- 1Department of Psychiatry, Hangzhou Seventh People’s Hospital, Hangzhou, China
- 2Mental Health Center, Zhejiang University, School of Medicine, Hangzhou, China
Abnormity in brain regional function and inter-regional cooperation have been linked with the dysfunction during cognitive and emotional processing in bipolar disorder (BD) patients. Recent evidences have suggested that brain function is not static but temporal dynamic. In present study, we aimed to characterize the temporal dynamics of regional function and inter-regional cooperation in BD and its relationship with executive dysfunction, an important deficit in BD. Resting-state functional MRI was performed in patients with bipolar I disorder (BDI) (n = 18) and healthy controls (HCs, n = 19). We first assessed local-function temporal variety with dynamic amplitude of low-frequency fluctuation (dALFF). Region with significant inter-groups difference in dALFF was chosen as a seed to calculate inter-regions connective temporal variety with dynamic functional connectivity (dFC). The executive function was measured by Verbal Fluency Test (VFT). The relationship between executive function and brain dynamics were examined. Compared with HC, the BDI group showed decreased dALFF (less temporal variability) in the posterior cingulate cortex (PCC) and decreased dFC between PCC and medial prefrontal cortex (mPFC). The PCC-mPFC dFC was positively associated with VFT in BDI patients, but not in HC. These findings implicated the reduced temporal variability in local region and inter-regions cooperation in BDI, which may be a neural substrate of executive-function deficit in BDI.
Introduction
Bipolar disorder (BD) is a common psychiatric disorder with remarkable mood instability, characterized by recurrent episodes of mania, hypomania, depression, and euthymic mood states (1). Bipolar I disorder and bipolar II disorder represent the most severe types of BD: the first former is characterized by alternating depressive and manic episodes of great severity that frequently requires hospitalization, while the latter is characterized by depressive and hypomanic episodes. Besides mood instability, neurocognitive dysfunction is another core feature of BD, which not only present in affective episodes, but also in euthymic periods. The neurocognitive dysfunctions involve multiple cognitive processes, including memory, attention, reward and executive function (2–5). Executive dysfunction is an important domain in BD, even considered as the specific deficit in BD. Importantly, executive dysfunction in BD are associated with poor social function (6, 7), delayed recovery (8) and higher readmission rate (9). However, the neural substrates of the executive dysfunction are still unclear for now.
Previous studies about the neural underpinnings of executive functions consistently implicated the key role of prefrontal cortex in healthy individuals (10). As a key node in emotion regulation network, prefrontal cortex certainly will contribute to the development of BD (11). Evidences from task-based fMRI studies have suggested abnormal activation in prefrontal cortex among BDI patients during executive tasks. However, these results are usually inconsistent. Strakowski SM et al. reported decreased activation in the lateral PFC in BDI during an executive task (12). In contrast, other studies indicated the hyperactivation of the lateral PFC among BD patients during verbal fluency task (VFT) (13, 14), a simple but class executive task. These inconsistent results may attribute to several factors, such as the type of BD and different tasks.
Recently, task-free fMRI (resting-state fMRI, RS-fMRI) provide a new insight to explore the neural substrate of executive dysfunction in BD. RS-fMRI could reveal the brain’s intrinsic neural activity in abnormal brain with blood oxygen level-dependent (BOLD) signals at rest. Zang Y et al. developed an index, amplitude of low-frequency fluctuation (ALFF), to detect the regional intensity of spontaneous activity (15). Studies with ALFF have found aberrant regional activity in several regions among patients with BD (16, 17). Notably, functional connectivity is another important index, which reflects inter-regional cooperation at rest. Mounting studies have identified abnormal functional connectivity and its relationship with emotional symptoms and executive deficits in BD (18, 19). Besides, the functional connectivity has been applied to reveal a host of intrinsic connectivity networks, referred to as brain networks, with distinct spatial and temporal profiles. The default mode network (DMN) is one of the most usually reported networks in psychiatric disorders, including BD. The DMN was initially identified as areas that consistently presented deactivation during most tasks and activation during resting state (20). Abnormal connectivity within DMN and inter-works connectivity of DMN with other networks have been frequently reported among patients with BD (21, 22).
Previous studies about functional connectivity mainly referred to the static functional connectivity (sFC), which is based on the assumption that the connection is spatial and temporal stationarity throughout the scanning period. This assumption may ignore the time-varying characteristics of connections, which have been proved by other measurements, such as electroencephalo-graph (EEG) (23, 24). To address this issue, recent studies with RS-fMRI developed dynamic analysis to estimate the variability of inter-region connections, i.e., dynamic functional connectivity (dFC). The credibility of the dFC has been demonstrated by evidence from simultaneous EEG/fMRI studies (25, 26).
Actually, several evidences have implicated the aberrant dFC among BD patients. Tanya T. Nguyen et al. reported the decreased dFC with a seed-seed wised approach (27). Although the findings are interesting, the seed-seed wised approach has several limitations, especially the seed-defined process. Recently, studies increasingly reported the dynamics of local brain activity among normal and dysfunctional brain at voxel level. Indeed, the local brain activity is supposed to be a reflection of mental activity (28), which may cause high time-varying (29). Multiple studies have found the abnormal temporal dynamics of local brain activity in affective disorder. For example, Li J. et al. computed the dynamic amplitude of low-frequency fluctuation (dALFF) to characterize the dynamics of local brain activity in depressed patients with suicidal ideation and found decreased brain dynamics in several limbic areas (30).
In the present study, we aimed at exploring the dynamic local activity and functional connectivity and its relationship with executive dysfunction in patients with BDI at voxel level. Based on the fact that the DMN plays a key role in the development of mood instability and executive dysfunction, we assumed that BDI patients present abnormal temporal variability of local region and inter-regions cooperations among key nodes in DMN, such as PCC and mPFC, as previous findings suggested. These decreased temporal variabilities may be associated with executive function deficit in BDI patients.
Materials and Methods
Participants
A total of 19 inpatients were recruited from the Hangzhou Seventh People’s Hospital, Hangzhou, China and diagnosed with bipolar disorder I (BDI) according to the Diagnostic and Statistical Manual of Mental Disorders, Fourth Edition (DSM-IV). The diagnosis was conducted by two psychiatrists based on structured clinical interviews. Patients would not be enrolled if the diagnosis is inconsistent between two psychiatrists. Patients would also be excluded due to the following exclusion criteria: (1) electroconvulsive therapy history during the last 6 months; (2) age over 65 years; (3) diagnosed with substance misuse, schizoaffective disorder or schizophrenia; (4) past or current neurological illness; (5) other contraindications of MRI scan administration; (6) too large head motion (>3 mm in translation or 3 in rotation). One patient was excluded because of large head motion, so 18 patients were included in the final analysis.
We also recruited 19 gender-, age-, and education-matched healthy subjects as controls via local advertisements. They were carefully screened through a diagnostic interview, the Structured Clinical Interview for DSM-IV (nonpatient edition), to rule out the presence of current or past psychiatric illness. Further exclusion criteria for the controls were any history of psychiatric illness in first-degree relatives and any current or past significant medical illness or mental disorders. This study was approved by the Hangzhou Seventh People’s Hospital Ethics Committee, and written informed consent was obtained from all participants.
Clinical Evaluation
The Bech-Rafaelsen Mania Rating Scale (BRMS) was used to evaluate clinical symptoms for all participants during the 7-day period prior to the scan. The executive function was assessed by the verbal fluency test (VFT). During the VFT, participants were required to say as many words as possible describing an animal and a vegetable within 1 min, respectively. One point was scored when participants gave a correct term for either the correct description of an animal or a vegetable.
MRI Data Acquisition
Resting-state and structural images of all participants were acquired at the Hangzhou Seventh People’s Hospital. Patients were instructed to keep their eyes closed and do not move and think as little as possible during the MRI scanning. Resting-state MRI scans were conducted under a 1.5 T MRI scanner (Signa HDxt 1.5 T, GE Healthcare, Buckinghamshire, UK) composed of 180 echo-planar imaging volumes with the following parameters: TR = 2000 ms; TE = 40 ms; flip angle = 85°; matrix size = 64 × 64, field of view = 240 × 240 mm; slice thickness = 3 mm; 28 continuous slices. Total acquisition of resting-state MRI lasted 6 min. A T1-weighted anatomical image was also acquired for each patient to further elucidate and discard gross radiological alterations. (TR = 9.5 ms; TE = 3.1 ms; flip angle = 20°; field of view = 240 mm × 240 mm; slice thickness = 1.2 mm).
Functional Data Preprocessing
Functional MRI data were preprocessed with the Data Processing Assistant for Resting-State Functional MR Imaging toolkit (31). For each participant, we applied the following processing steps: discarding of the first 5 volumes to achieve a steady-state, slice timing correction, realignment, spatial normalization based on the unified segmentation of structural images, nuisance regressors with 24 Friston motion parameters, white matter high signal, cerebrospinal fluid signal and global signals as regressors, filter with a temporal band-pass of 0.01–0.1 Hz and spatial smoothing (Gaussian kernel = 4 mm × 4 mm × 4 mm). Finally, motion scrubbing was conducted using the method of cubic spline to minimize the influence of the time points with high motion [frame-wise displacement (FD) > 0.5], as well as one time point prior to, and two time points following each of these high motion time points. Finally, there are 9 frames with high motion for BDI group and 16 frames for healthy control (HC) group. The range of FD is between 0 and 4.62 for BDI group and between 0 and 2.22 for HC group.
dALFF and dFC were calculated using DynamicBC software with the sliding window approach (32). According to previous study, we chose 30 TRs (60 s) as the window length for resting-state dynamic analysis (30, 33), and the window was shifted by one TRs. In our data, the full-length time series was comprised of 175 TRs, so there were 145 windows for each participant. For the time series in each window, the ALFF map was obtained. The ALFF of each voxel was divided by the global mean ALFF value to normalize the global effects. To study the temporal variability of ALFF, we computed the variance of dALFF maps (standard deviation of ALFF at each voxel) across sliding-window dynamics.
The functionally abnormal region in local-activity dynamics (PCC, see Results section) was selected as seed to calculate dFC. The Pearson’s correlation coefficient of the PCC with all other voxels in brain was calculated and Fisher’s z-transformed, yielding a sliding-window z-value map. Then, for each participant, a set of sliding-window z-value maps were used to calculate the dFC map (standard deviation in z values at each voxel). Finally, the dFC maps were converted to z-values using Fisher’s r-to-z transformation to improve normality.
In our functional data preprocessing, we conducted global signal regression, which may increase tissue sensitivity and decrease motion dependency. However, the impact of global signal regression (GSR) on dynamic FC is obscure for now (34).
To probe the effect of GSR on dynamic FC, we add complementary analysis without regressing global regression during preprocessing.
Statistical Analysis
The Pearson’s χ2 test was applied to compare sex differences and two-sample t-tests were applied to check for differences in age, educational years, clinical symptoms and executive-function performance between two groups. Voxel-wise two-sample t-tests with gender, age, educational years, and head motion indexed by FD as covariates were used to quantitatively compare the differences in the dALFF and dFC of posterior cingulate cortex (PCC, see below) the between two groups within the grey matter mask. All statistical maps were corrected using the Gaussian Random Field method at the threshold for voxel P < 0.001 and cluster P < 0.05. Then, we extracted ROI data to conduct correlation analysis. Pearson’s correlation analyses were performed to examine the associations between the brain dynamics and behavioral performance (clinical symptoms and executive-function performance). Significance was determined by P < 0.05 (two-tailed), with no correction.
Results
Demographic and Clinical Characteristic
Present study included 18 patients with BDI and 19 HCs. Demographic characteristic of the two groups are shown in Table 1. There was no significant difference between two groups in terms of age, gender, or head motion. Compared with the HCs, participants with BDI presented greater BRMS and lower VFT scores.
Group Comparison of dALFF
We explored the difference of dALFF between the two groups based on voxel level. Compared with HCs, patients with BDI presented decreased dALFF in posterior cingulate gyrus (PCC) (voxel size = 22; peak coordinate = −3, −66, 30) (shown in Figure 1). No other regions with significant different dALFF were found between two groups.
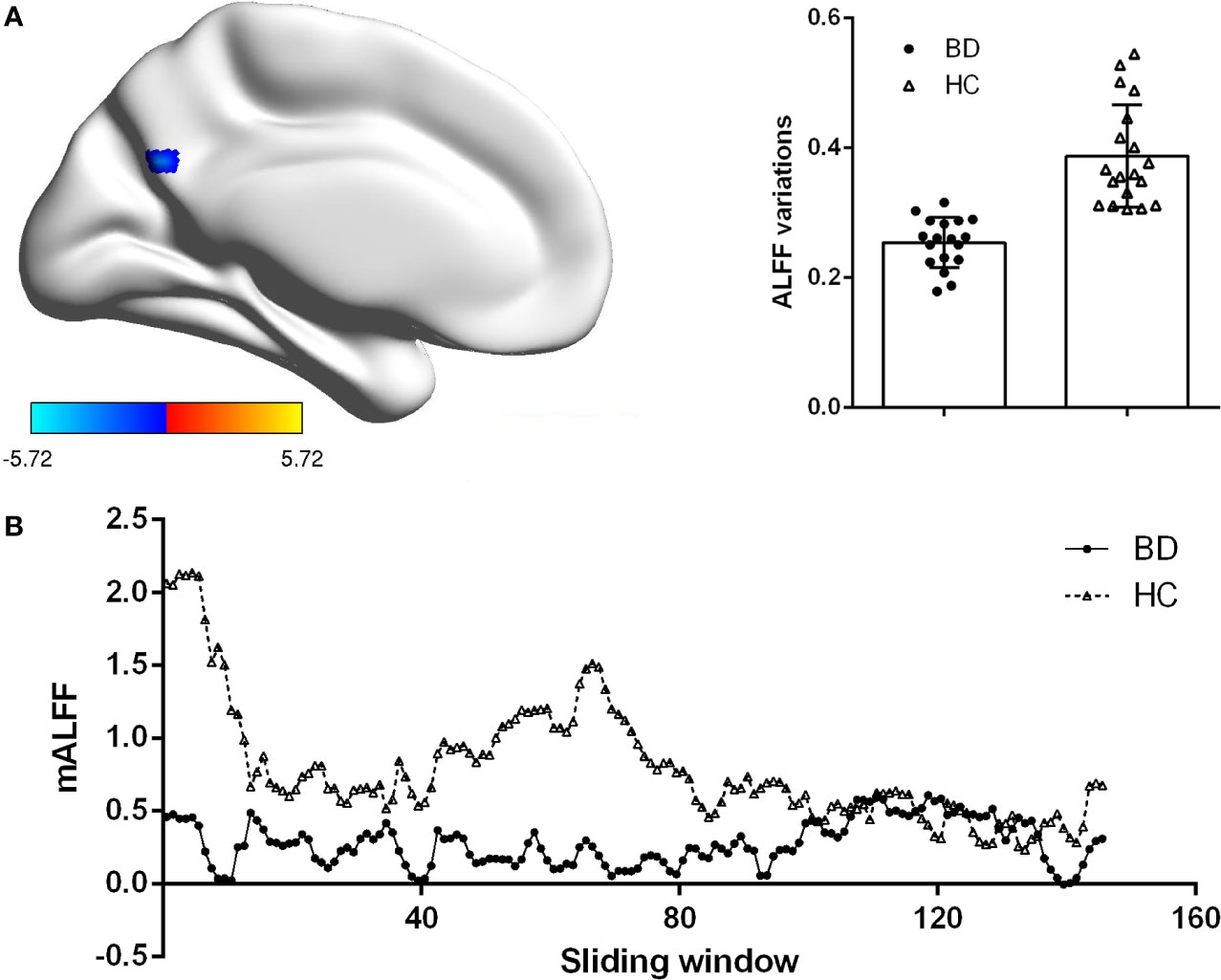
Figure 1 Group difference on the ALFF variations. (A) Patients BDI showed decreased ALFF variations (dALFF) in posterior cingulate cortex (PCC) than HC. Statistical map were corrected with Gaussian Random Field (GRF) method at threshold of voxel P < 0.001, cluster P < 0.05. Scatter plots present ALFF variations of each participant in two groups. (B) The mean ALFF were displayed for a single participant in the BDI group and a single participant in the HC group across all sliding windows.
Group-Level Comparison of dFC Based on the Seed of PCC
Figure 2 showed the difference of dFC based on PCC between patients with BDI and HCs. Compared with HCs, depressive individuals presented decreased dFC between PCC and medial prefrontal cortex (mPFC) (voxel size = 35; peak coordinate = −3, −57, 25). No other regions with significant different dFC were found between two groups.
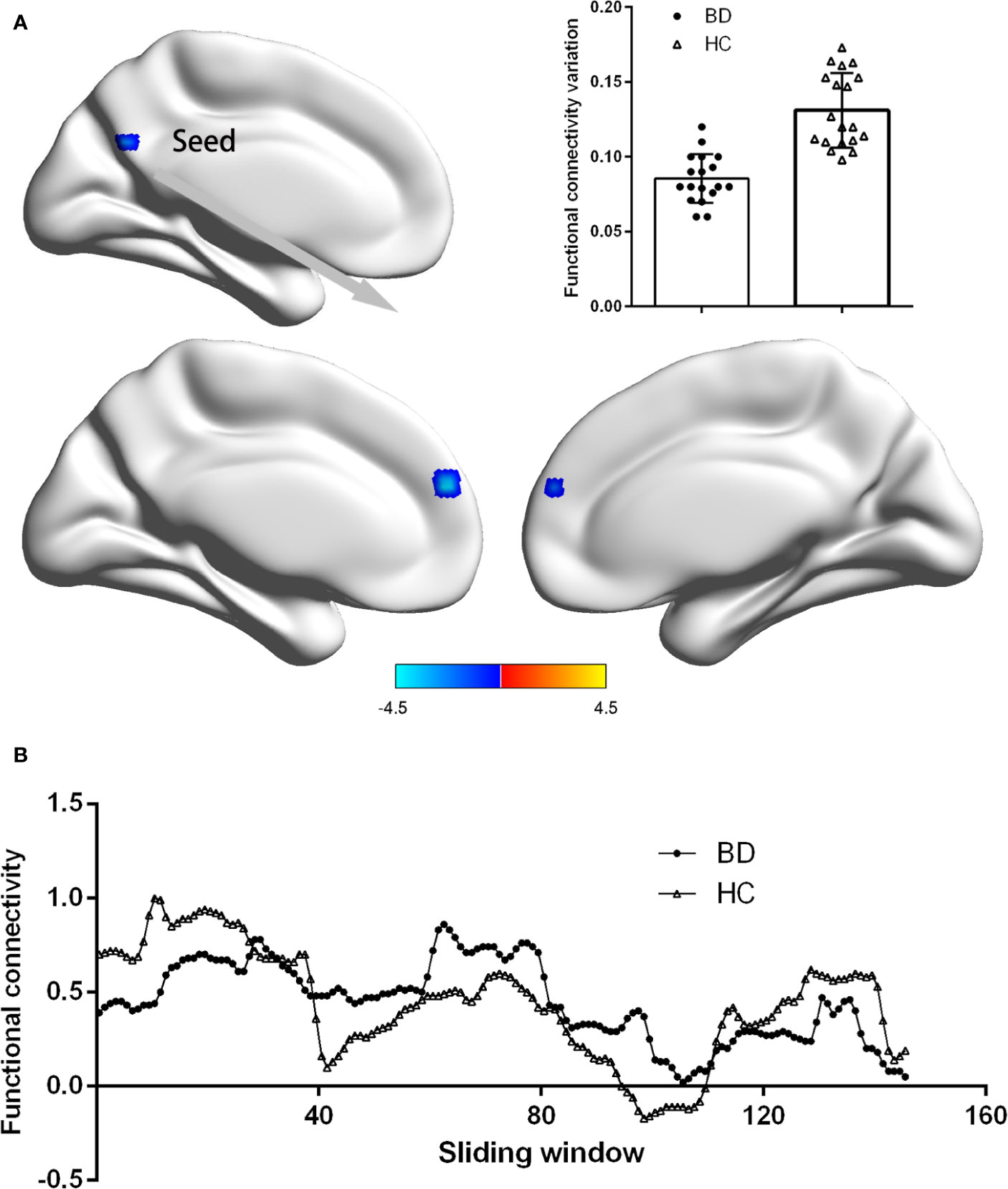
Figure 2 Group difference on the functional connectivity variations of PCC. (A) Patients BDI showed decreased functional connectivity variations (dFC) between PCC and medial prefrontal cortex (mPFC) than HC. Statistical map were corrected with Gaussian Random Field (GRF) method at threshold of voxel P < 0.001, cluster P < 0.05. Scatter plots present PCC-mPFC dFC of each participant in two groups. (B) The PCC-mPFC functional connectivity were displayed for a single participant in the BDI group and a single participant in the HC group across all sliding windows.
Group-Level Comparison of dFC Based on the Seed of PCC
To probe the effect of GSR on dynamic FC, we add complementary analysis without regressing global regression during preprocessing. Our results suggested that after without GSR, the difference of dALFF and dFC were significant at the threshold for voxel P < 0.005, but not significant for voxel P < 0.001 (see Supporting Informationl, Figure S1).
The Relationship Between dALFF and dFC and Behavioral Performance
A positive relationship (r = 0.521, p = 0.026) existed between right PCC-mPFC dFC and VFT scores among patients with BDI as shown in Figure 3. No significant correlation was found between PCC dALFF and VFT scores (r = −0.057, p = 0.822). There was also no significant correlation between clinical-symptoms severity and PCC dALFF or PCC-mPFC dFC (r = 0.052, p = 0.839; r = 0.055, p = 0.830).
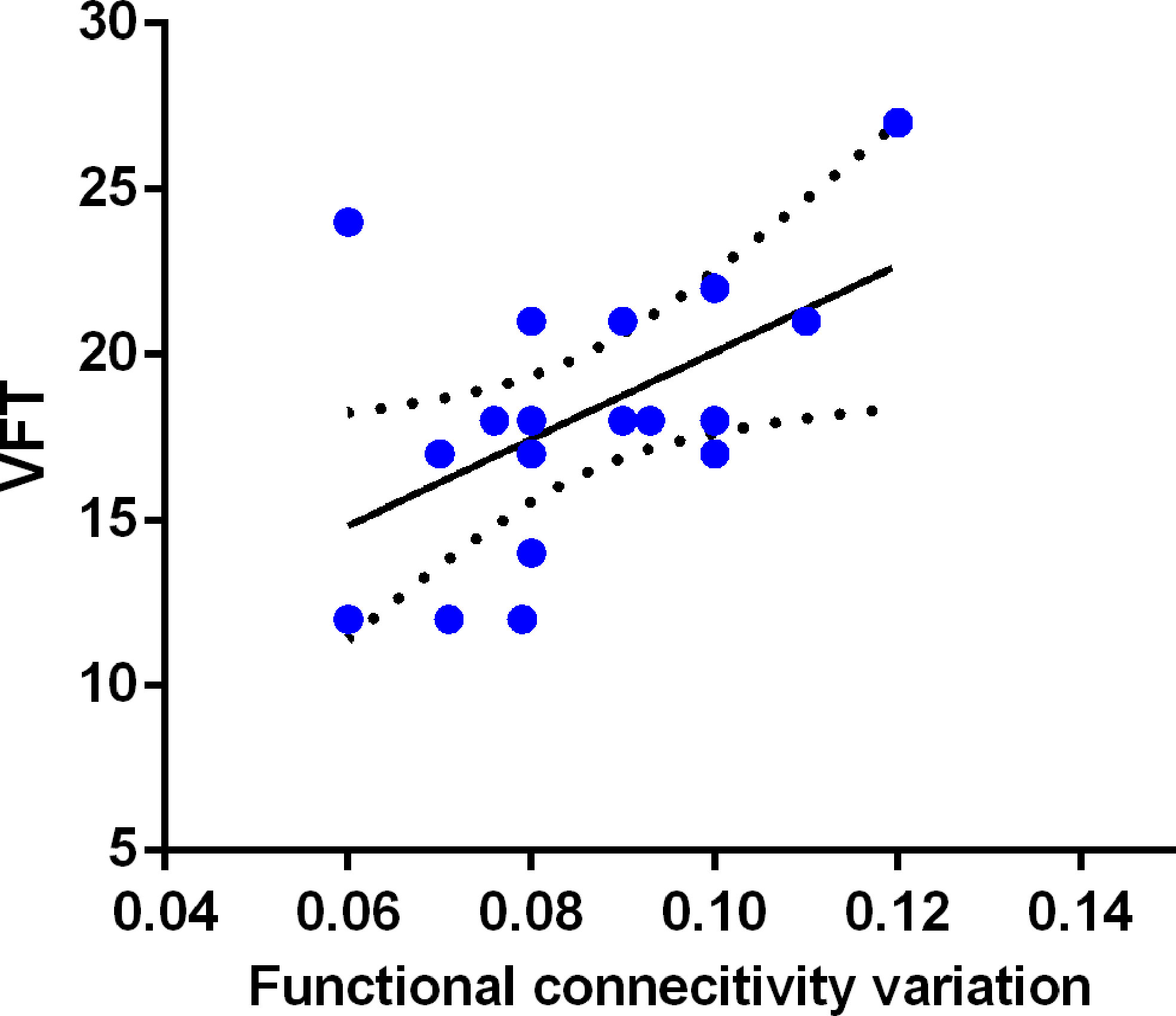
Figure 3 Relationship between PCC-mPFC dFC and executive function in BDI patients. The PCC-mPFC dFC was positively correlated with executive function evaluated by the Verbal Fluency Test (VFT) in patients with BDI.
Discussion
In present study, we mainly aimed at exploring the temporal dynamics of resting-state local activity and functional connectivity in patients with BDI, as well as its relationship with clinical symptoms and executive function. Our results suggested that patients with BDI showed decreased dALFF (less temporal variability) in the PCC and decreased dFC between PCC and mPFC. The PCC-mPFC dFC was positively associated with VFT in BDI patients, but not in HC. These findings suggest the reduced temporal variability in local region and inter-regions cooperations in BDI, which may be a neural substrate of executive-function deficit in BDI.
As a common and less time-consuming cognitive measurement, the VFT has been applied to investigate executive function. Present study found that BDI patients displayed weaker scores in the VFT, i.e., executive deficits. Prior literature has reported euthymic BDI were impaired in verbal memory and executive functioning which can be considered as a potential endophenotype of BDI (35–37). However, it must be admitted that the VFT may reflect reasoning ability and perceptual speed, only a fraction of executive function, which encompass various aspects, such as response inhibition, working memory and cognitive flexibility (38). Research with more multiple tests is required to replicate present findings.
The PCC is a key node in the DMN and was implicated in self-referential and reflective processes, such as episodic memory retrieval, images, and emotions (39). Although results were inconsistent, previous studies have linked DMN abnormalities with multiple mood disorders, such as depression and BDI (40–42). Indexed with static ALFF, the DMN abnormalities have been reported by plentiful studies. For example, Zhong et al. has reported that BD patients showed decreased ALFF in precuneus and PCC which are nodes of DMN (43). These abnormalities may underlie cognitive and affective processing problems in psychiatric conditions (41, 44). Consistent with previous studies, our results suggested the abnormal DMN temporal dynamics of spontaneous activity in BDI individuals. Unfortunately, we did not find the relationship between PCC local-activity dynamics (dALFF) and clinical symptoms or executive dysfunctions. Small sample size, high sample heterogeneity may be the potential factors which contribute to this confused condition. More importantly, this embarrassed status may also related with the fact that BDI is as a disorder driven by different regions interconnected dysregulation, rather than a dysfunction in one single brain area (45, 46).
Indeed, our results revealed the decreased PCC-mPFC connection variations in patients with BDI. The mPFC has been reported to be involved in several executive processing, such as response inhibition, inhibitory control, and task switching (10, 47, 48). Compared with healthy individuals, patients with BDI have been showed hypoactivation in mPFC (49). Evidence from resting-state connectivity studies also suggested the decreased connection between mPFC and PCC in BDI patients (50). Individuals with reduced PCC-mPFC connectivity exhibited impairment in executive function (51). In addition, the mPFC is also component of DMN and the reduced connectivity within DMN in patients with BDI has been frequently reported (51–53). The dysfunctions of DMN have been closely linked with both emotional and executive impairments of BDI (22, 54).
Besides static connection, the connective dynamics may be also important for the executive function and emotional regulation. Kucyi et al. suggested dFC within DMN is positively associated with emotion control (55). Abnormal intra- and internetwork connectivity variability are linked with mood disorders (56). Inter-network connectivity dynamics also contribute to cognitive flexibility (57), which is a key element of executive function. Consistent with these findings, our results revealed that the decreased PCC-mPFC connectivity dynamics in BDI is positively associated with the executive performance. Indeed, the relationship between PCC-mPFC connectivity dynamics and executive impairments in BDI patients has been indicated by previous study based on region-of-interest (ROI) level (27). As borderless regions, it is difficult to define accurately PCC or mPFC that may limit the strength of evidence. To improve this situation, we first explore the abnormal dynamics of spontaneous activity at voxel level. Intriguingly, we found reduced PCC spontaneous activity variety and further revealed reduced PCC-mPFC connectivity, which is in keeping with the fact that the PCC is an important node in interacting with other brain networks and optimizing cognitive function (58).
Intriguingly, several studies reported increased dynamic FC in major depression (42), which is converse with our results in BDI. These findings may be a potential biomarker for differentiating the two diseases. Indeed, as two related but distinct diseases, major depression and BD patients may present disparate brain-function temporal dynamics. However, there is no uniform conclusion about the common or specific alterations of brain-function temporal dynamics (56, 59). Further studies included BD I and II type and major depression to explore the differentiating signatures of brain-function temporal dynamics.
Several limitations in present study are worthy of discussion. First, our study enrolled small-size sample. Larger samples in future are needed to confirm current findings. Second, all patients included in the present study were administrated with drugs; we still cannot eliminate the effects of drugs on our findings. Third, the scanning quality is not most suitable for dynamic analysis, such as long repetition time (2 s), insufficient time points (180) and inferior magnetic field intensity (1.5 T). Finally, present study only included BDI patients during mania episode but not the depressive episode, which impeded us to exploring that the reduced PCC-mPFC connectivity variety is a state or trait biomarker of BDI.
Data Availability Statement
The raw data supporting the conclusions of this article will be made available by the authors, without undue reservation, to any qualified researcher.
Ethics Statement
The studies involving human participants were reviewed and approved by Hangzhou Seventh People’s Hospital Ethics Committee. The patients/participants provided their written informed consent to participate in this study.
Author Contributions
ZC supervised present study. YaL and XJ performed the analysis and wrote paper. WZ, YS, FX, and YiL helped to collect data.
Funding
This work was supported by funding from the Key Medical and Health Science and Technology Projects of Hangzhou (2015Z07) and Social development fund of science and technology bureau of Hangzhou (20191203B120).
Conflict of Interest
The authors declare that the research was conducted in the absence of any commercial or financial relationships that could be construed as a potential conflict of interest.
Acknowledgments
We thank all participants for their cooperation during this study.
Supplementary Material
The Supplementary Material for this article can be found online at: https://www.frontiersin.org/articles/10.3389/fpsyt.2020.537981/full#supplementary-material
References
1. Merikangas KR, Jin R, He JP, Kessler RC, Lee S, Sampson NA, et al. Prevalence and correlates of bipolar spectrum disorder in the world mental health survey initiative. Arch Gen Psychiatry (2011) 68:241–51. doi: 10.1001/archgenpsychiatry.2011.12
2. Chakrabarty T, Kozicky JM, Torres IJ, Lam RW, Yatham LN. Verbal memory impairment in new onset bipolar disorder: Relationship with frontal and medial temporal morphology. World J Biol Psychiatry (2015) 16:249–60. doi: 10.3109/15622975.2014.1000373
3. Pagliaccio D, Wiggins JL, Adleman NE, Harkins E, Curhan A, Towbin KE, et al. Behavioral and Neural Sustained Attention Deficits in Bipolar Disorder and Familial Risk of Bipolar Disorder. Biol Psychiatry (2017) 82:669–78. doi: 10.1016/j.biopsych.2016.09.006
4. Mason L, Eldar E, Rutledge RB. Mood Instability and Reward Dysregulation-A Neurocomputational Model of Bipolar Disorder. JAMA Psychiatry (2017) 74:1275–6. doi: 10.1001/jamapsychiatry.2017.3163
5. Kravariti E, Dixon T, Frith C, Murray R, McGuire P. Association of symptoms and executive function in schizophrenia and bipolar disorder. Schizophr Res (2005) 74:221–31. doi: 10.1016/j.schres.2004.06.008
6. Tabares-Seisdedos R, Balanza-Martinez V, Sanchez-Moreno J, Martinez-Aran A, Salazar-Fraile J, Selva-Vera G, et al. Neurocognitive and clinical predictors of functional outcome in patients with schizophrenia and bipolar I disorder at one-year follow-up. J Affect Disord (2008) 109:286–99. doi: 10.1016/j.jad.2007.12.234
7. Wingo AP, Harvey PD, Baldessarini RJ. Neurocognitive impairment in bipolar disorder patients: functional implications. Bipolar Disord (2009) 11:113–25. doi: 10.1111/j.1399-5618.2009.00665.x
8. Gruber SA, Rosso IM, Yurgelun-Todd D. Neuropsychological performance predicts clinical recovery in bipolar patients. J Affect Disord (2008) 105:253–60. doi: 10.1016/j.jad.2007.04.014
9. Levy B, Medina AM, Manove E, Weiss RD. The characteristics of a discrete mood episode, neuro-cognitive impairment and re-hospitalization in bipolar disorder. J Psychiatr Res (2011) 45:1048–54. doi: 10.1016/j.jpsychires.2011.01.005
10. Yuan P, Raz N. Prefrontal cortex and executive functions in healthy adults: a meta-analysis of structural neuroimaging studies. Neurosci Biobehav Rev (2014) 42:180–92. doi: 10.1016/j.neubiorev.2014.02.005
11. Zhang L, Opmeer EM, van der Meer L, Aleman A, Curcic-Blake B, Ruhe HG. Altered frontal-amygdala effective connectivity during effortful emotion regulation in bipolar disorder. Bipolar Disord (2018) 20:349–58. doi: 10.1111/bdi.12611
12. Strakowski SM, Adler CM, Holland SK, Mills NP, DelBello MP, Eliassen JC. Abnormal FMRI brain activation in euthymic bipolar disorder patients during a counting Stroop interference task. Am J Psychiatry (2005) 162:1697–705. doi: 10.1176/appi.ajp.162.9.1697
13. Curtis VA, Dixon TA, Morris RG, Bullmore ET, Brammer MJ, Williams SC, et al. Differential frontal activation in schizophrenia and bipolar illness during verbal fluency. J Affect Disord (2001) 66:111–21. doi: 10.1016/s0165-0327(00)00240-8
14. McIntosh AM, Whalley HC, McKirdy J, Hall J, Sussmann JE, Shankar P, et al. Prefrontal function and activation in bipolar disorder and schizophrenia. Am J Psychiatry (2008) 165:378–84. doi: 10.1176/appi.ajp.2007.07020365
15. Zang YF, He Y, Zhu CZ, Cao QJ, Sui MQ, Liang M, et al. Altered baseline brain activity in children with ADHD revealed by resting-state functional MRI. Brain Dev (2007) 29:83–91. doi: 10.1016/j.braindev.2006.07.002
16. Lu D, Jiao Q, Zhong Y, Gao W, Xiao Q, Liu X, et al. Altered baseline brain activity in children with bipolar disorder during mania state: a resting-state study. Neuropsychiatr Dis Treat (2014) 10:317–23. doi: 10.2147/ndt.s54663
17. Xu K, Liu H, Li H, Tang Y, Womer F, Jiang X, et al. Amplitude of low-frequency fluctuations in bipolar disorder: a resting state fMRI study. J Affect Disord (2014) 152-154:237–42. doi: 10.1016/j.jad.2013.09.017
18. Gong J, Chen G, Jia Y, Zhong S, Zhao L, Luo X, et al. Disrupted functional connectivity within the default mode network and salience network in unmedicated bipolar II disorder. Prog Neuropsychopharmacol Biol Psychiatry (2019) 88:11–8. doi: 10.1016/j.pnpbp.2018.06.012
19. Lencer R, Yao L, Reilly JL, Keedy SK, McDowell JE, Keshavan MS, et al. Alterations in intrinsic fronto-thalamo-parietal connectivity are associated with cognitive control deficits in psychotic disorders. Hum Brain Mapp (2019) 40:163–74. doi: 10.1002/hbm.24362
20. Raichle ME, MacLeod AM, Snyder AZ, Powers WJ, Gusnard DA, Shulman GL. A default mode of brain function. Proc Natl Acad Sci USA (2001) 98:676–82. doi: 10.1073/pnas.98.2.676
21. Syan SK, Smith M, Frey BN, Remtulla R, Kapczinski F, Hall GBC, et al. Resting-state functional connectivity in individuals with bipolar disorder during clinical remission: a systematic review. J Psychiatry Neurosci (2018) 43:170175. doi: 10.1503/jpn.170175
22. Breukelaar IA, Erlinger M, Harris A, Boyce P, Hazell P, Grieve SM, et al. Investigating the neural basis of cognitive control dysfunction in mood disorders. Bipolar Disord (2019) 22:286–95. doi: 10.1111/bdi.12844
23. Makeig S, Debener S, Onton J, Delorme A. Mining event-related brain dynamics. Trends Cognit Sci (2004) 8:204–10. doi: 10.1016/j.tics.2004.03.008
24. Onton J, Westerfield M, Townsend J, Makeig S. Imaging human EEG dynamics using independent component analysis. Neurosci Biobehav Rev (2006) 30:808–22. doi: 10.1016/j.neubiorev.2006.06.007
25. Britz J, Van De Ville D, Michel CM. BOLD correlates of EEG topography reveal rapid resting-state network dynamics. Neuroimage (2010) 52:1162–70. doi: 10.1016/j.neuroimage.2010.02.052
26. Chang C, Liu Z, Chen MC, Liu X, Duyn JH. EEG correlates of time-varying BOLD functional connectivity. Neuroimage (2013) 72:227–36. doi: 10.1016/j.neuroimage.2013.01.049
27. Nguyen TT, Kovacevic S, Dev SI, Lu K, Liu TT, Eyler LT. Dynamic functional connectivity in bipolar disorder is associated with executive function and processing speed: A preliminary study. Neuropsychology (2017) 31:73–83. doi: 10.1037/neu0000317
28. Raichle ME, Snyder AZ. A default mode of brain function: a brief history of an evolving idea. Neuroimage (2007) 37:1083–90; discussion 1097-9. doi: 10.1016/j.neuroimage.2007.02.041
29. Fu Z, Tu Y, Di X, Du Y, Pearlson GD, Turner JA, et al. Characterizing dynamic amplitude of low-frequency fluctuation and its relationship with dynamic functional connectivity: An application to schizophrenia. Neuroimage (2018) 180:619–31. doi: 10.1016/j.neuroimage.2017.09.035
30. Li J, Duan X, Cui Q, Chen H, Liao W. More than just statics: temporal dynamics of intrinsic brain activity predicts the suicidal ideation in depressed patients. Psychol Med (2019) 49:852–60. doi: 10.1017/S0033291718001502
31. Chao-Gan Y, Yu-Feng Z. DPARSF: A MATLAB Toolbox for “Pipeline” Data Analysis of Resting-State fMRI. Front Syst Neurosci (2010) 4:13. doi: 10.3389/fnsys.2010.00013
32. Liao W, Wu GR, Xu Q, Ji GJ, Zhang Z, Zang YF, et al. DynamicBC: a MATLAB toolbox for dynamic brain connectome analysis. Brain Connect (2014) 4:780–90. doi: 10.1089/brain.2014.0253
33. Leonardi N, Van De Ville D. On spurious and real fluctuations of dynamic functional connectivity during rest. Neuroimage (2015) 104:430–6. doi: 10.1016/j.neuroimage.2014.09.007
34. Xu H, Su J, Qin J, Li M, Zeng LL, Hu D, et al. Impact of global signal regression on characterizing dynamic functional connectivity and brain states. Neuroimage (2018) 173:127–45. doi: 10.1016/j.neuroimage.2018.02.036
35. Kosger F, Essizoglu A, Baltacioglu M, Ulkgun N, Yenilmez C. Executive function in parents of patients with familial versus sporadic bipolar disorder. Compr Psychiatry (2015) 61:36–41. doi: 10.1016/j.comppsych.2015.05.013
36. Altshuler LL, Ventura J, van Gorp WG, Green MF, Theberge DC, Mintz J. Neurocognitive function in clinically stable men with bipolar I disorder or schizophrenia and normal control subjects. Biol Psychiatry (2004) 56:560–9. doi: 10.1016/j.biopsych.2004.08.002
37. Erol A, Kosger F, Putgul G, Ersoy B. Ventral prefrontal executive function impairment as a potential endophenotypic marker for bipolar disorder. Nordic J Psychiatry (2013) 68:18–23. doi: 10.3109/08039488.2012.756062
38. Jurado MB, Rosselli M. The elusive nature of executive functions: a review of our current understanding. Neuropsychol Rev (2007) 17:213–33. doi: 10.1007/s11065-007-9040-z
39. Greicius MD, Menon V. Default-mode activity during a passive sensory task: uncoupled from deactivation but impacting activation. J Cognit Neurosci (2004) 16:1484–92. doi: 10.1162/0898929042568532
40. Bai T, Wei Q, Zu M, Xie W, Wang J, Gong-Jun J, et al. Functional plasticity of the dorsomedial prefrontal cortex in depression reorganized by electroconvulsive therapy: Validation in two independent samples. Hum Brain Mapp (2019) 40:465–73. doi: 10.1002/hbm.24387
41. Broyd SJ, Demanuele C, Debener S, Helps SK, James CJ, Sonuga-Barke EJ. Default-mode brain dysfunction in mental disorders: a systematic review. Neurosci Biobehav Rev (2009) 33:279–96. doi: 10.1016/j.neubiorev.2008.09.002
42. Wise T, Marwood L, Perkins AM, Herane-Vives A, Joules R, Lythgoe DJ, et al. Instability of default mode network connectivity in major depression: a two-sample confirmation study. Transl Psychiatry (2017) 7:e1105. doi: 10.1038/tp.2017.40
43. Zhong S, Chen G, Zhao L, Jia Y, Chen F, Qi Z, et al. Correlation between Intrinsic Brain Activity and Thyroid-Stimulating Hormone Level in Unmedicated Bipolar II Depression. Neuroendocrinology (2019) 108:232–43. doi: 10.1159/000497182
44. Ongur D, Lundy M, Greenhouse I, Shinn AK, Menon V, Cohen BM, et al. Default mode network abnormalities in bipolar disorder and schizophrenia. Psychiatry Res (2010) 183:59–68. doi: 10.1016/j.pscychresns.2010.04.008
45. Goya-Maldonado R, Brodmann K, Keil M, Trost S, Dechent P, Gruber O. Differentiating unipolar and bipolar depression by alterations in large-scale brain networks. Hum Brain Mapp (2016) 37:808–18. doi: 10.1002/hbm.23070
46. Martino M, Magioncalda P, Huang Z, Conio B, Piaggio N, Duncan NW, et al. Contrasting variability patterns in the default mode and sensorimotor networks balance in bipolar depression and mania. Proc Natl Acad Sci USA (2016) 113:4824–9. doi: 10.1073/pnas.1517558113
47. Chen CY, Muggleton NG, Tzeng OJ, Hung DL, Juan CH. Control of prepotent responses by the superior medial frontal cortex. Neuroimage (2009) 44:537–45. doi: 10.1016/j.neuroimage.2008.09.005
48. Rushworth MF, Hadland KA, Paus T, Sipila PK. Role of the human medial frontal cortex in task switching: a combined fMRI and TMS study. J Neurophysiol (2002) 87:2577–92. doi: 10.1152/jn.2002.87.5.2577
49. Joshi SH, Vizueta N, Foland-Ross L, Townsend JD, Bookheimer SY, Thompson PM, et al. Relationships Between Altered Functional Magnetic Resonance Imaging Activation and Cortical Thickness in Patients With Euthymic Bipolar I Disorder. Biol Psychiatry Cognit Neurosci Neuroimaging (2016) 1:507–17. doi: 10.1016/j.bpsc.2016.06.006
50. Magioncalda P, Martino M, Conio B, Escelsior A, Piaggio N, Presta A, et al. Functional connectivity and neuronal variability of resting state activity in bipolar disorder–reduction and decoupling in anterior cortical midline structures. Hum Brain Mapp (2015) 36:666–82. doi: 10.1002/hbm.22655
51. Andrews-Hanna JR, Snyder AZ, Vincent JL, Lustig C, Head D, Raichle ME, et al. Disruption of large-scale brain systems in advanced aging. Neuron (2007) 56:924–35. doi: 10.1016/j.neuron.2007.10.038
52. Calhoun VD, Maciejewski PK, Pearlson GD, Kiehl KA. Temporal lobe and “default” hemodynamic brain modes discriminate between schizophrenia and bipolar disorder. Hum Brain Mapp (2008) 29:1265–75. doi: 10.1002/hbm.20463
53. Meda SA, Ruano G, Windemuth A, O’Neil K, Berwise C, Dunn SM, et al. Multivariate analysis reveals genetic associations of the resting default mode network in psychotic bipolar disorder and schizophrenia. Proc Natl Acad Sci (2014) 111:E2066–75. doi: 10.1073/pnas.1313093111
54. Rey G, Desseilles M, Favre S, Dayer A, Piguet C, Aubry JM, et al. Modulation of brain response to emotional conflict as a function of current mood in bipolar disorder: preliminary findings from a follow-up state-based fMRI study. Psychiatry Res (2014) 223:84–93. doi: 10.1016/j.pscychresns.2014.04.016
55. Kucyi A, Salomons TV, Davis KD. Mind wandering away from pain dynamically engages antinociceptive and default mode brain networks. Proc Natl Acad Sci USA (2013) 110:18692–7. doi: 10.1073/pnas.1312902110
56. Wang J, Wang Y, Huang H, Jia Y, Zheng S, Zhong S, et al. Abnormal dynamic functional network connectivity in unmedicated bipolar and major depressive disorders based on the triple-network model. Psychol Med (2020) 50:465–74. doi: 10.1017/s003329171900028x
57. Chen T, Cai W, Ryali S, Supekar K, Menon V. Distinct Global Brain Dynamics and Spatiotemporal Organization of the Salience Network. PloS Biol (2016) 14:e1002469. doi: 10.1371/journal.pbio.1002469
58. Leech R, Sharp DJ. The role of the posterior cingulate cortex in cognition and disease. Brain (2014) 137:12–32. doi: 10.1093/brain/awt162
Keywords: bipolar disorder, executive function, dynamic local activity, dynamic functional connectivity, posterior cingulate cortex, medial prefrontal cortex
Citation: Liang Y, Jiang X, Zhu W, Shen Y, Xue F, Li Y and Chen Z (2020) Disturbances of Dynamic Function in Patients With Bipolar Disorder I and Its Relationship With Executive-Function Deficit. Front. Psychiatry 11:537981. doi: 10.3389/fpsyt.2020.537981
Received: 05 March 2020; Accepted: 02 September 2020;
Published: 24 September 2020.
Edited by:
Neeltje E. M. Van Haren, Erasmus University Rotterdam, NetherlandsReviewed by:
Luke Norman, University of Michigan, United StatesYanbin Jia, First Affiliated Hospital of Jinan University, China
Copyright © 2020 Liang, Jiang, Zhu, Shen, Xue, Li and Chen. This is an open-access article distributed under the terms of the Creative Commons Attribution License (CC BY). The use, distribution or reproduction in other forums is permitted, provided the original author(s) and the copyright owner(s) are credited and that the original publication in this journal is cited, in accordance with accepted academic practice. No use, distribution or reproduction is permitted which does not comply with these terms.
*Correspondence: Zhiyu Chen, aHpjenk1MzZAMTYzLmNvbQ==
†These authors have contributed equally to this work