- 1Human Genetics and Cognitive Functions, Institut Pasteur, UMR3571 CNRS, Université de Paris, Paris, France
- 2Child and Adolescent Psychiatry Department, Robert Debré Hospital, Paris, France
- 3Parietal Project-Team, INRIA Saclay – Île de France, Palaiseau, France
- 4Human Brain and Behavior Laboratory, Center for Complex Systems and Brain Sciences, Florida Atlantic University, Boca Raton, FL, United States
- 5Intelligent Systems Research Centre, University of Ulster, Derry Londonderry, United Kingdom
- 6Department of Psychiatry, Université de Montréal, Montreal, QC, Canada
- 7CHU Sainte-Justine Centre de Recherche, Precision Psychiatry and Social Physiology Laboratory, Montreal, QC, Canada
The human dynamic clamp (HDC) is a human–machine interface designed on the basis of coordination dynamics for studying realistic social interaction under controlled and reproducible conditions. Here, we propose to probe the validity of the HDC as a psychometric instrument for quantifying social abilities in children with autism spectrum disorder (ASD) and neurotypical development. To study interpersonal synchrony with the HDC, we derived five standardized scores following a gradient from sensorimotor and motor to higher sociocognitive skills in a sample of 155 individuals (113 participants with ASD, 42 typically developing participants; aged 5 to 25 years; IQ > 70). Regression analyses were performed using normative modeling on global scores according to four subconditions (HDC behavior “cooperative/competitive,” human task “in-phase/anti-phase,” diagnosis, and age at inclusion). Children with ASD had lower scores than controls for motor skills. HDC motor coordination scores were the best candidates for stratification and diagnostic biomarkers according to exploratory analyses of hierarchical clustering and multivariate classification. Independently of phenotype, sociocognitive skills increased with developmental age while being affected by the ongoing task and HDC behavior. Weaker performance in ASD for motor skills suggests the convergent validity of the HDC for evaluating social interaction. Results provided additional evidence of a relationship between sensorimotor and sociocognitive skills. HDC may also be used as a marker of maturation of sociocognitive skills during real-time social interaction. Through its standardized and objective evaluation, the HDC not only represents a valid paradigm for the study of interpersonal synchrony but also offers a promising, clinically relevant psychometric instrument for the evaluation and stratification of sociomotor dysfunctions.
Introduction
Autism spectrum disorder (ASD) is a complex neurodevelopmental disorder (1) defined by the co-occurrence of social communication problems, repetitive behaviors, and restricted interests. The prevalence of ASD has increased in recent years from <1 in 1,000 individuals to 1 in 58 (2, 3). With different levels of severity of symptoms, ASD is highly heterogeneous, both phenotypically (4) and genetically (5). More than 50% of patients suffer from at least four other psychiatric comorbid conditions (6). This strong heterogeneity complicates the development of psychometric assessment tools that allow for a personalized and thorough evaluation of a child's skills (7). Identification of robust, valid, and quantitative biomarkers of social communication disability, a key symptom of ASD, is thus a major societal challenge for improving early diagnosis and individualized care.
As a keystone of social communication, interpersonal synchrony (IS) is a fundamental aspect to explore in order to better understand and apprehend ASD. IS can be defined as a rhythmic matching of actions in time and in phase with another person based on nonverbal behaviors (8). IS comprises multiple components, involving sociocognitive, sensory motor, and motor skills, as well as adaptive capacities (9, 10). At the behavioral level, IS can be measured through microlevel detection of bonding-related behaviors (11), frame-by-frame analysis of video (12), or even using machine learning tools (13).
In this context, the human dynamic clamp (HDC) is a new paradigm of human–machine interaction based on the science of coordination (coordination dynamics) that enables the study of the neurobehavioral processes involved in IS (14–16). Controlled using empirically grounded models of coordination dynamics (17), the HDC allows a dynamic bidirectional interaction in real time between a human and a virtual avatar. The HDC paradigm has already been validated empirically in adults (15, 18, 19). Using high-resolution electroencephalography, it recently revealed how distributed neural dynamics integrate information from “low-level” sensorimotor mechanisms and “high-level” sociocognitive processes such as intention attribution or judgment of humanness (18). Using skin potential responses, we demonstrated that HDC is able to induce emotional reaction, especially when human participants believed that their partner was human and when movement coordination was stable (19). Finally, we also introduced the virtual teacher (VT) configuration that allows human participants to change their behavioral repertoire by internalizing new interpersonal coordination patterns (e.g., nontrivial relative phase between movements of the two interacting partners), thereby opening possibilities of applying HDC to rehabilitation (15).
IS seems to be substantially impaired in children and adolescents with ASD (20, 21). A few studies among children (6–11 years old) (22) and adolescents (10–16.5 years old) (23) have explored IS in automated motion analysis to quantify movements of body parts. Still, the exploratory paradigms are mainly rhythmic in children with ASD (3.5–10 years old) (24–27) and in adolescents (12–17 years old) (28, 29). However, even if children with ASD face difficulties in movement coordination during a social exchange, social embodiment seems preserved and appears to correlate with social cognitive ability (22).
One hypothesis currently under investigation suggests that motor and sensory motor skill development are linked to social cognition and cognitive development (25, 30). ASD is frequently found to be associated with difficulties in attributing mental states to oneself and to others (31), where intention attribution is characterized by an appraisal based on the intention underlying someone else's action (32). In addition to primary dysfunctions in social communication skills, deficits in perceptual–motor performance are found in between 50 and 80% of children diagnosed with ASD (moving with awareness, integrated self, proprioceptive feedback, visuo-perceptual performance, sensory integration) (23, 25, 33–37). About 80% also show motor skill impairments such as praxis, basic motor control, postural control, gait abnormalities, motor coordination, manual dexterity, gross and fine motor skills, and gestures in complex movement sequences (20, 25, 38).
Interventions targeting the development of IS are promising and show evidence for plasticity (39–41). Early detection and intervention directly focusing on the development of IS showed preliminary evidence of positive effects on motor and communication skills (42), especially later in both language and social abilities (39). Such evidence supports IS as a potent tool for the diagnosis and care of ASD children.
Up to now, language, cognitive ability, social engagement, and motor skills have emerged as the most robust predictors of ASD among toddlers (43–45) and during childhood and adolescence (46). Thus, early dysfunction in IS could have cascading consequences and even participate in explaining the heterogeneity of ASD. Such observations reflect the difficulty of assessment by means of reliable and age-scalable markers of IS and the need for personalized analysis [as has been done, for example, in studies of skill learning, cf. (47)].
In the present work, we first validate how the HDC measures different behavioral processes involved in social dynamic interactions in children with neurotypical development, and then evaluate how the HDC can assess IS alterations in children with ASD. A secondary objective is to standardize the test and develop indicators that measure and identify sociocognitive and sensorimotor markers. In order to highlight the specific heterogeneity of ASD compared to typical neurodevelopment, developmental trajectories are integrated into our analysis using normative modeling (48), and HDC behavioral measures are tested as reproducible and reliable clinical markers.
Methods
Sample
We enrolled in the study a sample of 156 individuals composed of 114 participants with ASD and 42 participants with typical development (Table 1). All participants were recruited at the Child Psychiatry Department of the Robert Debré University Hospital, Paris (France).
Patients with ASD were included after a systematic clinical and medical examination, including negative blood test results for Fragile-X and the exclusion of participants carrying a large deletion over 2 Mb as detected by the Illumina 700 SNPs array. The final diagnosis of ASD was based on DSM-5 criteria and outcomes from the Autism Diagnostic Observation Schedule-Second Edition (ADOS-II) (49), the Autism Diagnostic Interview-Revised (ADI-R) (50), and the Social Responsiveness Scale−2nd edition (SRS-2) (51) for the dimensional diagnosis of social skills and data from experts in the field. Intellectual functioning for all participants was estimated using the Wechsler Intelligence Scale for Children and Adolescents−5th edition (WISC-V) (52). The current threshold for intellectual disability (i.e., IQ <70) was used, following international standards (DSM-5). Among participants, 14 children (controls = 2, ASD = 12) were below 85 and 33 (controls = 14, ASD = 19) were above 115. Participants with normal neurotypical development were from the general population and reported no personal or familial history of ASD or axis I psychiatric conditions requiring specific needs.
An assessment of dexterity and motor coordination of hands and fingers was made using the Purdue Pegboard (53). For the present study, we used the versions with charts defined on a population aged 5 to 15 years 11 months and beyond the age of 16 years (54, 55). Only the “preferred hand score,” viz. place the most items using the preferred hand in a row in 30 s, was conserved. A z score was calculated according to age and gender. Children were also assessed with the Child Neuropsychological Assessment—second edition (NEPSY-II) (56) to specifically explore affect recognition (AF) and theory of mind (TOM).
The research was carried out in accordance with the recommendations of the local ethics committee of Hospital Robert Debré. All the parents of participants gave written informed consent in accordance with the Declaration of Helsinki. The protocol was approved by the INSERM Ethics Committee (study approval no. 08-029).
The Human Dynamic Clamp Paradigm
The HDC system (14–16) is a human–machine interface consisting of three parts: (1) a sensor measuring the movement of the participant's index finger which is fed into (2) a mathematical model integrating the position and velocity of the human's movement to simulate in real time [via the HKB model; (57)] the behavior of a virtual partner or avatar and 3) a screen facing the participant where the resulting finger movements of the virtual partner (VP) appear as a human hand. The HDC software computes in real time the corresponding position of the VP (Figure 1). At the beginning of each trial, an instruction was given to the participant to synchronize her/his movement in-phase (i.e., synchronize her/his movements to those of the VP) or anti-phase (i.e., synchronize her/his movements with a half-period offset with the VP's phase). In this experiment, while the partner is a virtual partner throughout, all participants were instructed that half the time the partner is virtual (i.e., movements are computer-driven) and half the time the partner is a real sex- and age-matched human performing the same task in another room of the hospital. The protocol was composed of 40 trials, divided into four blocks. The instructions to the participant stayed the same within each block. The instruction for the first block was randomly assigned at the beginning of the experiment. During the trials, the VP could adopt a “cooperative” or “competitive” behavior, meaning that it shares the same goal or the opposite goal to the one assigned to the participant (i.e., VP aims to move its finger in in-phase coordination when the participant aims to move his finger in anti-phase and vice versa, exactly as if the partner was not cooperating with but in opposition to the participant). Behavior of the VP was randomized across all trials, disregarding block structure. At the end of each trial, the participant was asked if s/he felt like s/he was playing with a human or a VP and to quantify the level of cooperativeness or competitiveness of the partner (see also 88).
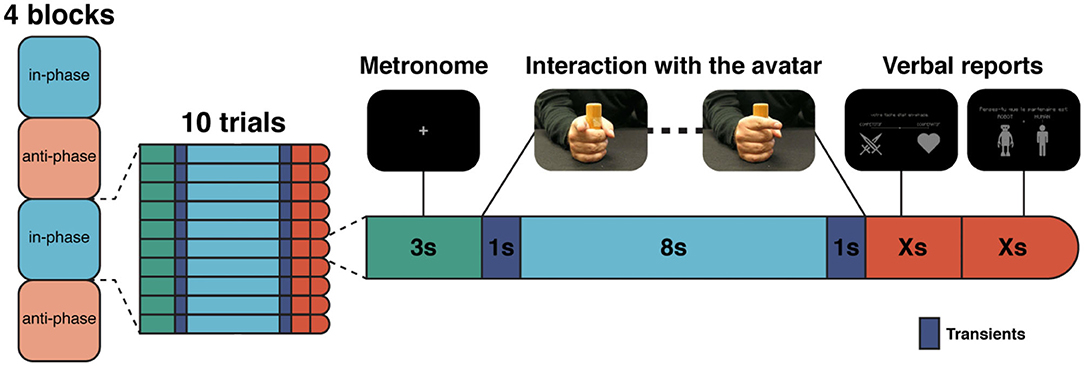
Figure 1. Experimental design. Structure of the protocol with four blocks alternating “in-phase/anti-phase.” Each block is divided into 10 trials (left). Each trial starts with the participant instructed to synchronize with the sound of a metronome for 3 s. Then, the participant interacts with the avatar according to the instruction (e.g., “in-phase”). At the end of the trial, two questions appear directly on the screen. First, the impression of the participant on the competitive or cooperative behavior of the avatar, and second regarding the humanness of the avatar (right). Shown are screenshots of what participants could see (top). In-phase refers to synchronized movements using homologous muscles of the limbs and anti-phase to alternating movements (180° out of phase). Cooperative and competitive refer respectively to the behavior of the virtual partner when it has shared or conflicting goals with the human participant.
HDC Behavioral Measures
In the present study, five normalized scores (between 0 and 1, 0 being the worst) of the HDC paradigm were automatically aimed at evaluating dimensions of social cognition, ranging from sensory motor to representational aspects: (1) a motor score which measures the difference of amplitude of imitative gestures between the participant and the VP; (2) a coordination score which corresponds to the temporal index of imitation; (3) a task score which is based on how well the ongoing relative phase of the VP and the participant match, taking into account the task condition; (4) an intention score which evaluates the ability of the participant to properly attribute intention toward the “cooperative” or “competitive” behavior of the VP; and (5) a humanness score which reflects quantitatively the impression of the participant on the human or robotic character of the partner (see Supplementary Material for more details).
Data Analysis Using Normative Modeling
All statistical data analyses were performed using Python 3.7 (58) [numpy 1.17.2 (59, 60) and scipy 1.3.1 (61)]. Normative modeling (NM) provides a metric similar to a z score, but accounts for the underlying structure of the population across multiple covariates. NM uses Gaussian processes (GP) to model the distribution of control group measures while estimating separately the overall trajectory in the covariate space, the heterogeneity in the population, and the uncertainty of the fit (62). The Python code is available in open access at https://github.com/GHFC/SoNeTAA/.
Results
Sociodemographic and Group Comparative Analyses
Overall, participants with ASD were younger than the control group [t(154) = 2.6, p = 2.6e-11], with a larger male/female ratio (Fisher exact, p = 0.002) than the control group. No statistically significant differences were found for IQ and handedness. As expected, the group with ASD scored higher in the SRS [t(154) = 14.3, p = 7.4e-29]. No statistically significant differences were found for IQ and handedness. The group with ASD scored lower on all the standardized psychometric instruments assessing social skills: NEPSY-II TOM total score (Mann–Whitney U = 104.5, p = 0.0005), NEPSY-II AF raw (U = 114, p = 0.0017), and the Purdue Pegboard, the validated task assessing motor coordination skills (U = 138, p = 0.0006).
Developmental Trajectories of HDC Scores
Within the entire cohort (both groups of participants with ASD and with typical development), a developmental trajectory was found with a statistically significant correlation of age with task comprehension (r = 0.33; p = 2.7e-05) (Figure 2A), intention attribution (r = 0.30; p = 0.00011) (Figure 2B), and humanness (r = 0.27; p = 0.00057) (Figure 2C). Only a few children with ASD diagnosis answered systematically the same rating of humanness across the whole experiment (N = 3 always human, i.e., humanness score = 1; N = 3 always robot, i.e., humanness score = 0). A significant interaction was observed between chronological age and comprehension score only in the control group (r = 0.40; p = 0.0084) (Figure 2A) (the older the participant is, the better the skills are) and with intention attribution (r = 0.21; p = 0.024) and humanness (r = 0.38; p = 3.8e-0.5) in the group with ASD.
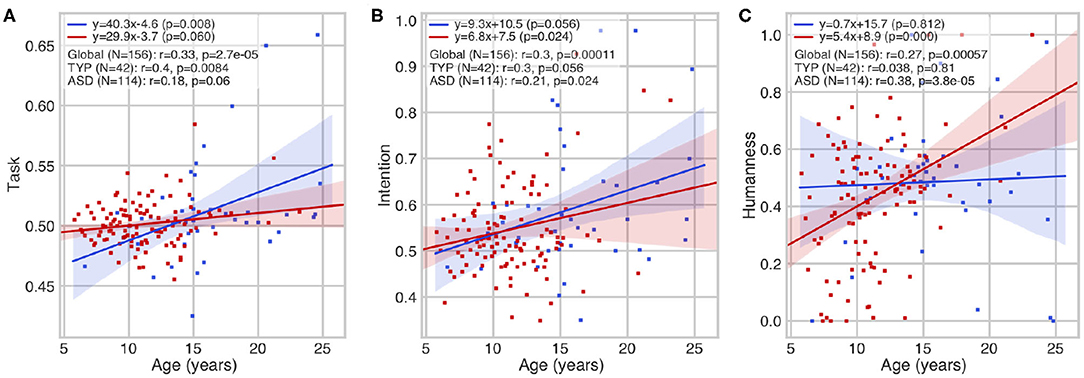
Figure 2. Developmental aspect of higher-level correlates of interpersonal synchrony (IS). Correlations between age at inclusion and task comprehension (A), intention attribution (B), and humanness (C) scores. The three scores show a remarkable positive correlation with age, suggesting a developmental trajectory of sociocognitive skills. No outliers were removed.
Comparison With Standardized Tests Using Normative Models
Using normative modeling allows us to correct any developmental bias on the HDC scores. We were then able to observe how these “age-controlled HDC scores” related to standard neuropsychological tests (Table 2).
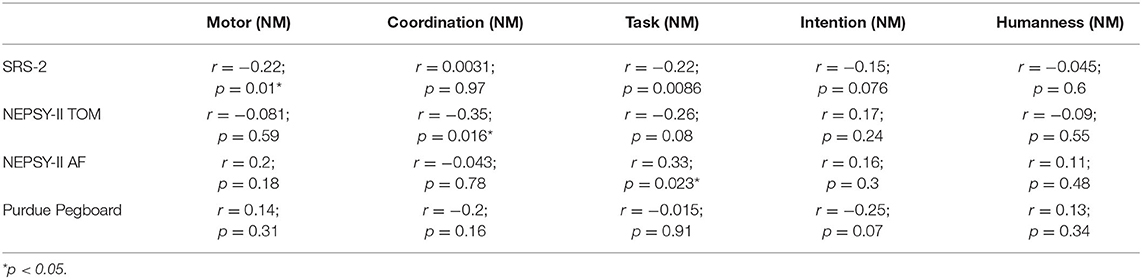
Table 2. Summary of the main correlations between HDC scores and those from the NEPSY-II [affect recognition (AF) and theory of mind (TOM) subdomains], the Social Responsiveness Scale—second edition (SRS-2), and the Purdue Pegboard.
We observed a significant interaction effect between the SRS-2 and motor score (r = −0.22; p = 0.01) (Figure 3A); high SRS scores (in favor of the diagnosis of ASD) are correlated with low motor scores. The NEPSY-II test showed a significant interaction effect between AF score and the HDC task comprehension score (r = 0.33; p = 0.02) (Figure 3B); good skills in the AF task of the NEPSY-II are associated with good scores at the HDC task comprehension score. The Supplementary Data Sheet contain the details of the correlation per group, along with the HDC scores before normative modeling correction.
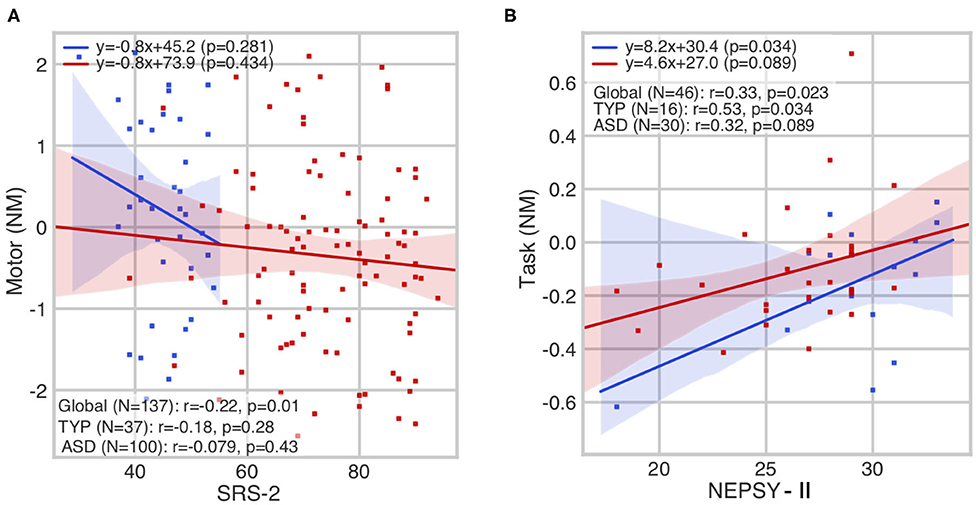
Figure 3. Significant correlations between sociocognitive and motor skills in children with (in red) autism spectrum disorder (ASD) or with typical development (TYP) (in blue): (A) SRS-2 vs. motor score: a dimensional diagnosis of ASD correlates with lower levels of motor skills; and (B) NEPSY-II affect recognition (AF) vs. HDC task score: greater cognitive abilities correlate with higher levels of affect recognition skill; NM, normative models.
Global Comparative Analysis Between Participants With ASD and Typical Development Groups Using Normative Models
Comparative analysis between the two groups revealed a statistically significant decrease of the motor score (d = −0.5; p = 0.0029) in individuals with an ASD diagnosis compared with individuals with typical development. We also observed evidence of better understanding of the task among participants with ASD diagnosis compared with those with typical development (d = 0.23; p = 0.0077). Interactions between the two groups for the other scores (coordination: d = −0.21, p = 0.12; intention: d = −0.12, p = 0.49; humanness: d = 0.12, p = 0.19) were not significant (Figure 4).
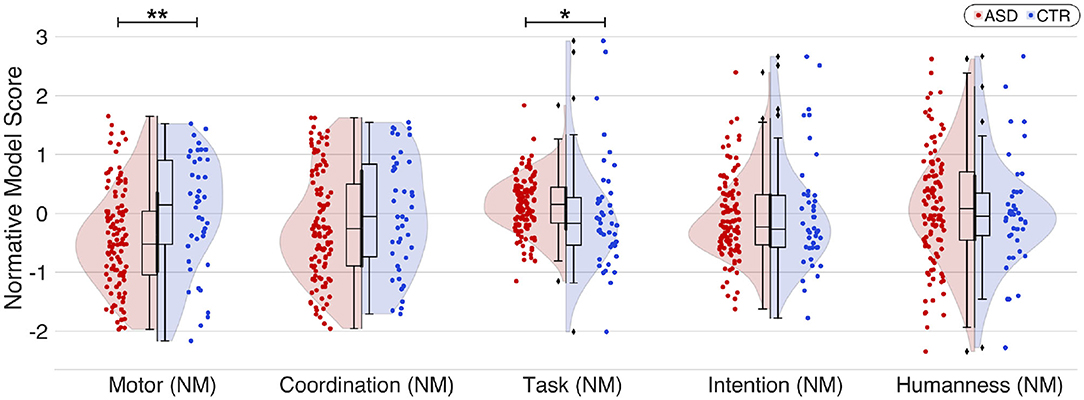
Figure 4. Comparison between the two groups with ASD and typical development for different behavioral scores derived from the HDC protocol and corrected with normative modeling (NM). Only the motor score really discriminates between the two populations (d = −0.5; p < 0.005**), with significantly lower results among ASD. The lines represent linear regressions. Colored areas: 95% confidence intervals (CI). Neurotypical participants: blue; participants with ASD: red. *p < 0.05; **p < 0.005.
HDC Scores Analysis by Subconditions
Different subconditions are associated with the HDC paradigm: the diagnosis, the age, the avatar behavior, and the humanness or robotic character of the HDC (see Figure 5 for a summary). Multiple regression was thus calculated to predict the different normalized HDC scores based on the diagnosis (coded as 0 = ASD and 1 = CTR), age (in years), avatar behavior (coded as 0 = competitive and 1 = cooperative), and the humanness or robotic character discrimination task (coded as 0 = anti-phase and 1 = in-phase).
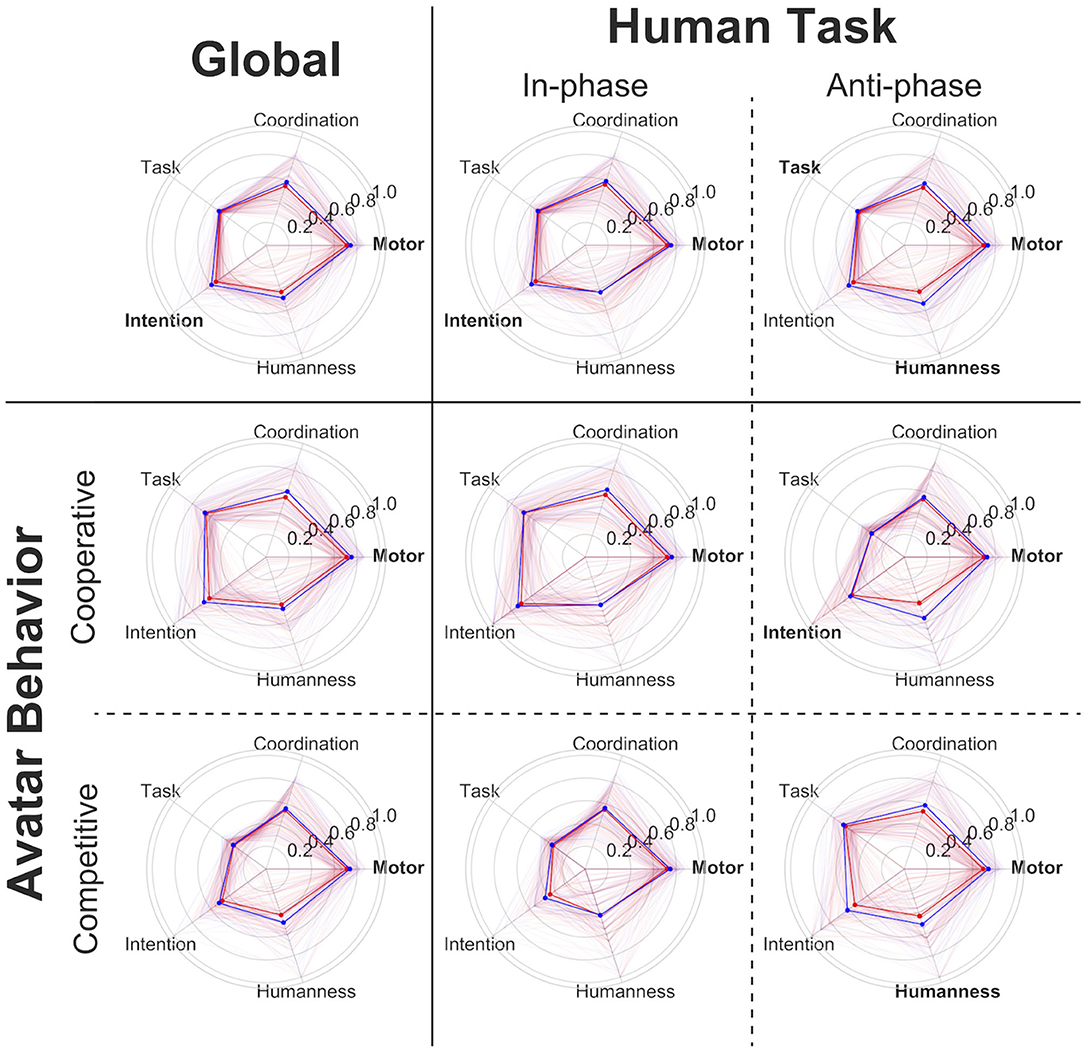
Figure 5. Score analysis by subcondition of the two groups (human task: in-phase/anti-phase and avatar behavior: cooperation/competition). Both the global and the human task were significant predictors of the motor score, with the control group having greater scores (coeff = 0.4413, p < 0.001**) and “in-phase” task also leading to higher scores (coeff = 0.2726, p = 0.016*). Controls are in blue and participants with ASD in red. Scores with statistically significant differences between participants with ASD and participants with typical development are in bold typeface. *p < 0.05; **p < 0.005.
We found a significant regression equation for the motor score [F(5, 618) = 7.634, p = 5.64e-07]. Both the diagnostic and the human task were significant predictors of the motor score, with the control group having higher scores (coeff = 0.44, p < 0.001), as well as the in-phase task (coeff = 0.27, p = 0.016). There was also a significant regression equation for the coordination score [F(5, 618) = 3.252, p = 0.006], with age, as might be expected, a significant predictor (coeff = 0.0272, p = 0.02). A significant regression equation for task score [F(5, 618) = 409.1, p = 2.42e-193] revealed that avatar behavior was a significant predictor, with a cooperative behavior of the VP having a huge effect on the task comprehension of participants (coeff = 6.96, p < 0.001). Further analysis of a significant regression equation for the intention score [F(5, 618) = 28.84, p = 2.46e-26] showed that both human task and avatar behavior were significant predictors of VP intention. Participants tended to better detect the intention of the VP while “anti-phase” (coeff = −1.27, p < 0.001), and “in-phase” if the VP takes on a cooperative behavior (coeff = 2.31, p < 0.001).
For the motor score, detailed analysis indicates that during both VP “cooperative” (“in-phase”: d = −0.51; p = 0.006 and “anti-phase”: d = −0.59; p = 0.002) and “competitive” behavior (“in-phase”: d = −0.34; p = 0.025 and “anti-phase”: d = −0.35; p = 0.025), the task allows to distinguish the two groups (cf. Table 3).
Discussion
Aim of the Study
The main objective of the study was to identify which HDC scores distinguish our two populations of children with and without a diagnosis of ASD and, thus, provide potential predictors of the condition. A particularly interesting aspect is that our results show the motor score discriminates between the two groups. Motor abnormalities in the disorder are widely described. However, they are still currently little taken into account in the diagnosis. As a reminder, the HDC is validated in adults as an instrument to artificially recreate a social interaction, from low-level (motor and coordination scores) to higher-level domains of social coordination [intention attribution to another and human (/or robotic) judgment of an interaction]. Our secondary objective was to study the developmental trajectory of HDC scores and to demonstrate by a valid scientific approach that interpersonal synchrony captures the coupling between low-level sensorimotor and high-level sociocognitive skills in a population of children.
Developmental Aspects of Sociocognitive Skills and Intervention Based on Interpersonal Synchrony in Children With ASD
The literature on interpersonal synchrony attests to the significance of development and plasticity in affording therapeutic detection and action (39, 41, 43). The present HDC results are in line with this developmental aspect of sociocognitive skills, with significant effects of intention attribution, humanness, and task comprehension in children and adolescents with and without ASD. Interventions targeting early development of socially synchronous interactions in toddlers with ASD attest to its effectiveness (39), with improvement in child language comprehension being linked to the severity of ASD symptoms (63). The neurodevelopmental trajectory observed here only in the group with ASD is fully in line with this picture.
Coupling Between Low-Level Sensorimotor and High-Level Sociocognitive Skills
The present findings also show that affect recognition may be associated with better task comprehension. Greater cognitive abilities are correlated with a higher level of affect recognition skill hinting at the possibility of a mediating effect of IQ on the recognition of emotions. At the same time, we found that lower motor skills are associated with a higher probability of a dimensional diagnosis of ASD. Motricity in ASD will be discussed further, but this result suggests a linkage between the so-called “lower-level” motor skills and “higher-level” social–cognitive skills in this population. Some support already exists for a strong pairing between the mirror and mentalizing systems during communicative gestures, suggesting a cognitive–motor coupling in children (64). The mechanisms involved range from the release of endogenous opioids (dopamine, endorphins, serotonin, and oxytocin) (65, 66) to the recruitment of now well-described neural processes (67, 68). From an evolutionary perspective, IS is thought to play a role in shared common goals that lead to: a) cooperative expectations and joint action behaviors (69); b) shared basic affective states and emotions; c) better attribution ability of one's self and others; and d) in general, better comprehension of social situations (70).
Motor Skills as a Developmental Marker of Children and Adolescents at Risk With ASD
The motor score is the only HDC measure that allows a distinction between the two groups. In overall terms, this motor low-level score is found to be statistically lower among participants with ASD, confirming current data finding altered motor skills in ASD. Despite the small sample size, it is interesting to note that the HDC motor score is also one of the two scores (together with the motor coordination score) on which the classification into two clusters is essentially based—a classification that significantly respects the status of participants (see Supplementary Material) (71). These results demonstrate the essential nature of motor assessment, including the use of HDC, in participants with ASD, suggesting a major role in ASD diagnosis (72). Alterations in motor control (38), and particularly of executive motor control (73), have been widely demonstrated in children with ASD. However, although motor disorders are associated with the diagnosis of children with ASD in 50 to 80% (74), their prevalence apparently increasing with age (75), they remain underdiagnosed in clinical practice (1.34%) (75). On the other hand, the estimated prevalence of motor disorders (36%) makes them almost as frequent as cognitive disorders (38%) among children under 6 years (75). Children and adolescents with ASD tend to have difficulties in planning and sequencing movements (76), which are also associated with higher levels of neuromotor noise (77) [i.e., disturbing action (motor commands) and perception (sensory feedback) (20)]. Such variability can have multiple substrates but relies on hypotheses that can be explained using Bayesian models, namely an imbalance between prediction, inputs, and expectations (78). Indeed, ASD is associated with alterations in the ability to integrate social stimuli (79) and a reduced ability to incorporate somatosensory and visual information into accurate motor responses (37). Moreover, some studies now describe deficits in joint-attention as an endophenotype of ASD (80, 81). Vis-à-vis our results, children with ASD may have difficulties in sustaining attention long enough to perceive the stimulus. Further analysis showed that the instruction given to the participant (human task: “in-phase”) is associated with a better motor score among the control group (coeff = 0.2726, p = 0.016*). Also, we observed interactions by subcondition. Children with ASD tend to have lower results than children with typical development in all the conditions, i.e., “in-phase” as well as “anti-phase” during both competitive and cooperative behavior of the avatar (Table 3). This result accords with Wang et al. (82) who reported that during a cooperative task of synchronization (i.e., “in-phase”), children with severe diagnosis of ASD tend to exhibit lower neural activity.
Sociocognitive Skills Based on Interpersonal Synchrony
Mentalizing deficits have repeatedly been described in the population with ASD (83). Mentalizing requires preserved metacognitive skills, yet metacognitive monitoring is found diminished in children with ASD (84). Higher-level scores (intention attribution and task comprehension) demand efficient use of metacognitive processes. However, for both scores, we did not observe a group effect: it seemed easier for participants to detect the intention of the VP when it takes on a cooperative behavior whether “anti-phase” or “in-phase”. Furthermore, comprehension of the task is better if the avatar is cooperative. A result that seems difficult to interpret is that participants with an ASD diagnosis appear to have a better understanding of the task than controls. This could be due to the tendency of ASD children to generate mainly the same movement “in-phase” with the avatar without taking the instruction into account–or it could be due to the lack of a real-time social context (85). Such a possibility of bias may produce a false positive result.
In addition, only children with a diagnosis of ASD showed a persistence of the same response in the assessment of the attribution of humanness (or robotic) judgment to the avatar. This result reinforces the previous observation of an insistence on sameness in ASD (86, 87) and may be consistent with the repetitive behaviors that are part of the diagnosis (DSM-5).
Implications of Findings for Clinical Practice and Public Health
One of the challenges of the present approach is to develop an application of HDC that can be used for the early assessment and training of motor coordination and interpersonal synchrony in order to improve social skills (42). The aim of our study was also to offer standardized ways to assess the efficacy of the HDC. We were able to generate percentile ranks for each HDC score from the results obtained in control patients (see Supplementary Table 1). This step made it possible to estimate a child's skills for each assessment.
Research has tried to identify clinical markers in ASD ranging from early signs of regression patterns (44, 88) to atypical neural responses of gaze (89). Later possibilities include neurological soft signs (90), abnormalities of sensorimotor priors (34), and anomalies in proprioceptive and sensory motor development (including alteration of motor priors, micromovements, and the presence of noise in sensory motor variables that may be associated with lack of embodiment) (34).
Systematic and reliable metrics of the HDC, normalized across developmental trajectories by means of normative models (91), could help predict phenotypic profiles and, thus, refine the diagnosis, associated comorbidities, and stratification of the disorder. Here, we highlighted how HDC measures can provide new markers of ASD, either alone or in combination with psychometric scales for assessing social and motor coordination skills. Despite the limited sample size, exploratory analyses of stratification and multivariate predictive diagnosis (see Supplementary Material for more details on the methodology and the preliminary results) tend to confirm the potential of the HDC paradigm for ASD diagnostics with the motor and coordination scores, individually, and/or combined with other clinical evidence of ASD, appearing as the most promising candidates. Clearly, there is a need to develop dedicated HDC-based predictive models and for further data collection and analyses to be carried out to assess them rigorously (92). Many studies have reported divergences in the core symptoms of ASD by gender (93) and level of intellectual disability (94). Future studies in larger cohorts will allow disentangling such key factors in the development of interpersonal synchrony.
Conclusion
The HDC is an effective means to evaluate interpersonal synchrony at both low and high levels of social cognition during live interactions. It can also probe the developmental aspects of their evolving relationship. On the other hand, the psychometric evaluation of HDC provides reliable, reproducible, objective, and standardized scores, derived from a natural movement. As a new psychometric test, HDC provides motor and social markers that help to improve the early detection of neurobehavioral abnormalities during human interaction. The HDC paradigm also provides a dynamical basis for the development of further therapeutic approaches, for instance in the area of serious games (e.g., in mixed reality: https://vimeo.com/277085489).
Data Availability Statement
The datasets generated for this study will not be made publicly available because of the clauses in the ethical consents.
Ethics Statement
The studies involving human participants were reviewed and approved by Hospital Robert Debré. Written informed consent to participate in this study was provided by the participants' legal guardian/next of kin.
Author Contributions
FB, AL, and AP collected all the data. FB, AL, FA, and AM worked on the inclusion of patients and their clinical exploration. FB, AL, YB, and DE participated in both analysis and writing. RD and TB participated in the design and relecture. GD participated in the design, analysis, and writing. All authors contributed to the article and approved the submitted version.
Funding
This work was supported by the funding of the Institut Pasteur, the INSERM, the Fondation FondaMental, the APHP, the DHU Protect, the Fondation Bettencourt-Schueller, the Fondation Cognacq Jay, the Fondation Conny-Maeva, the Fondation de France, and the Labex BioPsy. FB was funded by the Fondation FondaMental and the Congrès Français de Psychiatrie. GD is funded by the Institute for Data Valorization (IVADO), Montreal, and the Fonds de recherche du Québec (FRQ). JK is funded by NIMH grant MH080838.
Conflict of Interest
The authors declare that the research was conducted in the absence of any commercial or financial relationships that could be construed as a potential conflict of interest.
Acknowledgments
The authors would like to thank the participants and their families who participated in this study. They also thank Benjamin Landman, Anna Banki, and Ramon Aparicio-Garcia for their early help in the project.
Supplementary Material
The Supplementary Material for this article can be found online at: https://www.frontiersin.org/articles/10.3389/fpsyt.2020.510366/full#supplementary-material
Supplementary Data Sheet. Additional methods regarding the calculation of the HDC scores, the stratification using clustering analysis, and the supervised learning with logistic regression.
Supplementary Figure 1. Stratification based on HDC scores. (A) Optimal number of clusters between k = 2 to k = 20 obtained by NbClust with 26 indices. The majority vote (9 of 26) indicates k = 2 as the optimal cluster configuration. (B) Corresponding Hierarchical Clustering using Euclidian distance and the Ward method.
Supplementary Table 1. HDC percentile ranks by age group.
Supplementary Table 2. Comparison of HDC and clinical scores between the two clusters identified.
References
1. American Psychiatric Association. Diagnostic and Statistical Manual of Mental Disorders (DSM-5). American Psychiatric Association (APA). (2013). doi: 10.1176/appi.books.9780890425596
2. Baxter AJ, Brugha TS, Erskine HE, Scheurer RW, Vos T, Scott JG. The epidemiology and global burden of autism spectrum disorders. Psychol Med. (2015) 45:601. doi: 10.1017/S003329171400172X
4. Georgiades S, Szatmari P, Boyle M, Hanna S, Duku E, Zwaigenbaum L, et al. Investigating phenotypic heterogeneity in children with autism spectrum disorder: a factor mixture modeling approach. J Child Psychol Psychiatry. (2013) 54:206–15. doi: 10.1111/j.1469-7610.2012.02588.x
5. Huguet G, Ey E, Bourgeron T. The genetic landscapes of autism spectrum disorders. Ann Rev Genom Hum Genet. (2013) 14:191–213. doi: 10.1146/annurev-genom-091212-153431
6. Soke GN, Maenner MJ, Christensen D, Kurzius-Spencer M, Schieve LA. Prevalence of co-occurring medical and behavioral conditions/symptoms among 4-and 8-year-old children with autism spectrum disorder in selected areas of the United States in 2010. J Aut Dev Disord. (2018) 48:2663–76. doi: 10.1007/s10803-018-3521-1
7. Vivanti G, Trembath D, Dissanayake C. Mechanisms of imitation impairment in autism spectrum disorder. J abnormal child psychol. (2014) 42:1395–405. doi: 10.1007/s10802-014-9874-9
8. Mogan R, Fischer R, Bulbulia JA. To be in synchrony or not? A meta-analysis of synchrony's effects on behavior, perception, cognition and affect. J Exp Soc Psychol. (2017) 72:13–20. doi: 10.1016/j.jesp.2017.03.009
9. Nebel MB, Eloyan A, Nettles CA, Sweeney KL, Ament K, Ward RE, et al. Intrinsic visual-motor synchrony correlates with social deficits in autism. Biol Psychiatry. (2016) 79:633–41. doi: 10.1016/j.biopsych.2015.08.029
10. Xavier J, Magnat J, Sherman A, Gauthier S, Cohen D, Chaby L. A developmental and clinical perspective of rhythmic interpersonal coordination: From mimicry toward the interconnection of minds. J Physiol. (2016) 110:420–6. doi: 10.1016/j.jphysparis.2017.06.001
11. Atzil S, Hendler T, Feldman R. Specifying the neurobiological basis of human attachment: brain, hormones, and behavior in synchronous and intrusive mothers. Neuropsychopharmacology. (2011) 36:2603–15. doi: 10.1038/npp.2011.172
12. Dumas G, Nadel J, Soussignan R, Martinerie J, Garnero L. Inter-Brain synchronization during social interaction. Édité par Jan Lauwereyns. PLoS ONE. (2010) 5:e12166. doi: 10.1371/journal.pone.0012166
13. Delaherche E, Chetouani M, Mahdhaoui A, Saint-Georges C, Viaux S, Cohen D. Interpersonal synchrony: a survey of evaluation methods across disciplines. IEEE Trans Affect Comp. (2012) 3:349–65. doi: 10.1109/T-AFFC.2012.12
14. Dumas G, de Guzman GC, Tognoli E, Kelso JAS. The human dynamic clamp as a paradigm for social interaction. Proc Natl Acad Sci USA. (2014) 111:E3726–34. doi: 10.1073/pnas.1407486111
15. Kostrubiec V, Dumas G, Zanone PG, Kelso JAS. The virtual teacher (VT) paradigm: learning new patterns of interpersonal coordination using the human dynamic clamp. PLoS ONE. (2015) 10:e0142029. doi: 10.1371/journal.pone.0142029
16. Kelso JAS, de Guzman GC, Reveley C, Tognoli E. Virtual partner interaction (VPI): exploring novel behaviors via coordination dynamics. PLoS ONE. (2009) 4:e5749. doi: 10.1371/journal.pone.0005749
17. Kelso JAS. Coordination dynamics. In: Meyers RA, editors. Encyclopedia of Complexity and System Science. Heidelberg: Springer (2009/2013). p. 1537–64. doi: 10.1007/978-0-387-30440-3_101
18. Dumas GQ, Moreau E, Kelso JAS. The human dynamic clamp reveals the fronto-parietal network linking real-time social coordination and cognition. Cerebral Cortex, (2019) 30:3271–85. doi: 10.1101/651232
19. Zhang M, Dumas G, Kelso JAS, Tognoli E. Enhanced emotional responses during social coordination with a virtual partner. Int J Psychophysiol. (2016) 104:33–43. doi: 10.1016/j.ijpsycho.2016.04.001
20. Xavier J, Gauthier S, Cohen D, Zahoui M, Chetouani M, Villa F, et al. Interpersonal synchronization, motor coordination, and control are impaired during a dynamic imitation task in children with autism spectrum disorder. Front Psychol. (2018) 9:1467. doi: 10.3389/fpsyg.2018.01467
21. McNaughton KA, Redcay E. Interpersonal synchrony in autism. Curr Psychiatry Rep. (2020) 22:12. doi: 10.1007/s11920-020-1135-8
22. Romero V, Fitzpatrick P, Roulier S, Duncan A, Richardson MJ, Schmidt RC. Evidence of embodied social competence during conversation in high functioning children with autism spectrum disorder. PLoS ONE. (2018) 13:e0193906. doi: 10.1371/journal.pone.0193906
23. Noel JP, De Niear MA, Lazzara NS, Wallace MT. Uncoupling between multisensory temporal function and nonverbal turn-taking in autism spectrum disorder. IEEE Trans Cogn Dev Syst. (2017) 10:973–82. doi: 10.1109/TCDS.2017.2778141
24. Marsh KL, Isenhower RW, Richardson MJ, Helt M, Verbalis AD, Schmidt RC, et al. Autism and social disconnection in interpersonal rocking. Front Integr Neurosci. (2013) 7:4. doi: 10.3389/fnint.2013.00004
25. Kaur M, Srinivasan SM, Bhat AN. Comparing motor performance, praxis, coordination, and interpersonal synchrony between children with and without Autism Spectrum Disorder (ASD). Res Dev Dis. (2018) 72:79–95. doi: 10.1016/j.ridd.2017.10.025
26. Fitzpatrick P, Romero V, Amaral JL, Duncan A, Barnard H, Richardson MJ, et al. Social motor synchronization: insights for understanding social behavior in autism. J Aut Dev Disord. (2017) 47:2092–107. doi: 10.1007/s10803-017-3124-2
27. Fitzpatrick P, Romero V, Amaral JL, Duncan A, Barnard H, Richardson MJ, et al. Evaluating the importance of social motor synchronization and motor skill for understanding autism. Autism Res. (2017) 10:1687–99. doi: 10.1002/aur.1808
28. Marchena A, de Eigsti AM. Conversational gestures in autism spectrum disorders: asynchrony but not decreased frequency. Aut Res. (2010) 3:311–22. doi: 10.1002/aur.159
29. Fitzpatrick P, Frazier JA, Cochran DM, Mitchell T, Coleman C, Schmidt RC. Impairments of social motor synchrony evident in autism spectrum disorder. Front Psychol. (2016) 7:1323. doi: 10.3389/fpsyg.2016.01323
30. Bhat AN, Srinivasan SM, Woxholdt C, Shield A. Differences in praxis performance and receptive language during fingerspelling between deaf children with and without autism spectrum disorder. Autism. (2018) 22:271–82. doi: 10.1177/1362361316672179
31. Zalla T, Korman J. Prior knowledge, episodic control and theory of mind in autism: toward an integrative account of social cognition. Front Psychol. (2018) 9:752. doi: 10.3389/fpsyg.2018.00752
32. Hilton BC, Kuhlmeier VA. Intention attribution and the development of moral evaluation. Front Psychol. (2019) 9:2663. doi: 10.3389/fpsyg.2018.02663
33. Bhat AN, Landa RJ, Galloway JC. Current perspectives on motor functioning in infants, children, and adults with autism spectrum disorders. Phys Ther. (2011) 91:1116–29. doi: 10.2522/ptj.20100294
34. Torres EB, Nguyen J, Suresh C, Yanovich P, Kolevzon A. Noise from the periphery in autism spectrum disorders of idiopathic origins and of known etiology. In: Paper Presentation at the Annual Meeting of the Society for Neuroscience SFN. (2013).
35. Righi G, Tenenbaum EJ, McCormick C, Blossom M, Amso D, Sheinkopf SJ. Sensitivity to audio-visual synchrony and its relation to language abilities in children with and without ASD. Aut Res. (2018) 11:645–53. doi: 10.1002/aur.1918
36. Greenfield K, Ropar D, Smith AD, Carey M, Newport R. Visuo-tactile integration in autism: atypical temporal binding may underlie greater reliance on proprioceptive information. Mol Aut. (2015) 6:51. doi: 10.1186/s13229-015-0045-9
37. Lim YH, Partridge K, Girdler S, Morris SL. Standing postural control in individuals with autism spectrum disorder: systematic review and meta-analysis. J Aut Dev Disord. (2017) 47:2238–53. doi: 10.1007/s10803-017-3144-y
38. Fournier KA, Hass CJ, Naik SK, Lodha N, Cauraugh JH. Motor coordination in autism spectrum disorders: a synthesis and meta-analysis. J Aut Dev Disord. (2010) 40:1227–40. doi: 10.1007/s10803-010-0981-3
39. Landa RJ, Holman KC, O'Neill AH, Stuart EA. Intervention targeting development of socially synchronous engagement in toddlers with autism spectrum disorder: a randomized controlled trial. J Child Psychol Psychiatry. (2011) 52:13–21. doi: 10.1111/j.1469-7610.2010.02288.x
40. Zhou B, Xu Q, Li H, Zhang Y, Wang Y, Rogers SJ, et al. Effects of parent-implemented Early Start Denver Model intervention on Chinese Toddlers with autism spectrum disorder: A non-randomized controlled trial. Aut Res. (2018) 11:654–66. doi: 10.1002/aur.1917
41. Franz L, Dawson G. Implementing early intervention for autism spectrum disorder: a global perspective. Pediatr Med. (2019) 2:44. doi: 10.21037/pm.2019.07.09
42. Eapen V, Crnčec R, Walter A. Clinical outcomes of an early intervention program for preschool children with Autism Spectrum Disorder in a community group setting. BMC Pediatr. (2013) 13:3. doi: 10.1186/1471-2431-13-3
43. Landa RJ, Gross AL, Stuart EA, Faherty A. Developmental trajectories in children with and without autism spectrum disorders: the first 3 years. Child Dev. (2013) 84:429–2. doi: 10.1111/j.1467-8624.2012.01870.x
44. Ozonoff S, Gangi D, Hanzel EP, Hill A, Hill MM, Miller M, et al. Onset patterns in autism: Variation across informants, methods, and timing. Aut Res. (2018) 11:788–97. doi: 10.1002/aur.1943
45. Iverson JM, Shic F, Wall CA, Chawarska K, Curtin S, Estes A, et al. Early motor abilities in infants at heightened versus low risk for ASD: A Baby Siblings Research Consortium (BSRC) study. J Abnorm Psychol. (2019) 128:69. doi: 10.1037/abn0000390
46. Stahmer AC, Akshoomoff N, Cunningham AB. Inclusion for toddlers with autism spectrum disorders: the first ten years of a community program. Autism. (2011) 15:625–41. doi: 10.1177/1362361310392253
47. Kostrubiec V, Zanone PG, Fuchs A, Kelso JAS. Beyond the blank slate: routes to learning new coordination patterns depend on the intrinsic dynamics of the learner:experimental evidence and theoretical model. Front Hum Neurosci. (2012) 6:222 doi: 10.3389/fnhum.2012.00222
48. Marquand AF, Rezek I, Buitelaar J, Beckmann CF. Understanding heterogeneity in clinical cohorts using normative models: beyond case-control studies. Biol Psychiatry. (2016) 80:552–61. doi: 10.1016/j.biopsych.2015.12.023
49. Lord C, Rutter M, DiLavore PC, Risi S, Gotham K, Bishop SL. Autism Diagnostic Observation Schedule. 2nd (ADOS-2). Torrance, CA: Western Psychological Services (2012).
50. Lord C, Rutter M, Le Couteur. A. Autism diagnostic interview-revised: a revised version of a diagnostic interview for caregivers of individuals with possible pervasive developmental disorders. J Aut Dev Disord. (1994) 24:659–85. doi: 10.1007/BF02172145
51. Constantino JN. Social responsiveness scale. In: Volkmar FR, editor. Encyclopedia of Autism Spectrum Disorders. New York, NY: Springer New York (2013). p. 2919–29. doi: 10.1007/978-1-4419-1698-3_296
52. Wechsler D, Pearson Education Inc, Psychological Corporation. WISC-V: Wechsler Intelligence Scale for Children. San Antonio, TX: NCS Pearson, Inc. (2014).
53. Tiffin J, Asher EJ. The Purdue Pegboard: norms and studies of reliability and validity. J Appl Psychol. (1948) 32:234. doi: 10.1037/h0061266
54. Gardner RA, Broman M. The Purdue Pegboard: Normative data on 1334 school children. J Clin Child Adolesc Psychol. (1979) 8:156–62. doi: 10.1080/15374417909532912
55. Yeudall LT, Fromm D, Reddon JR, Stefanyk WO. Normative data stratified by age and sex for 12 neuropsychological tests. J Clin Psychol. (1986) 42:918–46. doi: 10.1002/1097-4679(198611)42:6<918::AID-JCLP2270420617>3.0.CO;2-Y
56. Kemp SL, Korkman M. Essentials of NEPSY-II Assessment (Vol. 69). San Diego, CA: John Wiley and Sons (2010).
57. Haken H, Kelso JAS, Bunz H. A theoretical model of phase transitions in human hand movements. Biol Cybern. (1985) 51:347–56. doi: 10.1007/BF00336922
58. Millman KJ, Aivazis M. Python for scientists and engineers. Comput Sci Engin. (2011) 13:9–12. doi: 10.1109/MCSE.2011.36
60. Walt SVD, Colbert SC, Varoquaux G. The NumPy array: a structure for efficient numerical computation. Comp Sci Engin. (2011) 13:22–30. doi: 10.1109/MCSE.2011.37
61. Virtanen P, Gommers R, Oliphant TE, Haberland M, Reddy T, Cournapeau D, et al. SciPy 1.0: fundamental algorithms for scientific computing in Python. Nat Methods. (2020) 17:261–72. doi: 10.1038/s41592-019-0686-2
62. Zabihi M, Oldehinkel M, Wolfers T, Frouin V, Goyard D, Loth E, et al. Dissecting the heterogeneous cortical anatomy of autism spectrum disorder using normative models. Biol Psychiatry. (2019) 4:567–78. doi: 10.1016/j.bpsc.2018.11.013
63. Tachibana Y, Miyazaki C, Ota E, Mori R, Hwang Y, Kobayashi E, et al. A systematic review and meta-analysis of comprehensive interventions for pre-school children with autism spectrum disorder (ASD). PLoS ONE. (2017) 12:e0186502. doi: 10.1371/journal.pone.0186502
64. Nadel J. Perception-action coupling and imitation in autism spectrum disorder. Dev Med Child Neurol. (2015) 57:55–8. doi: 10.1111/dmcn.12689
65. Mu Y, Guo C, Han S. Oxytocin enhances inter-brain synchrony during social coordination in male adults. Soc Cogn Affect Neurosci. (2016) 11:1882–93. doi: 10.1093/scan/nsw106
66. Lang M, Bahna V, Shaver JH, Reddish P, Xygalatas D. Sync to link: Endorphin-mediated synchrony effects on cooperation. Biol Psychol. (2017) 127:191–7. doi: 10.1016/j.biopsycho.2017.06.001
67. Bhat AN, Michael D, Hoffman SL, McKenzie TL, Culotta EJ, Daisuke T, et al. Cortical activation during action observation, action execution, and interpersonal synchrony in adults: a functional near-infrared spectroscopy (FNIRS) study. Front Hum Neurosci. (2017) 11:431. doi: 10.3389/fnhum.2017.00431
68. Valencia A, Froese T. What binds us? Inter-brain neural synchronization and its implications for theories of human consciousness. Neurosci. Conscious. (2020) 2020, niaa010. doi: 10.1093/niaa010
69. Valdesolo P, Ouyang J, DeSteno D. The rhythm of joint action: Synchrony promotes cooperative ability. J Exp Soc Psychol. (2010) 46:693–5. doi: 10.1016/j.jesp.2010.03.004
70. Valdesolo P, DeSteno D. Synchrony and the social tuning of compassion. Emotion. (2011) 11:262. doi: 10.1037/a0021302
71. Malika C, Ghazzali N, Boiteau V, Niknafs A. NbClust: an R package for determining the relevant number of clusters in a data Set. J Stat Softw. (2014) 61:1–36. doi: 10.18637/jss.v061.i06
72. Chukoskie L, Townsend J, Westerfield M. Motor skill in autism spectrum disorders: a subcortical view. In: International Review of Neurobiology. San Diego, CA: Academic Press. (2013). p. 207–49. doi: 10.1016/B978-0-12-418700-9.00007-1
73. Demetriou EA, Lampit A, Quintana DS, Naismith SL, Song YJC, Pye JE, et al. Autism spectrum disorders: a meta-analysis of executive function. Mol Psychiatry. (2018) 23:1198–204. doi: 10.1038/mp.2017.75
74. Green D, Charman T, Pickles A, Chandler S, Loucas TOM, Simonoff E, et al. Impairment in movement skills of children with autistic spectrum disorders. Dev Med Child Neurol. (2009) 51:311–6. doi: 10.1111/j.1469-8749.2008.03242.x
75. Licari MK, Alvares GA, Varcin K, Evans KL, Cleary D, Reid SL, et al. Prevalence of motor difficulties in autism spectrum disorder: analysis of a population-based cohort. Aut Res. (2019) 2019:2230. doi: 10.1002/aur.2230
76. Grace N, Johnson BP, Rinehart NJ, Enticott PG. Are motor control and regulation problems part of the ASD motor profile? A handwriting study. Dev Neuropsychol. (2018) 43:581–94. doi: 10.1080/87565641.2018.1504948
77. Torres EB, Mistry S, Caballero C, Whyatt CP. Stochastic signatures of involuntary head micro-movements can be used to classify females of ABIDE into different subtypes of neurodevelopmental disorders. Front Integr Neurosci. (2017) 11:10. doi: 10.3389/fnint.2017.00010
78. Bolis D, Schilbach L. Beyond one Bayesian brain: Modeling intra-and inter-personal processes during social interaction: Commentary on “Mentalizing homeostasis: the social origins of interoceptive inference” by Fotopoulou and Tsakiris. Neuropsychoanalysis. (2017) 19:35–8. doi: 10.1080/15294145.2017.1295215
79. Thye MD, Bednarz HM, Herringshaw AJ, Sartin EB, Kana RK. The impact of atypical sensory processing on social impairments in autism spectrum disorder. Dev Cogn Neurosci. (2018) 29:151–67. doi: 10.1016/j.dcn.2017.04.010
80. Mundy P, Novotny S, Swain-Lerro L, McIntyre N, Zajic M, Oswald T. Joint-attention and the social phenotype of school-aged children with ASD. J Aut Dev Disord. (2017) 47:1423–35. doi: 10.1007/s10803-017-3061-0
81. Jones EJ, Venema K, Earl RK, Lowy R, Webb SJ. Infant social attention: an endophenotype of ASD-related traits? J Child Psychol Psychiatry. (2017) 58:270–81. doi: 10.1111/jcpp.12650
82. Wang Q, Han Z, Hu X, Feng S, Wang H, Liu T, et al. Autism symptoms modulate interpersonal neural synchronization in children with autism spectrum disorder in cooperative interactions. Brain Topogr. (2020) 33:112–22. doi: 10.1007/s10548-019-00731-x
83. Bliksted V, Ubukata S, Koelkebeck K. Discriminating autism spectrum disorders from schizophrenia by investigation of mental state attribution on an on-line mentalizing task: A review and meta-analysis. Schizophr Res. (2016) 171:16–26. doi: 10.1016/j.schres.2016.01.037
84. Grainger C, Williams DM, Lind SE. Metacognitive monitoring and control processes in children with autism spectrum disorder: Diminished judgement of confidence accuracy. Conscious Cogn. (2016) 42:65–74. doi: 10.1016/j.concog.2016.03.003
85. Forgeot d'Arc B, Devaine M, Daunizeau J. Social behavioural adaptation in Autism. PLoS Comp Biol. (2020) 16:e1007700. doi: 10.1371/journal.pcbi.1007700
86. Gotham K, Bishop SL, Hus V, Huerta M, Lund S, Buja A, et al. Exploring the relationship between anxiety and insistence on sameness in autism spectrum disorders. Aut Res. (2013) 6:33–41. doi: 10.1002/aur.1263
87. Kanner L. Early infantile autism. J Pediatr. (1944) 25:211–7. doi: 10.1016/S0022-3476(44)80156-1
88. Ozonoff S, Iosif AM. Changing conceptualizations of regression: what prospective studies reveal about the onset of autism spectrum disorder. Neurosci Biobehav Rev. (2019) 100:296–304. doi: 10.1016/j.neubiorev.2019.03.012
89. Jones EJ, Gliga T, Bedford R, Charman T, Johnson MH. Developmental pathways to autism: a review of prospective studies of infants at risk. Neurosci Biobehav Rev. (2014) 39:1–33. doi: 10.1016/j.neubiorev.2013.12.001
90. Tani P, Lindberg N, Appelberg B, Nieminen-von Wendt T, Wendt von L, Porkka-Heiskanen T. Clinical neurological abnormalities in young adults with Asperger syndrome. Psychiatry Clin Neurosci. (2006) 60:253–5. doi: 10.1111/j.1440-1819.2006.01494.x
91. Lefebvre A, Delorme R, Delano C, Amsellem F, Beggiato A, Germanaud D, et al. Alpha waves as a neuromarker of autism spectrum disorder: the challenge of reproducibility and heterogeneity. Front Neurosci. (2018) 12:662. doi: 10.3389/fnins.2018.00662
92. Poldrack RA, Huckins G, Varoquaux G. Establishment of best practices for evidence for prediction: a review. JAMA Psychiatry. (2020) 77:534–40. doi: 10.1001/jamapsychiatry.2019.3671
93. Beggiato A, Peyre H, Maruani A, Scheid I, Rastam M, Amsellem F, et al. Gender differences in autism spectrum disorders : Divergence among specific core symptoms. Aut Res. (2017) 10:680–9. doi: 10.1002/aur.1715
Keywords: computational psychiatry, human-machine interface (HMI), psychometric, interpersonal synchrony, autism spectrum disorder, coordination dynamics
Citation: Baillin F, Lefebvre A, Pedoux A, Beauxis Y, Engemann DA, Maruani A, Amsellem F, Kelso JAS, Bourgeron T, Delorme R and Dumas G (2020) Interactive Psychometrics for Autism With the Human Dynamic Clamp: Interpersonal Synchrony From Sensorimotor to Sociocognitive Domains. Front. Psychiatry 11:510366. doi: 10.3389/fpsyt.2020.510366
Received: 06 November 2019; Accepted: 12 October 2020;
Published: 26 November 2020.
Edited by:
Danilo Bzdok, Helmholtz Association of German Research Centers (HZ), GermanyReviewed by:
Jeroen M. van Baar, Brown University, United StatesSandy Overgaauw, Leiden University, Netherlands
Copyright © 2020 Baillin, Lefebvre, Pedoux, Beauxis, Engemann, Maruani, Amsellem, Kelso, Bourgeron, Delorme and Dumas. This is an open-access article distributed under the terms of the Creative Commons Attribution License (CC BY). The use, distribution or reproduction in other forums is permitted, provided the original author(s) and the copyright owner(s) are credited and that the original publication in this journal is cited, in accordance with accepted academic practice. No use, distribution or reproduction is permitted which does not comply with these terms.
*Correspondence: Guillaume Dumas, guillaume.dumas@centraliens.net
†These authors have contributed equally to this work