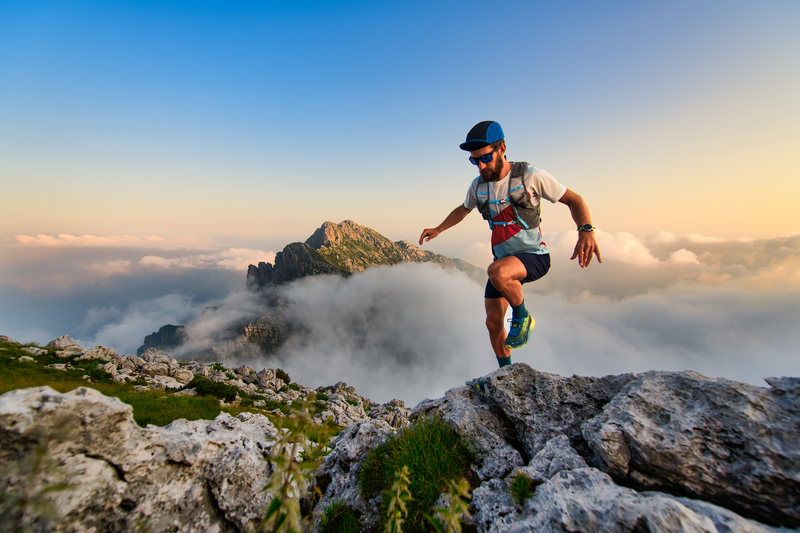
95% of researchers rate our articles as excellent or good
Learn more about the work of our research integrity team to safeguard the quality of each article we publish.
Find out more
STUDY PROTOCOL article
Front. Psychiatry , 21 April 2020
Sec. Schizophrenia
Volume 11 - 2020 | https://doi.org/10.3389/fpsyt.2020.00340
This article is part of the Research Topic Genetic Mechanisms of Biomarkers in Schizophrenia, Bipolar Disorder and Depression View all 18 articles
Background: The Seoul Pluripotent Risk for Mental Illness (SPRIM) study was designed to identify predictors leading to mental illness in help-seeking individuals by securing sufficient statistical power through transdiagnostic approaches. The SPRIM study aims to examine the clinical characteristics of high-risk individuals for mental illness and to identify proteomic biomarkers that can predict the onset of mental illness.
Methods: This paper describes the study protocol of the SPRIM study. We aim to recruit 150 participants who meet the criteria for high risk for major mental illness, 150 patients with major psychiatric disorders (schizophrenia, bipolar disorder, and major depressive disorder), and 50 matched healthy control subjects for 2 years. Clinical evaluations, self-report measures, and proteomic analyses will be implemented. The assessment points are at baseline, 6, 12, 18, and 24 months.
Conclusions: In the present study, we introduced the study protocol of the SPRIM study, which is the first prospective cohort study of transdiagnostic high-risk concepts using proteomic biomarkers. This study has a paradigm that encompasses various diseases without aiming at predicting and preventing the development of a specific mental illness in help-seeking individuals. The transdiagnostic high-risk concept could be extended to provide a perspective for people with various psychopathological tendencies below a threshold, such that they do not meet the existing diagnostic criteria of mental illnesses, to determine what may lead them to a specific disease and help identify appropriate preventative interventions.
For the past 20 years, the concept of ultra/clinical high-risk for psychosis (CHR-P) has been proposed and developed for the early detection and prevention of the transition to psychosis (1–4). Previous studies have shown good predictability compared with other medical diseases, and it was expected that effective interventions for early psychosis would soon be available (5). However, as time went on, the incidence of psychotic transition in CHR-P was found to gradually decrease in several cohort studies, and the reasons, such as lead-time bias due to early referral, high rate of false positives, and prevention through effective treatments, have been discussed (6–8). Recent studies have revealed that CHR-P is not pluripotent but highly specific for psychosis, and the problem of comorbidity is not significantly affected (9, 10), however since the necessity of specialized clinics with trained experts, we realized that the current high-risk concept covers only a fraction of the total schizophrenia incidence (11, 12). This epidemiologic inadequacy is also supported by the fact that psychosis still develops, although at a low rate, in clinical high-risk for non-psychotic mental disorders (13). Lack of the pragmatic transdiagnostic ability of the CHR-P designation and modest statistical power due to the recruitment difficulties and low conversion rate do not given much latitude in high-risk research (14, 15). As a way to overcome the limitation of statistical power, a method of expanding to a broad range of disorders has been proposed instead of limiting the outcome to schizophrenia. Recently, McGorry and his colleagues presented the CHARMS approach, a pioneering new concept, which is a transdiagnostic cohort program (16). This program is a high-risk program that covers a variety of outcomes, including psychotic disorder, bipolar disorder, depressive disorder, etc., unlike the existing CHR cohort program aimed at the onset of psychosis. The CHARMS approach is somewhat different from the current CHR concept that targets only schizophrenia and more closely reflects the clinical staging model that distinguishes a distress that needs only a little help or a stage that requires clinical attention just before transitioning over the threshold on the continuum of mental illness (17, 18). Despite the fact that there are already other high-risk approaches such as the bipolar prodrome, it is expected that the high-risk studies adopting this clinical staging perspective will provide a transdiagnostic understanding of mental illnesses (19–21). Although there have been many studies on the transition and remission in high-risk populations, few studies have been conducted using the definition of transdiagnostic high risk and investigated the long-term outcomes from this approach (22–24). However, this approach is a more common practice in the community because it is more practical for addressing mental illness that can be performed under normal circumstances and does not need a specialized clinic. The cohort program for a broad range of mental disorders is expected to provide greater flexibility in finding biomarkers to predict the development of mental disorders based on higher statistical power by recruiting a wider range of subjects.
The identification of biomarkers in mental illness plays an essential role in the differential diagnosis of disease and prediction of prognosis (25, 26). In psychiatry, which is based on phenotypic classification, biomarker research has a very long history (27, 28). In high-risk for psychosis, meta-analytical sequential testing simulations showed that probabilistic risk assessment using the information from clinical assessment has a greater predictive accuracy compared with that of the phenotypical model only (29). Moreover, considering phenotypical nondisjunction and biotypical reclassification of schizophrenia spectrum disorders or schizophrenia and bipolar disorder, applying the current high-risk concepts based on the existing classification systems of mental illness makes it more difficult to predict outcomes (30, 31). Therefore, a transdiagnostic approach with biomarkers would be a prerequisite for more accurate diagnosis and prognosis prediction. Recently, biomarker studies using proteomics have gained more attention (32, 33). Proteomics generally refers to the large-scale experimental analysis of proteins and proteomes; it has the advantage of being able to identify alterations in certain steps of the biochemical pathways of mental illness because it can distinguish the overexpression of specific proteins (34). Proteomic analyses are used to identify disease-specific alterations, including those for schizophrenia, bipolar disorder, and major depressive disorder (35). Furthermore, proteomics profiling has revealed that inflammatory biomarkers may serve as predictors of antidepressant treatment response (36). Besides, data-driven proteomic analysis using feature selection is expected to provide a new basis for the transdiagnostic approach (37, 38).
Since proteomic analysis is performed through blood sampling, it can be performed more easily than other biomarker studies, including neuroimaging or electrophysiology. However, no studies have used proteomics analyses in a high-risk group before the onse of the disease. Therefore, studies using proteomic biomarkers in a high-risk population before a transition to mental illnesses will enable further classification and prediction at the molecular level.
In this prospective cohort study, we have three goals. First, we will examine the clinical characteristics and clinical outcomes for subjects who do not meet the diagnostic criteria for mental illness, and these subjects will be classified as high-risk individuals for specific psychiatric disorders (schizophrenia, bipolar disorder, major depressive disorder, and undifferentiated). Second, we will identify proteomic biomarkers that differentiate (1) patients with mental illnesses and healthy individuals, and (2) patients with schizophrenia, bipolar disorder, and major depressive disorder. Third, we will attempt to explore the proteomic markers that predict the transition of the high-risk group to mental illness.
The Seoul Pluripotent Risk for Mental Illness (SPRIM) study is a prospective cohort study and aims to recruit 150 participants who meet the criteria for high risk for major mental illness for 2 years. To verify disease-specific proteomic profiles and to use them as templates for classifying and predicting the course of high-risk individuals, we will also recruit 150 patients with major psychiatric disorders (schizophrenia, bipolar disorder, major depressive disorder) and 50 matched, healthy control subjects.
Participants are being recruited from the Seoul National University Hospital, Seoul National University Bundang Hospital, SMG-SNU Boramae Medical Center, Hanyang University Hospital, Inha University Hospital, National Mental Health Center, Korean Armed Forces Capital Hospital, and Gwanak-gu Public Health Center. All institutions are located in Seoul, covering community, military service, and general hospital populations. All participants are referred to the Seoul Youth Clinic or Mood Disorders Clinic of Seoul National University Hospital and evaluated by experienced psychiatrists.
Potential participants are high-risk individuals for major mental disorders aged 15–45 who were recruited and referred to Seoul National University Hospital. High-risk subjects participating in the SPRIM study are classified into four categories. The inclusion criteria for each group are shown in Table 1. Patients with major mental disorders (schizophrenia, bipolar disorder, and major depressive disorder) who had been diagnosed within the previous 5 years are also being recruited from the Seoul National University Hospital. The exclusion criteria are as follows: the presence of mental retardation, a pervasive developmental disorder, neurological disorders, a history of head trauma with loss of consciousness, pregnancy, medical conditions that may cause mental illness, and substance abuse.
The first recruitment of high-risk subjects started in September 2017 and is expected to be completed in September 2019, and the follow-up evaluations will be continued until September 2021. After obtaining informed consent, a baseline interview is scheduled. If the subject is a minor, informed consent from their guardian is also obtained. A flow chart of the study procedure is shown in Figure 1. For the high-risk participants, they receive prospective follow-up evaluations, and the assessment points are at baseline, 6, 12, 18, and 24 months. A long-term follow-up investigation will continue at 6-month intervals if desired by the subjects, even if the 24-month follow-up period is over. All high-risk participants will receive case management and supportive psychotherapy on a bimonthly basis and will receive an intensive assessment within the 6-month intervals if they are expected to be converted or remitted. The conversion to a major mental illness and remission from the current high-risk status is determined by an intensive assessment and a consensus meeting. The definition of the transition was defined based on the diagnostic instruments 39, 40, and full remission was defined as no positive symptom item that met the severity corresponding to the criteria for a high-risk group and attribution criteria > 6 months. The patients with major mental disorders are also evaluated at baseline.
At baseline, the high-risk participants are interviewed and diagnosed by a psychiatrist who has had many years of experience through the following diagnostic instruments: Structured Clinical Interview for the DSM-IV (SCID-I, II); Structured Interview for Prodromal Syndrome (SIPS) (39); and Bipolar Prodrome Symptom Scale-Prospective (BPSS-P) (40). To assess the clinical status and function at baseline, the following scales are performed: Positive and Negative Syndrome Scale (PANSS); Hamilton Depression Rating Scale (HAM-D) (41); Hamilton Anxiety Rating Scale (HAM-A) (42); Young's mania rating scale (YMRS) (43); Biological Rhythms Interview of Assessment in Neuropsychiatry (BRIAN) (44); Clinical Global Impression-Severity (CGI-S); Global Assessment of Functioning (GAF) (45); and Global Functioning: Social and Role Scales (GF) (46). The high-risk participants are also interviewed at the 6-, 12-, 18-, and 24-month follow-ups using the same instruments. For the patients with major mental disorders, they are also interviewed at baseline by the psychiatrist using the following diagnostic instruments: SCID-I, II; HAM-D; HAM-A; YMRS; CGI-S; GAF; and Brief Psychiatric Rating Scale (BPRS).
At baseline, the high-risk participants complete the following self-report measures: Barratt Impulsiveness Scale (BIS) (47); Childhood Trauma Questionnaire (CTQ) (48); Morningness-Eveningness Questionnaire (MEQ) (49); WHO Quality of Life-BREF (WHOQOL-BREF) (50); Connor-Davidson Resilience Scale (CD-RISC) (51); Prodromal Questionnaire-Brief (PQ-B) (52); Quick Inventory of Depressive Symptomatology (QIDS) (53); Seasonal Pattern Assessment Questionnaire (SPAQ) (54); and State-Trait Anxiety Inventory (STAI) (55). The high-risk participants also complete the same self-report instruments at the 6-, 12-, 18-, and 24-month follow-up evaluations.
To elucidate potential blood biomarkers of (1) the disease-specific alterations in schizophrenia, bipolar disorder, and major depressive disorder and (2) the transition to major psychiatric disorders in high-risk groups, mass spectrometry-based proteomics analysis is performed in both the high-risk participants and the patients with major mental disorders. For protein identification and label-free quantification, mass spectra were processed, and peptide lists were searched against the human UniProt FASTA database (version 2014.10). After the plasma collection, sample preparations including protein digestion and peptide purification are performed as described above. Target proteins that are discovered in the major psychiatric disorder cohort are analyzed in the individual samples by liquid chromatography-tandem mass spectrometry (LC-MRM-MS) run using triple quadrupole (QQQ) mass spectrometry. These results are used as a template for each disease to validate the proteomic profiles in high-risk subjects. For proteomic analysis of blood biomarkers in a prospective cohort of transdiagnostic high-risk participants, targeted quantification is performed using multiple reaction monitoring (MRM) (56).
Descriptive statistics will be used to show the demographic and clinical characteristics of the participants at baseline and to examine the baseline characteristics in relation to the outcomes of the subjects. The Kaplan-Meier survival analysis will be used to examine the transition or remission in each high-risk group and the high-risk group as a whole. Cox regression will be used to determine the difference in hazard ratios between each high-risk group and in the high-risk group as a whole.
In the present study, we introduced the study protocol of the SPRIM study, which is the first prospective cohort study of transdiagnostic high-risk concepts using proteomic biomarkers. This study, an extension of studies of high-risk patients with psychosis that has lasted for 20 years, has a paradigm that encompasses various diseases without the aim of predicting and preventing the development of specific mental illnesses in help-seeking individuals. McGorry and his colleagues have already proposed a pluripotent prospective program that reflects this concept. The CHARM study encompasses schizophrenia, mood disorder, and borderline personality disorder outcomes and implements a clinical staging model along the mental health continuum (57). The CHARM study will also provide predictive and discriminant validity of the proposed criteria 16. Our study, on the other hand, focused on identifying the clinical characteristics in an Asian sample, and further look at predictors for blood biomarkers. Therefore, it is expected that the clinical usefulness of the transdiagnostic approach at an early stage will be tested. The transdiagnostic high-risk concept could be extended to provide a perspective for people with various psychopathological tendencies below a threshold, such that they do not meet the existing diagnostic criteria of mental illnesses, to determine what may lead them to a specific disease and help identify appropriate preventative interventions. Since the pluripotent high-risk group encompasses most mental illnesses, studies with large sample sizes will be needed to avoid false-negative consequences. In addition, further studies of other biomarkers that are receiving attention from psychosis will be needed (58–60). Further research into the pathophysiology leading to a risk for a specific mental illness in the pluripotent risk state and more common treatments such as cognitive behavioral therapy or anti-inflammatory therapy should be undertaken.
The study was designed in accordance with the Declaration of Helsinki, and the protocol was approved by the Institutional Review Board of Seoul National University Hospital (no. 1704-075-846). Written informed consent will be obtained from the participants, or their parents when required.
All authors were responsible for the design of the whole study and wrote the protocol. Author TL wrote the manuscript. All authors supported the manuscript preparation and approved the final manuscript.
This research was supported by the Brain Research Program through the National Research Foundation of Korea (NRF) funded by the Ministry of Science, ICT and Future Planning (Grant Nos. HI17C0870, 2019M3C7A1030625, 2019R1A2B5B03100844).
The authors declare that the research was conducted in the absence of any commercial or financial relationships that could be construed as a potential conflict of interest.
1. Cannon TD, Cadenhead K, Cornblatt B, Woods SW, Addington J, Walker E, et al. Prediction of psychosis in youth at high clinical risk: A multisite longitudinal study in North America. Arch Gen Psychiatry (2008) 65(1):28–37. doi: 10.1001/archgenpsychiatry.2007.3
2. Fusar-Poli P, Borgwardt S, Bechdolf A, Addington J, Riecher-Rössler A, Schultze-Lutter F, et al. The psychosis high-risk state: a comprehensive state-of-the-art review. JAMA Psychiatry (2013) 70(1):107–20. doi: 10.1001/jamapsychiatry.2013.269
3. McGorry PD, Nelson B, Amminger GP, Bechdolf A, Francey SM, Berger G, et al. Intervention in individuals at ultra-high risk for psychosis: A review and future directions. J Clin Psychiatry (2009) 70:1206–12. doi: 10.4088/JCP.08r04472
4. Ruhrmann S, Schultze-Lutter F, Salokangas RK, Heinimaa M, Linszen D, Dingemans P, et al. Prediction of psychosis in adolescents and young adults at high risk: Results from the prospective European prediction of psychosis study. Arch Gen Psychiatry (2010) 67:241–51. doi: 10.1001/archgenpsychiatry.2009.206
5. Fusar-Poli P, Cappucciati M, Rutigliano G, Schultze-Lutter F, Bonoldi I, Borgwardt S, et al. At risk or not at risk? A meta-analysis of the prognostic accuracy of psychometric interviews for psychosis prediction. World Psychiatry: Off J World Psychiatr Assoc (WPA) (2015) 14:322–32. doi: 10.1002/wps.20250
6. Hartmann JA, Yuen HP, McGorry PD, Yung AR, Lin A, Wood SJ, et al. Declining transition rates to psychotic disorder in “ultra-high risk” clients: Investigation of a dilution effect. Schizophr Res (2016) 170(1):130–6. doi: 10.1016/j.schres.2015.11.026
7. Lim KO, Lee TY, Kim M, Chon MW, Yun JY, Kim SN, et al. Early referral and comorbidity as possible causes of the declining transition rate in subjects at clinical high risk for psychosis. Early Intervent Psychiatry (2018) 12:596–604. doi: 10.1111/eip.12363
8. Yung AR, Yuen HP, Berger G, Francey S, Hung TC, Nelson B, et al. Declining transition rate in ultra high risk (prodromal) services: Dilution or reduction of risk? Schizophr Bull (2007) 33:673–81. doi: 10.1093/schbul/sbm015
9. Fusar-Poli P, Nelson B, Valmaggia L, Yung AR, McGuire PK. Comorbid depressive and anxiety disorders in 509 individuals with an at-risk mental state: Impact on psychopathology and transition to psychosis. Schizophr Bull (2014) 40(1):120–31. doi: 10.1093/schbul/sbs136
10. Woods SW, Powers AR 3rd, Taylor JH, Davidson CA, Johannesen JK, Addington J, et al. Lack of diagnostic pluripotentiality in patients at clinical high risk for psychosis: Specificity of comorbidity persistence and search for pluripotential subgroups. Schizophr Bull (2018) 44:254–63. doi: 10.1093/schbul/sbx138
11. Fusar-Poli P. Extending the Benefits of Indicated Prevention to Improve Outcomes of First-Episode Psychosis. JAMA Psychiatry (2017) 74(7):667–8. doi: 10.1001/jamapsychiatry.2017.1009
12. Fusar-Poli P, Schultze-Lutter F, Cappucciati M, Rutigliano G, Bonoldi I, Stahl D, et al. The Dark Side of the Moon: Meta-analytical Impact of Recruitment Strategies on Risk Enrichment in the Clinical High Risk State for Psychosis. Schizophr Bull (2016) 42(3):732–43. doi: 10.1093/schbul/sbv162
13. Lee TY, Lee J, Kim M, Choe E, Kwon JS. Can we predict psychosis outside the clinical high-risk state? A systematic review of non-psychotic risk syndromes for mental disorders. Schizophr Bull (2018) 44:276–85. doi: 10.1093/schbul/sbx173
14. Fusar-Poli P. The Hype Cycle of the Clinical High Risk State for Psychosis: The Need of a Refined Approach. Schizophr Bull (2018) 44(2):250–3. doi: 10.1093/schbul/sbx181
15. Tognin S, van Hell HH, Merritt K, Winter-van Rossum I, Bossong MG, Kempton MJ, et al. Towards Precision Medicine in Psychosis: Benefits and Challenges of Multimodal Multicenter Studies-PSYSCAN: Translating Neuroimaging Findings From Research into Clinical Practice. Schizophr Bull (2019) 46(2):432–41. doi: 10.1093/schbul/sbz067
16. Hartmann JA, Nelson B, Spooner R, Paul Amminger G, Chanen A, Davey CG, et al. Broad Clinical High-Risk Mental State (CHARMS): Methodology of a cohort study validating criteria for pluripotent risk. Early Intervent Psychiatry (2017) 13(3):379–86. doi: 10.1111/eip.12483
17. Hartmann JA, Nelson B, Ratheesh A, Treen D, McGorry PD. At-risk studies and clinical antecedents of psychosis, bipolar disorder and depression: A scoping review in the context of clinical staging. Psychol Med (2019) 49(2):177–89. doi: 10.1017/S0033291718001435
18. McGorry PD, Hartmann JA, Spooner R, Nelson B. Beyond the “at risk mental state” concept: Transitioning to transdiagnostic psychiatry. World Psychiatry: Off J World Psychiatr Assoc (WPA) (2018) 17(2):133–42. doi: 10.1002/wps.20514
19. Fusar-Poli P, De Micheli A, Rocchetti M, Cappucciati M, Ramella-Cravaro V, Rutigliano G, et al. Semistructured Interview for Bipolar at Risk States (SIBARS). Psychiatry Res (2018) 264:302–9. doi: 10.1016/j.psychres.2018.03.074
20. Hafeman DM, Merranko J, Axelson D, Goldstein BI, Goldstein T, Monk K, et al. Toward the definition of a bipolar prodrome: Dimensional predictors of bipolar spectrum disorders in at-risk youths. Am J Psychiatry (2016) 173:695–704. doi: 10.1176/appi.ajp.2015.15040414
21. Van Meter A, Guinart D, Bashir A, Sareen A, Cornblatt BA, Auther A, et al. Bipolar prodrome symptom scale - abbreviated screen for patients: Description and validation. J Affect Disord (2019) 249:357–65. doi: 10.1016/j.jad.2019.02.040
22. Correll CU, Smith CW, Auther AM, McLaughlin D, Shah M, Foley C, et al. Predictors of remission, schizophrenia, and bipolar disorder in adolescents with brief psychotic disorder or psychotic disorder not otherwise specified considered at very high risk for schizophrenia. J Child Adolesc Psychopharmacol (2008) 18:475–90. doi: 10.1089/cap.2007.110
23. Lee TY, Kim SN, Correll CU, Byun MS, Kim E, Jang JH, et al. Symptomatic and functional remission of subjects at clinical high risk for psychosis: A 2-year naturalistic observational study. Schizophr Res (2014) 156:266–71. doi: 10.1016/j.schres.2014.04.002
24. Woods SW, Walsh BC, Addington J, Cadenhead KS, Cannon TD, Cornblatt BA, et al. Current status specifiers for patients at clinical high risk for psychosis. Schizophr Res (2014) 158(1-3):69–75. doi: 10.1016/j.schres.2014.06.022
25. McGorry P, Keshavan M, Goldstone S, Amminger P, Allott K, Berk M, et al. Biomarkers and clinical staging in psychiatry. World Psychiatry: Off J World Psychiatr Assoc (WPA) (2014) 13:211–23. doi: 10.1002/wps.20144
27. Abi-Dargham A, Horga G. The search for imaging biomarkers in psychiatric disorders. Nat Med (2016) 22:1248–55. doi: 10.1038/nm.4190
28. Le-Niculescu H, Kurian SM, Yehyawi N, Dike C, Patel SD, Edenberg HJ, et al. Identifying blood biomarkers for mood disorders using convergent functional genomics. Mol Psychiatry (2009) 14(2):156–74. doi: 10.1038/mp.2008.11
29. Schmidt A, Cappucciati M, Radua J, Rutigliano G, Rocchetti M, Dell'Osso L, et al. Improving prognostic accuracy in subjects at clinical high risk for psychosis: Systematic review of predictive models and meta-analytical sequential testing simulation. Schizophr Bull (2017) 43:375–88. doi: 10.1093/schbul/sbw098
30. Clementz BA, Sweeney JA, Hamm JP, Ivleva EI, Ethridge LE, Pearlson GD, et al. Identification of distinct psychosis biotypes using brain-based biomarkers. Am J Psychiatry (2016) 173:373–84. doi: 10.1176/appi.ajp.2015.14091200
31. Reininghaus U, Bohnke JR, Chavez-Baldini U, Gibbons R, Ivleva E, Clementz BA, et al. Transdiagnostic dimensions of psychosis in the Bipolar-Schizophrenia Network on Intermediate Phenotypes (B-SNIP). World Psychiatry: Off J World Psychiatr Assoc (WPA) (2019) 18(1):67–76. doi: 10.1002/wps.20607
32. Sokolowska I, Wetie AG, Wormwood K, Thome J, Darie CC, Woods AG. The potential of biomarkers in psychiatry: Focus on proteomics. J Neural Transm (2015) 122(Suppl 1):S9–S18. doi: 10.1007/s00702-013-1134-6
33. Taurines R, Dudley E, Grassl J, Warnke A, Gerlach M, Coogan AN, et al. Proteomic research in psychiatry. J Psychopharmacol (2011) 25(2):151–96. doi: 10.1177/0269881109106931
34. Tyers M, Mann M. From genomics to proteomics. Nature (2003) 422(6928):193–7. doi: 10.1038/nature01510
35. Johnston-Wilson NL, Sims CD, Hofmann JP, Anderson L, Shore AD, Torrey EF, et al. Disease-specific alterations in frontal cortex brain proteins in schizophrenia, bipolar disorder, and major depressive disorder. The Stanley Neuropathology Consortium. Mol Psychiatry (2000) 5(2):142–9. doi: 10.1038/sj.mp.4000696
36. Gadad BS, Jha MK, Grannemann BD, Mayes TL, Trivedi MH. Proteomics profiling reveals inflammatory biomarkers of antidepressant treatment response: Findings from the CO-MED trial. J Psychiatr Res (2017) 94:1–6. doi: 10.1016/j.jpsychires.2017.05.012
37. Iniesta R, Stahl D, McGuffin P. Machine learning, statistical learning and the future of biological research in psychiatry. Psychol Med (2016) 46(12):2455–65. doi: 10.1017/S0033291716001367
38. Lualdi M, Fasano M. Statistical analysis of proteomics data: A review on feature selection. J Proteomics (2019) 198:18–26. doi: 10.1016/j.jprot.2018.12.004
39. Miller TJ, McGlashan TH, Rosen JL, Somjee L, Markovich PJ, Stein K, et al. Prospective diagnosis of the initial prodrome for schizophrenia based on the structured interview for prodromal syndromes: Preliminary evidence of interrater reliability and predictive validity. Am J Psychiatry (2002) 159:863–5. doi: 10.1176/appi.ajp.159.5.863
40. Correll CU, Olvet DM, Auther AM, Hauser M, Kishimoto T, Carrion RE, et al. The Bipolar Prodrome Symptom Interview and Scale-Prospective (BPSS-P): Description and validation in a psychiatric sample and healthy controls. Bipolar Disord (2014) 16:505–22. doi: 10.1111/bdi.12209
41. Hamilton M. A rating scale for depression. J Neurol Neurosurg Psychiatry (1960) 23:56–62. doi: 10.1136/jnnp.23.1.56
42. Hamilton M. The assessment of anxiety states by rating. Br J Med Psychol (1959) 32(1):50–5. doi: 10.1111/j.2044-8341.1959.tb00467.x
43. Young RC, Biggs JT, Ziegler VE, Meyer DA. A rating scale for mania: Reliability, validity and sensitivity. Br J Psychiatry (1978) 133:429–35. doi: 10.1192/bjp.133.5.429
44. Cho CH, Jung SY, Kapczinski F, Rosa AR, Lee HJ. Validation of the Korean version of the biological rhythms interview of assessment in neuropsychiatry. Psychiatry Invest (2018) 15:1115–20. doi: 10.30773/pi.2018.10.21.1
45. Hall RC. Global assessment of functioning. A modified scale. Psychosomatics (1995) 36:267–75. doi: 10.1016/S0033-3182(95)71666-8
46. Cornblatt BA, Auther AM, Niendam T, Smith CW, Zinberg J, Bearden CE, et al. Preliminary findings for two new measures of social and role functioning in the prodromal phase of schizophrenia. Schizophr Bull (2007) 33:688–702. doi: 10.1093/schbul/sbm029
47. Lee S-R, Lee W-H, Park J-S, Kim S-M, Kim J-W, Shim J-H. The study on reliability and validity of korean version of the barratt impulsiveness scale-11-revised in nonclinical adult subjects. J Korean Neuropsychiatr Assoc (2012) 51:378–86. doi: 10.4306/jknpa.2012.51.6.378
48. Kim D, Park SC, Yang H, Oh DH. Reliability and validity of the Korean version of the childhood trauma questionnaire-short form for psychiatric outpatients. Psychiatry Invest (2011) 8:305–11. doi: 10.4306/pi.2011.8.4.305
49. Lee JH, Kim SJ, Lee SY, Jang KH, Kim IS, Duffy JF. Reliability and validity of the Korean version of morningness-eveningness questionnaire in adults aged 20-39 years. Chronobiol Int (2014) 31:479–86. doi: 10.3109/07420528.2013.867864
50. Min SK, Kim KI, Lee CI, Jung YC, Suh SY, Kim DK. Development of the Korean versions of WHO quality of life scale and WHOQOL-BREF. Qual Life Res (2002) 11:593–600. doi: 10.1023/A:1016351406336
51. Baek HS, Lee KU, Joo EJ, Lee MY, Choi KS. Reliability and validity of the korean version of the connor-davidson resilience scale. Psychiatry Invest (2010) 7(2):109–15. doi: 10.4306/pi.2010.7.2.109
52. Jang YE, Lee TY, Hur JW, Kwon JS. Validation of the Korean version of the prodromal questionnaire-brief version in non-help-seeking individuals. Psychiatry Invest (2019) 16(2):109–14. doi: 10.30773/pi.2018.10.23
53. Rush AJ, Trivedi MH, Ibrahim HM, Carmody TJ, Arnow B, Klein DN, et al. The 16-item Quick Inventory of Depressive Symptomatology (QIDS), clinician rating (QIDS-C), and self-report (QIDS-SR): A psychometric evaluation in patients with chronic major depression. Biol Psychiatry (2003) 54:573–83. doi: 10.1016/S0006-3223(02)01866-8
54. Baek JH, Kim JS, Huh I, Lee K, Park JH, Park T, et al. Prevalence, behavioral manifestations and associated individual and climatic factors of seasonality in the Korean general population. Compr Psychiatry (2015) 57:148–54. doi: 10.1016/j.comppsych.2014.10.014
55. Lee Y-J, Bhang S-Y, Choi S, Lee H-K, Kim B-W, Kim W, et al. Korean state-trait anxiety inventory application study in middle and high school students. J Korean Neuropsychiatr Assoc (2008) 47:471–80.
56. Kennedy JJ, Abbatiello SE, Kim K, Yan P, Whiteaker JR, Lin C, et al. Demonstrating the feasibility of large-scale development of standardized assays to quantify human proteins. Nat Methods (2014) 11(2):149–55. doi: 10.1038/nmeth.2763
57. McGorry PD, Mei C. Ultra-high-risk paradigm: Lessons learnt and new directions. Evidence-Based Ment Health (2018) 21(4):131–3. doi: 10.1136/ebmental-2018-300061
58. Bodatsch MA, Brockhaus-Dumke J, Klosterkotter J, Ruhrmann S. Forecasting psychosis by event-related potentials-systematic review and specific meta-analysis. Biol Psychiatry (2015) 77((11)):951–8. doi: 10.1016/j.biopsych.2014.09.025
59. Ebisch SJ, Mantini D, Northoff G, Salone A, De Berardis D, Ferri F, et al. Altered brain long-range functional interactions underlying the link between aberrant self-experience and self-other relationship in first-episode schizophrenia. Schizophr Bull (2014) 40((5)):1072–82. doi: 10.1093/schbul/sbt153
Keywords: bipolar disorder, high-risk for mental illness, major depressive disorder, pluripotential, proteomics, schizophrenia, transdiagnostic
Citation: Lee TY, Lee J, Lee HJ, Lee Y, Rhee SJ, Park DY, Paek MJ, Kim EY, Kim E, Roh S, Jung HY, Kim M, Kim SH, Han D, Ahn YM, Ha K and Kwon JS (2020) Study Protocol for a Prospective Longitudinal Cohort Study to Identify Proteomic Predictors of Pluripotent Risk for Mental Illness: The Seoul Pluripotent Risk for Mental Illness Study. Front. Psychiatry 11:340. doi: 10.3389/fpsyt.2020.00340
Received: 18 November 2019; Accepted: 03 April 2020;
Published: 21 April 2020.
Edited by:
Shaohua Hu, Zhejiang University, ChinaReviewed by:
Massimo Tusconi, University of Cagliari, ItalyCopyright © 2020 Lee, Lee, Lee, Lee, Rhee, Park, Paek, Kim, Kim, Roh, Jung, Kim, Kim, Han, Ahn, Ha and Kwon. This is an open-access article distributed under the terms of the Creative Commons Attribution License (CC BY). The use, distribution or reproduction in other forums is permitted, provided the original author(s) and the copyright owner(s) are credited and that the original publication in this journal is cited, in accordance with accepted academic practice. No use, distribution or reproduction is permitted which does not comply with these terms.
*Correspondence: Kyooseob Ha, a3lvb2hhQHNudS5hYy5rcg==
Disclaimer: All claims expressed in this article are solely those of the authors and do not necessarily represent those of their affiliated organizations, or those of the publisher, the editors and the reviewers. Any product that may be evaluated in this article or claim that may be made by its manufacturer is not guaranteed or endorsed by the publisher.
Research integrity at Frontiers
Learn more about the work of our research integrity team to safeguard the quality of each article we publish.