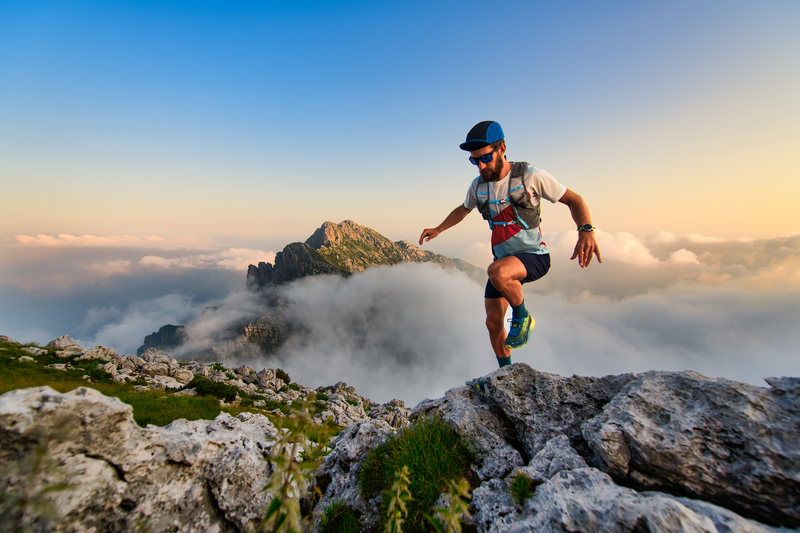
94% of researchers rate our articles as excellent or good
Learn more about the work of our research integrity team to safeguard the quality of each article we publish.
Find out more
ORIGINAL RESEARCH article
Front. Psychiatry , 21 July 2020
Sec. Mood Disorders
Volume 11 - 2020 | https://doi.org/10.3389/fpsyt.2020.00688
Disrupted circadian rhythms and sleep patterns are frequently observed features of psychiatric disorders, and especially mood disorders. Sleep deprivation treatment (SD) exerts rapid but transient antidepressant effects in depressed patients and has gained recognition as a model to study quick-acting antidepressant effects. It is of interest how locomotor activity patterns during SD might be associated with and potentially predict treatment response. The present study is an analysis of locomotor activity data, previously collected over a 24 h period, to examine the night of SD (Trautmann et al. 2018) as mood disorder patients suffering from a depressive episode (n = 78; after exclusions n = 59) underwent SD. In this exploratory analysis, the associations between response to SD, locomotor activity, and subjective mood during the 24 h period of SD were explored. Higher levels of activity overall were observed in non-responders (n = 18); in particular, non-responders moved more during the evening of SD until midnight and remained high thereafter. In contrast, activity in responders (n = 41) decreased during the evening and increased in the morning. Subjective mood was not found to be associated with locomotor activity. The window of data available in this analysis being limited, additional data from before and after the intervention are required to fully characterize the results observed. The present results hint at the possible utility of locomotor activity as a predictor and early indicator of treatment response, and suggest that the relationship between SD and locomotor activity patterns should be further investigated.
Disruption of sleep and circadian rhythms are often reported as features of psychiatric disorders, and chronotherapeutic treatments targeting the circadian system have shown the ability to affect disease symptoms (1–5). Therapeutic sleep deprivation (SD), involving a night without sleep, is one such treatment which has been found to rapidly induce an antidepressant response in depressed patients. Interestingly, this effect is transient with depressive symptoms reappearing directly after recovery sleep (6–10). While response to pharmacological antidepressants often takes weeks to emerge, SD can be quickly administered in a controlled fashion, providing an efficient model to study fast-acting antidepressants. SD Response is thought to be associated with various factors; several hypotheses about mechanisms of action have been proposed (11–14), with a popular one suggesting that SD acts through resetting circadian processes which are dysregulated in depression (15–19).
Altered psychomotor behavior has been found to be associated with many psychiatric disorders and disrupted circadian rhythmicity observed in affective disorders can also be reflected in locomotor activity patterns (20–29). While there is evidence suggesting that better mood is correlated with increased (everyday/non-exercise) locomotor activity (30–34), it is becoming apparent that the relationship between locomotor activity with psychiatric disorders is complex. For example, a pedigree study examining genetic contributions to circadian rhythms segregating by bipolar disorder (BD) severity showed that BD individuals consistently demonstrated lower levels of activity than their non-BD relatives (35). Studies in unrelated patients showed that mania patients display more complex activity patterns in the active morning period compared to depressed patients (36). Meanwhile, compared to healthy controls, individuals suffering from depression display lower mean activity levels (27, 36, 37) but higher variability over the whole day as well as in the active morning period (36). The characterization of these relationships is becoming increasingly feasible thanks to recent developments in wearable and mobile sensing technologies, especially in easy and non-invasive monitoring of locomotor activity (38–40) using wristwatch-type actigraph, making it a promising method which can be implemented with high compliance in clinical contexts.
It is of interest to explore how locomotor activity of patients changes through SD and whether this change is associated with response. Various actigraphy studies have been carried out in depressed populations (21–23, 30, 40, 41) but only a few studies in the SD context have assessed aspects of locomotor activity during treatment, and those in limited samples (18, 42–45). One study found that several manic-depressive patients emerging from depressive phases advanced awakening times, and in a depressed patient, that moving forward the sleep-wake cycle had an antidepressant effect (42). Another study investigated the effects of partial SD (PSD) on motor activity, finding that at baseline, responders moved more than non-responders and that all subjects increased activity following PSD (18). One other study, which used ethological methods to assess activation in a pre-SD interview, found the amount of “looking at the interviewer” to be negatively correlated with response to treatment, while observing a positive correlation between “body and object-touching hand movements” and SD response (43). More recently, a study using repeated SD and light therapy in 39 bipolar patients experiencing depressive episodes observed that responders showed increases in activity during the day, and advance in phase of activity-rest rhythms as well as reduced sleep at night after SD, while in contrast, non-responders showed no such changes (44). Another recent paper on 34 patients with major depression did not observe significant differences in activity related to response on the day before SD; during the night, compared to non-responders, responders had increased activity and rested less, and were more active the day after SD (45).
In a recent study, we examined the clinical and genetic factors associated with SD response in a large SD sample (46). In this naturalistic study, SD was conducted in 78 depressed major mood disorder inpatients; 72% responded to SD. Responders and non-responders differed in mood, but not in symptom severity or chronotype. Response was also found to be associated with being younger during SD treatment, and with higher age at time of lifetime disease onset. Significantly higher polygenic risk scores for depression were found in non-responders than healthy controls, while responders had intermediate risk scores.
In the present exploratory secondary analysis, we examined the relationship between SD response and locomotor activity which had been acquired during SD treatment in the same sample (46). Locomotor activity and measurements of subjective mood had been acquired over the course of 1 day as patients underwent SD therapy. Given the equivocal nature of previous reports of locomotor activity in responders and non-responders during SD, we compared the trajectories of locomotor activity in responders vs. non-responders. The relationship between locomotor activity and subjective mood was also examined.
The cohort studied here has been previously described in (46). As an exploratory secondary analysis, we examined locomotor activity data acquired during SD in the same sample. Seventy-eight mood disorder inpatients (34F; age in years mean ± std. dev. = 43.54 ± 14.80, major depressive disorder, n = 71; BD I, n = 6; and BD II, n = 1) experiencing major depressive episodes (ICD-10) were recruited from consecutive admissions to the depression unit of the Department of Psychiatry and Psychotherapy of the Central Institute of Mental Health (CIMH, Mannheim, Germany). Patients had to have been on stable medication regimens for 5+ days prior to undergoing SD. The study was approved by the CIMH ethics committee (II). After being given an explanation of the study, all participants gave written informed consent.
Baseline variables were assessed on Day 1 (Figure 1). Patients engaged in regular ward activities in the daytime on Day 2. In the evening on Day 2, small groups (1–5 patients) underwent staff-supervised SD. Participants stayed awake for ~36 h from 6:00 am (Day 2) to 6:00 pm (Day 3). On Day 3, patients returned to regular ward activities until they began recovery sleep (6:00 pm–1:00am).
Figure 1 Experimental timeline and sample subjective mood and locomotor data (mg) for a responder and non-responder over the course of sleep deprivation treatment (thicker bar). Response was evaluated between 1600 and 1700 h on Day 3. Dark blue bar denotes time during which locomotor activity was recorded.
The senior clinical researcher (MD) assessed response to SD using the seven-point Clinical Global Impression Scale of Global Improvement or Change (CGIC) (47) between 4:00 and 5:00pm on Day 3. In the CGIC, the clinician assesses improvement or worsening of the illness relative to before the intervention. Response was dichotomized: response was defined as scores of ≤2 (1: very much improved; 2: much improved) and non-response was define as scores ≥3 (3: minimally improved; 4: No change; 5: Minimally worse; 6: Much worse; 7: very much worse).
The SOMNOwatch (SOMNOmedics GmbH, Germany), a wrist-worn actigraph equipped with three activity sensors (x, y, z-axis), from which acceleration data are stored (magnitude, calculated as the square root of x2 + y2 + z2), was used to assess locomotor activity. The range of measurement of the SOMNOwatch is ±8.7 G, and the device has a sensitivity of 0.004 G. Activity was sampled at 60 Hz and stored every 60 s. The devices were worn from 5:00 pm on Day 2 to 6:00 pm on Day 3 (i.e. until recovery sleep, a total of 1,500 min). Patients had been instructed to wear actigraphs at all times except for when they were in the shower or engaging in exercise.
Participants were asked to record if they removed the devices on a wear log. Data were transferred to computer via USB connection.
Participants completed a VAS (48) assessing subjective mood (hereafter referred to as “mood”) at 2-h intervals from 10:00 am on Day 2 to 6:00 pm on Day 3. Possible ratings ranged from “worst mood imaginable (0)” to “best mood imaginable (10)”; patients indicated their mood in 0.5 point increments. Our previously reported study observed that mood was better in responders than non-responders (46).
IBM SPSS Statistics for Windows (v. 25) was used to perform statistical analyses. An alpha of 0.05 was used as the threshold for statistical significance.
Locomotor activity data were preprocessed prior to analysis. Four subjects who were excluded in the previous study for napping were also excluded in the present analysis. No locomotor data was available for five individuals, whose data were excluded from the analysis. Individual data traces were visually inspected. Furthermore, data from patients (n = 7) who had more than 60 consecutive minutes of no activity detected during the recording period were excluded (potentially indicating that they had removed the devices, and perhaps had forgotten to wear them again for a period of time). It was decided that 60 min would be used as a reasonable allowance of time for a patient to engage in exercise or taking a shower.
Mean locomotor activity was calculated for a window of 60 min around (i.e. +/−30 min) VAS mood assessments, as in (31), hereafter referred to as “locomotor activity.” A total of 12 mood assessments (every 2 h from Day 2 at 6:00 pm to Day 3 at 4:00 pm) from each subject were included to match the window recorded locomotor activity. Three subjects for whom no mood data were available were excluded from analysis, giving 59 patients who entered the final analysis, comprising 691 matched mood (a few participants did not indicate mood for several time points) and locomotor activity values. It should be noted that while the total sample was the same as in (46), there were differences in the data available, for example, in the present analysis, patients without locomotor data were excluded, but included in the previous study as they had genetic data.
To explore relationships between response to SD, locomotor activity, and mood, linear mixed models were employed (49). Models with random intercepts and random or fixed slopes for within-subject variables were estimated using maximum likelihood estimation, using a stepwise approach, and the goodness-of-fit of models was compared by using the deviance test on -2 log likelihood. Akaike’s Information Criterion (AIC) was also examined and agreed with the results of the deviance test. Non-significant random effects were not included in the model, and where models did not differ significantly, the more parsimonious model (with fewer effects) was chosen. The resulting main model was specified as follows. Locomotor activity was specified as the dependent variable. Response (response/non-response) and sex (female/male) were included as fixed factors. BMI, age, mood centered around subject mean, and mean subject mood were included as fixed continuous variables. Time point and time point2 (to model a circadian effect) were also included as fixed continuous variables. Time point was also added as a random effect. Interactions between Response x time point as well as response x time point2 were specified as fixed effects. Time point variables were centered to midnight. The intercept was included in the model as a random effect.
To further examine associations of locomotor activity with response to treatment and subjective mood at specific time points, another model was specified with the same fixed factors and continuous variables as the above main model, but with time point included as a fixed categorical factor. Time point2 was not included.
Sixty-nine point five percent of patients (41/59) were evaluated to be responders while 30.5% (18/59) were non-responders. The main mixed model for mean locomotor activity (see Table 1) revealed main effects of response, with non-responders moving more than responders (t(66.856) = 2.570, p = 0.012). A significant main effect of time point2 was observed, indicating a U-shaped curve of activity (t(582.872) = 2.730, p = 0.007). A significant main effect of age was observed, with increased age associated with decreased locomotor activity (t(58.621) = −2.874, p = 0.006). No significant effects of subject mean-centered mood, mean subject mood, sex, BMI, and time point were observed (all p > 0.05). A significant random effect of time point was observed (p = 0.02), revealing variability between subjects in the within-subject relationship between time point and locomotor activity. To examine whether diagnosis of bipolar disorder influenced results, the analysis was repeated with a variable differentiating between unipolar/bipolar depression—no significant differences were observed and the variable was not included in the analysis.
The model calculated using time point specified as a discrete variable observed only a significant main effect of age (t(58.222) = −2.750, p = 0.008); no other fixed effects were significant. The interaction term between response x time point revealed higher locomotor activity levels in non-responders the evening of SD (i.e. 2000 h t(632.876) = 2.178, p = 0.030; 2200 h t(632.873) = 2.046, p = 0.041; 2400 h t(632.871) = 2.028, p = 0.043). Figure 2 shows trajectories of locomotor activity for responders and non-responders.
Figure 2 Locomotor activity trajectories for Responders (n = 41) and Non-Responders (n = 18). Figure is drawn based on estimated marginal means calculated in mixed model with time specified as a discrete variable. Error bars denote standard error of mean. *p < 0.05.
Next, a post-hoc analysis was used to examine whether locomotor activity and mood might be differentially correlated between responders and non-responders. The same model as above was used, stratifying by response status. Mood trajectory (subject centered mood) was not significantly associated with locomotor activity in responders (t(440.277) = −1.057, p = 0.291) or non-responders (t(190.845) = 0.882, p = 0.379).
In responders, a significant main effect of time point2 (t(440,710) = 2.559, p = 0.011) and no significant effects of mean subject mood, sex, time point, age, and BMI were observed. In non-responders, a significant main effect of age (t(17.637) = −2.885, p = 0.010) and no significant effects of time point, time point2, mean subject mood, sex, and BMI (all p > 0.05) were observed.
The present analysis examined locomotor activity throughout a 24-h time period as depressed patients underwent SD. We observed higher activity in non-responders compared to responders throughout SD and the differences were most pronounced during the evening of the day of SD until midnight. Activity remained constantly high in non-responders while in responders a dip in activity was observed, followed by an increase over time; the post-hoc test stratified for response showed a significant U-shaped curve in responders but not non-responders.
One interpretation of the present observations is that non-responders have more severely disrupted rest-activity cycles. Lower relative amplitude, or difference between activity in active and rest periods, as assessed via actigraphy, has been associated with higher risk for mental health issues and poorer subjective wellbeing (20). It has also been observed that compared to healthy controls, depressed patients have lower activity levels during the day time but higher activity levels at night (23). It is possible that the circadian activity of responders is closer to that of healthy controls, while non-responders may have more disrupted circadian rhythms, thus showing decreased amplitude. Indeed, our findings are in line with a study observing that responders showed changes to activity-rest patterns while non-responders did not (44); blunting of circadian amplitude is suggested to be the main chronobiological abnormality in depression (50).
Unfortunately, many prior SD studies are not directly comparable, as they have used e.g., differing SD regimens (18, 44), actigraphy assessments (see below) and/or different definitions of response (compared to ours and each other). The present findings offer a different perspective from (18), who observed that responders showed higher locomotor activity pre-SD, however, they reported activity during the day and did not record in the late evening, as well as from (45), who reported that responders have higher activity than non-responders during SD. While some studies define response as % reduction in composite scores of ratings such as HAM-D (44, 45) the CGIC (47) performed by experienced clinicians which is a comparative rating well suited to assessing change was used in the present study. As an analysis of pre-existing data, the present study examined locomotor activity only during the SD regimen, in the hospital environment, as opposed to during regular activity. Although differences were observed in the evening before regular sleep hours (i.e. still part of the patient’s “regular” circadian pattern), further investigation outside of the SD protocol will be needed to clarify movement patterns and their meaning.
Studies employing actigraphy in studying response to pharmacological antidepressant treatments (largely using pre-post designs) are also not yet conclusive as to their relationships (51), with some studies observing no changes in motor activity associated with treatment response (52) while others suggest that, for example, the change in quantity of actigraphic movements reflects change in depression psychopathology (53). That said, our results might fit with a previous actigraphic pharmacological study on treatment response, which reported that activity at treatment onset and clinical improvement were negatively correlated, and which observed a trend towards higher activity in nonresponders than responders, and which suggested further exploration of this difference as a predictor of treatment response (54).
It has been proposed that one use of actigraphy might be to identify agitation in depression (23) and the present sample offers an additional point to examine. In our previous report, depressive symptom severity was assessed by clinician (Montgomery-Åsberg Depression Rating Scale) (55) and patient (Beck Depression Inventory-II, BDI-II, self-report version) (56), with consistent significant correlations observed between the scales (46); no differences were observed at baseline between responders and non-responders. The BDI-II contains an item assessing the symptom “agitation.” We conducted an additional post-hoc test (one-way analysis of variance) to examine whether responders and non-responders differed in BDI-II “agitation” scores, finding that baseline (p = 0.698) and post-SD (p = 0.354) scores did not differ between groups. This suggests that results did not reflect differences in agitation (i.e. agitated depression), but it may also be that this is a demonstration of an advantage that actigraphy, as an objective measure, can add to classical assessment methods.
Mood is often positively correlated to locomotor activity in the literature, but this was not found in the present results; this may be explained by several factors. As shown in our previous work (46), subjective mood assessments do not necessarily reflect the same changes as did the global clinical judgement of response. Next, the present mood data were acquired in the midst of the fatigue-inducing SD intervention whereas positive mood-locomotor correlations are often observed in data acquired during daily life. Furthermore, it is uncertain whether mood improvements resulting from SD, changes in locomotor activity, and their relationship, are linear processes with respect both to magnitude and timing and it may also be that the present dataset is too sparse to exhibit associations. Additional research examining different dimensions of mood and assessing specific factors (e.g. depressive symptoms) may help to clarify relationships with locomotor activity.
Varying protocols and devices used so far make it difficult to assess the interaction between locomotor activity and therapeutic responsiveness (57), and this is a relevant factor in interpreting the present findings in context to the literature. A detailed description of different actigraphic methodologies is beyond the scope of this article, and more detail can be found elsewhere (58–60) (Table S1 provides an overview of actigraphic models used in relevant studies).
Briefly, the digitally integrated magnitude vector acquired in the present study is the most recently developed and yields a more comprehensive measure of physical acceleration than other methods (time-over threshold, zero-crossing), taking into account the amplitude of movement. It is not that one method is necessarily better than the others, but researchers should be aware of the differences between devices as different types and settings can produce very different amounts of activity when recording the same thing (60).
There were several limitations to this study. First, given that this was a naturalistic study and recruitment was done from consecutive admissions, patients were not randomized or stratified during recruitment. Second, the present analysis explored a pre-existing dataset in which the recording period was restricted, providing a useful but limited picture; future research should monitor locomotor activity for extended periods preceding (potentially informing patient screening) and following SD (to examine effects of SD on rest-activity patterns), allowing comparison with baseline and post treatment activity. To better test the utility of locomotor activity as a distinguishing feature which can predict response and non-response, recording during a run-in baseline period will be required. Next, while individual locomotor activity traces were inspected carefully, it may not be possible to definitively distinguish periods of time where participants may have been briefly resting, from when they may have taken short naps, using actigraphy alone. This issue requires further examination. It has been observed that naps potentially disrupt a successful SD response and can reverse antidepressant effects (61). While this was not an issue during night-time supervised SD and during ward activities the next day, we cannot exclude that brief naps may have occurred in some of the patients, possibly leading to early relapse to depression and thus potential misclassification of responders as non-responders. However, given clinical protocols, we believe that misclassification is unlikely—patients were seen in ward rounds the morning after SD and given CGI ratings in the afternoon. The CGI was assessed by a clinician very familiar with the patient and also based on reports about SD response from staff on the ward accompanying the patient throughout the night.
Prior findings and those from the present study suggest to further explore the correlation between locomotor activity and mood and its potential role as a predictor of treatment response. Availability and use of wearable and mobile sensing devices has increased exponentially (38), and given the suitability of the devices and the ambulatory assessment approach (62, 63) to longitudinally study sleep and circadian disruptions, they should be employed further to study psychopharmacologic effects (57). We propose that application of such an approach, augmented with the acquisition of additional physiological, biological, and behavioral factors will go beyond the identification of predictors and eventually lead to better understanding of mechanisms behind not only fast-acting treatments such as SD, but of disease mechanisms on a larger scale.
The data analyzed in this study is subject to the following licenses/restrictions: privacy regulations. Requests to access these datasets should be directed to the corresponding author.
The studies involving human participants were reviewed and approved by Central Institute of Mental Health Ethics Committee (II). The patients/participants provided their written informed consent to participate in this study.
JF: formal analysis, writing-original draft, review and editing (R&E), visualization. LS: writing-R&E. NT: investigation, writing-R&E. JK: formal analysis, writing-R&E. SW: conceptualization, methodology, writing-R&E. FS: writing-R&E. JF: writing-R&E. LZ: writing-R&E. AM-L: resources, writing-R&E. UE-P: writing-R&E. CS: resources, writing-R&E. MS: resources, writing-R&E. YY: writing-R&E. MG: project administration, supervision, investigation. MD: writing-R&E, project administration, supervision, investigation, conceptualization, methodology, funding acquisition. MR: writing-R&E, supervision, investigation, conceptualization, methodology, funding acquisition.
This study was supported by the German Federal Ministry of Education and Research (BMBF) under the e:Med Programme (Target-OXY: 031L0190A) and ERA-NET NEURON (EMBED: 01EW1904 and SYNSCHIZ: 01EW1810). AM-L acknowledges support from the BMBF (Grants 01ZX1314G, 01GS08147, 01EF1803A, 255156212 CRC 1158 subproject B09) and the Ministry of Science, Research and the Arts of the State of Baden-Wuerttemberg, Germany (MWK, grants 42-04HV.MED(16)/16/1, 42-04HV.MED(16)/27/1). The funders had no involvement in the study design, collection or analysis and interpretation of data, writing of the report, or decision to submit the manuscript for publication.
AM-L has received consultant fees from Boehringer Ingelheim, Brainsway, Elsevier, Lundbeck Int. Neuroscience Foundation and Science Advances.
The remaining authors declare that the research was conducted in the absence of any commercial or financial relationships that could be construed as a potential conflict of interest.
The Supplementary Material for this article can be found online at: https://www.frontiersin.org/articles/10.3389/fpsyt.2020.00688/full#supplementary-material
1. Salvatore P, Indic P, Murray G, Baldessarini RJ. Biological rhythms and mood disorders. Dialogues Clin Neurosci (2012) 14(4):369–79.
2. Smolensky MH, Hermida RC, Reinberg A, Sackett-Lundeen L, Portaluppi F. Circadian disruption: New clinical perspective of disease pathology and basis for chronotherapeutic intervention. Chronobiol Int (2016) 33(8):1101–19. doi: 10.1080/07420528.2016.1184678
3. Logan RW, McClung CA. Rhythms of life: circadian disruption and brain disorders across the lifespan. Nat Rev Neurosc (2019) 20(1):49–65. doi: 10.1038/s41583-018-0088-y
4. Barnes J, Mondelli V, Pariante CM. Genetic Contributions of Inflammation to Depression. Neuropsychopharmacology (2017) 42(1):81–98. doi: 10.1038/npp.2016.169
5. Wirz-Justice A, Benedetti F. Perspectives in affective disorders: Clocks and sleep. Eur J Neurosci (2019) 51:346–65. doi: 10.1111/ejn.14362
6. Gillin JC. The sleep therapies of depression. Prog Neuropsychopharmacol Biol Psychiatry (1983) 7(2-3):351–64. doi: 10.1016/0278-5846(83)90123-9
7. Wu JC, Bunney WE. The biological basis of an antidepressant response to sleep deprivation and relapse: review and hypothesis. Am J Psychiatry (1990) 147(1):14–21. doi: 10.1176/ajp.147.1.14
8. Benedetti F, Barbini B, Colombo C, Smeraldi E. Chronotherapeutics in a psychiatric ward. Sleep Med Rev (2007) 11(6):509–22. doi: 10.1016/j.smrv.2007.06.004
9. Benedetti F, Colombo C. Sleep deprivation in mood disorders. Neuropsychobiology (2011) 64(3):141–51. doi: 10.1159/000328947
10. Giedke H, Schwärzler F. Therapeutic use of sleep deprivation in depression. Sleep Med Rev (2002) 6(5):361–77. doi: 10.1053/smrv.2002.0235
11. Wolf E, Kuhn M, Normann C, Mainberger F, Maier JG, Maywald S, et al. Synaptic plasticity model of therapeutic sleep deprivation in major depression. Sleep Med Rev (2016) 30:53–62. doi: 10.1016/j.smrv.2015.11.003
12. Borbély AA, Wirz-Justice A. Sleep, sleep deprivation and depression. A hypothesis derived from a model of sleep regulation. Hum Neurobiol (1982) 1(3):205–10.
13. Borbély AA, Daan S, Wirz-Justice A, Deboer T. The two-process model of sleep regulation: a reappraisal. J Sleep Res (2016) 25(2):131–43. doi: 10.1111/jsr.12371
14. Bunney BG, Bunney WE. Mechanisms of rapid antidepressant effects of sleep deprivation therapy: clock genes and circadian rhythms. Biol Psychiatry (2013) 73(12):1164–71. doi: 10.1016/j.biopsych.2012.07.020
15. Bunney BG, Li JZ, Walsh DM, Stein R, Vawter MP, Cartagena P, et al. Circadian dysregulation of clock genes: clues to rapid treatments in major depressive disorder. Mol Psychiatry (2015) 20(1):48–55. doi: 10.1038/mp.2014.138
16. Haug HJ. Prediction of sleep deprivation outcome by diurnal variation of mood. Biol Psychiatry (1992) 31(3):271–8. doi: 10.1016/0006-3223(92)90050-A
17. Reinink E, Bouhuys N, Wirz-Justice A, van den Hoofdakker R. Prediction of the antidepressant response to total sleep deprivation by diurnal variation of mood. Psychiatry Res (1990) 32(2):113–24. doi: 10.1016/0165-1781(90)90077-I
18. Szuba MP, Baxter LR, Fairbanks LA, Guze BH, Schwartz JM. Effects of partial sleep deprivation on the diurnal variation of mood and motor activity in major depression. Biol Psychiatry (1991) 30(8):817–29. doi: 10.1016/0006-3223(91)90237-G
19. Martiny K, Refsgaard E, Lund V, Lunde M, Sørensen L, Thougaard B, et al. The Day-to-Day Acute Effect of Wake Therapy in Patients with Major Depression Using the HAM-D6 as Primary Outcome Measure: Results from a Randomised Controlled Trial. PloS One (2013) 8(6):1–12. doi: 10.1371/journal.pone.0067264
20. Lyall LM, Wyse CA, Graham N, Ferguson A, Lyall DM, Cullen B, et al. Association of disrupted circadian rhythmicity with mood disorders, subjective wellbeing, and cognitive function: a cross-sectional study of 91 105 participants from the UK Biobank. Lancet Psychiatry (2018) 5(6):507–14. doi: 10.1016/S2215-0366(18)30139-1
21. Teicher MH. Actigraphy and motion analysis: new tools for psychiatry. Harv Rev Psychiatry (1995) 3(1):18–35. doi: 10.3109/10673229509017161
22. Teicher MH, Glod CA, Magnus E, Harper D, Benson G, Krueger K, et al. Circadian rest-activity disturbances in seasonal affective disorder. Arch Gen Psychiatry (1997) 54(2):124–30. doi: 10.1001/archpsyc.1997.01830140034007
23. Burton C, McKinstry B, Szentagotai Tătar A, Serrano-Blanco A, Pagliari C, Wolters M. Activity monitoring in patients with depression: a systematic review. J Affect Disord (2013) 145(1):21–8. doi: 10.1016/j.jad.2012.07.001
24. Indic P, Murray G, Maggini C, Amore M, Meschi T, Borghi L, et al. Multi-scale motility amplitude associated with suicidal thoughts in major depression. PloS One (2012) 7(6):e38761. doi: 10.1371/journal.pone.0038761
25. Walther S, Hugli S, Hofle O, Federspiel A, Horn H, Bracht T, et al. Frontal white matter integrity is related to psychomotor retardation in major depression. Neurobiol Dis (2012) 47(1):13–9. doi: 10.1016/j.nbd.2012.03.019
26. Volkers AC, Tulen JH, van den Broek WW, Bruijn JA, Passchier J, Pepplinkhuizen L. Motor activity and autonomic cardiac functioning in Variance. J Affect Disord (2003) 76(1-3):23–30. doi: 10.1016/s0165-0327(02)00066-6
27. Berle JO, Hauge ER, Oedegaard KJ, Holsten F, Fasmer OB. Actigraphic registration of motor activity reveals a more structured behavioural pattern in schizophrenia than in major depression. BMC Res Notes (2010) 3:149. doi: 10.1186/1756-0500-3-149
28. Hallgren M, Herring MP, Owen N, Dunstan D, Ekblom O, Helgadottir B, et al. Exercise, Physical Activity, and Sedentary Behavior in the Treatment of Depression: Broadening the Scientific Perspectives and Clinical Opportunities. Front Psychiatry (2016) 7:36. doi: 10.3389/fpsyt.2016.00036
29. Nakamura T, Kiyono K, Wendt H, Abry P, Yamamoto Y. Multiscale Analysis of Intensive Longitudinal Biomedical Signals and Its Clinical Applications. Proc IEEE (2016) 104(2):242–61. doi: 10.1109/JPROC.2015.2491979
30. Kim J, Nakamura T, Kikuchi H, Yoshiuchi K, Sasaki T, Yamamoto Y. Covariation of depressive mood and spontaneous physical activity in major depressive disorder: toward continuous monitoring of depressive mood. IEEE J BioMed Health (2015) 19(4):1347–55. doi: 10.1109/JBHI.2015.2440764
31. Kim J, Nakamura T, Kikuchi H, Sasaki T, Yamamoto Y. Co-variation of depressive mood and locomotor dynamics evaluated by ecological momentary assessment in healthy humans. PloS One (2013) 8(9):e74979. doi: 10.1371/journal.pone.0074979
32. Koch ED, Tost H, Braun U, Gan G, Giurgiu M, Reinhard I, et al. Mood Dimensions Show Distinct Within-Subject Associations With Non-exercise Activity in Adolescents: An Ambulatory Assessment Study. Front Psychol (2018) 9:268. doi: 10.3389/fpsyg.2018.00268
33. Kanning MK, Ebner-Priemer UW, Schlicht WM. How to Investigate Within-Subject Associations between Physical Activity and Momentary Affective States in Everyday Life: A Position Statement Based on a Literature Overview. Front Psychol (2013) 4:187. doi: 10.3389/fpsyg.2013.00187
34. Reichert M, Tost H, Reinhard I, Zipf A, Salize H-J, Meyer-Lindenberg A, et al. Within-Subject Associations between Mood Dimensions and Non-exercise Activity: An Ambulatory Assessment Approach Using Repeated Real-Time and Objective Data. Front Psychol (2016) 7:918. doi: 10.3389/fpsyg.2016.00918
35. Pagani L, St Clair PA, Teshiba TM, Service SK, Fears SC, Araya C, et al. Genetic contributions to circadian activity rhythm and sleep pattern phenotypes in pedigrees segregating for severe bipolar disorder. Proc Natl Acad Sci U.S.A. (2016) 113(6):E754–61. doi: 10.1073/pnas.1513525113
36. Krane-Gartiser K, Henriksen TE, Vaaler AE, Fasmer OB, Morken G. Actigraphically assessed activity in unipolar depression: a comparison of inpatients with and without motor retardation. J Clin Psychiatry (2015) 76(9):1181–7. doi: 10.4088/JCP.14m09106
37. Gershon A, Ram N, Johnson SL, Harvey AG, Zeitzer JM. Daily Actigraphy Profiles Distinguish Depressive and Interepisode States in Bipolar Disorder. Clin Psychol Sci (2016) 4(4):641–50. doi: 10.1177/2167702615604613
38. Kumar S, Nilsen W, Pavel M, Srivastava M. Mobile Health: Revolutionizing Healthcare Through Transdisciplinary Research. IEEE Computer (2013) 46(1):28–35. doi: 10.1109/MC.2012.392
39. Steinhubl SR, Muse ED, Topol EJ. The emerging field of mobile health. Sci Transl Med (2015) 7(283):283rv3. doi: 10.1126/scitranslmed.aaa3487
40. Reichert M, Lutz A, Deuschle M, Gilles M, Hill H, Limberger MF, et al. Improving motor activity assessment in depression: which sensor placement, analytic strategy and diurnal time frame are most powerful in distinguishing patients from controls and monitoring treatment effects. PloS One (2015) 10(4):e0124231. doi: 10.1371/journal.pone.0124231
41. Nakamura T, Takumi T, Takano A, Aoyagi N, Yoshiuchi K, Struzik ZR, et al. Of mice and men–universality and breakdown of behavioral organization. PloS One (2008) 3(4):e2050. doi: 10.1371/journal.pone.0002050
42. Wehr TA, Wirz-Justice A, Goodwin FK, Duncan W, Gillin JC. Phase advance of the circadian sleep-wake cycle as an antidepressant. Sci (New York NY) (1979) 206(4419):710–3. doi: 10.1126/science.227056
43. Bouhuys AL, Beersma DG, van den Hoofdakker RH. Observed behavior as a predictor of the response to sleep deprivation in depressed patients. Psychiatry Res (1989) 28(1):47–61. doi: 10.1016/0165-1781(89)90197-2
44. Benedetti F, Dallaspezia S, Fulgosi MC, Barbini B, Colombo C, Smeraldi E. Phase advance is an actimetric correlate of antidepressant response to sleep deprivation and light therapy in bipolar depression. Chronobiol Int (2007) 24(5):921–37. doi: 10.1080/07420520701649455
45. Bruckner TU, Wiegand MH. Motor activity in depressed patients during therapeutic sleep deprivation. Eur Psychiatry (2010) 25(8):465–7. doi: 10.1016/j.eurpsy.2009.11.004
46. Trautmann N, Foo JC, Frank J, Witt SH, Streit F, Treutlein J, et al. Response to therapeutic sleep deprivation: a naturalistic study of clinical and genetic factors and post-treatment depressive symptom trajectory. Neuropsychopharmacology (2018) 43:2572–7. doi: 10.1101/179457
47. Guy W. ECDEU Assessment Manual for Psychopharmacology - revised. Rockville, MD, U.S.: U.S. Department of Health, Education, and Welfare, Public Health Service, Alcohol, Drug Abuse, and Mental Health Administration, NIMH Psychopharmacology Research Branch, Division of Extramural Research Programs (1976).
48. Aitken RC. Measurement of feelings using visual analogue scales. Proc R Soc Med (1969) 62(10):989–93. doi: 10.1177/003591576906201005
49. Singer JD, Willett JB. Applied longitudinal data analysis: Modeling change and event occurrence. New York, NY, US: Oxford University Press (2003).
50. Souetre E, Salvati E, Belugou JL, Pringuey D, Candito M, Krebs B, et al. Circadian rhythms in depression and recovery: evidence for blunted amplitude as the main chronobiological abnormality. Psychiatry Res (1989) 28(3):263–78. doi: 10.1016/0165-1781(89)90207-2
51. Razavi N, Horn H, Koschorke P, Hugli S, Hofle O, Muller T, et al. Measuring motor activity in major depression: the association between the Hamilton Depression Rating Scale and actigraphy. Psychiatry Res (2011) 190(2-3):212–6. doi: 10.1016/j.psychres.2011.05.028
52. Baune BT, Caliskan S, Todder D. Effects of adjunctive antidepressant therapy with quetiapine on clinical outcome, quality of sleep and daytime motor activity in patients with treatment-resistant depression. Hum Psychopharmacol (2007) 22(1):1–9. doi: 10.1002/hup.817
53. Todder D, Caliskan S, Baune BT. Longitudinal changes of day-time and night-time gross motor activity in clinical responders and non-responders of major depression. World J Biol Psychia (2009) 10(4):276–84. doi: 10.3109/15622970701403081
54. Raoux N, Benoit O, Dantchev N, Denise P, Franc B, Allilaire JF, et al. Circadian pattern of motor activity in major depressed patients undergoing antidepressant therapy: relationship between actigraphic measures and clinical course. Psychiatry Res (1994) 52(1):85–98. doi: 10.1016/0165-1781(94)90122-8
55. Montgomery SA, Asberg M. A new depression scale designed to be sensitive to change. Br J Psychiatry (1979) 134:382–9. doi: 10.1192/bjp.134.4.382
56. Beck AT, Steer RA, Brown GK. Manual for Beck depression inventory-II. 2nd ed edition. San Antonio: Harcourt Brace (1996).
57. Stanley N. Actigraphy in human psychopharmacology: a review. Hum Psychopharmacol (2003) 18(1):39–49. doi: 10.1002/hup.471
58. Gorny SW, Spiro JR. Comparing different methodologies used in wrist actigraphy. Sleep Rev (2001) 40–2.
59. Blackwell T, Redline S, Ancoli-Israel S, Schneider JL, Surovec S, Johnson NL, et al. Comparison of sleep parameters from actigraphy and polysomnography in older women: the SOF study. Sleep (2008) 31(2):283–91. doi: 10.1093/sleep/31.2.283
60. Ancoli-Israel S, Cole R, Alessi C, Chambers M, Moorcroft W, Pollak CP. The role of actigraphy in the study of sleep and circadian rhythms. Sleep (2003) 26(3):342–92. doi: 10.1093/sleep/26.3.342
61. Riemann D, Wiegand M, Lauer CJ, Berger M. Naps after total sleep deprivation in depressed patients: are they depressiogenic? Psychiatry Res (1993) 49(2):109–20. doi: 10.1016/0165-1781(93)90099-3
62. Trull TJ, Ebner-Priemer U. Ambulatory assessment. Ann Rev Clin Psych (2013) 9:151–76. doi: 10.1146/annurev-clinpsy-050212-185510
Keywords: locomotor activity, sleep deprivation treatment, depression, mood, treatment response
Citation: Foo JC, Sirignano L, Trautmann N, Kim J, Witt SH, Streit F, Frank J, Zillich L, Meyer-Lindenberg A, Ebner-Priemer U, Schilling C, Schredl M, Yamamoto Y, Gilles M, Deuschle M and Rietschel M (2020) Association of Locomotor Activity During Sleep Deprivation Treatment With Response. Front. Psychiatry 11:688. doi: 10.3389/fpsyt.2020.00688
Received: 10 April 2020; Accepted: 30 June 2020;
Published: 21 July 2020.
Edited by:
Andrea De Bartolomeis, University of Naples Federico II, ItalyReviewed by:
Vincenzo Natale, University of Bologna, ItalyCopyright © 2020 Foo, Sirignano, Trautmann, Kim, Witt, Streit, Frank, Zillich, Meyer-Lindenberg, Ebner-Priemer, Schilling, Schredl, Yamamoto, Gilles, Deuschle and Rietschel. This is an open-access article distributed under the terms of the Creative Commons Attribution License (CC BY). The use, distribution or reproduction in other forums is permitted, provided the original author(s) and the copyright owner(s) are credited and that the original publication in this journal is cited, in accordance with accepted academic practice. No use, distribution or reproduction is permitted which does not comply with these terms.
*Correspondence: Jerome Clifford Foo, amVyb21lLmZvb0B6aS1tYW5uaGVpbS5kZQ==
Disclaimer: All claims expressed in this article are solely those of the authors and do not necessarily represent those of their affiliated organizations, or those of the publisher, the editors and the reviewers. Any product that may be evaluated in this article or claim that may be made by its manufacturer is not guaranteed or endorsed by the publisher.
Research integrity at Frontiers
Learn more about the work of our research integrity team to safeguard the quality of each article we publish.