neuropathology, the over/under reliance on prior belief could co-exist through a failed prediction error feedback mechanism.
The failure of prediction error feedback could distort perceptions by disrupting both the top-down modulation and bottom-up sensory projection. Such deficit of patients with schizophrenia in prediction error was more clearly demonstrated by MMN studies using other forms of oddball paradigm. In a local-global oddball study, patients with schizophrenia showed reduced MMN to both the local and global deviant compared to healthy control (154). Although this paradigm could not distinguish repetition suppression and deviance detection from one to each other, it revealed that the prediction error deficit of schizophrenia covers both the lower-level and higher-level processing layer. The reduced MMN was also shown in another study using roving paradigm (109, 155). Interestingly, the patients’ MMN only become visible with increasing repetitions of the standard tones. With a longer sequence of standard, MMN is believed to be predominated by the “release” of SSA-sensitive N1, which suggested that the SSA function for schizophrenia remains intact. This finding is consistent with other studies using many-standard control and omission deviant, which revealed that the prediction error for schizophrenia was mainly influenced by deviance detection rather than repetition suppression (154, 156, 157).
Patients with schizophrenia also had a progressive volume reduction in STG (158), a site known to associate with deviance detection (97). Previous research has also revealed that MMN is associated with the N-methyl-D-aspartate glutamate (NMDA) system (159–162). For example, an animal study showed that ketamine as an NMDA-antagonist can suppress both the local- and global-deviant triggered MMNs (163). Such association between MMN and NMDA, however, is only found in the deviance detection process, but not adaptation (102). This appeared to be consistent with the NMDA receptor (NMDA) hypofunction model on schizophrenia, which proposed that the pathology of schizophrenia involved a dysfunction or a blockage of the NMDA receptor (164–168).
It is worth mentioning that the hyperactivity in the dopaminergic system is tied to positive symptoms of schizophrenia such as verbal hallucination and persecutory delusion (169, 170) since some of the positive symptoms can be induced by dopamine stimulants (e.g., methamphetamine administration) and most of the antipsychotics that act as D2 receptor (D2R) antagonists for positive symptoms. Aberrant reward response was often associated with the above positive symptoms; thus, the dysfunction and imbalance of D1 receptor (D1R) and D2R were presumably linked to reward prediction error deficit (171–174). Such deficit prevents our brain from updating and correcting the internal prediction model that is modulated by reward which leads to false or hallucinatory percepts (169).
Previous studies have hypothesized that the dopaminergic pathway in reward system relies on the valence coding function of D1R and D2R: the former is responsible for the positive, while the latter is for the negative valence (175). Considering the clinical pharmacological findings of D2R and the functions of serotonin, positive and depressive symptoms were once expressed as the imbalance of D1R and D2R functions, with serotonin as a modulator for dopamine signalling (176). However, a recent study found that during the learning process, D1R in the nucleus accumbens and D2R were responsible for the generalization and discrimination phrases respectively (177). The excessive D1 and insufficient D2 activation may lead to overgeneralization that results in false belief and delusions, whereas the opposite may inhibit beliefs and prediction.
Collectively, we demonstrated how the impaired function of prediction contributes to psychiatric disorder/symptoms using schizophrenia as an example from the predictive coding perspective. The deficit of deviance detection in psychotic disorder as shown in MMN studies indicated that psychotic symptoms could originate from the failed sensory prediction located at the higher cortical level. Such impairments could be associated with the dopaminergic system that would stop sensory cues to be encoded into experiences and beliefs (172), and thus disrupting the sensory prediction scheme, causing delusions or other positive symptoms.
Conclusion
Predictive coding models perceive MMN as an indicator of neuronal prediction error in a hierarchical structure. Although this model may have overstated the actual neural responses of prediction error, it provides a simple working model for researchers to concisely examine the functional or anatomical impairment of different neuropsychiatric population. Given a large number of relevant studies, we believe that in the future of the field, efforts should be made to obtain knowledge with more in-depth “details” instead of staying with its “simplicity” and “elegance”. Since prediction and prediction error were hypothesized to dynamically interact at each hierarchy, an obvious future direction could focus on the prediction signal. This could be achieved by isolating the adaptation mechanism from MMN using the classical oddball paradigm in combination with the many-standard control and cascadic control in which prediction error is minimized. More importantly, researchers seemed to accept the logic of neural arithmetics (i.e., prediction error = sensory input - prediction), despite the fact that how such computation could be carried out at each neuronal level are completely unknown. Considered the cost of monitoring the neural activities at the cellular level, refining and utilizing oddball paradigms such as local-global or prediction paradigm to achieve hierarchal segmentation appears as a sensible solution to unveil the full picture of sensory prediction.
Author Contributions
CF and WL contributed to writing the draft manuscript. TU and SK contributed to conceptualization of this review and reviewing the manuscript. All authors contributed to the article and approved the submitted version.
Funding
This research was supported by the Agency for Medical Research and Development (AMED) under grant numbers JP20dm0207069 and by the Japan Society for the Promotion of Science (JSPS) KAKENHI grant numbers 19H03579 and 19H04878. This study was also supported by the University of Tokyo Center for Integrative Science of Human Behavior (CiSHuB) and the International Research Center for Neurointelligence (WPI-IRCN) at the University of Tokyo Institutes for Advanced Study (UTIAS).
Conflict of Interest
The authors declare that the research was conducted in the absence of any commercial or financial relationships that could be construed as a potential conflict of interest.
References
1. Beer RD. The dynamics of brain–body–environment systems: A status report. In: Calvo P, Gomila T, editors. Handbook of Cognitive Science: An Embodied Approach. San Diego: Elsevier (2008). p. 99–120. doi: 10.1016/B978-0-08-046616-3.00006-2
2. Friston K, Kiebel S. Predictive coding under the free-energy principle. Philos Trans R Soc Lond B Biol Sci (2009) 364(1521):1211–21. doi: 10.1098/rstb.2008.0300
3. Rao RP, Ballard DH. Predictive coding in the visual cortex: a functional interpretation of some extra-classical receptive-field effects. Nat Neurosci (1999) 2(1):79–87. doi: 10.1038/4580
4. Spratling MW. A review of predictive coding algorithms. Brain Cognit (2017) 112:92–7. doi: 10.1016/j.bandc.2015.11.003
5. Friston K. Does predictive coding have a future? Nat Neurosci (2018) 21(8):1019–21. doi: 10.1038/s41593-018-0200-7
6. Aitchison L, Lengyel M. With or without you: predictive coding and Bayesian inference in the brain. Curr Opin Neurobiol (2017) 46:219–27. doi: 10.1016/j.conb.2017.08.010
7. Lee TS, Mumford D. Hierarchical Bayesian inference in the visual cortex. J Opt Soc Am A Opt Image Sci Vis (2003) 20(7):1434–48. doi: 10.1364/JOSAA.20.001434
8. Weilnhammer V, Stuke H, Hesselmann G, Sterzer P, Schmack K. A predictive coding account of bistable perception - a model-based fMRI study. PloS Comput Biol (2017) 13(5):e1005536. doi: 10.1371/journal.pcbi.1005536
9. Friston KJ, Stephan KE. Free-energy and the brain. Synthese (2007) Dec159(3):417–58. doi: 10.1007/s11229-007-9237-y
10. Bubic A, von Cramon DY, Schubotz RI. Prediction, cognition and the brain. Front Hum Neurosci (2010) 4:25. doi: 10.3389/fnhum.2010.00025
11. Shadmehr R, Smith MA, Krakauer JW. Error correction, sensory prediction, and adaptation in motor control. Annu Rev Neurosci (2010) 33(1):89–108. doi: 10.1146/annurev-neuro-060909-153135
12. Cheer JF, Aragona BJ, Heien ML, Seipel AT, Carelli RM, Wightman RM. Coordinated accumbal dopamine release and neural activity drive goal-directed behavior. Neuron (2007) 54(2):237–44. doi: 10.1016/j.neuron.2007.03.021
13. Goto Y, Grace AA. Dopaminergic modulation of limbic and cortical drive of nucleus accumbens in goal-directed behavior. Nat Neurosci (2005) Jun8(6):805–12. doi: 10.1038/nn1471
14. Bastiaansen MC, Böcker KB, Cluitmans PJ, Brunia CH. Event-related desynchronization related to the anticipation of a stimulus providing knowledge of results. Clin Neurophysiol (1999) 110(2):250–60. doi: 10.1016/S0013-4694(98)00122-9
15. Gómez CM, Vaquero E, Vázquez-Marrufo M. A neurocognitive model for short-term sensory and motor preparatory activity in humans. Psicol (Valencia) (2004) 25(2):217–29.
16. Sanmiguel I, Saupe K, Schröger E. I know what is missing here: electrophysiological prediction error signals elicited by omissions of predicted “what” but not “when”. Front Hum Neurosci (2013) 7:407. doi: 10.3389/fnhum.2013.00407
17. Carlsson K, Petrovic P, Skare S, Petersson KM, Ingvar M. Tickling expectations: neural processing in anticipation of a sensory stimulus. J Cognit Neurosci (2000) 12(4):691–703. doi: 10.1162/089892900562318
18. Hohwy J. Attention and conscious perception in the hypothesis testing brain. Front Psychol (2012) 3:96. doi: 10.3389/fpsyg.2012.00096
19. Lotter W, Kreiman G, Cox D. Deep predictive coding networks for video prediction and unsupervised learning. In: Proceedings of the 5th International Conference on Learning Representations; 2017 Apr 24-26; Toulon, France: ICLR Conference Track Proceedings (2017). Available at: https://openreview.net/forum?id=B1ewdt9xe.
20. Seth AK. Interoceptive inference, emotion, and the embodied self. Trends Cognit Sci (2013) 17(11):565–73. doi: 10.1016/j.tics.2013.09.007
21. Wacongne C, Changeux JP, Dehaene S. A neuronal model of predictive coding accounting for the mismatch negativity. J Neurosci (2012) 32(11):3665–78. doi: 10.1523/JNEUROSCI.5003-11.2012
22. Fogelson N, Litvak V, Peled A, Fernandez-del-Olmo M, Friston K. The functional anatomy of schizophrenia: A dynamic causal modeling study of predictive coding. Schizophr Res (2014) 158(1-3):204–12. doi: 10.1016/j.schres.2014.06.011
23. Pellicano E, Burr D. When the world becomes ‘too real’: a Bayesian explanation of autistic perception. Trends Cognit Sci (2012) 16(10):504–10. doi: 10.1016/j.tics.2012.08.009
24. Wacongne C. A predictive coding account of MMN reduction in schizophrenia. Biol Psychol (2016) 116:68–74. doi: 10.1016/j.biopsycho.2015.10.011
25. Engel-Yeger B, Muzio C, Rinosi G, Solano P, Geoffroy PA, Pompili M, et al. Extreme sensory processing patterns and their relation with clinical conditions among individuals with major affective disorders. Psychiatry Res (2016) 236:112–8. doi: 10.1016/j.psychres.2015.12.022
26. De Berardis D, Fornaro M, Orsolini L, Valchera A, Carano A, Vellante F, et al. Alexithymia and suicide risk in psychiatric disorders: a mini-review. Front Psychiatry (2017) 8:148. doi: 10.3389/fpsyt.2017.00148
27. Palser ER, Palmer CE, Galvez-Pol A, Hannah R, Fotopoulou A, Kilner JM. Alexithymia mediates the relationship between interoceptive sensibility and anxiety. PloS One (2018) 13(9):e0203212. doi: 10.1371/journal.pone.0203212
28. Gershman SJ, Uchida N. Believing in dopamine. Nat Rev Neurosci (2019) 20(11):703–14. doi: 10.1038/s41583-019-0220-7
29. Trapp S, Bar M. Prediction, context, and competition in visual recognition. Ann N Y Acad Sci (2015) 1339(1):190–8. doi: 10.1111/nyas.12680
30. Hosoya T, Baccus SA, Meister M. Dynamic predictive coding by the retina. Nature (2005) 436(7047):71–7. doi: 10.1038/nature03689
31. Spratling MW. Unsupervised learning of generative and discriminative weights encoding elementary image components in a predictive coding model of cortical function. Neural Comput (2012) 24(1):60–103. doi: 10.1162/NECO_a_00222
32. Treisman AM, Gelade G. A feature-integration theory of attention. Cognit Psychol (1980) 12(1):97–136. doi: 10.1016/0010-0285(80)90005-5
33. Atick JJ. Entropy minimization: A design principle for sensory perception? Int J Neural Syst (1992) 3 supp01:81–90. doi: 10.1142/S0129065792000413
34. Srinivasan MV, Laughlin SB, Dubs A. Predictive coding: a fresh view of inhibition in the retina. Proc R Soc Lond B Biol Sci (1982) 216(1205):427–59. doi: 10.1098/rspb.1982.0085
35. Dan Y, Atick JJ, Reid RC. Efficient coding of natural scenes in the lateral geniculate nucleus: experimental test of a computational theory. J Neurosci (1996) 16(10):3351–62. doi: 10.1523/JNEUROSCI.16-10-03351.1996
36. Goldberg ME, Bisley JW, Powell KD, Gottlieb J. Saccades, salience and attention: the role of the lateral intraparietal area in visual behavior. Prog Brain Res (2006) 155:157–75. doi: 10.1016/S0079-6123(06)55010-1
37. Treue S. Visual attention: the where, what, how and why of saliency. Curr Opin Neurobiol (2003) 13(4):428–32. doi: 10.1016/S0959-4388(03)00105-3
38. Bastos AM, Usrey WM, Adams RA, Mangun GR, Fries P, Friston KJ. Canonical microcircuits for predictive coding. Neuron (2012) 76(4):695–711. doi: 10.1016/j.neuron.2012.10.038
39. Kanai R, Komura Y, Shipp S, Friston K. Cerebral hierarchies: predictive processing, precision and the pulvinar. Philos Trans R Soc B: Biol Sci (2015) 370(1668):20140169. doi: 10.1098/rstb.2014.0169
40. Summerfield C, Egner T. Expectation (and attention) in visual cognition. Trends Cognit Sci (2009) 13(9):403–9. doi: 10.1016/j.tics.2009.06.003
41. Runeson S. Visual prediction of collision with natural and nonnatural motion functions. Percept Psychophys (1975) 18(4):261–6. doi: 10.3758/BF03199372
42. Nijhawan R. Visual prediction: psychophysics and neurophysiology of compensation for time delays. Behav Brain Sci (2008) 31(2):179–98. doi: 10.1017/S0140525X08003804
43. Wilson HR, Ferrera VP, Yo C. A psychophysically motivated model for two-dimensional motion perception. Vis Neurosci (1992) 9(1):79–97. doi: 10.1017/S0952523800006386
44. Moore T, Fallah M. Control of eye movements and spatial attention. Proc Natl Acad Sci USA (2001) 98(3):1273–6. doi: 10.1073/pnas.98.3.1273
45. Moore T, Armstrong KM. Selective gating of visual signals by microstimulation of frontal cortex. Nature (2003) 421(6921):370–3. doi: 10.1038/nature01341
46. Ghose GM, Maunsell JH. Attentional modulation in visual cortex depends on task timing. Nature (2002) 419(6907):616–20. doi: 10.1038/nature01057
47. Janssen P, Shadlen MN. A representation of the hazard rate of elapsed time in macaque area LIP. Nat Neurosci (2005) 8(2):234–41. doi: 10.1038/nn1386
48. Stefanics G, Astikainen P, Czigler I. Visual mismatch negativity (vMMN): a prediction error signal in the visual modality. Front Hum Neurosci (2015) 8:1074. doi: 10.3389/fnhum.2014.01074
49. Hamm JP, Yuste R. Somatostatin interneurons control a key component of mismatch negativity in mouse visual cortex. Cell Rep (2016) 1916(3):597–604. doi: 10.1016/j.celrep.2016.06.037
50. Blank H, Davis MH. Prediction errors but not sharpened signals simulate multivoxel fMRI patterns during speech perception. PloS Biol (2016) 14(11):e1002577. doi: 10.1371/journal.pbio.1002577
51. Gagnepain P, Henson RN, Davis MH. Temporal predictive codes for spoken words in auditory cortex. Curr Biol (2012) 22(7):615–21. doi: 10.1016/j.cub.2012.02.015
52. Näätänen R, Lehtokoski A, Lennes M, Cheour M, Huotilainen M, Iivonen A, et al. Language-specific phoneme representations revealed by electric and magnetic brain responses. Nature (1997) 385(6615):432–4. doi: 10.1038/385432a0
53. Koelsch S, Vuust P, Friston K. Predictive processes and the peculiar case of music. Trends Cognit Sci (2019) 23(1):63–77. doi: 10.1016/j.tics.2018.10.006
54. Näätänen R, Gaillard AW, Mäntysalo S. Early selective-attention effect on evoked potential reinterpreted. Acta Psychol (Amst) (1978) 42(4):313–29. doi: 10.1016/0001-6918(78)90006-9
55. Kremláček J, Kreegipuu K, Tales A, Astikainen P, Põldver N, Näätänen R, et al. Visual mismatch negativity (vMMN): A review and meta-analysis of studies in psychiatric and neurological disorders. Cortex (2016) 80:76–112. doi: 10.1016/j.cortex.2016.03.017
56. Stefanics G, Kremláček J, Czigler I. Visual mismatch negativity: a predictive coding view. Front Hum Neurosci (2014) 8:666. doi: 10.3389/fnhum.2014.00666
57. Light GA, Swerdlow NR. Future clinical uses of neurophysiological biomarkers to predict and monitor treatment response for schizophrenia. Ann N Y Acad Sci (2015) 1344(1):105–19. doi: 10.1111/nyas.12730
58. Squires NK, Squires KC, Hillyard SA. Two varieties of long-latency positive waves evoked by unpredictable auditory stimuli in man. Electroencephalogr Clin Neurophysiol (1975) 38(4):387–401. doi: 10.1016/0013-4694(75)90263-1
59. Näätänen R, Paavilainen P, Tiitinen H, Jiang D, Alho K. Attention and memory. Psychophysiol (1993) 30(5):436–50. doi: 10.1111/j.1469-8986.1993.tb02067.x
60. Lee M, Sehatpour P, Hoptman MJ, Lakatos P, Dias EC, Kantrowitz JT, et al. Neural mechanisms of mismatch negativity dysfunction in schizophrenia. Mol Psychiatry (2017) 22(11):1585–93. doi: 10.1038/mp.2017.3
61. Alho K. Cerebral generators of mismatch negativity (MMN) and its magnetic counterpart (MMNm) elicited by sound changes. Ear Hear (1995) 16(1):38–51. doi: 10.1097/00003446-199502000-00004
62. Molholm S, Ritter W, Javitt DC, Foxe JJ. Multisensory visual–auditory object recognition in humans: a high-density electrical mapping study. Cereb Cortex (2004) 14(4):452–65. doi: 10.1093/cercor/bhh007
63. Giard MH, Lavikahen J, Reinikainen K, Perrin F, Bertrand O, Pernier J, et al. Separate representation of stimulus frequency, intensity, and duration in auditory sensory memory: an event-related potential and dipole-model analysis. J Cognit Neurosci (1995) 7(2):133–43. doi: 10.1162/jocn.1995.7.2.133
64. Molholm S, Martinez A, Ritter W, Javitt DC, Foxe JJ. The neural circuitry of pre-attentive auditory change-detection: an fMRI study of pitch and duration mismatch negativity generators. Cereb Cortex (2005) 15(5):545–51. doi: 10.1093/cercor/bhh155
65. Paavilainen P, Alho K, Reinikainen K, Sams M, Näätänen R. Right hemisphere dominance of different mismatch negativities. Electroencephalogr Clin Neurophysiol (1991) 78(6):466–79. doi: 10.1016/0013-4694(91)90064-B
66. Schröger E. Measurement and interpretation of the mismatch negativity. Behav Res Methods Instrum Comput (1998) 30(1):131–45. doi: 10.3758/BF03209423
67. Giard MH, Perrin F, Pernier J, Bouchet P. Brain generators implicated in the processing of auditory stimulus deviance: a topographic event-related potential study. Psychophysiology (1990) 27(6):627–40. doi: 10.1111/j.1469-8986.1990.tb03184.x
68. Jemel B, Achenbach C, Müller BW, Röpcke B, Oades RD. Mismatch negativity results from bilateral asymmetric dipole sources in the frontal and temporal lobes. Brain Topogr (2002) 15(1):13–27. doi: 10.1023/A:1019944805499
69. Lappe C, Steinsträter O, Pantev C. A beamformer analysis of MEG data reveals frontal generators of the musically elicited mismatch negativity. PloS One (2013) 8(4):e61296. doi: 10.1371/journal.pone.0061296
70. Näätänen R, Jacobsen T, Winkler I. Memory-based or afferent processes in mismatch negativity (MMN): a review of the evidence. Psychophysiology (2005) 42(1):25–32. doi: 10.1111/j.1469-8986.2005.00256.x
71. Rinne T, Alho K, Ilmoniemi RJ, Virtanen J, Näätänen R. Separate time behaviors of the temporal and frontal mismatch negativity sources. Neuroimage (2000) 12(1):14–9. doi: 10.1006/nimg.2000.0591
72. Antunes FM, Malmierca MS. An overview of stimulus-specific adaptation in the auditory thalamus. Brain Topogr (2014) 27(4):480–99. doi: 10.1007/s10548-013-0342-6
73. Gaebler AJ, Zweerings J, Koten JW Jr, König AA, Turetsky BI, Zvyagintsev M, et al. Impaired Subcortical Detection of Auditory Changes in Schizophrenia but Not in Major Depression. Schizophr Bull (2020) 46(1):193–201. doi: 10.1093/schbul/sbz027
74. Ruusuvirta T, Astikainen P, Wikgren J, Nokia M. Hippocampus responds to auditory change in rabbits. Neuroscience (2010) 170(1):232–7. doi: 10.1016/j.neuroscience.2010.06.062
75. Ruusuvirta T, Lipponen A, Pellinen E, Penttonen M, Astikainen P. Auditory cortical and hippocampal-system mismatch responses to duration deviants in urethane-anesthetized rats. PloS One (2013) 8(1):e54624. doi: 10.1371/journal.pone.0054624
76. Fishman YI. The mechanisms and meaning of the mismatch negativity. Brain Topogr (2014) 27(4):500–26. doi: 10.1007/s10548-013-0337-3
77. May PJ, Tiitinen H. Mismatch negativity (MMN), the deviance-elicited auditory deflection, explained. Psychophysiology (2010) 47(1):66–122. doi: 10.1111/j.1469-8986.2009.00856.x
78. Fishman YI, Steinschneider M. Searching for the mismatch negativity in primary auditory cortex of the awake monkey: deviance detection or stimulus specific adaptation? J Neurosci (2012) 32(45):15747–58. doi: 10.1523/JNEUROSCI.2835-12.2012
79. Wehr M, Zador AM. Synaptic mechanisms of forward suppression in rat auditory cortex. Neuron (2005) 47(3):437–45. doi: 10.1016/j.neuron.2005.06.009
80. Horváth J, Czigler I, Jacobsen T, Maess B, Schröger E, Winkler I. MMN or no MMN: no magnitude of deviance effect on the MMN amplitude. Psychophysiology (2008) 45(1):60–9. doi: 10.1111/j.1469-8986.2007.00599.x
81. Yabe H, Tervaniemi M, Reinikainen K, Näätänen R. Temporal window of integration revealed by MMN to sound omission. Neuroreport (1997) 8(8):1971–4. doi: 10.1097/00001756-199705260-00035
82. Chao ZC, Takaura K, Wang L, Fujii N, Dehaene S. Large-scale cortical networks for hierarchical prediction and prediction error in the primate brain. Neuron (2018) 100(5):1252–66. doi: 10.1016/j.neuron.2018.10.004
83. Carbajal GV, Malmierca MS. The neuronal basis of predictive coding along the auditory pathway: from the subcortical roots to cortical deviance detection. Trends Hear (2018) 22:2331216518784822. doi: 10.1177/2331216518784822
84. Malmierca MS, Anderson LA, Antunes FM. The cortical modulation of stimulus-specific adaptation in the auditory midbrain and thalamus: a potential neuronal correlate for predictive coding. Front Syst Neurosci (2015) 9:19. doi: 10.3389/fnsys.2015.00019
85. Yarden TS, Nelken I. Stimulus-specific adaptation in a recurrent network model of primary auditory cortex. PloS Comput Biol (2017) 13(3):e1005437. doi: 10.1371/journal.pcbi.1005437
86. He J. Corticofugal modulation of the auditory thalamus. Exp Brain Res (2003) 153(4):579–90. doi: 10.1007/s00221-003-1680-5
87. Hernández O, Rees A, Malmierca MS. A GABAergic component in the commissure of the inferior colliculus in rat. Neuroreport (2006) 17(15):1611–4. doi: 10.1097/01.wnr.0000236857.70715.be
88. Malmierca MS, Sanchez-Vives MV, Escera C, Bendixen A. Neuronal adaptation, novelty detection and regularity encoding in audition. Front Syst Neurosci (2014) 8:111. doi: 10.3389/fnsys.2014.00111
89. Ayala YA, Udeh A, Dutta K, Bishop D, Malmierca MS, Oliver DL. Differences in the strength of cortical and brainstem inputs to SSA and non-SSA neurons in the inferior colliculus. Sci Rep (2015) 5(1):10383. doi: 10.1038/srep10383
90. Parras GG, Nieto-Diego J, Carbajal GV, Valdés-Baizabal C, Escera C, Malmierca MS. Neurons along the auditory pathway exhibit a hierarchical organization of prediction error. Nat Commun (2017) 8(1):2148. doi: 10.1038/s41467-017-02038-6
91. Ohmae S, Uematsu A, Tanaka M. Temporally specific sensory signals for the detection of stimulus omission in the primate deep cerebellar nuclei. J Neurosci (2013) 33(39):15432–41. doi: 10.1523/JNEUROSCI.1698-13.2013
92. Kameda M, Ohmae S, Tanaka M. Entrained neuronal activity to periodic visual stimuli in the primate striatum compared with the cerebellum. eLife (2019) 8:8. doi: 10.7554/eLife.48702
93. Garrido MI, Kilner JM, Stephan KE, Friston KJ. The mismatch negativity: a review of underlying mechanisms. Clin Neurophysiol (2009) 120(3):453–63. doi: 10.1016/j.clinph.2008.11.029
94. Sikkens T, Bosman CA, Olcese U. The role of top-down modulation in shaping sensory processing across brain states: implications for consciousness. Front Syst Neurosci (2019) 13:31. doi: 10.3389/fnsys.2019.00031
95. Restuccia D, Della Marca G, Marra C, Rubino M, Valeriani M. Attentional load of the primary task influences the frontal but not the temporal generators of mismatch negativity. Brain Res Cognit Brain Res (2005) 25(3):891–9. doi: 10.1016/j.cogbrainres.2005.09.023
96. Doeller CF, Opitz B, Mecklinger A, Krick C, Reith W, Schröger E. Prefrontal cortex involvement in preattentive auditory deviance detection: neuroimaging and electrophysiological evidence. Neuroimage (2003) 20(2):1270–82. doi: 10.1016/S1053-8119(03)00389-6
97. Ishishita Y, Kunii N, Shimada S, Ibayashi K, Tada M, Kirihara K, et al. Deviance detection is the dominant component of auditory contextual processing in the lateral superior temporal gyrus: A human ECoG study. Hum Brain Mapp (2019) 40(4):1184–94. doi: 10.1002/hbm.24438
98. Romanski LM, Tian B, Fritz J, Mishkin M, Goldman-Rakic PS, Rauschecker JP. Dual streams of auditory afferents target multiple domains in the primate prefrontal cortex. Nat Neurosci (1999) 2(12):1131–6. doi: 10.1038/16056
99. Boly M, Garrido MI, Gosseries O, Bruno MA, Boveroux P, Schnakers C, et al. Preserved feedforward but impaired top-down processes in the vegetative state. Science (2011) 332(6031):858–62. doi: 10.1126/science.1202043
100. Ham BK, Chen J, Yan Y, Lucas WJ. Insights into plant phosphate sensing and signaling. Curr Opin Biotechnol (2018) 49:1–9. doi: 10.1016/j.copbio.2017.07.005
101. Althen H, Grimm S, Escera C. Fast detection of unexpected sound intensity decrements as revealed by human evoked potentials. PloS One (2011) 6(12):e28522. doi: 10.1371/journal.pone.0028522
102. Farley BJ, Quirk MC, Doherty JJ, Christian EP. Stimulus-specific adaptation in auditory cortex is an NMDA-independent process distinct from the sensory novelty encoded by the mismatch negativity. J Neurosci (2010) 30(49):16475–84. doi: 10.1523/JNEUROSCI.2793-10.2010
103. Schröger E, Wolff C. Mismatch response of the human brain to changes in sound location. Neuroreport (1996) 7(18):3005–8. doi: 10.1097/00001756-199611250-00041
104. Tada M, Kirihara K, Mizutani S, Uka T, Kunii N, Koshiyama D, et al. Mismatch negativity (MMN) as a tool for translational investigations into early psychosis: A review. Int J Psychophysiol (2019) 145:5–14. doi: 10.1016/j.ijpsycho.2019.02.009
105. Ruhnau P, Herrmann B, Schröger E. Finding the right control: the mismatch negativity under investigation. Clin Neurophysiol (2012) 123(3):507–12. doi: 10.1016/j.clinph.2011.07.035
106. Baldeweg T. ERP repetition effects and mismatch negativity generation: a predictive coding perspective. J Psychophysiol (2007) 21(3-4):204–13. doi: 10.1027/0269-8803.21.34.204
107. Leung S, Greenwood L, Michie P, Croft R. The role of stimulus train length in mismatch negativity (MMN) abnormalities in schizophrenia: A comparison of the’roving’and’oddball’MMN paradigms. In: XII International Conference on Cognitive Neuroscience. 2014 Jul 27-31; Brisbane, Queensland, Australia. ICON-XII Conference Abstract (2015). doi: 10.3389/conf.fnhum.2015.217.00153
108. Schmidt A, Bachmann R, Kometer M, Csomor PA, Stephan KE, Seifritz E, et al. Mismatch negativity encoding of prediction errors predicts S-ketamine-induced cognitive impairments. Neuropsychopharmacology (2012) 37(4):865–75. doi: 10.1038/npp.2011.261
109. McCleery A, Mathalon DH, Wynn JK, Roach BJ, Hellemann GS, Marder SR, et al. Parsing components of auditory predictive coding in schizophrenia using a roving standard mismatch negativity paradigm. Psychol Med (2019) 49(7):1195–206. doi: 10.1017/S0033291718004087
110. Wacongne C, Labyt E, van Wassenhove V, Bekinschtein T, Naccache L, Dehaene S. Evidence for a hierarchy of predictions and prediction errors in human cortex. Proc Natl Acad Sci USA (2011) 108(51):20754–9. doi: 10.1073/pnas.1117807108
111. Dürschmid S, Edwards E, Reichert C, Dewar C, Hinrichs H, Heinze HJ, et al. Hierarchy of prediction errors for auditory events in human temporal and frontal cortex. Proc Natl Acad Sci USA (2016) 113(24):6755–60. doi: 10.1073/pnas.1525030113
112. Erickson MA, Ruffle A, Gold JM. A meta-analysis of mismatch negativity in schizophrenia: from clinical risk to disease specificity and progression. Biol Psychiatry (2016) 79(12):980–7. doi: 10.1016/j.biopsych.2015.08.025
113. Nagai T, Tada M, Kirihara K, Araki T, Jinde S, Kasai K. Mismatch negativity as a “translatable” brain marker toward early intervention for psychosis: a review. Front Psychiatry (2013) 4:115. doi: 10.3389/fpsyt.2013.00115
114. Umbricht D, Krljes S. Mismatch negativity in schizophrenia: a meta-analysis. Schizophr Res (2005) 76(1):1–23. doi: 10.1016/j.schres.2004.12.002
115. Koshiyama D, Kirihara K, Tada M, Nagai T, Koike S, Suga M, et al. Duration and frequency mismatch negativity shows no progressive reduction in early stages of psychosis. Schizophr Res (2017) 190:32–8. doi: 10.1016/j.schres.2017.03.015
116. Näätänen R, Kähkönen S. Central auditory dysfunction in schizophrenia as revealed by the mismatch negativity (MMN) and its magnetic equivalent MMNm: a review. Int J Neuropsychopharmacol (2009) 12(1):125–35. doi: 10.1017/S1461145708009322
117. Suga M, Nishimura Y, Kawakubo Y, Yumoto M, Kasai K. Magnetoencephalographic recording of auditory mismatch negativity in response to duration and frequency deviants in a single session in patients with schizophrenia. Psychiatry Clin Neurosci (2016) 70(7):295–302. doi: 10.1111/pcn.12397
118. Neuhaus AH, Brandt ES, Goldberg TE, Bates JA, Malhotra AK. Evidence for impaired visual prediction error in schizophrenia. Schizophr Res (2013) 147(2-3):326–30. doi: 10.1016/j.schres.2013.04.004
119. Urban A, Kremlácek J, Masopust J, Libiger J. Visual mismatch negativity among patients with schizophrenia. Schizophr Res (2008) 102(1-3):320–8. doi: 10.1016/j.schres.2008.03.014
120. Turetsky BI, Bilker WB, Siegel SJ, Kohler CG, Gur RE. Profile of auditory information-processing deficits in schizophrenia. Psychiatry Res (2009) 165(1-2):27–37. doi: 10.1016/j.psychres.2008.04.013
121. Nagai T, Tada M, Kirihara K, Yahata N, Hashimoto R, Araki T, et al. Auditory mismatch negativity and P3a in response to duration and frequency changes in the early stages of psychosis. Schizophr Res (2013) 150(2-3):547–54. doi: 10.1016/j.schres.2013.08.005
122. Shiga T, Horikoshi S, Kanno K, Kanno-Nozaki K, Hikita M, Itagaki S, et al. Plasma levels of dopamine metabolite correlate with mismatch negativity in patients with schizophrenia. Psychiatry Clin Neurosci (2020) 74(5):289–93. doi: 10.1111/pcn.12984
123. Kaur M, Battisti RA, Lagopoulos J, Ward PB, Hickie IB, Hermens DF. Neurophysiological biomarkers support bipolar-spectrum disorders within psychosis cluster. J Psychiatry Neurosci (2012) 37(5):313–21. doi: 10.1503/jpn.110081
124. Koshiyama D, Fukunaga M, Okada N, Yamashita F, Yamamori H, Yasuda Y, et al. Role of subcortical structures on cognitive and social function in schizophrenia. Sci Rep (2018) 8(1):1183. doi: 10.1038/s41598-017-18950-2
125. Fujioka M, Kirihara K, Koshiyama D, Tada M, Nagai T, Usui K, et al. Mismatch Negativity Predicts Remission and Neurocognitive Function in Individuals at Ultra-High Risk for Psychosis. Front Psychiatry (2020) 11:770. doi: 10.3389/fpsyt.2020.00770
126. Kim M, Lee TH, Yoon YB, Lee TY, Kwon JS. Predicting remission in subjects at clinical high risk for psychosis using mismatch negativity. Schizophr Bull (2018) 44(3):575–83. doi: 10.1093/schbul/sbx102
127. Thomas ML, Green MF, Hellemann G, Sugar CA, Tarasenko M, Calkins ME, et al. Modeling deficits from early auditory information processing to psychosocial functioning in schizophrenia. JAMA Psychiatry (2017) 74(1):37–46. doi: 10.1001/jamapsychiatry.2016.2980
128. Atkinson RJ, Michie PT, Schall U. Duration mismatch negativity and P3a in first-episode psychosis and individuals at ultra-high risk of psychosis. Biol Psychiatry (2012) 71(2):98–104. doi: 10.1016/j.biopsych.2011.08.023
129. Jahshan C, Wynn JK, Mathis KI, Altshuler LL, Glahn DC, Green MF. Cross-diagnostic comparison of duration mismatch negativity and P3a in bipolar disorder and schizophrenia. Bipolar Disord (2012) 14(3):239–48. doi: 10.1111/j.1399-5618.2012.01008.x
130. Bodatsch M, Ruhrmann S, Wagner M, Müller R, Schultze-Lutter F, Frommann I, et al. Prediction of psychosis by mismatch negativity. Biol Psychiatry (2011) 69(10):959–66. doi: 10.1016/j.biopsych.2010.09.057
131. Brockhaus-Dumke A, Tendolkar I, Pukrop R, Schultze-Lutter F, Klosterkötter J, Ruhrmann S. Impaired mismatch negativity generation in prodromal subjects and patients with schizophrenia. Schizophr Res (2005) 73(2-3):297–310. doi: 10.1016/j.schres.2004.05.016
132. Javitt DC, Shelley A, Ritter W. Associated deficits in mismatch negativity generation and tone matching in schizophrenia. Clin Neurophysiol (2000) 111(10):1733–7. doi: 10.1016/S1388-2457(00)00377-1
133. Salisbury DF, Shenton ME, Griggs CB, Bonner-Jackson A, McCarley RW. Mismatch negativity in chronic schizophrenia and first-episode schizophrenia. Arch Gen Psychiatry (2002) 59(8):686–94. doi: 10.1001/archpsyc.59.8.686
134. Umbricht DS, Bates JA, Lieberman JA, Kane JM, Javitt DC. Electrophysiological indices of automatic and controlled auditory information processing in first-episode, recent-onset and chronic schizophrenia. Biol Psychiatry (2006) 59(8):762–72. doi: 10.1016/j.biopsych.2005.08.030
135. Murphy JR, Rawdon C, Kelleher I, Twomey D, Markey PS, Cannon M, et al. Reduced duration mismatch negativity in adolescents with psychotic symptoms: further evidence for mismatch negativity as a possible biomarker for vulnerability to psychosis. BMC Psychiatry (2013) 13(1):45. doi: 10.1186/1471-244X-13-45
136. Perez VB, Woods SW, Roach BJ, Ford JM, McGlashan TH, Srihari VH, et al. Automatic auditory processing deficits in schizophrenia and clinical high-risk patients: forecasting psychosis risk with mismatch negativity. Biol Psychiatry (2014) 75(6):459–69. doi: 10.1016/j.biopsych.2013.07.038
137. Devrim-Uçok M, Keskin-Ergen HY, Uçok A. Mismatch negativity at acute and post-acute phases of first-episode schizophrenia. Eur Arch Psychiatry Clin Neurosci (2008) 258(3):179–85. doi: 10.1007/s00406-007-0772-9
138. Salisbury DF, Kuroki N, Kasai K, Shenton ME, McCarley RW. Progressive and interrelated functional and structural evidence of post-onset brain reduction in schizophrenia. Arch Gen Psychiatry (2007) 64(5):521–9. doi: 10.1001/archpsyc.64.5.521
139. Shinozaki N, Yabe H, Sato Y, Hiruma T, Sutoh T, Nashida T, et al. The difference in Mismatch negativity between the acute and post-acute phase of schizophrenia. Biol Psychol (2002) 59(2):105–19. doi: 10.1016/S0301-0511(01)00129-6
140. Kaur M, Lagopoulos J, Lee RS, Ward PB, Naismith SL, Hickie IB, et al. Longitudinal associations between mismatch negativity and disability in early schizophrenia-and affective-spectrum disorders. Prog Neuropsychopharmacol Biol Psychiatry (2013) 46:161–9. doi: 10.1016/j.pnpbp.2013.07.002
141. Light GA, Braff DL. Mismatch negativity deficits are associated with poor functioning in schizophrenia patients. Arch Gen Psychiatry (2005) 62(2):127–36. doi: 10.1001/archpsyc.62.2.127
142. Umbricht D, Koller R, Schmid L, Skrabo A, Grübel C, Huber T, et al. How specific are deficits in mismatch negativity generation to schizophrenia? Biol Psychiatry (2003) 53(12):1120–31. doi: 10.1016/S0006-3223(02)01642-6
143. Hermens DF, Chitty KM, Kaur M. Mismatch negativity in bipolar disorder: A neurophysiological biomarker of intermediate effect? Schizophr Res (2018) 191:132–9. doi: 10.1016/j.schres.2017.04.026
144. Jahshan C, Cadenhead KS, Rissling AJ, Kirihara K, Braff DL, Light GA. Automatic sensory information processing abnormalities across the illness course of schizophrenia. Psychol Med (2012) 42(1):85–97. doi: 10.1017/S0033291711001061
145. Andersson S, Barder HE, Hellvin T, Løvdahl H, Malt UF. Neuropsychological and electrophysiological indices of neurocognitive dysfunction in bipolar II disorder. Bipolar Disord (2008) 10(8):888–99. doi: 10.1111/j.1399-5618.2008.00638.x
146. Hirakawa N, Hirano Y, Nakamura I, Hirano S, Sato J, Oribe N, et al. Right hemisphere pitch-mismatch negativity reduction in patients with major depression: an MEG study. J Affect Disord (2017) 215:225–9. doi: 10.1016/j.jad.2017.03.046
147. Qiao Z, Yu Y, Wang L, Yang X, Qiu X, Zhang C, et al. Impaired pre-attentive change detection in major depressive disorder patients revealed by auditory mismatch negativity. Psychiatry Res (2013) 211(1):78–84. doi: 10.1016/j.pscychresns.2012.07.006
148. Chen J, Zhang Y, Wei D, Wu X, Fu Q, Xu F, et al. Neurophysiological handover from MMN to P3a in first-episode and recurrent major depression. J Affect Disord (2015) 174:173–9. doi: 10.1016/j.jad.2014.11.049
149. Kim S, Jeon H, Jang KI, Kim YW, Im CH, Lee SH. Mismatch negativity and cortical thickness in patients with schizophrenia and bipolar disorder. Schizophr Bull (2019) 45(2):425–35. doi: 10.1093/schbul/sby041
150. Corlett PR, Horga G, Fletcher PC, Alderson-Day B, Schmack K. Powers AR 3rd. Hallucinations and strong priors. Trends Cognit Sci (2019) 23(2):114–27. doi: 10.1016/j.tics.2018.12.001
151. Powers AR, Mathys C, Corlett PR. Pavlovian conditioning-induced hallucinations result from overweighting of perceptual priors. Science (2017) 357(6351):596–600. doi: 10.1126/science.aan3458
152. Dima D, Roiser JP, Dietrich DE, Bonnemann C, Lanfermann H, Emrich HM, et al. Understanding why patients with schizophrenia do not perceive the hollow-mask illusion using dynamic causal modelling. Neuroimage (2009) 46(4):1180–6. doi: 10.1016/j.neuroimage.2009.03.033
153. Dima D, Dietrich DE, Dillo W, Emrich HM. Impaired top-down processes in schizophrenia: a DCM study of ERPs. Neuroimage (2010) 52(3):824–32. doi: 10.1016/j.neuroimage.2009.12.086
154. Sauer A, Zeev-Wolf M, Grent-’t-Jong T, Recasens M, Wacongne C, Wibral M, et al. Impairment in predictive processes during auditory mismatch negativity in ScZ: Evidence from event-related fields. Hum Brain Mapping (2017) 38(10):5082–93. doi: 10.1002/hbm.23716
155. McCleery A, Wynn JK, Mathalon DH, Roach BJ, Green MF. Hallucinations, neuroplasticity, and prediction errors in schizophrenia. Scand J Psychol (2018) 59(1):41–8. doi: 10.1111/sjop.12413
156. Coffman BA, Haigh SM, Murphy TK, Salisbury DF. Impairment in mismatch negativity but not repetition suppression in schizophrenia. Brain Topogr (2017) 30(4):521–30. doi: 10.1007/s10548-017-0571-1
157. Koshiyama D, Kirihara K, Tada M, Nagai T, Fujioka M, Usui K, et al. Reduced auditory mismatch negativity reflects impaired deviance detection in schizophrenia. Schizophr Bull (2020) 46(4):937–46. doi: 10.1093/schbul/sbaa006
158. Kasai K, Shenton ME, Salisbury DF, Hirayasu Y, Lee CU, Ciszewski AA, et al. Progressive decrease of left superior temporal gyrus gray matter volume in patients with first-episode schizophrenia. Am J Psychiatry (2003) 160(1):156–64. doi: 10.1176/appi.ajp.160.1.156
159. Grimm S, Escera C. Auditory deviance detection revisited: evidence for a hierarchical novelty system. Int J Psychophysiol (2012) 85(1):88–92. doi: 10.1016/j.ijpsycho.2011.05.012
160. Grimm S, Escera C, Nelken I. Early indices of deviance detection in humans and animal models. Biol Psychol (2016) 116:23–7. doi: 10.1016/j.biopsycho.2015.11.017
161. Harms L, Fulham WR, Todd J, Meehan C, Schall U, Hodgson DM, et al. Late deviance detection in rats is reduced, while early deviance detection is augmented by the NMDA receptor antagonist MK-801. Schizophr Res (2018) 191:43–50. doi: 10.1016/j.schres.2017.03.042
162. Umbricht D, Koller R, Vollenweider FX, Schmid L. Mismatch negativity predicts psychotic experiences induced by NMDA receptor antagonist in healthy volunteers. Biol Psychiatry (2002) 51(5):400–6. doi: 10.1016/S0006-3223(01)01242-2
163. Uhrig L, Janssen D, Dehaene S, Jarraya B. Cerebral responses to local and global auditory novelty under general anesthesia. Neuroimage (2016) 141:326–40. doi: 10.1016/j.neuroimage.2016.08.004
164. Coyle JT, Tsai G, Goff D. Converging evidence of NMDA receptor hypofunction in the pathophysiology of schizophrenia. Ann N Y Acad Sci (2003) 1003(1):318–27. doi: 10.1196/annals.1300.020
165. Kristiansen LV, Huerta I, Beneyto M, Meador-Woodruff JH. NMDA receptors and schizophrenia. Curr Opin Pharmacol (2007) 7(1):48–55. doi: 10.1016/j.coph.2006.08.013
166. Kort NS, Ford JM, Roach BJ, Gunduz-Bruce H, Krystal JH, Jaeger J, et al. Role of N-methyl-D-aspartate receptors in action-based predictive coding deficits in schizophrenia. Biol Psychiatry (2017) 81(6):514–24. doi: 10.1016/j.biopsych.2016.06.019
167. Mohn AR, Gainetdinov RR, Caron MG, Koller BH. Mice with reduced NMDA receptor expression display behaviors related to schizophrenia. Cell (1999) 98(4):427–36. doi: 10.1016/S0092-8674(00)81972-8
168. Olney JW, Newcomer JW, Farber NB. NMDA receptor hypofunction model of schizophrenia. J Psychiatr Res (1999) 33(6):523–33. doi: 10.1016/S0022-3956(99)00029-1
169. Horga G, Schatz KC, Abi-Dargham A, Peterson BS. Deficits in predictive coding underlie hallucinations in schizophrenia. J Neurosci (2014) 34(24):8072–82. doi: 10.1523/JNEUROSCI.0200-14.2014
170. Tobler PN, Fiorillo CD, Schultz W. Adaptive coding of reward value by dopamine neurons. Science (2005) 307(5715):1642–5. doi: 10.1126/science.1105370
171. Gold JM, Waltz JA, Prentice KJ, Morris SE, Heerey EA. Reward processing in schizophrenia: a deficit in the representation of value. Schizophr Bull (2008) 34(5):835–47. doi: 10.1093/schbul/sbn068
172. McCutcheon RA, Krystal JH, Howes OD. Dopamine and glutamate in schizophrenia: biology, symptoms and treatment. World Psychiatry (2020) 19(1):15–33. doi: 10.1002/wps.20693
173. Schultz W. Dopamine reward prediction-error signalling: a two-component response. Nat Rev Neurosci (2016) 17(3):183–95. doi: 10.1038/nrn.2015.26
174. Yagishita D, Chui RW, Yamakawa K, Rajendran PS, Ajijola OA, Nakamura K, et al. Sympathetic nerve stimulation, not circulating norepinephrine, modulates T-peak to T-end interval by increasing global dispersion of repolarization. Circulat: Arrhythmia Electrophysiol (2015) 8(1):174–85. doi: 10.1161/CIRCEP.114.002195
175. Kravitz AV, Tye LD, Kreitzer AC. Distinct roles for direct and indirect pathway striatal neurons in reinforcement. Nat Neurosci (2012) 15(6):816–8. doi: 10.1038/nn.3100
176. Yagishita S. Transient and sustained effects of dopamine and serotonin signaling in motivation-related behavior. Psychiatry Clin Neurosci (2020) 74(2):91–8. doi: 10.1111/pcn.12942
Keywords: prediction, MMN, mismatch negativity, schizophrenia, predictive coding
Citation: Fong CY, Law WHC, Uka T and Koike S (2020) Auditory Mismatch Negativity Under Predictive Coding Framework and Its Role in Psychotic Disorders. Front. Psychiatry 11:557932. doi: 10.3389/fpsyt.2020.557932
Received: 30 April 2020; Accepted: 18 August 2020;
Published: 10 September 2020.
Edited by:
Gregory Light, University of California, San Diego, United StatesReviewed by:
Gianluca Serafini, San Martino Hospital (IRCCS), ItalyKairi Kreegipuu, University of Tartu, Estonia
Copyright © 2020 Fong, Law, Uka and Koike. This is an open-access article distributed under the terms of the Creative Commons Attribution License (CC BY). The use, distribution or reproduction in other forums is permitted, provided the original author(s) and the copyright owner(s) are credited and that the original publication in this journal is cited, in accordance with accepted academic practice. No use, distribution or reproduction is permitted which does not comply with these terms.
*Correspondence: Shinsuke Koike, c2tvaWtlLXRreUB1bWluLmFjLmpw