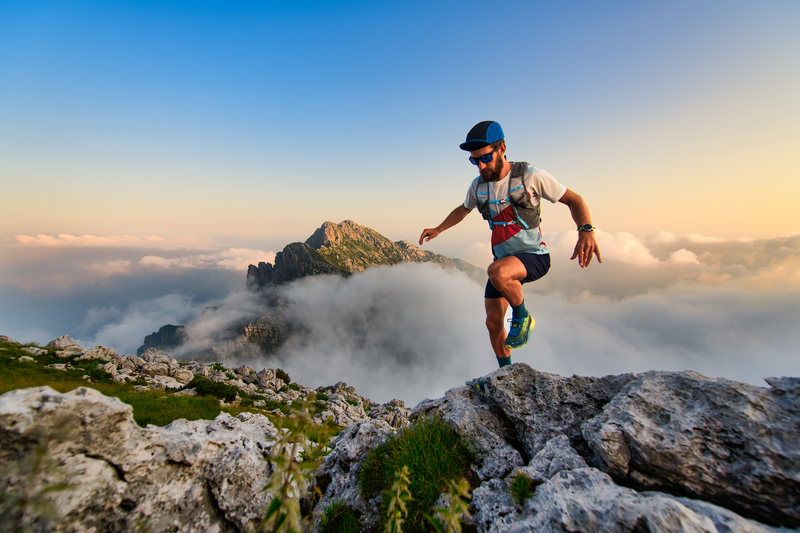
94% of researchers rate our articles as excellent or good
Learn more about the work of our research integrity team to safeguard the quality of each article we publish.
Find out more
ORIGINAL RESEARCH article
Front. Psychiatry , 18 March 2020
Sec. Molecular Psychiatry
Volume 11 - 2020 | https://doi.org/10.3389/fpsyt.2020.00151
Major depressive disorder (MDD) is a serious mental disease with negative effects on both mental and physical health of the patient. Currently, antidepressants are among the major ways to ease or treat MDD. However, the existing antidepressants have limited efficacy in treating MDD, with a large fraction of patients either responding inadequately or differently to antidepressants during the treatment. Pharmacogenetics studies have found that the genetic features of some genes are associated with the antidepressant efficacy. In order to obtain a better understanding on the relationship between the genetic factors and antidepressant treatment response, we compiled a list of 233 single-nucleotide polymorphisms (SNPs) significantly associated with the antidepressant efficacy in treating MDD. Of the 13 non-synonymous SNPs in the list, three (rs1065852, rs3810651, and rs117986340) may influence the structures and function of the corresponding proteins. Besides, the influence of rs1065852 on the structure of CYP2D6 was further investigated via molecular dynamics simulations. Our results showed that compared to the native CYP2D6 the flexibility of the F-G loop was reduced in the mutant. As a portion of the substrate access channel, the lower flexibility of F-G loop may reduce the ability of the substrates to enter the channel, which may be the reason for the lower enzyme activity of mutant. This study may help us to understand the impact of genetic variation on antidepressant efficacy and provide clues for developing new antidepressants.
Major depressive disorder (MDD) is a serious mental disorder that influences ~6% population worldwide (1, 2). The lifetime incidence of depression is ~16.6% (3, 4), and the rate for females is twice that of males (5). The symptoms of MDD are complicated, including anxiety, cognitive impairment, suicidal tendencies, and emotional, somatic, and functional impairments (6). The disease can negatively affect almost all aspects of a person, including personal life, work life, and education, as well as general health. It does not only severely limit the psychosocial functioning and deteriorate life quality of the patients, but also brings heavy spiritual and economic burden to their families and the society (7). Actually, MDD is among the most burdensome disease worldwide (8, 9), both in the developed and developing countries (1, 10, 11), and the World Health Organization predicted that MDD will become the major cause of years lost to disability in 2030 (12). Thus, it is still a huge challenge to develop more effective therapeutic approaches that can accurately diagnose and treat MDD.
Currently, antidepressants are among the major ways to treat or alleviate MDD. The available antidepressants can be classified into several types according to their structure and the way they work, including selective serotonin reuptake inhibitors (SSRIs), monoamine oxidase inhibitors, serotonin and norepinephrine reuptake inhibitors, tricyclic antidepressants, and the other antidepressants. However, studies have shown that the existing antidepressants have limited efficacy in treating MDD, and the response of patients to antidepressants is difficult to predict (13). For example, it is found that the common antidepressants are effective in approximately only 40% of MDD patients (14), and 30–50% of patients have no response to the initial treatment of antidepressants (14). Even if a patient responds to an antidepressant, the recurrence rate is usually high (15).
The biological mechanisms of antidepressant response are still unclear, but earlier studies indicate that genetic factors may play an important part in this procedure (16–18). Pharmacogenetic studies show that the genetic characteristics of an individual can make an antidepressant more or less effective, and the single-nucleotide polymorphisms (SNPs) of some genes are related to the drug efficacy (19). Genes affecting antidepressant efficacy can be roughly grouped into two major categories, that is, genes of cytochrome P450 (CYP) family and those involved in the serotonin biochemical pathway (20). Members of the CYP family, for example, CYP2D6, CYP1A2, CYP3A4, and CYP2C19, have been found to play important roles in the metabolism of antidepressants (19, 21–23). Because an SNP may lead to abolished, reduced, or increased enzyme activity, some members of CYP families, such as CYP2D6, may have four phenotypes in individuals, including normal metabolizers, intermediate metabolizers, poor metabolizers, and ultrarapid metabolizers (24). Hence, there is a connection between the CYP2D6 polymorphisms and the plasma levels of antidepressants; and MDD patients need a dose of antidepressant appropriate to their genetic characteristics to achieve better efficacy and fewer side effects. For genes in the serotonin biochemical pathway, such as the serotonin transporter gene (SLC6A4) and 5-hydroxytryptamine receptor 2A (HTR2A), genotypes are also associated with the antidepressant efficacy (19).
Until now, the association between genotype and the efficacy of antidepressants has been detected in a number of studies (25–27). For example, it is found that rs6265 in brain-derived neurotrophic factor (BDNF) is significantly associated with response to antidepressants in MDD (28–31). Some studies show that the SNPs in 5-hydroxytryptamine receptor 1B (HTR1B), HTR1A, and HTR2A are significantly associated with efficacy of antidepressants (32–34). Several genome-wide association studies (GWASs) have also been performed to detect the association between SNPs and antidepressant efficacy, such as the Pharmacogenomic Research Network Antidepressant Medication Pharmacogenomic Study (PGRN-AMPS) (35), the International SSRI Pharmacogenomics Consortium (ISPC) (36), the Sequenced Treatment Alternatives to Relieve Depression (STAR*D) study (37), the Genome-Based Therapeutic Drugs for Depression (GENDEP) project in whole sample (38), and the Munich Antidepressant Response Signature (MARS) (39). Although none of these studies reported the results that achieved the genome-wide significance threshold (40), a meta-analysis based on the data of PGRN-AMPS, ISPC, and STAR*D study identified an SNP (rs2456568) on hypoxanthine phosphoribosyltransferase pseudogene 4 (HPRTP4) at the genome-wide significance level (36). In another GWAS of antidepressant response in Koreans, two significant SNPs (rs7785360 and rs12698828) on autism susceptibility candidate 2 (AUTS2) were found to be related to antidepressant response (41).
Although a number of SNPs have been found to be significantly associated with antidepressant efficacy in treating MDD, it is still unclear how these SNPs affect the interaction between proteins and the drugs, as well as the response of antidepressants. Hence, exploring the correlation between the SNPs and the structures of the corresponding proteins may provide valuable information to understand the molecular mechanism underlying response to antidepressants and help us to develop novel antidepressants. In this article, we implemented a comprehensive curation of SNPs related with antidepressant efficacy from genetic studies and identified the non-synonymous SNPs (nsSNPs) potentially influencing the biological function and structure of the proteins.
First, SNPs associated with antidepressant efficacy were collected. The Pharmacogenomics Knowledgebase (PharmGKB, https://www.pharmgkb.org/), a comprehensive resource for clinical information, gene-drug associations, and genotype-phenotype relationships (42), was queried with different keywords. For terms “antidepressants and depressive disorder, major,” “antidepressants and depressive disorder,” and “antidepressants and depression,” 307, 108, and 193 publications were retrieved respectively. In addition, another list of 245 publications related to other antidepressants was retrieved, among which the contents of 38 publications were closely related to depression. In addition, 225 publications were retrieved from PubMed (https://www.ncbi.nlm.nih.gov/pubmed/) via the query term “(Depressive Disorder, Major [Mesh]) AND (Antidepressive Agents [Mesh]) AND (Polymorphism, Single Nucleotide [Mesh] OR Pharmacogenetics [Mesh]).” For these publications, the redundant ones were removed. Then, the abstracts of the remaining publications were reviewed, and only studies related to antidepressant response were selected. Most of these studies were GWASs, meta-analysis, and candidate gene analysis of antidepressant responses. In each case, we selected only the SNPs that were significantly associated with the response of antidepressants in MDD. To reduce false-positive findings, the publications reporting negative or insignificant correlations were excluded, although some SNPs explored in these studies might be really associated with antidepressant responses. The full texts of the selected publications were examined to ensure the conclusions were consistent with the content. We narrowed our selection by focusing on those purporting one or more SNPs significantly associated with antidepressant responses. Finally, we retrieved 117 publications (Figure 1).
Figure 1. PRISMA flow diagram illustrating search strategy and studies included in the analysis. PRISMA, Preferred Reporting Items for Systematic Reviews and Meta-Analyses (http://www.prisma-statement.org/).
We estimated the influence of amino acid substitution on protein function by several tools, including SIFT (https://sift.bii.a-star.edu.sg/index.html), PolyPhen-2 (http://genetics.bwh.harvard.edu/pph2/), and SNAP2 (https://rostlab.org/services/snap2web/). SIFT is a tool to predict the change of protein function caused by single amino acid mutation based on homologous protein sequence and the physical properties of amino acids (43). The output of the tool is a tolerance index score measuring whether an amino acid substitution in a protein is tolerated or damaging, and an SNP with tolerance index score < 0.05 is defined to be deleterious. PolyPhen-2 predicts the effects of human nsSNPs on protein structure and function based on the features of sequence and structural information (44). The output of PolyPhen-2 is a score ranging from 0 to 1, with larger scores indicating higher likelihood of being damaging. SNAP2 is used to identify the potential effect of mutations by analyzing various sequence and variant features via the artificial neural network. It measures each substitution with a score ranging from −100 (strongly predicted “neutral”) to +100 (strongly predicted “effect”) (45). SNAP2 is used to differentiate between effect and neutral nsSNPs by thinking about the various sequence and variant features (45). And the score of each substitution ranges from −100 (strongly predicted “neutral”) to +100 (strongly predicted “effect”).
The mutant protein stability changes were analyzed by I-MUTANT 3.0 (http://gpcr2.biocomp.unibo.it/cgi/predictors/I-Mutant3.0/I-Mutant3.0.cgi). I-MUTANT can detect the changes of protein stability according to single amino acid substitution (46).
Because an evolutionary conservative an amino acid may be closely related to the protein structure and function, we assessed the conservation of amino acids in a given protein to identify the amino acids whose substitutions may be important for the molecule. The ConSurf (http://consurf.tau.ac.il/2016/) was utilized to evaluate the evolutionary conservation of amino acid positions in protein based on the phylogenetic relationships between homologous protein sequences (47).
For purpose of detecting the influence of the most deleterious nsSNP on structure, we performed molecular modeling and molecular dynamics (MD) simulation for the native and mutant proteins, respectively. We selected the most closely related protein structure from PDB (Protein Data Bank, https://www.rcsb.org). Then, the structure of mutant protein was constructed based on the structure of native protein by SWISS-MODEL (https://swissmodel.expasy.org) (48). Finally, energy minimization and MD simulations were implemented on the native and mutant protein to investigate the structural deviation and fluctuation due to deleterious nsSNPs. All the analyses were implemented by using GROMACS 5.1.4 package (49).
We used the AMBER99SB-ILDN force field in both native and mutant model systems (50). The proteins being simulated were at the center of the cubic box. Then, we filled the box with the three-site transferrable intermolecular potential water solvent model and added chlorine ions to the system to neutralize counter-ions. The cutoff for short-range electrostatic and van der Waals interactions was selected as 1.4 nm. In the analysis, we first performed the energy minimizations for the initial structures by using the algorithm of the steepest descent minimization. Next, we conducted the equilibration via a two-stage procedure, that is, an NVT (N, constant number; V, volume; and T, temperature) ensemble was first conducted for 1.0 ns and stabilized the temperature of the system at 300 K; then, an NPT (N, constant number; P, pressure; and T, temperature) ensemble was conducted for 5.0 ns to ensure the system was well-equilibrated with respect to pressure and density. After the system is well-equilibrated at the expected temperature and pressure, we performed the MD simulations for 200 ns to explore the structural deviation and fluctuation of native and mutant protein due to deleterious nsSNPs. Finally, in order to evaluate the convergence of the MD simulations, we calculated the root-mean-square deviation (RMSD). Furthermore, root-mean-square fluctuation (RMSF) was calculated to assess the differences in structural flexibility between the native and mutant proteins. The RMSD and RMSF were obtained from the trajectory files of the MD results, which were produced after the MD simulation.
From the available studies, we collected 233 SNPs significantly associated with the antidepressant response (Supplementary Table 1). Of these SNPs, 13 were nsSNPs (missense), and 21 were synonymous SNPs in coding region. In the non-coding region, 133 SNPs were intron variant (intronic), and 7 and 2 SNPs were in 3′ UTR and 5′ UTR, respectively (Figure 2). These SNPs were mapped to 110 genes. Among them there were four serotonin receptors, that is, HTR1A, HTR1B, HTR2A, and HTR7; one dopamine receptor, that is, DRD3; two corticotropin-releasing hormone receptors, that is, CRHR1 and CRHR2; two glutamate receptors, that is, GRIK2 and GRIK4; two serotonin biosynthesis genes, that is, TPH1 and TPH2; and some genes encoding transporters, that is, SLC6A1, SLC6A2, SLC6A3, SLC6A4, SLC39A14, and ABCA1. In addition, some genes were related to drug transport, that is, CACNA1A, DTNBP1, GDNF, CRH, SLC6A2, SLC6A3, GAL, SNCA, and CNR1; some genes were involved in the exogenous drug catabolic process, that is, CYP1A2, CYP2C19, and CYP2D6; and some genes were related to response to drug (COMT, SRP19, NCAM1, NR3C1, CREB1, CRH, CRHBP, CRHR1, BDNF, GSK3B, ITPR2, ARRB2, SERPINE1, RGS17, MAPK1, SNCA, and IL1B). The functional diversity of these genes clearly indicated the complexity of the mechanisms for antidepressant response. In addition, ABCB1, TPH2, ANO2, ZNF385D, and CYP1A2 appeared more frequently in these genes. For these SNPs, most were located in the non-coding regions. Currently, it still remains a significant challenge to explain how SNPs located in intronic or intergenic regions affect drug response. In addition, because synonymous SNPs in the coding region do not change the protein sequence, it is difficult to experimentally address the function of every mutation seen in these regions. Therefore, we focused only on the nsSNPs in the coding region in the following analyses (Table 1).
To explore the potential influence of amino acid substitutions on the function of protein caused by nsSNPs, we analyzed the 13 nsSNPs via SIFT, PolyPhen, and SNAP2. Of these nsSNPs, each tool predicted 5 as deleterious (Table 2), among which 3 nsSNPs (rs1065852, rs3810651, and rs117986340) were commonly identified by SIFT, PolyPhen-2, and SNAP2, which were selected for further investigation. Then, we performed I-MUTANT analysis on the three nsSNPs (Table 3). Because the DDG values for all these SNPs were negative (i.e., smaller than 0 kcal/mol), the amino acid substitutions might decrease the stability of the corresponding proteins.
As we know, the functional important domains of protein usually are highly conserved, which means if an nsSNP is located in conservative domains, it may potentially be important for maintaining the protein structure and function. The conservation of the three deleterious nsSNPs was analyzed using ConSurf. In this study, we performed ConSurf analysis through PSI-BLAST algorithm with default parameters to collect the homologous sequences in UniRef90 protein databases (47) (Table 4). The conservation scores of I478F (rs3810651) and G999C (rs117986340) were 1 and 3, respectively, revealing they were variable. But the conservation score of P34S (rs1065852) was 9, which indicated that the rs1065852 located in a conserved region and may be important for maintaining protein function.
The mutation of a proline (P) into a serine (S) occurred at position 34 due to deleterious nsSNPs rs1065852 (Figure 3). The difference (amino acid properties, structure) of proline (P) and serine (S) may lead to structural deviation and fluctuation, which may cause the functional deviations between the native and mutant CYP2D6. Thus, we implemented MD simulations for the native and mutant CYP2D6 structures to determine the reason of the structural difference.
Figure 3. Schematic structures of the native (left) and mutant (right) amino acid. The backbone colored red and the same for each amino acid, and side chain unique for each amino acid is colored black; the mutation of proline into serine at position 34 for rs1065852.
The native structure of CYP2D6 was obtained from PDB (PDB ID: 3QM4) (51). Then, we deleted the prinomastat and waters from 3QM4 to construct the initial structure. Then, using 3QM4 as template, the initial structure of mutant was constructed by SWISS-MODEL. After the systems reached being well-equilibrated, 200-ns MD simulations were implemented for the initial structures of native and mutant CYP2D6.
We analyzed the RMSDs for backbone of the native and mutant CYP2D6 to evaluate the convergence of the systems. Root-mean-square deviation indicates the average change in displacement of the selected atoms for a particular frame in respect of a reference coordinate system. The initial structures were used as the references of RMSDs. Figure 4 shows that the simulations of native and mutant systems were both converged. In the last 20 ns of simulation, the average RMSD of native structure was 0.228 nm, whereas that of mutant structure was 0.219 nm. In both structures, the structural deviations were observed during simulations. In addition, the fluctuations of RMSDs were smaller at the end of the simulations, which indicated that the simulations generated stable trajectories. Therefore, the results could be used for further analysis.
For purpose of detecting the changes of flexibility between the native and mutant CYP2D6 structures, we calculated the RMSFs. The results are shown in Figure 5. For the RMSFs of native structure, the highest peak was observed near the 230th residue, that is, F-G loop in 3QM4, which indicated that this portion of the native CYP2D6 was flexible. While the RMSFs of mutant in this portion were lower, the flexibility of the F-G loop is reduced compared to the native CYP2D6. In addition, the entrance of the substrate access channel of CYP2D6 is made up of F-G loop, B-C loop, and the loop in the β1 sheet and β2 sheet (Figure 6). The CYP2D6 structural flexibility contributes to its catalytic versatility. As a constituent portion of the substrate access channel, the lower flexibility of F-G loop may affect the substrates that enter into the channel entrance. Hence, this may be the reason for the low enzyme activity of mutant.
Although a number of SNPs are found to be significantly associated with the response of antidepressants in MDD, it is still unclear how they affect the interaction between genes carrying these SNPs and antidepressants. In this study, we collected the SNPs significantly associated with the response of antidepressants and explored the potential mechanism underlying the different antidepressant efficacy among MDD patients. Most of the SNPs were included in the intronic or intergenic regions. For the 13 nsSNPs, three of them (rs1065852, rs3810651, and rs117986340) were predicted to be deleterious to the corresponding proteins. Evolutionary conservation analysis showed that P34S (rs1065852) was the only one located in the conserved protein domain, indicating it may be important for maintaining protein function. Subsequently, the results of the MD simulations revealed that the flexibility of the F-G loop for mutant was reduced compared to the native CYP2D6. As a constituent portion of the substrate access channel, this may affect the substrates that enter into the channel entrance. Hence, this may be the reason for the low enzyme activity of mutant.
In humans, there are ~60 CYP genes (52). The CYP enzymes are related to the synthesis and metabolism of various molecules and chemicals. As a member of the CYPs superfamily, CYP2D6 plays an important role in drug metabolism. It is responsible for the removal of at least 20% of the compounds, including antidepressants (53). Earlier studies have found the polymorphisms of CYP2D6 can cause the differences for antidepressant response among MDD patients, among which include CYP2D6 P34S (rs1065852). However, our understanding on the effect of the polymorphisms in CYP2D6 on the antidepressant efficacy is limited (54), and available studies have found mixed results. Some studies reported negative findings (55–57). For instance, Hodgson et al. (56) analyzed the data from GENDEP and found there was no significant association between CYP2D6 polymorphisms and response to antidepressants. In contrast, some studies reported positive associations between CYP2D6 polymorphisms and response to antidepressants (58–61). For example, Zastrozhin et al. (59) found that patients with 1846G>A of CYP2D6 polymorphism (rs3892097) had significantly reduced efficacy of fluvoxamine. For the P34S (rs1065852) substitution in CYP2D6. Tsai et al. (60) suggested that CYP2D6 polymorphisms (CYP2D6*4,CYP2D6*5, and CYP2D6*10) may be predicting patient treatment outcomes. In our study, we obtained the results of Han et al. (61), which found the P amino acid of the P34S (rs1065852) substitution in CYP2D6 is a favorable factor in the treatment of escitalopram for MDD and that the P34S (rs1065852) substitution may be a good genetic marker for predicting the treatment outcomes of escitalopram.
The results of the MD simulations indicated that the flexibility of the F-G loop for mutant was reduced compared to the native CYP2D6. The P34S(rs1065852) substitution is located in a highly conserved region encoding the proline-rich N-terminus in the three-dimensional structure of CYP2D6 (51), which was consistent with the results of our previous evolutionary conservation analysis. In addition, we analyzed the difference between the native and mutant amino acids in terms of amino acid properties, structure, and domains by HOPE (http://www.cmbi.ru.nl/hope/), which is a tool that analyzes the structural effects of a point mutation in protein sequence (62). For amino acid properties, the serine is smaller than the native residue proline at position 34, which may cause a loss of external interactions (62). And the native residue is more hydrophobic than the mutant residue (62), which might cause the damage of hydrophobic interactions with other molecules on the surface of CYP2D6. From the comparison of the structures of mutant and original amino acids, prolines are very rigid and produce a particular backbone conformation, which might be needed at this position. The amino acid substitution can disturb this particular conformation. For domain, the mutant residue may affect the domain that is important for the activity of CYP2D6 (62). Therefore, structural perturbation of this region may cause the change of protein stability and enzyme activity. Research showed that the P34S (rs1065852) affected the hydrogen bonding network in the interdomain between of the N-terminal and the F-G loop (63, 64). The results indicated that the hydrogen bonds located in the interdomain between the N-terminal and the F-G loop in mutant were fewer than in native CYP2D6. And the hydrophobic effect may alter the structure of F-G loop. Hence, the change of N-terminal loop due to the mutant P34S (rs1065852) influenced the hydrogen bonding network and ultimately may alter the structure of F-G loop. The mutation might disturb signal transfer from N-terminal to the distant protein from the mutated position through hydrophobic effect (64) and influence the structure of the distant residues from the mutant position. Other studies also reported the activity of mutant CYP2D6 has significantly reduced in vivo (65–67). The results of an early study suggested that the mutant of P34S affected the enzymatic activities (68). Kim et al. (69) indicated that the loss of functions in CYP2D6 alleles can be attributed to the P34S(rs1065852) substitution. These reports are consistent with our findings. The findings may facilitate us to understand why the P amino acid of the P34S (rs1065852) substitution in CYP2D6 is a favorable factor than S amino acid in the treatment of escitalopram for MDD and provide theoretical basis for the development of new antidepressants and personalized medicine in MDD.
Although rs3810651 and rs117986340 are not located in highly conserved regions, their involvements in the antidepressant efficacy are clear. SNP rs3810651 (I478F) is located in the coding region of γ-aminobutyric acid type A receptor, subunit theta (GABRQ), whose genotype AA + AT (F) is significantly associated with increased response to venlafaxine in people with MDD as compared to genotype TT(I) (70). GABRQ is the site of action of a number of important pharmacologic agents (71). The mutated residue phenylalanine is bigger than the original residue isoleucine at position 478 for rs3810651, and the phenylalanine is located in a domain that is important for the activity of GABRQ. Therefore, the mutation might have influence on the activity of the extracellular ligand-gated ion channel (62). The SNP rs117986340 (G999C)is located on lysine methyltransferase 2E (KMT2E), whose genotype GG(G) is significantly associated with increased response to duloxetine in people with MDD as compared to genotype GT(C) (72). The original residue glycine at position 999 is smaller and more hydrophilic than the mutated residue cysteine, which might be necessary for the function of KMT2E. In view of structure, glycine is more flexible to generate the torsion angles compared with the mutant residue; the mutation will result in an inaccurate conformation and disturb the local structure (62).
Besides, of the 13 nsSNPs, several other SNPs are also frequently studied. It is found that rs6314 genotype AG is associated with improved response when treated with paroxetine in people with MDD as compared to genotypes AA + GG (73, 74). The HTR2A carrying rs6314 is related to postsynaptic serotonin signaling and is a target for many antidepressants (75). HTR2A involves the serotonin synthesis, release, reuptake, and mediation of SSRIs in human brain. Therefore, the polymorphisms of HTR2A are associated with the response of antidepressants in MDD (34, 76, 77). The variation may be associated with antidepressant response by affecting serotonin signaling cascades (73). The rs6314 is an SNP in the third exon of HTR2A gene and leads to the change between histidine (His) and tyrosine (Tyr) at position 452. In addition, rs6311, rs17288723, rs7997012, rs9316233, rs6313, and rs2770296 of this gene are also significantly associated with antidepressant efficacy in MDD. This may result in the changes in protein structure and affect the interaction between HTR2A and antidepressants. In addition, several earlier studies found that rs6265 genotypes in BDNF were significantly associated with the response to antidepressants in MDD (29–31). BDNF is related to the survival of existing neurons, as well as the development and differentiation of new neurons and synapses in the central nervous system (78). The rs6265(Val66Met) locates within the signal peptide; it may be important for the maturation of the protein (62). Mutation may cause the change in structure and function of BDNF and ultimately result in the difference of antidepressant efficacy in individuals.
Undeniably, there are some limitations in the current study. First, our results depend on the retrieved existing studies that purported the SNPs associated with the antidepressant efficacy. Given that identification of such genetic factors is an ongoing process, more SNPs and genes may be identified in the future. Second, because of the difference in methods and sample size of the available studies, it is possible that some SNPs with nominal or moderate association with the antidepressant efficacy are not included in our analysis, and some SNPs collected might be false positive; hence, in order to obtain a more comprehensive SNPs list and validate the results, further investigation with a larger sample size or different technical approaches is needed. Finally, although we combined different approaches to identify the deleterious nsSNPs that may influence the structure and function of proteins, some may still be missed. With the SNP data and analysis approaches becoming more comprehensive and accurate, these problems would be avoided.
In this study, we conducted a computational analysis on the SNPs associated with antidepressant efficacy. From 233 SNPs collected from available human pharmacogenetic studies, we screened 13 nsSNPs and found three of them (i.e., rs1065852, rs3810651, and rs117986340) were likely to be deleterious in the encoding proteins, particularly P34S (rs1065852) in CYP2D6. Results of the subsequent MD simulation regarding rs1065852 indicated that the flexibility of the F-G loop for mutant was reduced compared to the native CYP2D6, which may be responsible for the decrease in enzyme activity by hindering the substrate recognition and access. In addition, our results may provide theoretical basis for the development of new antidepressants and personalized medicine in MDD.
All datasets generated for this study are included in the article/Supplementary Material.
JX, MY, YP, and JW: conceived, designed, and performed the experiments and wrote the paper. JX, MY, and YP: analyzed the data.
This study was supported in part by grants from National Key Research and Development Program of China (No. 2016YFC0906300), National Natural Science Foundation of China (Nos. 31271411 and 91746205). The funding bodies played no role in the design of the study and collection, analysis and interpretation of data, or in writing of the manuscript.
The authors declare that the research was conducted in the absence of any commercial or financial relationships that could be construed as a potential conflict of interest.
The Supplementary Material for this article can be found online at: https://www.frontiersin.org/articles/10.3389/fpsyt.2020.00151/full#supplementary-material
1. Kessler RC, Bromet EJ. The epidemiology of depression across cultures. Annu Rev Public Health. (2013) 34:119–38. doi: 10.1146/annurev-publhealth-031912-114409
3. Dunn EC, Brown RC, Dai Y, Rosand J, Nugent NR, Amstadter AB, et al. Genetic determinants of depression: recent findings and future directions. Harv Rev Psychiatr. (2015) 23:1–18. doi: 10.1097/HRP.0000000000000054
4. Singh AB, Bousman CA, Ng C, Berk M. Antidepressant pharmacogenetics. Curr Opin Psychiatr. (2014) 27:43–51. doi: 10.1097/YCO.0000000000000023
5. Muglia P, Tozzi F, Galwey NW, Francks C, Upmanyu R, Kong XQ, et al. Genome-wide association study of recurrent major depressive disorder in two European case-control cohorts. Mol Psychiatry. (2010) 15:589–601. doi: 10.1038/mp.2008.131
6. Sheehan DV, Nakagome K, Asami Y, Pappadopulos EA, Boucher M. Restoring function in major depressive disorder: a systematic review. J Affect Disord. (2017) 215:299–313. doi: 10.1016/j.jad.2017.02.029
7. Wakefield JC, Schmitz MF, First MB, Horwitz AV. Extending the bereavement exclusion for major depression to other losses: evidence from the National Comorbidity Survey. Arch Gen Psychiatr. (2007) 64:433–440. doi: 10.1001/archpsyc.64.4.433
8. Ustün TB, Ayuso-Mateos JL, Chatterji S, Mathers C, Murray CJ. Global burden of depressive disorders in the year (2000). Br J Psychiatr. (2004) 184:386–92. doi: 10.1192/bjp.184.5.386
9. Bruffaerts R, Vilagut G, Demyttenaere K, Alonso J, Alhamzawi A, Andrade LH, et al. Role of common mental and physical disorders in partial disability around the world. Br J Psychiatr. (2012) 200:454–61. doi: 10.1192/bjp.bp.111.097519
10. Greenberg PE, Fournier AA, Sisitsky T, Pike CT, Kessler RC. The economic burden of adults with major depressive disorder in the United States (2005 and 2010). J Clin Psychiatr. (2015) 76:155–62. doi: 10.4088/JCP.14m09298
11. Hsieh CR, Qin X. Depression hurts, depression costs: the medical spending attributable to depression and depressive symptoms in China. Health Econ. (2018) 27:525–44. doi: 10.1002/hec.3604
12. Lepine JP, Briley M. The increasing burden of depression. Neuropsychiatr Dis Treat. (2011) 7:3–7. doi: 10.2147/NDT.S19617
13. Lin E, Lane HY. Genome-wide association studies in pharmacogenomics of antidepressants. Pharmacogenomics. (2015) 16:555–66. doi: 10.2217/pgs.15.5
14. Singh AB, Bousman CA. Antidepressant pharmacogenetics. Am J Psychiatr. (2017) 174:417–8. doi: 10.1176/appi.ajp.2017.17020173
15. Zajecka JM. Treating depression to remission. J Clin Psychiatr. (2003) 64:7–12. Available online at: https://www.psychiatrist.com/JCP/article/Pages/2003/v64s15/v64s1503.aspx
16. Nierenberg AA. Predictors of response to antidepressants general principles and clinical implications. Psychiatr Clin North Am. (2003) 26:345–52. doi: 10.1016/S0193-953X(02)00105-3
17. Perlis RH. Pharmacogenetic studies of antidepressant response: how far from the clinic? Psychiatr Clin North Am. (2007) 30:125–38. doi: 10.1016/j.psc.2006.12.004
18. O'Reilly RL, Bogue L, Singh SM. Pharmacogenetic response to antidepressants in a multicase family with affective disorder. Biol Psychiatr. (1994) 36:467–71. doi: 10.1016/0006-3223(94)90642-4
19. Porcelli S, Drago A, Fabbri C, Gibiino S, Calati R, Serretti A. Pharmacogenetics of antidepressant response. J Psychiatry Neurosci. (2011) 36:87–113. doi: 10.1503/jpn.100059
20. Binder EB, Holsboer F. Pharmacogenomics and antidepressant drugs. Ann Med. (2006) 38:82–94. doi: 10.1080/07853890600551045
21. Zackrisson AL, Lindblom B, Ahlner J. High frequency of occurrence of CYP2D6 gene duplication/multiduplication indicating ultrarapid metabolism among suicide cases. Clin Pharmacol Ther. (2010) 88:354–9. doi: 10.1038/clpt.2009.216
22. Höfer P, Schosser A, Calati R, Serretti A, Massat I, Kocabas NA, et al. Cytochrome P450 CYP1A2, CYP2C9, CYP2C19 and CYP2D6 genes are not associated with response and remission in a sample of depressive patients. Int Clin Psychopharmacol. (2009) 24:250–6. doi: 10.1097/YIC.0b013e32832e5b0d
23. Peters EJ, Slager SL, Kraft JB, Jenkins GD, Reinalda MS, McGrath PJ, et al. Pharmacokinetic genes do not influence response or tolerance to citalopram in the STAR*D sample. PLoS ONE. (2008) 3:e1872. doi: 10.1371/journal.pone.0001872
24. Laika B, Leucht S, Heres S, Steimer W. Intermediate metabolizer: increased side effects in psychoactive drug therapy. The key to cost-effectiveness of pretreatment CYP2D6 screening? Pharmacogenomics J. (2009). 9:395–403. doi: 10.1038/tpj.2009.23
25. Gupta M, Neavin D, Liu D, Biernacka J, Hall-Flavin D, Bobo WV, et al. TSPAN5, ERICH3 and selective serotonin reuptake inhibitors in major depressive disorder: pharmacometabolomics-informed pharmacogenomics. Mol Psychiatr. (2016) 21:1717–25. doi: 10.1038/mp.2016.6
26. Nouraei H, Firouzabadi N, Mandegary A, Zomorrodian K, Bahramali E, Shayesteh MRH, et al. Glucocorticoid receptor genetic variants and response to fluoxetine in major depressive disorder. J Neuropsychiatry Clin Neurosci. (2018) 30:45–50. doi: 10.1176/appi.neuropsych.16120322
27. Petit AC, El Asmar K, David DJ, Gardier AM, Becquemont L, Fève B, et al. The association of beta-arrestin2 polymorphisms with response to antidepressant treatment in depressed patients. Prog Neuropsychopharmacol Biol Psychiatr. (2018) 81:74–9. doi: 10.1016/j.pnpbp.2017.10.006
28. Murphy GM Jr, Sarginson JE, Ryan HS, O'Hara R, Schatzberg AF, Lazzeroni LC. BDNF and CREB1 genetic variants interact to affect antidepressant treatment outcomes in geriatric depression. Pharmacogenet Genomics. (2013) 23:301–13. doi: 10.1097/FPC.0b013e328360b175
29. Wang XC, Xu DJ, Chen GH, Xia Q, Liu LN. Association of 2 neurotrophic factor polymorphisms with efficacy of paroxetine in patients with major depressive disorder in a Chinese population. Ther Drug Monit. (2014) 36:612–7. doi: 10.1097/FTD.0000000000000062
30. Colle R, Gressier F, Verstuyft C, Deflesselle E, Lépine JP, Ferreri F, et al. Brain-derived neurotrophic factor Val66Met polymorphism and 6-month antidepressant remission in depressed Caucasian patients. J Affect Disord. (2015) 175:233–40. doi: 10.1016/j.jad.2015.01.013
31. Chi MH, Chang HH, Lee SY, Lee IH, Gean PW, Yang YK, et al. Brain derived neurotrophic factor gene polymorphism (Val66Met) and short-term antidepressant response in major depressive disorder. J Affect Disord. (2010) 126:430–5. doi: 10.1016/j.jad.2010.07.006
32. Gassó P, Rodríguez N, Blázquez A, Monteagudo A, Boloc D, Plana MT, et al. Epigenetic and genetic variants in the HTR1B gene and clinical improvement in children and adolescents treated with fluoxetine. Prog Neuropsychopharmacol Biol Psychiatr. (2017) 75:28–34. doi: 10.1016/j.pnpbp.2016.12.003
33. Kato M, Fukuda T, Wakeno M, Okugawa G, Takekita Y, Watanabe S, et al. Effect of 5-HT1A gene polymorphisms on antidepressant response in major depressive disorder. Am J Med Genet B Neuropsychiatr Genet. (2009) 150:115–23. doi: 10.1002/ajmg.b.30783
34. Lin JY, Jiang MY, Kan ZM, Chu Y. Influence of 5-HTR2A genetic polymorphisms on the efficacy of antidepressants in the treatment of major depressive disorder: a meta-analysis. J Affect Disord. (2014) 168:430–8. doi: 10.1016/j.jad.2014.06.012
35. Mrazek DA, Biernacka JM, McAlpine DE, Benitez J, Karpyak VM, Williams MD, et al. Treatment outcomes of depression: the pharmacogenomic research network antidepressant medication pharmacogenomic study. J Clin Psychopharmacol. (2014) 34:313–7. doi: 10.1097/JCP.0000000000000099
36. Biernacka JM, Sangkuhl K, Jenkins G, Whaley RM, Barman P, Batzler A, et al. The international SSRI pharmacogenomics consortium (ISPC): a genome-wide association study of antidepressant treatment response. Transl Psychiatr. (2015) 5:e553. doi: 10.1038/tp.2015.47
37. Garriock HA, Kraft JB, Shyn SI, Peters EJ, Yokoyama JS, Jenkins GD, et al. A genomewide association study of citalopram response in major depressive disorder. Biol Psychiatr. (2010) 67:133–8. doi: 10.1016/j.biopsych.2009.08.029
38. Uher R, Perroud N, Ng MY, Hauser J, Henigsberg N, Maier W, et al. Genome-wide pharmacogenetics of antidepressant response in the GENDEP project. Am J Psychiatr. (2010) 167:555–64. doi: 10.1176/appi.ajp.2009.09070932
39. Ising M, Lucae S, Binder EB, Bettecken T, Uhr M, Ripke S, et al. A genomewide association study points to multiple loci that predict antidepressant drug treatment outcome in depression. Arch Gen Psychiatr. (2009) 66:966–975. doi: 10.1001/archgenpsychiatry.2009.95
40. Laje G, McMahon FJ. Genome-wide association studies of antidepressant outcome: a brief review. Prog Neuropsychopharmacol Biol Psychiatr. (2011) 35:1553–7. doi: 10.1016/j.pnpbp.2010.11.031
41. Myung W, Kim J, Lim SW, Shim S, Won HH, Kim S, et al. A genome-wide association study of antidepressant response in Koreans. Transl Psychiatr. (2015) 5:e633. doi: 10.1038/tp.2015.127
42. Whirl-Carrillo M, McDonagh EM, Hebert JM, Gong L, Sangkuhl K, Thorn CF, et al. Pharmacogenomics knowledge for personalized medicine. Clin Pharmacol Ther. (2012) 92:414–7. doi: 10.1038/clpt.2012.96
43. Sim NL, Kumar P, Hu J, Henikoff S, Schneider G, Ng PC. SIFT web server: predicting effects of amino acid substitutions on proteins. Nucleic Acids Res. (2012) 40:W452–7. doi: 10.1093/nar/gks539
44. Adzhubei IA, Schmidt S, Peshkin L, Ramensky VE, Gerasimova A, Bork P, et al. A method and server for predicting damaging missense mutations. Nat Methods. (2010) 7:248–9. doi: 10.1038/nmeth0410-248
45. Hecht M, Bromberg Y, Rost B. Better prediction of functional effects for sequence variants. BMC Genomics. (2015) 16:S1. doi: 10.1186/1471-2164-16-S8-S1
46. Capriotti E, Fariselli P, Casadio R. I-Mutant2.0: predicting stability changes upon mutation from the protein sequence or structure. Nucleic Acids Res. (2005) 33:W306–10. doi: 10.1093/nar/gki375
47. Ashkenazy H, Abadi S, Martz E, Chay O, Mayrose I, Pupko T, et al. ConSurf 2016: an improved methodology to estimate and visualize evolutionary conservation in macromolecules. Nucleic Acids Res. (2016) 44:W344–50. doi: 10.1093/nar/gkw408
48. Waterhouse A, Bertoni M, Bienert S, Studer G, Tauriello G, Gumienny R, et al. SWISS-MODEL: homology modelling of protein structures and complexes. Nucleic Acids Res. (2018) 46:W296–303. doi: 10.1093/nar/gky427
49. Abraham MJ, Murtola TJ, Schulz R, Páll S, Smith JC, Hess B, et al. GROMACS: high performance molecular simulations through multi-level parallelism from laptops to supercomputers. SoftwareX. (2015) 1–2:19–25. doi: 10.1016/j.softx.2015.06.001
50. Lindorff-Larsen K, Piana S, Palmo K, Maragakis P, Klepeis JL, Dror RO, et al. Improved side-chain torsion potentials for the Amber ff99SB protein force field. Proteins. (2010) 78:1950–8. doi: 10.1002/prot.22711
51. Wang A, Savas U, Hsu MH, Stout CD, Johnson EF. Crystal structure of human cytochrome P450 2D6 with prinomastat bound. J Biol Chem. (2012) 287:10834–43. doi: 10.1074/jbc.M111.307918
52. Fujikura K, Ingelman-Sundberg M, Lauschke VM. Genetic variation in the human cytochrome P450 supergene family. Pharmacogenet Genomics. (2015) 25:584–94. doi: 10.1097/FPC.0000000000000172
53. Maréchal JD, Kemp CA, Roberts GC, Paine MJ, Wolf CR, Sutcliffe MJ. Insights into drug metabolism by cytochromes P450 from modelling studies of CYP2D6-drug interactions. Br J Pharmacol. (2008) 153(Suppl. 1):S82–9. doi: 10.1038/sj.bjp.0707570
54. Zhou SF. Polymorphism of human cytochrome P450 2D6its clinical significance: part II. Clin Pharmacokinet. (2009) 48:761–804. doi: 10.2165/11318070-000000000-00000
55. Ng C, Sarris J, Singh A, Bousman C, Byron K, Peh LH, et al. Pharmacogenetic polymorphisms and response to escitalopram and venlafaxine over 8 weeks in major depression. Hum Psychopharmacol. (2013) 28:516–22. doi: 10.1002/hup.2340
56. Hodgson K, Tansey K, Dernovsek MZ, Hauser J, Henigsberg N, Maier W, et al. Genetic differences in cytochrome P450 enzymes and antidepressant treatment response. J Psychopharmacol. (2014) 28:133–41. doi: 10.1177/0269881113512041
57. Taranu A, Colle R, Gressier F, El Asmar K, Becquemont L, Corruble E, et al. Should a routine genotyping of CYP2D6 and CYP2C19 genetic polymorphisms be recommended to predict venlafaxine efficacy in depressed patients treated in psychiatric settings? Pharmacogenomics. (2017) 18:639–50. doi: 10.2217/pgs-2017-0003
58. Torrellas C, Carril JC, Cacabelos R. Optimization of antidepressant use with pharmacogenetic strategies. Curr Genomics. (2017) 18:442–9. doi: 10.2174/1389202918666170426164940
59. Zastrozhin MS, Grishina EA, Denisenko NP, Skryabin VY, Markov DD, Savchenko LM, et al. Effects of CYP2D6 genetic polymorphisms on the efficacy and safety of fluvoxamine in patients with depressive disorder and comorbid alcohol use disorder. Pharmacogenomics Pers Med. (2018) 11:113–9. doi: 10.2147/PGPM.S160763
60. Tsai MH, Lin KM, Hsiao MC, Shen WW, Lu ML, Tang HS, et al. Genetic polymorphisms of cytochrome P450 enzymes influence metabolism of the antidepressant escitalopram and treatment response. Pharmacogenomics. (2010) 11:537–46. doi: 10.2217/pgs.09.168
61. Han KM, Chang HS, Choi IK, Ham BJ, Lee MS. CYP2D6 P34S polymorphism and outcomes of escitalopram treatment in Koreans with major depression. Psychiatry Invest. (2013) 10:286–93. doi: 10.4306/pi.2013.10.3.286
62. Venselaar H, Te Beek TA, Kuipers RK, Hekkelman ML, Vriend G. Protein structure analysis of mutations causing inheritable diseases. An e-Science approach with life scientist friendly interfaces. BMC Bioinformatics. (2010) 11:548. doi: 10.1186/1471-2105-11-548
63. Dong AN, Ahemad N, Pan Y, Palanisamy UD, Yiap BC, Ong CE. Functional and structural characterisation of common cytochrome P450 2D6 allelic variants-roles of Pro34 and Thr107 in catalysis and inhibition. Naunyn Schmiedebergs Arch Pharmacol. (2019) 392:1015–29. doi: 10.1007/s00210-019-01651-0
64. Fukuyoshi S, Kometani M, Watanabe Y, Hiratsuka M, Yamaotsu N, Hirono S, et al. Molecular dynamics simulations to investigate the influences of amino acid mutations on protein three-dimensional structures of cytochrome P450 2D6.1, 2, 10, 14A, 51, and 62. PLoS ONE. (2016) 11:e0152946. doi: 10.1371/journal.pone.0152946
65. Sakuyama K, Sasaki T, Ujiie S, Obata K, Mizugaki M, Ishikawa M, et al. Functional characterization of 17 CYP2D6 allelic variants (CYP2D6.2, 10, 14A-B, 18, 27, 36, 39, 47-51, 53-55, and 57). Drug Metab Dispos. (2008) 36:2460–7. doi: 10.1124/dmd.108.023242
66. Gaedigk A, Bradford LD, Alander SW, Leeder JS. CYP2D6*36 gene arrangements within the cyp2d6 locus: association of CYP2D6*36 with poor metabolizer status. Drug Metab Dispos. (2006) 34:563–9. doi: 10.1124/dmd.105.008292
67. Matsunaga M, Yamazaki H, Kiyotani K, Iwano S, Saruwatari J, Nakagawa K, et al. Two novel CYP2D6*10 haplotypes as possible causes of a poor metabolic phenotype in Japanese. Drug Metab Dispos. (2009) 37:699–701. doi: 10.1124/dmd.108.026096
68. Muroi Y, Saito T, Takahashi M, Sakuyama K, Niinuma Y, Ito M, et al. Functional characterization of wild-type and 49 CYP2D6 allelic variants for N-desmethyltamoxifen 4-hydroxylation activity. Drug Metab Pharmacokinet. (2014) 29:360–6. doi: 10.2133/dmpk.DMPK-14-RG-014
69. Kim J, Lim YR, Han S, Han JS, Chun YJ, Yun CH, et al. Functional influence of human CYP2D6 allelic variations: P34S, E418K, S486T,R296C. Arch Pharm Res. (2013) 36:1500–6. doi: 10.1007/s12272-013-0212-5
70. Pu M, Zhang Z, Xu Z, Shi Y, Geng L, Yuan Y, et al. Influence of genetic polymorphisms in the glutamatergic and GABAergic systems and their interactions with environmental stressors on antidepressant response. Pharmacogenomics. (2013) 14:277–88. doi: 10.2217/pgs.13.1
71. Whiting PJ, Bonnert TP, McKernan RM, Farrar S, Le Bourdellès B, Heavens RP, et al. Molecular and functional diversity of the expanding GABA-A receptor gene family. Ann N Y Acad Sci. (1999) 868:645–53. doi: 10.1111/j.1749-6632.1999.tb11341.x
72. Maciukiewicz M, Marshe VS, Tiwari AK, Fonseka TM, Freeman N, Kennedy JL, et al. Genome-wide association studies of placebo and duloxetine response in major depressive disorder. Pharmacogenomics J. (2018) 18:406–12. doi: 10.1038/tpj.2017.29
73. Wilkie MJ, Smith G, Day RK, Matthews K, Smith D, Blackwood D, et al. Polymorphisms in the SLC6A4 and HTR2A genes influence treatment outcome following antidepressant therapy. Pharmacogenomics J. (2009) 9:61–70. doi: 10.1038/sj.tpj.6500491
74. Minov C, Baghai TC, Schüle C, Zwanzger P, Schwarz MJ, Zill P, et al. Serotonin-2A-receptor and -transporter polymorphisms: lack of association in patients with major depression. Neurosci Lett. (2001) 303:119–22. doi: 10.1016/S0304-3940(01)01704-9
75. Smith RM, Papp AC, Webb A, Ruble CL, Munsie LM, Nisenbaum LK, et al. Multiple regulatory variants modulate expression of 5-hydroxytryptamine 2A receptors in human cortex. Biol Psychiatry. (2013) 73:546–54. doi: 10.1016/j.biopsych.2012.09.028
76. McMahon FJ, Buervenich S, Charney D, Lipsky R, Rush AJ, Wilson AF, et al. Variation in the gene encoding the serotonin 2A receptor is associated with outcome of antidepressant treatment. Am J Hum Genet. (2006) 78:804–14. doi: 10.1086/503820
77. Horstmann S, Lucae S, Menke A, Hennings JM, Ising M, Roeske D, et al. Polymorphisms in GRIK4, HTR2A, and FKBP5 show interactive effects in predicting remission to antidepressant treatment. Neuropsychopharmacology. (2010) 35:727–40. doi: 10.1038/npp.2009.180
Keywords: major depressive disorder, antidepressants, non-synonymous SNPs, protein structure, molecular dynamics simulations
Citation: Xin J, Yuan M, Peng Y and Wang J (2020) Analysis of the Deleterious Single-Nucleotide Polymorphisms Associated With Antidepressant Efficacy in Major Depressive Disorder. Front. Psychiatry 11:151. doi: 10.3389/fpsyt.2020.00151
Received: 22 November 2019; Accepted: 18 February 2020;
Published: 18 March 2020.
Edited by:
Chad A. Bousman, University of Calgary, CanadaReviewed by:
Yaowaluck Hongkaew, Mahidol University, ThailandCopyright © 2020 Xin, Yuan, Peng and Wang. This is an open-access article distributed under the terms of the Creative Commons Attribution License (CC BY). The use, distribution or reproduction in other forums is permitted, provided the original author(s) and the copyright owner(s) are credited and that the original publication in this journal is cited, in accordance with accepted academic practice. No use, distribution or reproduction is permitted which does not comply with these terms.
*Correspondence: Ju Wang, d2FuZ2p1QHRtdS5lZHUuY24=
Disclaimer: All claims expressed in this article are solely those of the authors and do not necessarily represent those of their affiliated organizations, or those of the publisher, the editors and the reviewers. Any product that may be evaluated in this article or claim that may be made by its manufacturer is not guaranteed or endorsed by the publisher.
Research integrity at Frontiers
Learn more about the work of our research integrity team to safeguard the quality of each article we publish.