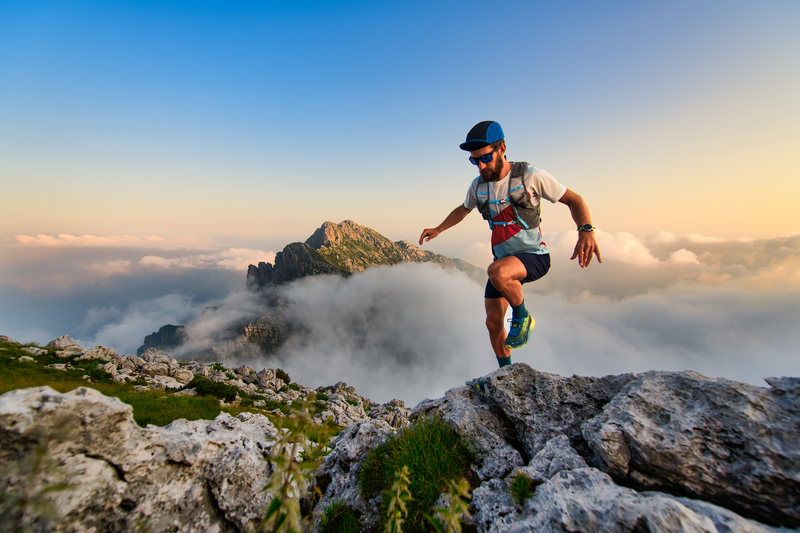
94% of researchers rate our articles as excellent or good
Learn more about the work of our research integrity team to safeguard the quality of each article we publish.
Find out more
CLINICAL TRIAL article
Front. Psychiatry , 02 September 2020
Sec. Sleep Disorders
Volume 11 - 2020 | https://doi.org/10.3389/fpsyt.2020.00855
Background: Autism spectrum disorder (ASD) is a type of neurodevelopmental disease that is frequently accompanied by sleep disorder. Herein, we investigated changes in the gut microbiota and its metabolites correlated with core symptoms and sleep problems in children with ASD.
Methods: One hundred and twenty children diagnosed with ASD based on Diagnostic and Statistical Manual of Mental Disorders (DSM-5) criteria were enrolled in our study. The Autism Behavior Checklist (ABC), Social Responsiveness Scale (SRS), and Childhood Autism Rating Scale (CARS) were used to assess autism symptoms, and the Children Sleep Habits Questionnaire (CSHQ) was employed to evaluate sleep problems in children with ASD. The 120 children were divided into a sleep disorder group (n = 60) and a control group without sleep disorder (n = 60) according to the CSHQ answers. Illumina MiSeq analysis of 16S rRNA genes was used to compare differences in gut microbiota, and metabolomics analysis was employed to asses associated metabolites.
Results: SRS and CARS scores for the sleep disorder group were significantly higher than for the control group (p < 0.05). The abundances of butyrate-producing bacteria Faecalibacterium and Agathobacter were reduced significantly in the sleep disorder group (p < 0.05), and this was negatively correlated with CSHQ score (p = 0.007 and p = 0.014, respectively). The abundance of Agathobacter was also negatively associated with the ABC language score (p = 0.044). Furthermore, levels of 3-hydroxybutyric acid and melatonin were significantly lower (p < 0.05) while serotonin levels were higher (p < 0.05) in the sleep disorder group. The 3-hydroxybutyric acid level was positively associated with Faecalibacterium abundance (p = 0.000), and melatonin was positively associated with the abundance of Faecalibacterium (p = 0.036) and Agathobacter (p = 0.041). We also observed negative correlations between 3-hydroxybutyric acid and CSHQ (p = 0.000) and CARS (p = 0.009), between melatonin and CSHQ (p = 0.002) and ABC sensory score (p = 0.021), and a positive correlation between serotonin and CSHQ (p = 0.002) and ABC sensory score (p = 0.025).
Conclusions: ASD children with sleep disorder exhibited declines in the abundance of Faecalibacterium and Agathobacter, decreased levels of 3-hydroxybutyric acid and melatonin, and an increase in serotonin. These changes may aggravate sleep problems and core symptoms in children with ASD.
Autism spectrum disorder (ASD) is a type of neurodevelopmental disease characterized by impaired social interactions and communication, accompanied by stereotypic behavior, and restricted interests or activities (1). In addition to these core symptoms, children with ASD often suffer from other developmental disorders, gastrointestinal symptoms, and sleep problems. Among these problems, several studies have shown that 50%−80% of children with ASD have sleep disorders (2–5). Common sleep problems include waking up at night, parasomnias, and sleep onset delay. Furthermore, the severity of core symptoms of ASD are higher in ASD children with sleep disorder (4).
Although ASD could be classified as a neurological disease in terms of pathology, there is increasing evidence to demonstrate that the gastrointestinal system and its resident microbiota plays an important role in certain symptoms of ASD. Changes in gut microbiota are common in children with ASD. Studies have reported a significant elevation in Clostridium spp., Desulfovibrio spp. and Lactobacillus in the intestines of children with ASD, and the ratio of Firmicutes to Bacteroidetes is lower than in normal controls (6–11). Most studies have shown that changes in the gut microbiota are closely related to the severity of autism (11, 12), anxiety, and depression (13). Social impairment was observed in germ-free mice (14), and probiotic therapy had a beneficial effect on autism behavior in autism animal models (15, 16). However, little research has been conducted on whether the gut microbiota can affect sleep problems in ASD.
Evidence suggests that brain function and behavior are affected by metabolites of the microbiota, and the key molecules are short-chain fatty acids (SCFAs) (17). As is common in gut dysbiosis, levels of SCFAs such as butyrate are decreased in ASD (18). Butyrate inhibits histone deacetylase (HDAC) enzymes which participate in the serotonergic system (19), and can induce melatonin (20). Elevated whole-blood serotonin levels were observed in patients with ASD following meta-analysis (21), and levels of melatonin in urine, plasma, and the pineal gland are also lower in patients with ASD (22). However, whether SCFAs such as butyrate produced by the gut microbiota are associated with sleep problems in children with ASD remains unknown.
Therefore, the present study aimed to investigate changes in the gut microbiota and associated metabolites in sleep problems, and explore correlations with core symptoms in children with ASD.
We collected 293 ASD children who completed the questionnaires, blood biochemistry test and collection of fecal samples in Hainan Province, China during 2018‑2019. And we divided these children into those with sleep problems (n=183) and no sleep problems (n=110) according to the standard of Children Sleep Habits Questionnaire (CSHQ), and 1:1 randomly selected 60 children with age and gender matching from each group.
Finally, a total of 120 children diagnosed with ASD, comprising 100 boys and 20 girls, were recruited in our study (60 suffered sleep disorder and 60 did not). Inclusion criteria were as follows: 1) all of these ASD children were diagnosed by a developmental pediatrician and psychologist in Hainan Women and Children’s Medical Center following a series of structured interviews according to the Diagnostic and Statistical Manual of Mental Disorders (DSM-5) criteria; 2) symptoms of patients were evaluated by the Autism Behaviour Checklist (ABC; score <53 is normal), the Childhood Autism Rating Scale (CARS; score <30 is normal) (23), and the Social Responsiveness Scale (SRS; score <65 is normal) (24); 3) sleeping conditions were evaluated by the Children Sleep Habits Questionnaire (CSHQ; score <41 is normal) (25); 4) parents of all ASD children signed written informed consent, and participation in this research was voluntary. Those with a history of other developmental disorders or psychiatric diseases, including genetic disorders with comorbid autism, Rett Syndrome, cerebral palsy, chronic seizures, other congenital diseases, and recent infection, were excluded.
The ethics review committee of the Institutional Review Board of Children’s Hospital, Chongqing Medical University, approved this study. This cross-sectional case-control study was based on a clinical trial which was registered in the Chinese Clinical Trial Registry (ChiCTR; registration number: ChiCTR-ROC-14005442).
Parents of ASD children completed the following questionnaires according to the standard instructions; a background questionnaire including questions concerning demographic data (e.g. name, age, gender) and medical history; a CSHQ comprising 41 questions, of which 33 were scoring items, divided into eight dimensions (bedtime resistance, sleep onset delay, sleep duration, sleep anxiety, night waking, parasomnia, sleep disordered breathing, daytime sleepiness). The CSHQ is an effective and reliable indicator that can effectively distinguish the sleep problems of clinical and community samples (e.g., Cronbach’s α = 0.68 for community samples and 0.78 for clinical samples) (26). Parents were asked to recall sleep behaviors occurring in a recent week. Items are rated on a 3-point scale; “usually” related to a sleep behavior occurring 5 to 7 times per week (3 points); “sometimes” related to 2 to 4 times per week (2 points); “rarely” related to 0 to 1 time per week (1 point). The higher the total score, the more serious the sleep problem. A total CSHQ score ≥41 was used as the criterion for evaluating sleep disorder (25).
Fresh stool samples were collected from participants who did not receive antibiotic treatment and supplemental probiotics or prebiotics within the past month. Samples were placed in sterile stool tubes, frozen on dry ice within 24 h, and transported in a dry ice box to the Children’s Hospital of Chongqing Medical University within 5 days, where they were immediately stored at -80°C for future use.
According to the manufacturer’s protocol, microbial DNA was extracted from stool samples using an OMEGA DNA Kit (Omega Bio-Tek, USA). The final concentration of purified DNA was determined using a NanoDrop 2000 UV-vis spectrophotometer (Thermo Scientific, Wilmington, USA), and the quality of DNA was checked by 1% agarose gel electrophoresis. Bacterial 16S rRNA gene V3−V4 hypervariable regions were amplified with primers 338F (5’-ACTCCTACGGGAGGCAGCAG-3’) and 806R (5’-GGACTACHVGGGTWTCTAAT-3’) using a GeneAmp 9700 PCR thermocycler system (ABI, USA). Thermal cycling included an initial denaturation at 95°C for 3 min, followed by 27 cycles 95°C for 30 s, annealing at 55°C for 30 s, elongation at 72°C for 45 s, and a final extension at 72°C for 10 min. Reactions were performed in triplicate 20 μl mixtures containing 4 μl of 5 × FastPfu Buffer, 2 μl of 2.5 mM dNTPs, 0.8 μl of each primer (5 μM), 0.4 μl of FastPfu Polymerase, and 10 ng of template DNA. The resulting PCR products were extracted from a 2% agarose gel, further purified with an AxyPrep DNA Gel Extraction Kit (Axygen Biosciences, Union City, CA, USA), and quantified with QuantiFluor-ST (Promega, USA) in accordance with the manufacturer’s protocol.
According to the standard protocols of Majorbio Bio-Pharm Technology Co. Ltd. (Shanghai, China), purified amplicons were pooled in equimolar concentrations and paired-end sequenced (2 × 300) on an Illumina MiSeq platform (Illumina, San Diego, USA). Raw reads were submitted to the NCBI Sequence Read Archive (SRA) database (Accession Number: SRA:254243).
Raw fastq files were demultiplexed, quality-filtered by Trimmomatic, and merged by FLASH according to the following criteria: i) over a 50 bp sliding window, reads for any site with an average quality score <20 were truncated; ii) primers were exactly matched allowing two nucleotide mismatches, and reads containing ambiguous bases were deleted; iii) sequences with overlaps longer than 10 bp were merged in accordance with their overlapping sequence.
Operational taxonomic units (OTUs) were obtained with a 97% similarity cutoff using UPARSE (Version 7.1 http://drive5.com/uparse/) and chimeric sequences were identified and removed with UCHIME. The taxonomy of each 16S rRNA gene sequence was analyzed by the RDP Classifier algorithm (http://rdp.cme.msu.edu/) against the Silva (SSU132) 16S rRNA database using a confidence threshold of 70%.
OTUs with 97% similarity levels were analyzed for community richness (Chao, ACE, Sobs), community diversity (Shannon, Simpson), and rarefaction curve. Comparisons of Chao, ACE, Sobs, Shannon, and Simpson indices between sleep disorder and control groups were analyzed by student’s t-test. A partial least squares discriminant analysis (PLS-DA) was performed to effectively distinguish the groups.
Stool samples (100 mg) were separately ground with liquid nitrogen and the homogenate was resuspended in prechilled methanol and 0.1% formic acid by vortexing thoroughly. Samples were incubated on ice for 5 min then centrifuged at 15,000 rpm at 4°C for 5 min. Some supernatants were diluted with liquid chromatography-mass spectrometry (LC-MS)-grade water to a final concentration of 60% methanol. Hereafter, samples were transferred into a fresh Eppendorf tube through a 0.22 μm filter then centrifuged at 15,000 g at 4°C for 10 min. Finally, the filtrate was injected into the LC-MS/MS system for analysis.
LC-MS/MS analyses were performed using a Vanquish UHPLC system (Thermo Fisher) and an Orbitrap Q Exactive HF-X mass spectrometer (Thermo Fisher). Samples were injected onto an Hyperil Gold column (100 mm × 2.1 mm, 1.9 μm) at a flow rate of 0.2 ml/min and separated using a 16 min linear gradient. The eluents for positive polarity mode were eluent A (0.1% formic acid in water) and eluent B (methanol). The eluents for negative polarity mode were eluent A (5 mM ammonium acetate, pH 9.0) and eluent B (methanol). The solvent gradient was set as follows: 2% B 1.5 min, 2%−100% B 12.0 min, 100% B 14.0 min, 100%−2% B 14.1 min, 2% B 16 min. The Q Exactive HF-X mass spectrometer was operated in positive/negative polarity mode with a spray voltage of 3.2 kV, a sheath gas flow rate of 35 arb, an aux gas flow rate of 10 arb, and capillary temperature of 320°C.
Compound Discoverer 3.0 (CD 3.0, Thermo Fisher) was used to process raw data files generated by UHPLC-MS/MS to perform peak alignment, peak selection, and quantification for each metabolite. The main parameters were set as follows: retention time tolerance 0.2 min, actual mass tolerance 5 ppm, signal intensity tolerance 30%, signal/noise ratio 3, minimum intensity 100,000. Peak intensities were normalized against the total spectral intensity, and normalized data were used to predict the molecular formula based on additive ions, molecular ion peaks, and fragment ions. Peaks were matched with mzCloud (https://www.mzcloud.org/) and ChemSpider (http://www.chemspider.com/) databases to obtain accurate qualitative and relative quantitative results.
Data were analyzed using SPSS statistical software (Version 17.0, SPSS Inc., USA) and GraphPad Prism (Version 5.0, GraphPad Software, San Diego California USA). Means, standard deviations (SDs), and percentages were used to describe all data, in addition to microbial data and metabolite levels. To compare levels between the two groups, two-tailed Student’s t-tests and the chi-square tests were used. To compare the abundances of microbiota and levels of metabolites between groups, two-tailed Student’s t-tests and the Mann-Whitney tests were used. To analyze the relationship between microbiota, metabolites, and scores for each scale, Spearman correlation analysis was used. Significance was presumed at p < 0.05.
A total of 120 children with ASD were recruited, and divided into a sleep disorder group (n = 60, 3.993 ± 0.1682 years old, 48 boys and 12 girls) and a no sleep disorder group (n = 60, 3.925 ± 0.1394 years old, 52 boys and 8 girls) according to the CSHQ score standard. As shown in Table 1, there was no significant difference in age and sex composition between the two groups (p > 0.05). The CSHQ score for the sleep disorder group was significantly higher than for the no sleep disorder group (45.50 ± 0.4002 vs. 36.52 ± 0.3457; p < 0.0001). Previous studies have reported that the severity of the core symptoms of ASD in ASD children suffering sleep problems is increased (4). Therefore, we compared ABC, SRS, and CARS scores among participants. As shown in Table 1, SRS and CARS scores for the sleep disorder group were significantly higher than for the no sleep disorder group (p < 0.05). These results suggest that core symptoms of autism are more severe in ASD children with sleep disorder.
Table 1 Comparison of general characteristics, CHSQ, and ASD Core Symptom Scale scores of ASD children with and without sleep disorder.
This study yielded 6,003,732 (50,031 reads per sample) high-quality sequences with an average length of 416.19 bp from 120 samples after OTU picking and chimera checking. We obtained numerous OTUs from valid sequences, and OTUs with 97% similarity were subjected to further statistical analysis. As the number of reads increased, the rarefaction curves of all samples shown in Figure 1A exhibited smooth growth trends and approached saturation plateaus, indicating that the amount of sequencing data obtained was sufficient.
Figure 1 Rarefaction curves, PLS-DA, and α-diversity analysis of fecal samples from ASD children with and without sleep disorder. (A) Rarefaction curves calculated for OTUs with 97% identity for gut microbiota in sleep disorder and no sleep disorder groups. Blue curves represent the no sleep disorder group, and red curves represent the sleep disorder group. (B) PLS-DA plot based on unweighted UniFrac distance metrics. Blue triangles represent the no sleep disorder group, and red circles represent the sleep disorder group. (C) Comparison of ACE, Chao, Sobs, Shannon, and Simpson indices among the two groups. The blue column represents the no sleep disorder group, the red column represents the sleep disorder group, and α-diversity indices analyzed by Student’s t-test are reported as mean ± SD (*p < 0.05).
An unweighted UniFrac distance matrix was calculated based on the OTUs of each sample to assess structural differences of microbial communities between the two groups. The results of the subsequent partial least squares discriminant analysis (PLS-DA) revealed obvious differences between the two groups (Figure 1B). This suggests that there may be differences in the distribution of the fecal microbiota between ASD children with or without sleep disorder.
The diversity indices ACE, Chao, and Sobs indicate the richness of the microbiota, whereas Shannon and Simpson diversity indices reflect the colony richness and evenness. As shown in Figure 1C, the ACE, Chao and Sobs diversity indices for the sleep disorder group were significantly higher than those for the no sleep disorder group (p < 0.05), while the Shannon index was higher and the Simpson index was lower for the sleep disorder group, but there were no significant differences between the two groups. These results demonstrated that the abundance and richness of the gut microbiota of ASD children with sleep disorder were greater than those of ASD children without sleep disorder.
Figure 2A shows the composition of the predominant microbiota in the two groups, and the relative abundance at the phylum level as >1%. In the sleep disorder group, the predominant phyla were Firmicutes (43.15%), Actinobacteria (25.88%), Bacteroidetes (22.57%), Proteobacteria (6.34%), and Verrucomicrobia (1.62%). Meanwhile, the predominant phyla were Firmicutes (43.32%), Actinobacteria (28.30%), Bacteroidetes (20.69%), Proteobacteria (5.58%), and Verrucomicrobia (1.29%) in the no sleep disorder group. However, there were no significant differences in these predominant phyla between the two groups (p > 0.05). Also, the Firmicutes/Bacteroidetes ratio was not significantly different (p > 0.05; data not shown). The composition of the predominant microbiota at the genus level is shown in Figure 2B, and the relative abundances in the two groups were >1%. As shown in Figure 2C, the abundances of Faecalibacterium and Agathobacter were significantly lower in the sleep disorder group than the no sleep disorder group (p < 0.05).
Figure 2 The relative abundance of bacteria in feces from ASD children with or without sleep disorder. (A) Dominant bacterial phyla with relative abundances greater than 1%. (B) Dominant bacterial genera with relative abundances greater than 1%. (C) Differences in Faecalibacterium and Agathobacter abundance in no sleep disorder and sleep disorder groups at the genus level (*p < 0.05, Mann-Whitney test).
Furthermore, we also observed a significantly negative correlation between CSHQ scores and the abundances of Faecalibacterium (rs = -0.244, p = 0.007) and Agathobacter (rs = -0.225, p = 0.014) according to correlation analysis (Table 2). Additionally, the abundance of Agathobacter was negatively associated with the ABC language score (rs = -0.184, p = 0.044). These results suggest that the abundance of Faecalibacterium and Agathobacter might impact the severity of sleep disorder and autism core symptoms in ASD children. Several studies have reported that the gut microbiota may influence the central nervous system (CNS) by producing short-chain fatty acids (SCFAs) (27), and the main metabolite produced by Faecalibacterium and Agathobacter is butyrate, which belongs to the SCFAs.
Table 2 Correlation analysis between Faecalibacterium and Agathobacter abundance, and CHSQ and ASD core symptom score.
To further explore the effect of the gut microbiota and its metabolites on the autism symptoms and sleep problems of children with ASD, we analyzed the metabolites of feces from the two groups. Analysis of metabolites in 120 fecal samples from ASD children with or without sleep disorder identified 4,531 species in positive ion mode and 2,405 species in negative ion mode. The results of subsequent PLS-DA are shown in Figure 3A, and there are clear differences between the two groups in both positive and negative ion mode. The total number of differential metabolites between the sleep disorder and no sleep disorder groups was 175 in positive ion mode and 99 in negative ion mode, and volcano maps are shown in Figure 3B. There were three main differential metabolites between sleep disorder and no sleep disorder groups (Figure 3C). Levels of 3-hydroxybutyric acid and melatonin were significantly lower in the sleep disorder group, while serotonin levels were significantly higher (p < 0.05). As shown in Table 3, 3-hydroxybutyric acid levels were positively correlated with Faecalibacterium abundance (rs = 0.382, p = 0.000), and the melatonin level was also positively correlated with both Faecalibacterium (rs = 0.197, p = 0.036) and Agathobacter (rs = 0.192, p = 0.041) abundance. Additionally, the 3-hydroxybutyric acid level was positively associated with melatonin level (rs = 0.782, p = 0.000; Table 4). The results suggest that there were changes in fecal metabolites related to key gut microbiota components in ASD Children suffering sleep problems, and the 3-hydroxybutyric acid level may influence the melatonin level, which is associated with sleep disorder (28–30).
Figure 3 PLS-DA and differential metabolites of fecal samples from ASD children with and without sleep disorder. (A) PLS-DA analysis of metabolites using negative and positive ion modes. Blue circles represent the no sleep disorder group, and red circles represent the sleep disorder group. (B) Volcano maps of total differential metabolites in sleep disorder and no sleep disorder groups. Red dots represent elevated metabolites and green dots represent decreased metabolites. (C) The three main differential metabolites, 3-hydroxybutyric acid, serotonin, and melatonin, between sleep disorder and no sleep disorder groups (*p < 0.05, Student’s t-test and Mann-Whitney test).
Table 3 Correlation analysis between Faecalibacterium and Agathobacter abundance and metabolites levels.
Additionally, we analyzed the relationship between these three metabolites and CSHQ and autism core symptoms. We observed a negative correlation between 3-hydroxybutyric acid and CSHQ (rs = -0.441, p = 0.0001) and CARS scores (rs = -0.251, p = 0.009). There was a positive correlation between serotonin levels and CSHQ (rs = 0.276, p = 0.002) and ABC sensory score (rs = 0.205, p = 0.025). We also found that there was a negative association between melatonin level and CSHQ (rs = -0.284, p = 0.002) and sensory score in ABC (rs = -0.216, p = 0.021), as shown in Table 5. These results indicate that levels of 3-hydroxybutyric acid, serotonin, and melatonin may influence sleep and core symptoms in autistic children.
Table 5 Correlation analysis between serotonin, melatonin, 3-hydroxybutyric acid, CHSQ, and ASD core symptom score.
Children’s sleep behavior and sleep quality are a comprehensive manifestation of psychological, developmental, biological, environmental, and cultural influences (31). Sleep disturbance may be harmful to children’s cognitive development and daily functions such as behavior, learning, attention, memory, and mood regulation (32, 33). Studies have reported that disordered sleep may be a contributing factor to problems in autism behavior (4, 34). In our present study, the core symptoms of ASD children also suffering sleep disorder were more severe than those experiencing normal sleep, consistent with previous studies.
Several studies have demonstrated changes in the gut microbiota of children with ASD compared with normally developing children (9, 12, 35, 36), and differences in microbial composition are correlated with alterations in behavior and cognition associated with the microbiota-gut-brain axis (37). Components of the microbiota communicate with the brain through various routes including the enteric nervous system, the immune system, tryptophan metabolism, and the vagus nerve, via several microbial metabolites such as SFCAs, peptidoglycan, and branched-chain amino acids (27). The microbiota-gut-brain axis may also be associated with the pathogenesis of ASD (38). In our current study, the results of PLS-DA showed that the distribution and composition of bacteria in feces from ASD children with and without sleep disorder were clearly distinct. Chao and ACE diversity indices reflect species richness of the microbiota, while Shannon and Simpson diversity indices indicate colony richness and evenness. In the present study, the abundance and richness of the gut microbiota in ASD children suffering sleep disorder were significantly greater than those of ASD children without sleep disorder. However, some previous studies on changes in gut microbial diversity have reported different results. Although the abundance and richness of the microbiota in the sleep disorder group were higher, changes in the billions of microbes within the microbiota in the human gut may vary greatly. In our study, we found that the abundance of two key bacteria, Faecalibacterium and Agathobacter, was significantly reduced in the sleep disorder group, at the genus level. Additionally, the abundance of Faecalibacterium and Agathobacter was negatively correlated with CSHQ scores, while the abundance of Agathobacter was also negatively associated with ABC language score. This indicates that the abundance of Faecalibacterium and Agathobacter may be associated with sleep problems and autism core symptoms.
Faecalibacterium is the most abundant bacterium in the human intestinal microbiota, accounting for more than 5% of the total number of bacteria present (39). Faecalibacterium is one of the most functionally active members of the microbiome (40), and one of the top butyrate-producing bacteria in the gastrointestinal tract (41). Faecalibacterium may affect physiological functions and homeostasis to maintain health by producing butyrate in the gut. Agathobacter species are anaerobic, Gram-positive bacteria that represent a novel species of a new genus in the family Lachnospiraceae. The main fermentation products of this genus are butyrate, acetate, hydrogen, and lactate (42). Butyrate is an SCFA that plays an important role in gut physiology. It has multiple effects on the intestinal cell life cycle, and many beneficial effects on health by preventing pathogen invasion, modulating the immune system, and reducing cancer progression (43). Butyrate is mainly produced by anaerobic microorganisms via the fermentation of indigestible carbohydrates through the acetyl-coenzyme A (AcCoA) pathway (17). Levels of butyrate are decreased in ASD patients (18).
The ketone body compound 3-hydroxybutyric acid can be synthesized from AcCoA by host cells, and it appears to interact with butyrate metabolism. Studies have reported that high levels of butyrate can raise the levels of 3-hydroxybutyric acid in calve blood and cerebrospinal fluid (CSF) (44). This indicates that 3-hydroxybutyric acid may be one of metabolites of butyrate. In the present study, we did not detect changes in butyrate level via analysis of fecal metabolites, but the 3-hydroxybutyric acid level was significantly lower in the sleep disorder group than the no sleep disorder group, and positively correlated with Faecalibacterium abundance. Previous studies also reported that butyrate directly affects the release of serotonin and intestinal hormones in the enteric nervous system, thereby stimulating the vagus nerve and eliciting endocrine signaling, both affecting brain function (17). In our present study, we found that serotonin were significantly elevated in ASD children suffering sleep disorder.
Serotonin is produced by intestinal chromaffin cells in the gut (45), and it plays a key role as a neurotransmitter in the brain by regulating various behavioral, autonomic, and cognitive functions (46). Studies have shown that compared with normally developing control groups, average serotonin levels in blood are significantly higher in ~30% of autistic individuals (47). Additionally, melatonin levels in urine, plasma, and the pineal gland can be lower in ASD individuals (20, 48–51). Melatonin is derived from serotonin and produced mainly in the pineal gland (50). Melatonin is a pleiotropic neuroendocrine molecule, which is essential for synchronizing circadian, sleep/wake cycles, and seasonal rhythms, and it also has antioxidant, neuroprotective, and immunomodulatory effects (28–30). In the present study, melatonin levels in feces of ASD children were significantly lower in the sleep disorder group than in the no sleep disorder group, and the abundance of Faecalibacterium and Agathobacter were positively associated with melatonin level, as were 3-hydroxybutyric acid levels, suggesting that butyrate-producing bacteria and butyrate-related metabolites were associated with melatonin. Furthermore, we found a negative correlation between 3-hydroxybutyric acid level and CSHQ and CARS. By contrast, there was a positive correlation between serotonin level and CSHQ and ABC sensory score. Additionally, the melatonin level was also negatively associated with CSHQ and ABC sensory score. This indicates that a reduction in butyrate and its related metabolites may affect serotonin levels, leading to a decrease in melatonin, which in turn may worsen sleep problems and core autism symptoms.
There are some limitations with the present work. This was a cross-sectional case-control study, and additional cohort studies should be performed to further explore the dynamic changes in sleep problems, symptoms, and gut microbiota in ASD individuals. A normal control group should also be added in future studies to compare differences in sleeping issues and gut microbiota between ASD and normal children. Furthermore, it remains unclear how gut microbiota may affect sleep conditions and autism symptoms, and the relationship between sleep problems and core symptoms also requires further investigation.
In summary, sleep disorder is a common comorbidity in children with ASD that may exacerbate the core symptoms of ASD children. Thus, sleep problems have received widespread attention in recent years. Herein, the gut microbiota in ASD children with sleep problems was imbalanced, and the abundance of butyrate-producing bacteria Faecalibacterium and Agathobacter were reduced, leading to a decrease in butyrate and its metabolites, which further elicits an increase in serotonin and a decrease in melatonin, potentially aggravating sleep problems and core autism symptoms in children with ASD. Therefore, treatment strategies to ameliorate the gut microbiota and SCFAs, especially butyrate-producing bacteria, may have a potential role in relieving sleep disorder and core symptoms in ASD.
The datasets presented in this study can be found in online repositories. The names of the repository/repositories and accession number(s) can be found below: (https://www.ncbi.nlm.nih.gov/, SRA254243).
The studies involving human participants were reviewed and approved by Institutional Review Board of Children’s Hospital, Chongqing Medical University. Written informed consent to participate in this study was provided by the participants’ legal guardian/next of kin.
XH and JZ conducted data collection and analysis, and drafted and revised the manuscript. JZ, TY, MG, and QL supervised the collection and analysis of ASD patient samples. TL and JC conceived and designed the research, performed data analysis and interpretation, revised the article, and conducted general supervision. All authors contributed to the article and approved the submitted version.
This work was supported by the Key Scientific and Technological Projects of Guangdong Province (2018B030335001) and Guangzhou City (202007030002).
The authors declare that the research was conducted in the absence of any commercial or financial relationships that could be construed as a potential conflict of interest.
We thank all families and children who participated in the survey. We thank all doctors of the Children’s Hospital of Chongqing Medical University and Hainan Women and Children’s Medical Center for their assistance.
1. Chanques G, Ely EW, Garnier O, Perrigault F, Eloi A, Carr J, et al. The 2014 updated version of the Confusion Assessment Method for the Intensive Care Unit compared to the 5th version of the Diagnostic and Statistical Manual of Mental Disorders and other current methods used by intensivists. Ann Intensive Care (2018) 8(1):33–. doi: 10.1186/s13613-018-0377-7
2. Allik H, Larsson J-O, Smedje H. Sleep patterns of school-age children with Asperger syndrome or high-functioning autism. J Autism Dev Disord (2006) 36(5):585–95. doi: 10.1007/s10803-006-0099-9
3. Couturier JL, Speechley KN, Steele M, Norman R, Stringer B, Nicolson R. Parental perception of sleep problems in children of normal intelligence with pervasive developmental disorders: prevalence, severity, and pattern. J Am Acad Child Adolesc Psychiatry (2005) 44(8):815–22. doi: 10.1097/01.chi.0000166377.22651.87
4. Malow BA, Marzec ML, McGrew SG, Wang L, Henderson LM, Stone WL. Characterizing sleep in children with autism spectrum disorders: a multidimensional approach. Sleep (2006) 29(12):1563–71. doi: 10.1093/sleep/29.12.1563
5. Polimeni MA, Richdale AL. Francis AJP. A survey of sleep problems in autism, Asperger’s disorder and typically developing children. J Intellect Disabil Res (2005) 49(Pt 4):260–8. doi: 10.1111/j.1365-2788.2005.00642.x
6. Bolte ER. Autism and Clostridium tetani. Med Hypotheses (1998) 51(2):133–44. doi: 10.1016/s0306-9877(98)90107-4
7. Ecker C, Suckling J, Deoni SC, Lombardo MV, Bullmore ET, Baron-Cohen S, et al. Brain anatomy and its relationship to behavior in adults with autism spectrum disorder: a multicenter magnetic resonance imaging study. Arch Gen Psychiatry (2012) 69(2):195–209. doi: 10.1001/archgenpsychiatry.2011.1251
8. Finegold SM. State of the art; microbiology in health and disease. Intestinal bacterial flora in autism. Anaerobe (2011) 17(6):367–8. doi: 10.1016/j.anaerobe.2011.03.007
9. Parracho HMRT, Bingham MO, Gibson GR, McCartney AL. Differences between the gut microflora of children with autistic spectrum disorders and that of healthy children. J Med Microbiol (2005) 54(Pt 10):987–91. doi: 10.1099/jmm.0.46101-0
10. Song Y, Liu C, Finegold SM. Real-time PCR quantitation of clostridia in feces of autistic children. Appl Environ Microbiol (2004) 70(11):6459–65. doi: 10.1128/AEM.70.11.6459-6465.2004
11. Tomova A, Husarova V, Lakatosova S, Bakos J, Vlkova B, Babinska K, et al. Gastrointestinal microbiota in children with autism in Slovakia. Physiol Behav (2015) 138:179–87. doi: 10.1016/j.physbeh.2014.10.033
12. Adams JB, Johansen LJ, Powell LD, Quig D, Rubin RA. Gastrointestinal flora and gastrointestinal status in children with autism–comparisons to typical children and correlation with autism severity. BMC Gastroenterol (2011) 11:22–. doi: 10.1186/1471-230X-11-22
13. Foster JA, McVey Neufeld K-A. Gut-brain axis: how the microbiome influences anxiety and depression. Trends Neurosci (2013) 36(5):305–12. doi: 10.1016/j.tins.2013.01.005
14. Desbonnet L, Clarke G, Shanahan F, Dinan TG, Cryan JF. Microbiota is essential for social development in the mouse. Mol Psychiatry (2014) 19(2):146–8. doi: 10.1038/mp.2013.65
15. Gilbert JA, Krajmalnik-Brown R, Porazinska DL, Weiss SJ, Knight R. Toward effective probiotics for autism and other neurodevelopmental disorders. Cell (2013) 155(7):1446–8. doi: 10.1016/j.cell.2013.11.035
16. Hsiao EY, McBride SW, Hsien S, Sharon G, Hyde ER, McCue T, et al. Microbiota modulate behavioral and physiological abnormalities associated with neurodevelopmental disorders. Cell (2013) 155(7):1451–63. doi: 10.1016/j.cell.2013.11.024
17. Stilling RM, van de Wouw M, Clarke G, Stanton C, Dinan TG, Cryan JF. The neuropharmacology of butyrate: The bread and butter of the microbiota-gut-brain axis? Neurochem Int (2016) 99:110–32. doi: 10.1016/j.neuint.2016.06.011
18. Liu S, Li E, Sun Z, Fu D, Duan G, Jiang M, et al. Altered gut microbiota and short chain fatty acids in Chinese children with autism spectrum disorder. Sci Rep (2019) 9(1):287. doi: 10.1038/s41598-018-36430-z
19. Ge X, Pan J, Liu Y, Wang H, Zhou W, Wang X. Intestinal Crosstalk between Microbiota and Serotonin and its Impact on Gut Motility. Curr Pharm Biotechnol (2018) 19(3):190–5. doi: 10.2174/1389201019666180528094202
20. Pagan C, Goubran-Botros H, Delorme R, Benabou M, Lemière N, Murray K, et al. Disruption of melatonin synthesis is associated with impaired 14-3-3 and miR-451 levels in patients with autism spectrum disorders. Sci Rep (2017) 7(1):2096–. doi: 10.1038/s41598-017-02152-x
21. Gabriele S, Sacco R, Persico AM. Blood serotonin levels in autism spectrum disorder: a systematic review and meta-analysis. Eur Neuropsychopharmacol J Eur Coll Neuropsychopharmacol (2014) 24(6):919–29. doi: 10.1016/j.euroneuro.2014.02.004
22. Benabou M, Rolland T, Leblond CS, Millot GA, Huguet G, Delorme R, et al. Heritability of the melatonin synthesis variability in autism spectrum disorders. Sci Rep (2017) 7(1):17746. doi: 10.1038/s41598-017-18016-3
23. Rellini E, Tortolani D, Trillo S, Carbone S, Montecchi F. Childhood Autism Rating Scale (CARS) and Autism Behavior Checklist (ABC) correspondence and conflicts with DSM-IV criteria in diagnosis of autism. J Autism Dev Disord (2004) 34(6):703–8. doi: 10.1007/s10803-004-5290-2
24. Cen CQ, Liang YY, Chen QR, Chen KY, Deng HZ, Chen BY, et al. Investigating the validation of the Chinese Mandarin version of the Social Responsiveness Scale in a Mainland China child population. BMC Psychiatry (2017) 17(1):51. doi: 10.1186/s12888-016-1185-y
25. Li S, Jin X, Yan C, Wu S, Jiang F, Shen X. Sleep problems in chinese school-aged children with a parent-reported history of ADHD. J Atten Disord (2009) 13(1):18–26. doi: 10.1177/1087054708322992
26. Owens JA, Spirito A, McGuinn M. The Children’s Sleep Habits Questionnaire (CSHQ): psychometric properties of a survey instrument for school-aged children. Sleep (2000) 23(8):1043–51. doi: 10.1093/sleep/23.8.1d
27. Cryan JF, O’Riordan KJ, Cowan CSM, Sandhu KV, Bastiaanssen TFS, Boehme M, et al. The Microbiota-Gut-Brain Axis. Physiol Rev (2019) 99(4):1877–2013. doi: 10.1152/physrev.00018.2018
28. Goldman BD. The circadian timing system and reproduction in mammals. Steroids (1999) 64(9):679–85. doi: 10.1016/s0039-128x(99)00052-5
29. Reiter RJ. The melatonin rhythm: both a clock and a calendar. Experientia (1993) 49(8):654–64. doi: 10.1007/bf01923947
30. Reiter RJ, Tan D-X, Fuentes-Broto L. Melatonin: a multitasking molecule. Prog Brain Res (2010) 181:127–51. doi: 10.1016/S0079-6123(08)81008-4
31. Sateia MJ. International classification of sleep disorders-third edition: highlights and modifications. Chest (2014) 146(5):1387–94. doi: 10.1378/chest.14-0970
32. Gozal D. Sleep-disordered breathing and school performance in children. Pediatrics (1998) 102(3 Pt 1):616–20. doi: 10.1542/peds.102.3.616
33. Maquet P. The role of sleep in learning and memory. Science (2001) 294(5544):1048–52. doi: 10.1126/science.1062856
34. Krakowiak P, Goodlin-Jones B, Hertz-Picciotto I, Croen LA, Hansen RL. Sleep problems in children with autism spectrum disorders, developmental delays, and typical development: a population-based study. J Sleep Res (2008) 17(2):197–206. doi: 10.1111/j.1365-2869.2008.00650.x
35. De Angelis M, Piccolo M, Vannini L, Siragusa S, De Giacomo A, Serrazzanetti DI, et al. Fecal microbiota and metabolome of children with autism and pervasive developmental disorder not otherwise specified. PloS One (2013) 8(10):e76993–e. doi: 10.1371/journal.pone.0076993
36. Kang D-W, Park JG, Ilhan ZE, Wallstrom G, Labaer J, Adams JB, et al. Reduced incidence of Prevotella and other fermenters in intestinal microflora of autistic children. PloS One (2013) 8(7):e68322–e. doi: 10.1371/journal.pone.0068322
37. Stilling RM, Dinan TG, Cryan JF. Microbial genes, brain & behaviour - epigenetic regulation of the gut-brain axis. Genes Brain Behav (2014) 13(1):69–86. doi: 10.1111/gbb.12109
38. Fung TC, Olson CA, Hsiao EY. Interactions between the microbiota, immune and nervous systems in health and disease. Nat Neurosci (2017) 20(2):145–55. doi: 10.1038/nn.4476
39. Miquel S, Martin R, Rossi O, Bermudez-Humaran LG, Chatel JM, Sokol H, et al. Faecalibacterium prausnitzii and human intestinal health. Curr Opin Microbiol (2013) 16(3):255–61. doi: 10.1016/j.mib.2013.06.003
40. Li M, Wang B, Zhang M, Rantalainen M, Wang S, Zhou H, et al. Symbiotic gut microbes modulate human metabolic phenotypes. Proc Natl Acad Sci U S A (2008) 105(6):2117–22. doi: 10.1073/pnas.0712038105
41. Flint HJ, Scott KP, Duncan SH, Louis P, Forano E. Microbial degradation of complex carbohydrates in the gut. Gut Microbes (2012) 3(4):289–306. doi: 10.4161/gmic.19897
42. Rosero JA, Killer Jí, Sechovcová H, Mrázek J, Benada Oi, Fliegerová Ki, et al. Reclassification of Eubacterium rectale (Hauduroy1937) Prévot 1938 in a new genus Agathobacter gen. nov. as Agathobacter rectalis comb. nov., and description of Agathobacter ruminis sp. nov., isolated from the rumen contents of sheep and cows. Int J Syst Evol Microbiol (2016) 66(2):768–73. doi: 10.1099/ijsem.0.000788
43. Macfarlane GT, Macfarlane S. Fermentation in the human large intestine: its physiologic consequences and the potential contribution of prebiotics. J Clin Gastroenterol (2011) 45 Suppl:S120–S7. doi: 10.1097/MCG.0b013e31822fecfe
44. Iriki T, Tamura K, Ishii M, Tanaka H, Miyamoto T, Onda K. Concentrations of ketone body and antidiuretic hormone in cerebrospinal fluid in response to the intra-ruminal administration of butyrate in suckling calves. Anim Sci J (2009) 80(6):655–61. doi: 10.1111/j.1740-0929.2009.00683.x
45. Gershon MD. Review article: serotonin receptors and transporters – roles in normal and abnormal gastrointestinal motility. Aliment Pharmacol Ther (2004) 20 Suppl 7:3–14. doi: 10.1111/j.1365-2036.2004.02180.x
46. Murphy DL, Lesch K-P. Targeting the murine serotonin transporter: insights into human neurobiology. Nat Rev Neurosci (2008) 9(2):85–96. doi: 10.1038/nrn2284
47. Gabriele S, Sacco R, Persico AM. Blood serotonin levels in autism spectrum disorder: a systematic review and meta-analysis. Eur Neuropsychopharmacol (2014) 24(6):919–29. doi: 10.1016/j.euroneuro.2014.02.004
48. Kulman G, Lissoni P, Rovelli F, Roselli MG, Brivio F, Sequeri P. Evidence of pineal endocrine hypofunction in autistic children. Neuro Endocrinol Lett (2000) 21(1):31–4.
49. Melke J, Goubran Botros H, Chaste P, Betancur C, Nygren G, Anckarsäter H, et al. Abnormal melatonin synthesis in autism spectrum disorders. Mol Psychiatry (2008) 13(1):90–8. doi: 10.1038/sj.mp.4002016
50. Pagan C, Delorme R, Callebert J, Goubran-Botros H, Amsellem F, Drouot X, et al. The serotonin-N-acetylserotonin-melatonin pathway as a biomarker for autism spectrum disorders. Transl Psychiatry (2014) 4:e479. doi: 10.1038/tp.2014.120
Keywords: gut microbiota, metabolism, autism spectrum disorders, sleep disorder, microbiota-gut-brain axis
Citation: Hua X, Zhu J, Yang T, Guo M, Li Q, Chen J and Li T (2020) The Gut Microbiota and Associated Metabolites Are Altered in Sleep Disorder of Children With Autism Spectrum Disorders. Front. Psychiatry 11:855. doi: 10.3389/fpsyt.2020.00855
Received: 06 May 2020; Accepted: 05 August 2020;
Published: 02 September 2020.
Edited by:
David Gozal, University of Missouri, United StatesReviewed by:
Lucie Jurek, Université Claude Bernard Lyon 1, FranceCopyright © 2020 Hua, Zhu, Yang, Guo, Li, Chen and Li. This is an open-access article distributed under the terms of the Creative Commons Attribution License (CC BY). The use, distribution or reproduction in other forums is permitted, provided the original author(s) and the copyright owner(s) are credited and that the original publication in this journal is cited, in accordance with accepted academic practice. No use, distribution or reproduction is permitted which does not comply with these terms.
*Correspondence: Jie Chen, amNoZW4wMTBAaG9zcGl0YWwuY3FtdS5lZHUuY24=; Tingyu Li, dHlsaUB2aXAuc2luYS5jb20=
Disclaimer: All claims expressed in this article are solely those of the authors and do not necessarily represent those of their affiliated organizations, or those of the publisher, the editors and the reviewers. Any product that may be evaluated in this article or claim that may be made by its manufacturer is not guaranteed or endorsed by the publisher.
Research integrity at Frontiers
Learn more about the work of our research integrity team to safeguard the quality of each article we publish.