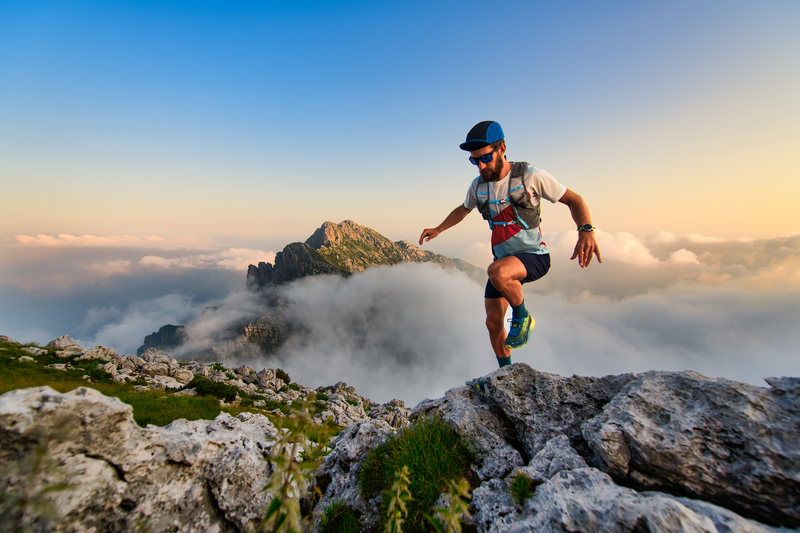
95% of researchers rate our articles as excellent or good
Learn more about the work of our research integrity team to safeguard the quality of each article we publish.
Find out more
REVIEW article
Front. Psychiatry , 30 October 2019
Sec. Psychological Therapies
Volume 10 - 2019 | https://doi.org/10.3389/fpsyt.2019.00779
This article is part of the Research Topic Returning to Mechanisms in Psychological Therapies: Understand the Engine Before Steaming In View all 10 articles
Mental imagery is a promising tool and mechanism of psychological interventions, particularly for mood and anxiety disorders. In parallel developments, neuromodulation techniques have shown promise as add-on therapies in psychiatry, particularly non-invasive brain stimulation for depression. However, these techniques have not yet been combined in a systematic manner. One novel technology that may be able to achieve this is neurofeedback, which entails the self-regulation of activation in specific brain areas or networks (or the self-modulation of distributed activation patterns) by the patients themselves, through real-time feedback of brain activation (for example, from functional magnetic resonance imaging). One of the key mechanisms by which patients learn such self-regulation is mental imagery. Here, we will first review the main mental imagery approaches in psychotherapy and the implicated brain networks. We will then discuss how these networks can be targeted with neuromodulation (neurofeedback or non-invasive or invasive brain stimulation). We will review the clinical evidence for neurofeedback and discuss possible ways of enhancing it through systematic combination with psychological interventions, with a focus on depression, anxiety disorders, and addiction. The overarching aim of this perspective paper will be to open a debate on new ways of developing neuropsychotherapies.
The neural mechanisms of psychotherapy have been investigated with methods of functional imaging for over 20 years (1–4). This line of investigation has fascinated psychotherapists and neuroscientists alike and has the potential of aiding the design of new therapies that are guided by neural mechanisms, as well as aiding the allocation of patients to particular treatment modalities. It is also a particularly challenging area of research because most therapies target a range of interacting processes, resulting in complex changes in neural activation, which are also difficult to study over the generally long timeframes of psychological treatment programmes. One success story in this respect has been the elucidation of mechanisms of mental imagery—in general and in its specific relevance for emotional states. The opportunity to study the neural substrates of mental imagery, which came with the development of non-invasive functional imaging methods, particularly functional magnetic resonance imaging (fMRI), has been parallel by an increasing interest in its use in modern cognitive and behavioural therapy approaches. An overview of this field is thus topic for a volume on mechanism of psychotherapy. In addition, the authors, and a growing international community of clinicians and researchers, have developed an interest in the combination of mental imagery with neuromodulation techniques, particularly neurofeedback. We will argue that the advances in the neuroscience of mental imagery and the increasing evidence for its utility particularly in affective and anxiety disorders now create a unique opportunity to exploit the enhancement of imagery approaches through neuromodulation (and vice versa) for the development of new integrated treatment tools, which may initially focus on depression, post-traumatic stress disorder, and specific phobias, but can, in principle, also be developed for a much wider range of psychological disorders.
While psychodynamic treatment approaches have analysed the symbolic properties of mental images for decades, cognitive therapies have recently developed efforts to incorporate mental imagery in order to alter affective states and modify their cognitive context (see the study by Edwards (5), and note, for example, the study by Clyne (6) for an active psychodynamic imagery approach). Independently, different mental imagery interventions were developed that aimed to facilitate the effects of mental imagery trough neurofeedback (7–11). By summarising the neuroscientific evidence on mental imagery with and without neurofeedback, this review highlights the potential for cross-disciplinary treatments that combine psychological and neuroscientific tools strategically.
Firstly, the main domains of mental imagery in psychotherapy will be introduced and neuroscientific evidence on their working mechanisms will be discussed. Secondly, psychiatric applications of neurofeedback and the main working mechanisms of neurofeedback trainings will be reviewed. Thirdly, existing evidence on interactions between psychotherapy and neurofeedback approaches will be summarised and future pathways of combining psychotherapy with neurofeedback will be discussed, as well as the potential benefit of brain stimulation techniques.
A crucial feature of mental images is their ability to induce emotional states (12–14). Taking into account that especially mood and anxiety disorders are marked by dysfunctions of the emotional system, this property of mental imagery has been repeatedly used to induce changes in the affective symptoms of these disorders.
A main category of mental imagery applications used to achieve this consists of repeated mental imagery with positive valence. Repeated positive imagery has been shown to increase the tendency to interpret ambiguous situations as more positive and induce positive mood in healthy participants (15, 16). As depression in particular is marked by pronounced negativity biases (see the review by Gotlib and Joormann (17) for an overview), i.e., the tendency to interpret ambiguous situations as more negative, it can be expected that positive mental imagery will improve depressive symptoms through strengthening positive affective reactions. Accordingly, a recent clinical trial has evaluated positive mental imagery as being effective in reducing such negative biases in depression (18).
Another main category of mental imagery applications consists of mental imagery of specific, fear inducing mental objects or contexts, i.e., imaginal exposure. For example, through imagining fear inducing stimuli that trigger phobic reactions repeatedly, patients can desensitize. This ‘imaginal exposure’ approach has been successfully implemented in the treatment of a variety of phobias (19–22). Symptoms of other anxiety disorders have also been shown to be significantly attenuated by imaginal exposure, including obsessive-compulsive disorder (23–25) and post-traumatic stress disorder (26, 27).
In addition to the two main valence categories of mental imagery (enhancing positive emotions, or imaginal exposure to negative material), some treatment approaches entail switches between negative and positive imagery, for example, imagery rescripting (28–32) or guided imagery (33–35). Switching valence is thereby usually achieved by either changing a mental image to an image with more positive features or wholly replacing it with another image (36). As negative mental imagery constitutes a crucial feature of major psychopathologies (36), it seems not surprising that such substitutions or alterations of negative mental images can be effective across a range of disorders (see the studies by Holmes et al. (37) and Stopa (38) for an overview), including post-traumatic stress disorder (PTSD) (30, 32, 39) and depression (40, 41).
While rescripting aims to alter the content of mental images and, accordingly, also their emotional effect (37, 42), strategies that focus on cognitive control instead aim at changing the interpretations of (mental) images, i.e., their cognitive context (42–44). Here, notably cognitive reappraisal has been widely used to alter the cognitive context of emotion-inducing material (45, 46). Reappraisal has thereby repeatedly been shown to function as an effective emotion regulation strategy, compared to other top-down emotion-regulation strategies as, for example, active suppression (47–49).
Considering that the main focus of mental imagery applications in psychotherapy lies in influencing emotional processes, an understanding of the neural basis of emotions is fundamental for understanding the neural effects of mental imagery. The current section will therefore provide an overview on the brain systems connecting mental imagery to affective processes (Figure 1).
Figure 1 Major functional components and associated brain systems of mental imagery during emotion regulation. (A) Cognitive control during mental imagery treatments relies on a distributed cortical network. The dorsolateral PFC and lateral parietal cortex (blue) control mental resources, while the semantic interpretation of the internal state is associated with activation in the ventrolateral PFC and the lateral temporal cortex (dark green). The anterior cingulate cortex (ACC, purple) and the medial PFC (brown) monitor the ongoing processes. The ACC together with the insula (red) thereby function as major connecting hubs between bottom-up saliency information and top-down attentional control. (B) Visual experiences during mental imagery mimic actual perception of external stimuli in the visual system (light green). The sensory content of a mental image is thereby activated across different levels of the visual processing hierarchy, ranging from low level visual areas to associate cortices. Modulations in the default-mode-network (turquoise) indicate increased processing of the endogenously generated information. (C) Particularly, the limbic system (purple), the basal ganglia (orange/yellow), and the insula (red) encode the hedonic value, arousal, and salience of the visual experience. This information can directly affect ongoing learning processes as particularly the hippocampus is crucially involved in encoding and retrieving emotional memories. The insula, the anterior cingulate cortex, the amygdala, and the ventral basal ganglia form a network that encodes the salience of a generated experience. As a connecting hub between various systems the insula constitutes a key region for introspection during mental imagery. ACC, anterior cingulate cortex; AG, angular gyrus; AMG, amygdala; BG, basal ganglia; DLPFC, dorsolateral PFC; DVS, dorsal visual stream; HC, hippocampus; INS, insula; LS, limbic system; LTL, lateral temporal lobe; MPFC, medial PFC; PCC/PreC, posterior cingulate cortex/precuneus; VC, visual cortex; VLPFC, ventrolateral PFC; VVS, ventral visual stream; CC, cingulate cortex.
With the immense growth of available neuroimaging data during the last century, a vast body of empirical evidence has grown that helps to relate activation in defined brain structures to emotional experiences. Studies that focused on specific involvement of certain brain regions showed some correspondence between brain structures and emotional states. The amygdala, for example, appears to reliably activate during the experience of fear (50–52). Such findings are in accordance with a body of neuropsychiatric evidence suggesting that lesions in these areas lead to attenuated fear responses (53, 54). Similar findings have been obtained for many other emotional states, e.g., for the insula as being crucial in experience for disgust (55–57) or the orbitofrontal cortex for anger (58, 59).
Although such findings show that activation in certain brain areas can reflect subjective psychological experiences of emotions, they do not imply that activation in these brain structures is necessarily specific to a particular emotional state. Meta-analysis combining data of different emotional states could reliably show that the amygdala contributes to various different emotions (60, 61), with negative as well as positive valence. Such findings support contemporary constructivist approaches, which constitute that emotional experiences do not emerge from increased activation of single brain structures, but rather from pattern of activation distributed across brain regions that subserve more general functions (see, for example, the studies by Barrett (62) and Lindquist and Barrett (63)). In constructivist approaches, a brain area contributes to an emotional experience by giving rise to one of the many subprocesses from which a holistic emotional experience is constructed. The amygdala, for example, has been implicated in indicating the salience of an emotional stimulus (64, 65) and its involvement in fear has thereby been related to the high salience of fear inducing stimuli. Accordingly, more general functions for various other structures involved in core affect have been suggested (see the study by Lindquist and Barrett (63) for an overview).
Notably, emotional experiences are additionally accompanied by activation in areas that are not directly implicated in core affect (60, 61, 66–68). Across emotional categories, activation increases have been observed in prefrontal areas related to higher order cognitive processes (see the studies by Storbeck and Clore (69) and Duncan and Barrett (70)), the memory system (notably, the medial temporal lobe complex, see the studies by Squire and Zola-Morgan (71); Dolcos et al. (72); Squire et al. (73), and Eichenbaum et al. (74)) or sensory processing (see, for example, the study by Saarimäki et al. (75)), and particularly modulations in visual cortex activity have been observed reliably (61, 76–78). It has therefore been repeatedly argued that emotional experiences arise from distributed neural representations that include affective, cognitive as well as sensory components (61, 69). Particularly, this embeddedness of the affective system into cognitive and sensory networks provides the possibility for mental imagery approaches to alter emotional states by targeting the sensory or cognitive components of emotions.
In accordance with the notion that emotional states are linked to associated sensory experiences, it has been suggested that, during mental imagery, especially, the sensory aspects of a mental image (in contrast to the semantic information contained in it) are fundamental in evoking emotions (36). Indeed, when comparing emotion induction through language to emotion induction by mental imagery, mental imagery evokes stronger emotional responses even when the semantic content is matched between modalities (37, 79). In contrast to actual visual perception, mental imagery is not triggered by external visual stimuli and constitutes a purely mental operation. Interestingly, patterns of brain activation in the visual system during mental imagery resemble activation of weak external visual stimuli (see the study by Pearson et al. (80) for an overview).
A considerable body of experimental psychology and cognitive neuroscience studies suggests that mental imagery can be considered as a form of top-down induced (i.e., internally generated) visual perception, although the exact neural mechanisms are the matter of an ongoing debate. Early fMRI studies revealed reliable brain activation during mental imagery in higher level visual areas, i.e., areas in which visual information is combined to comprehensive visual features (notably the associate visual cortex, early studies by D’Esposito et al. (81); Goebel et al. (82), and Knauff et al. (83). Activation in V1 in turn, i.e., the earliest cortical processing stage of visual information, has also been observed during mental imagery (84–86) but appears to be less pronounced (86) and the ability to form vivid mental images remains preserved after extensive lesioning of V1 (87, 88), supporting that V1 incorporates a less crucial function for the formation of mental images than higher level areas of the visual stream.
Notably, while emotion-related brain activation has been observed in the whole visual cortex, findings for V1 are strongly modulated by the use of visual stimulation in experiments (60). In contrast, higher level visual areas reliably activate also when no visual stimulation is provided (61), suggesting that they serve a more general function in relation to emotions. Crucially, it has been shown that mental imagery is comparably effective as other common mood induction procedures in evoking emotional states (12, 13, 89), underlining that mental imagery can modulate the affective system effectively.
In addition to the cortical pathway which processes complex visual information and gives rise to conscious visual percepts, a subcortical route of visual processing exists for simple, evolutionally meaningful stimuli such as snakes or spiders (90–93). Although the influence of mental imagery on such reflexive sensory-affect connections has not yet been investigated thoroughly, the effectiveness of mental imagery as treatment of the phobias associated with these groups of animals (see, for example, the studies by Lang et al. (94) and Hunt and Fenton (95)) suggests that, at least, the emotional reactivity of subcortical areas involved (prominently the amygdala) can be modulated through mental imagery. However, further research is needed to determine the influence of mental imagery on such basic visual triggers of the affective system. Neurofeedback and other neuromodulation strategies may play a particular role for the synergistic targeting of such limbic circuits.
While the affective and sensory experiences are intertwined, their relationship is modulated by past experiences and ongoing cognitive processes (96). Taking into account that retrieval of autobiographical memories is commonly associated with mental imagery, an overlapping neural mechanism of episodic memory and mental imagery has been suggested (79, 97). In parallel, it has been shown that mental simulations of fictional events share an extensive neural basis with episodic memory retrieval, including the default-mode and frontoparietal control network (FPCN) (see the studies by Botzung et al. (98); Gerlach et al. (99), and Benoit and Schacter (100)).
In clinical conditions, this interconnectedness of episodic memory and mental imagery appears to be of particular relevance. Several common psychopathologies are characterised by intrusive mental images of emotionally loaded past experiences, and in the case of PTSD, they constitute a core symptom (see the study by Hackmann and Holmes (101)). Brain activation in relation to such intrusive mental images in PTSD (“flashbacks”) has been associated with increased activation in higher-order visual areas in comparison to ordinary episodic memoires (102), supporting the clinical relevance of connections between mental images and the memory system.
While particularly autobiographical experiences have been suggested to contribute to the effects of mental imagery in emotion regulation (79), networks involved in processing the current cognitive context of a percept also strongly contribute to its effect on the affective system (103–105). In this context, anterior prefrontal areas along the midline (particularly the ventromedial PFC and anterior cingulate cortex) have been suggested to integrate incoming information from the limbic system and sensory areas with higher level cognitive goals (96). Lateral prefrontal and parietal areas, in turn, have been suggested to subserve primarily executive functions in the context of emotion regulation. Particularly, activation in the FPCN has been shown to relate to success in emotion generation (106). Interestingly, the FPCN has also been shown to be selectively involved in retrieving visuospatial features of episodic memory (107), supporting the interconnectedness of episodic memory, mental imagery, and emotion.
Overall, these findings suggest that the neural basis of mental imagery, especially in the context of emotion regulation, spans several different functional domains. Although the recruited networks are highly interconnected and partly overlapping, the different mental imagery-based treatment approaches put more weight on certain subsystems.
Approaches that focus on endogenous generation of emotions through mental imagery (e.g., by using mental imagery to induce positive mood or negative mental images for desensitization) firstly aim at modulating the affective core system. Such endogenous generation of emotions has been shown to involve distributed neural components that interact to create an emotional experience and are shared between positive and negative valance, as well as levels of arousal (106).
For generating the affective core state, particularly the salience network (i.e., a network crucial in determining the saliency of external or internal events) together with the limbic system appears to be crucial. The actual representation of an emotional experience, i.e., an affective state coupled to its mental content, is associated with coactivation of limbic areas, particularly the amygdala and the striatum, and areas related to inward directed attention, particularly the default mode network. Such representations of an emotional state additionally show modulations specific to the mental modality used for emotion generation, i.e., they are accompanied by different activation for mental imagery of visual/autobiographical memories, bodily experience, and semantic strategies. Additionally, before the representation of an affective experience is created, the FPCN has been suggested to initiate the emotion generation processes and, afterwards, contribute to the maintenance of the generated mental representation (106). Due to the role of the FPCN in executive functions and attention, it likely mobilizes and bundles the mental resources necessary to engage in the emotion generation procedure.
While endogenous emotion generation thereby also involves top-down control systems, other treatment approaches target higher order cognitive processes more explicitly. Notably, for cognitive reappraisal, several studies have tried to disentangle the involved brain systems (early studies by Beauregard et al. (108) and Ochsner et al. (109); see also the study by Buhle et al. (110) for an overview). Cognitive reappraisal implies that an emotionally salient event already is present (as the object of the reappraisal, which is represented physically or recalled in memory), this approach does not focus on initiating activation in the core affective system, but rather on altering activation in areas contributing to the cognitive context of the emotional event (which then leads to modulations in the affective core system). In this process, the ventrolateral PFC and the lateral temporal cortex have been suggested to contribute to selecting and representing the semantic context, while the dorsolateral PFC and lateral parietal cortex support shifting the semantic context by modulating the attentional focus towards particular aspects of the mental event and controlling working memory content. In addition, the ACC and the medial PFC have been suggested to monitor ongoing affective and cognitive processing and integrate information from both systems to execute appropriate top-down control (43, 96, 110–112). Most neuroimaging work converges to suggest that cognitive reappraisal is associated with downregulation of the amygdala (110), thereby potentially decreasing the salience of the emotional event.
As mental imagery and emotion regulation recruit defined brain systems, techniques that modulate brain activation can be implemented to support the neural processes that take place in these networks. Additionally, especially neuroimaging-based techniques can thereby provide valuable information on the neural effects of the mental operations that are performed. Neurofeedback approaches combine these two aspects. During a neurofeedback intervention, neuroimaging is applied in order to measure relevant markers of brain activation and feed this information back to participants. By providing neurofeedback during mental imagery, participants thereby become enabled to adapt their mental imagery strategies based on whether the strategies successfully contribute to achieving a desired brain target state.
For several decades, neurofeedback has been created from electrophysiological brain signals acquired through electroencephalography (EEG) (see the study by Kamiya (113) for an overview). While EEG can provide temporally accurate feedback and is accessible to communities, it is a matter for debate whether it can provide reliable signals from deeper brain structures (Nunez et al. (114); Babiloni et al. (115); Burle et al. (116), but see the study by Keynan et al. (117)). Functional magnetic resonance imaging (fMRI) on the other hand, can more accurately narrow down the location in the brain from which neurofeedback is provided and can create neurofeedback from subcortical areas (see the study by Auer and Auer (118)).
Neurofeedback can be incorporated in psychotherapy because of its active use of psychological techniques, which can range from classical and operant conditioning to explicit imagery and cognitive strategies. This section will review the development of clinical neurofeedback based on fMRI for psychiatric applications. It will focus on fMRI-based neurofeedback rather than incorporate the much larger field of EEG-based neurofeedback because the design of fMRI studies, targeting specific brain regions or networks, has generally followed the considerations of pathophysiological or compensatory networks outlined in the previous sections of this review and has often explicitly incorporated elements of mental imagery.
The clinical development of fMRI-neurofeedback (fMRI-NF) started in 2005 with the publication of a study in patients with fibromyalgia (119). Although conceptually, there should be great promise of such a self-regulation training in patients with chronic pain syndromes relatively little work has followed up on this. In 2011, we published our first study on fMRI-NF in neurorehabilitation, targeting the supplementary motor area (SMA) in Parkinson’s disease (120), which was followed up by further studies targeting SMA in neurodegenerative diseases (121, 122). In terms of psychiatric applications, patient studies have mainly been conducted in depression, anxiety disorders, and addiction. In a pilot study of fMRI-NF in depression, we trained patients with depression to upregulate brain networks responsive to positive affective stimuli. This paradigm was modelled on our previous work with healthy participants, which had shown that the neurofeedback component (compared to imagery alone) is required for reliable control over emotion networks (123). The eight patients who underwent this fMRI-NF protocol for four sessions improved significantly on the 17-item Hamilton Rating Scale for Depression, and this clinical improvement was not observed in eight control patients who engaged in a protocol of positive emotional imagery (matched to the fMRI-NF protocol for intervention and assessment times and affective stimuli) outside the scanner (124). In a follow-up randomised controlled trial (RCT) with a similar protocol, we found similar levels of clinical improvement; however, this was also seen for a control intervention using neurofeedback of the parahippocampal place area, an area involved in the processing of scenes and places (125).
A recent RCT pitting upregulation training of the amygdala against upregulation of a control area (the intraparietal sulcus region) found clinical improvement in patients with depression that was significantly stronger in the active (amygdala upregulation) than the control intervention (126). Thus, there is now evidence from RCTs to suggest that fMRI-NF targeting brain networks of emotions may have benefits for patients with depression although it is less clear how specific the protocols/trainings have to be to be effective.
In the area of anxiety disorders, several studies have investigated self-regulation training of the amygdala for PTSD (127–131), and another line of research is targeting the anterior cingulate cortex (131). These have mostly been feasibility studies and information about clinical outcomes is not yet available. Interestingly, both amygdala upregulation (during positive autobiographical memory) (129) and downregulation (during confrontation with traumatic experience) (130) have been employed. Pilot studies have also been conducted in specific phobia (132) and obsessive compulsive disorder (OCD) (133). FMRI-NF has also been piloted for several substance use disorders (134–137) and formal clinical trials are under way(138, 139).
Mental imagery is one of the most common strategies reported by participants training to control the neurofeedback signal and often explicitly suggested as a potential strategy by the investigators. For this reason, the same general networks related to self-regulation, emotion, and visual imagery are modulated during these neurofeedback interventions as discussed for the psychotherapeutic applications of mental imagery (124, 140, 141). As a crucial difference to conventional mental imagery approaches, neurofeedback training additionally entails providing information on a subcomponent of these networks to the participant.
Across different psychiatric populations, a common target of fMRI neurofeedback trainings is core emotional areas (Figure 1). By providing participants with the opportunity to identify mental strategies that boost/decrease activation in these regions, participants can learn to gain control over their emotional system. This is usually achieved by training participants to increase brain activation in relevant areas during positive mental imagery (123–125, 126, 142) or to decrease activation in areas responsive to negative affective stimuli (143, 144). While neurofeedback provides information on whether a mental strategy is effective in modulating the neural basis of an emotional state, it has also been shown that neurofeedback at the same time stimulates the reward system (141, 145, 146) thereby potentially reinforcing the desired neural target state additionally (see the study by Sitaram et al. (147) for an overview on prominent theoretical accounts on the working mechanisms of neurofeedback).
In addition to inducing changes in the affective core system, neurofeedback approaches can also facilitate specific mental content during mental imagery. In their recent neurofeedback trial in depression, Young et al. (126) showed that positive autobiographical imagery with neurofeedback from the amygdala selectively increases recall of positive memories, even when controlling for general effects of mental imagery. Other neurofeedback approaches have additionally shown that even specific properties of visual representations can be reinforced by neurofeedback. By providing feedback from pattern of activation (rather than only from decreases or increases in averaged regional brain activation), Shibata et al. (148) found evidence that neurofeedback can induce early visual cortex activation related to basic properties of visual stimuli, even in the absence of visual stimulation. While this study by Shibata et al. (148) demonstrated that neurofeedback can modulate low level features of internally generated visual representations, Taschereau-Dumouchel et al. (149) additionally demonstrated that neurofeedback training can also decrease emotional responses to visual-memory representations of fear inducing animals (see further discussion of this approach in the next section). Overall, the available evidence suggests that neurofeedback can indeed modulate specific mental representations, including perceptual, affective as well as memory components of emotional states.
Prefrontal and parietal control regions implicated in mental imagery are also recruited during most neurofeedback approaches (140, 145), which may reflect the use of mental imagery strategy in neurofeedback training (although other cognitive component processes may also recruit parts of this network). In comparison to mental imagery by itself, however, neurofeedback additionally modulates activation in areas related to reward learning, notably, the striatum (141). While neurofeedback can therefore also be expected to generally reinforce top-down control during mental imagery, it should specifically facilitate interactions between control systems and the neurofeedback target region. Studies investigating changes in brain connectivity have supported this notion by repeatedly showing training-related changes in connectivity between neurofeedback target regions and cortical control regions (150–152). Beyond the general effects of neurofeedback on brain connectivity, contemporary neurofeedback approaches can also target top-down control directly through neurofeedback, for example, by providing feedback from connectivity between control areas and affective core regions (153, 154).
By its very nature, a neurofeedback training can be thought of as a psychological as much as a physiological intervention. Many studies use functional localiser procedures, which aim to capture the psychological processes involved in the disorder, such as anhedonia/reduced reward sensitivity in the case of depression, excessive cue reactivity/salience in the case of addictions or excessive fear responses in the case of anxiety disorders. Furthermore, many protocols involve instructions or explicit mood induction strategies, for example, to engage with positive autobiographical memories (155), or offer patients the opportunity to use cognitive strategies learnt in the course of other treatment programmes to try and aid the self-regulation of disease-relevant circuits. In these approaches, mental imagery is commonly an integral part of the intervention. Participants gain control over the neurofeedback signal by identifying the mental content which most strongly affects the neural processes that is regulated. Finally, some studies have used homework exercises to transfer the mental strategies used in the MRI environment into everyday settings, which may be a crucial step to ensure sustainability of any clinical effects.
There is also often a strong element of personalisation. The functional localisers commonly use material that is relevant to the disease process or the putative functional deficit such as positive affective pictures for localisation of emotionally responsive regions in depression (124), contamination scenes in contamination anxiety (133), pictures of spiders in arachnophobia (132), pictures of food to train regulation of food craving (156), or pictures of addictive cues for use in addiction (157). Although generic stimuli from general picture databases such as the International Affective Pictures System will often be sufficient to induce the desired activation patterns (and accompanying physiological responses, e.g., arousal) for many of these protocols, a personalised approach is preferable. For example, if the aim of the neurofeedback training is to target dysfunctional activation patterns that contribute to clinical symptoms directly, the protocol will generally involve induction of these symptoms during the scanning with personalised stimuli. A classical example is OCD, where patients might be asked to take pictures of the scenarios that trigger their symptoms, which can then be used for symptom induction in the MRI environment (158). This approach is parallel to the use of exposure to triggering events in psychological interventions for PTSD. The use of such (personalised) disease-relevant stimuli is not confined to the functional localiser procedure.
In some protocols, such stimuli (images, scripts) are also presented during the neurofeedback runs. One example is the instruction to downregulate amygdala activity during exposure to trauma words (128). In such a scenario, fMRI-NF is incorporated in a broader programme of personalised extinction therapy. Neurofeedback has also been used to pilot a virtual exposure training that would not involve actual presentation of symptom-provoking stimuli but only the reinforcement of the related brain activation patterns. This approach is based on a method called decoded neurofeedback, which entails identification of multivoxel patterns associated with particular stimuli or mental states, which are then used as neurofeedback target (10). In this decoded neurofeedback approach, participants are generally not explicitly told about specific mental strategies, and indeed the basic concept entails that they have no explicit knowledge of the nature of the brain activation patterns being reinforced. In the virtual exposure training, participants then train to increase the occurrence of brain patterns associated with aversive stimuli (which could have been localised in other participants, obviating the need for ever exposing the patient directly to the aversive stimulus) in an operant conditioning task. A pilot study with this paradigm in people showing high subclinical fear for specific animal categories has yielded promising results in terms of attenuation of arousal responses (149, 159).
Neurofeedback treatments, particularly those employing real-time fMRI feedback, thus intrinsically incorporate elements of psychological therapy (exposure, reappraisal and other cognitive strategies, mental imagery) to varying degrees. Indeed, a key element for any clinical implementation of such a technique would seem to be its integration with psychological therapy and training programmes, both in order to harness synergies and ensure sustainability of any effects.
The research discussed in this review highlights the complexity of the neural systems recruited during mental imagery treatments, but also convergent pathways observed across studies that can be considered as well-validated targets for neurofeedback (Figure 1). This review has focused on the visual system because its involvement in mental imagery has been studied in greatest detail, and most clinical applications of mental imagery focus on the visual domain.
However, extensive evidence also suggests that other forms of imagery such as somatosensory or auditory imagery share a defined neural basis with their respective perceptual system (160–163). Additionally, it has been shown that these imagery modalities also modulate affective experiences and their neural basis (106, 164). Notably, individual differences exist with regard to which imagery modality participants prefer, and these differences are associated with differences in effectiveness of mental strategies (164). Future studies will have to evaluate in how far individual patients react better to certain types of mental imagery treatment. Here, neuroimaging and particularly neurofeedback could help to determine whether a certain type of mental imagery is more effective for modulating a neural target in an individual participant compared to other types of mental imagery. This appears to be especially relevant as a major limitation of mental imagery is that mental imagery success is experienced subjectively and mostly reported retrospectively. Such self-report procedures are generally affected by biases, especially when performed retrospectively (165, 166), while verbal reports during mental imagery might additionally distract patients in their mental operations.
Whereas neurofeedback could potentially constitute an objective measure of mental imagery success in the future, variations in applied mental imagery strategies strongly contribute to the heterogeneity of neurofeedback approaches at the moment. In addition to problems that are unspecific to neurofeedback as issues of statistical practice and transparency, particularly, differences in study design and analysis methods have contributed to a pronounced variability between different neurofeedback approaches with fMRI as well as EEG, making it difficult to draw robust clinical or mechanistic conclusions from existing research (see the study by Ros et al. (167) for a contemporary approach to target these issues).
Importantly, a consensus on which criteria should be applied to evaluate success in neurofeedback studies is still lacking. A recent meta-analysis has here made a notable advancement for fMRI neurofeedback by providing an overview on applied success criteria (11) but this information needs to be further utilised to create meta-analytic evidence. Standardising the applied success criteria will thereby enhance the possibility to compare different mental imagery strategies as well as different neurofeedback approaches.
Additionally, heterogeneity in the choice of neural markers used to construct the neurofeedback protocol constitutes a challenge. Most fMRI neurofeedback studies rely on average signals form single regions of interest (e.g., deCharms et al. (119); Haller et al. (168); Zotev et al. (169); Berman et al. (170); Garrison et al. (171); Greer et al. (150)), but several studies have used feedback from activation of whole networks (172, 173), or indices of connectivity between regions (174–177). So far, it is not known if and under which circumstances patients would benefit from training with one marker of neural activity compared to another. Consequently, it is not known whether training a clinically relevant subprocess with a limited number of associated brain regions should be preferred over training distributed pattern of brain activation that better represent the holistic character of an emotional experiences.
Furthermore, the technical set-up of a neurofeedback system additionally creates variance between studies as it determines which markers of brain activation can be extracted: fMRI is characterised by a poor temporal resolution and can therefore not differentiate between neural events that occur close in time. Furthermore, fMRI neurofeedback represents activation that occurred about six seconds before presentation of the feedback. While this delay itself is not necessarily disadvantageous for gaining control over regional brain activation compared to fast changing EEG neurofeedback (178), it constrains fMRI with regard to the information that can be used to create neurofeedback. It remains to be evaluated whether EEG neurofeedback would be preferable for certain psychiatric conditions in which the temporal information contained in the neurofeedback signal has a crucial impact on the treatment outcome.
While this review focussed on combinations between neurofeedback and mental imagery, another major class of neuromodulation techniques that bear potential for modulating brain systems implicated in mental imagery are brain stimulation approaches. These are technical set-ups that stimulate relevant brain areas using electromagnetic currents. Such neurostimulation techniques can generally be divided into invasive approaches, i.e., approaches that require surgery, and non-invasive approaches, that do not. Considering the risks implicated in neurosurgical procedures such as inflammation (179) and the need for restrictive definition of clinical indications, non-invasive neurostimulation techniques are generally more common. However, non-invasive brain stimulation approaches cannot stimulate deep brain structures reliably, with the possible exception of recently developed techniques for focused transcranial magnetic stimulation (180–182).
Due to the problem that deep brain structures can only be reached with invasive brain stimulation techniques, research is considerably sparse with regard to stimulations of subcortical areas during mental imagery but effects of deep brain stimulations on emotional processes have been demonstrated (183, 184). A major role for neurostimulation would therefor entail modulations of cortical areas that can be penetrated with non-invasive brain stimulation techniques. Several studies have shown that interactions between cortical control regions and lower level visual (185, 186) as well as affective areas (187–190) can be evoked through neuromodulation approaches, and stimulation of the dlPFC has been shown to improve the efficacy of emotion regulation (191). While the mechanistic effects of prefrontal and parietal cortex stimulation remain poorly understood (192), stimulation of lateral prefrontal areas has successfully been implemented as add-on treatment for depression (193, 194), furthermore supporting the strong potential of neuromodulation techniques to contribute to treatments of major psychopathologies. Considering that particularly transcranial-magnetic stimulation (TMS) has been found to be effective for depression, its combination with cognitive behavioural therapy may be promising in this respect (195).
Another direction towards improved interactions between mental imagery and neuromodulation techniques can be expected from approaches that combine neurofeedback with neurostimulation. In such setups a neural target state is achieved by recording brain activation and using information about the current brain state to guide stimulation of brain in order to achieve a certain target state (196–198). In this way, brain stimulation could be used to support the effects of mental imagery during neurofeedback. For example, brain stimulation of limbic areas could help to increase the affective effects of mental images. Moreover, applying brain stimulation and neurofeedback-guided mental imagery successively bears potential. For example, relevant neural systems could first be strengthened with neurostimulation, which does not require active participation of patients. After dysfunctional neural systems have been restabilized, subsequent mental imagery/neurofeedback training could be used to train emotional self-regulation skills and thereby build resilience.
The empirical evidence on mental imagery-based psychotherapeutic interventions supports a role for therapeutic approaches targeting/employing mental imagery in affective and anxiety disorders. As mental imagery can induce emotional experiences by triggering perceptual and memory system components of affective states, while at the same time providing the possibility for goal directed self-regulation, mental imagery can modulate a range of clinically relevant mechanisms. Moreover, a broad range of neuroscientific studies already provides an extensive knowledge based on how the implicated psychological processes are manifested in the brain, opening the door for neuromodulation treatments that facilitate the effects of mental imagery.
From the available neuromodulation approaches, particularly, neurofeedback has already been combined with mental imagery extensively. With the possibility to guide the mental operations to evoke maximal impact on clinically relevant brain systems and the possibility to reinforce associated brain states, neurofeedback provides interesting synergistic opportunities with imagery-based psychological interventions. The beneficial interplay between neuromodulation, cognitive self-regulation, and mental imagery, although this field is still in the early phases of clinical evidence-gathering.
Because mental imagery is one of the most common mental strategies applied during neurofeedback, a much larger amount of empirical research exists for combinations of mental imagery with neurofeedback compared to other neuromodulation techniques. Although neurofeedback protocols often incorporate mental strategies which bear resemblance to psychotherapeutic approaches (such as exposure or positive mental imagery), these parallels have not been exploited so far in a systematic fashion or through combined treatment manuals. Such exploitation remains an attractive task for the collaboration of clinical psychologists, psychiatrists, and neuroscientists.
In conclusion, mental imagery is a core process of many psychological interventions, particularly in affective and anxiety disorders. It has also been a core topic of investigation of cognitive neuroscience, and the neural and psychological mechanisms of mental imagery and its impact on emotional experience have been extensively studied. Based on this knowledge of processes, we can now exploit synergies between imagery and neuroscientific techniques, in order to use the latter to boost the former, or use the former to facilitate the latter. This combination is inherent in many neurofeedback protocols, particularly those employing fMRI signals, but has also been studied with non-invasive brain stimulation. The combination of mental imagery with neuromodulation is conceptually attractive because of the synergistic potential and the potential to achieve more sustainable effects for neurostimulation interventions through transfer to psychological processes.
Yet, the potential treatment combinations are manifold, and the same is true for the neuropsychological subprocesses that could be targeted, so that an extensive body of research is still needed in order to determine the most effective treatment combinations and parameters for this type of neuropsychotherapy.
Both authors designed, drafted and reviewed this review article.
The authors were supported by the BRAINTRAIN grant, a Collaborative Project supported by the European Commission, under the Health Cooperation Work Programme of the 7th Framework Programme, under the Grant Agreement no. 602186 (www.braintrainproject.eu).
The authors declare that the research was conducted in the absence of any commercial or financial relationships that could be construed as a potential conflict of interest.
1. Linden DEJ. How psychotherapy changes the brain–the contribution of functional neuroimaging. Mol Psychiatry (2006) 11(6):528. doi: 10.1038/sj.mp.4001816
2. Frewen PA, Dozois DJ, Lanius RA. Neuroimaging studies of psychological interventions for mood and anxiety disorders: empirical and methodological review. Clin Psychol Rev (2008) 28(2):228–46. doi: 10.1016/j.cpr.2007.05.002
3. Messina I, Sambin M, Palmieri A, Viviani R. Neural correlates of psychotherapy in anxiety and depression: a meta-analysis. PloS One (2013) 8(9):e74657. doi: 10.1371/journal.pone.0074657
4. Barsaglini A, Sartori G, Benetti S, Pettersson-Yeo W, Mechelli A. The effects of psychotherapy on brain function: A systematic and critical review. Prog Neurobiol (2014) 114:1–14. doi: 10.1016/j.pneurobio.2013.10.006
5. Edwards D. Restructuring implicational meaning through memory-based imagery: Some historical notes. J Behav Ther Exp Psychiatry (2007) 38(4):306–16. doi: 10.1016/j.jbtep.2007.10.001
6. Clyne MB. Guided Affective Imagery: Mental Imagery in Short-term Psychotherapy Vol. 1984. New York: Thieme-Stratton (1986). p. 211. doi: 10.1192/S0007125000211021
7. Weiskopf N, Scharnowski F, Veit R, Goebel R, Birbaumer N, Mathiak K. Self-regulation of local brain activity using real-time functional magnetic resonance imaging (fMRI). J Physiol -Paris (2004) 98(4-6):357–73. doi: 10.1016/j.jphysparis.2005.09.019
8. Weiskopf N. Real-time fMRI and its application to neurofeedback. Neuroimage (2012) 62(2):682–92. doi: 10.1016/j.neuroimage.2011.10.009
9. Sulzer J, Haller S, Scharnowski F, Weiskopf N, Birbaumer N, Blefari ML, et al. Real-time fMRI neurofeedback: progress and challenges. Neuroimage (2013) 76:386–99. doi: 10.1016/j.neuroimage.2013.03.033
10. Watanabe T, Sasaki Y, Shibata K, Kawato M. Advances in fMRI Real-Time Neurofeedback. Trends Cogn Sci (2017) 21(12):997–1010. doi: 10.1016/j.tics.2017.09.010
11. Thibault RT, MacPherson A, Lifshitz M, Roth RR, Raz A. Neurofeedback with fMRI: a critical systematic review. Neuroimage (2018) 172:786–807. doi: 10.1016/j.neuroimage.2017.12.071
12. Gerrards-Hesse A, Spies K, Hesse FW. Experimental inductions of emotional states and their effectiveness: A review. Br J Psychol (1994) 85(1):55–78. doi: 10.1111/j.2044-8295.1994.tb02508.x
13. Westermann R, Spies K, Stahl G, Hesse FW. Relative effectiveness and validity of mood induction procedures: A meta-analysis. Eur J Social Psychol (1996) 26(4):557–80. doi: 10.1002/(SICI)1099-0992(199607)26:4<557::AID-EJSP769>3.0.CO;2-4
14. Holmes EA, Mathews A, Mackintosh B, Dalgleish T. The causal effect of mental imagery on emotion assessed using picture-word cues. Emotion (2008b) 8(3):395. doi: 10.1037/1528-3542.8.3.395
15. Holmes EA, Coughtrey AE, Connor A. Looking at or through rose-tinted glasses? Imagery perspective and positive mood. Emotion (2008a) 8(6):875. doi: 10.1037/a0013617
16. Nelis S, Vanbrabant K, Holmes EA, Raes F. Greater positive affect change after mental imagery than verbal thinking in a student sample. J Expl Psychopathol (2012) 3(2):178–88. doi: 10.5127/jep.021111
17. Gotlib IH, Joormann J. Cognition and depression: current status and future directions. Ann Rev Clin Psychol (2010) 6:285–312. doi: 10.1146/annurev.clinpsy.121208.131305
18. Blackwell SE, Browning M, Mathews A, Pictet A, Welch J, Davies J, et al. Positive imagery-based cognitive bias modification as a web-based treatment tool for depressed adults: a randomized controlled trial. Clin Psychol Sci (2015) 3(1):91–111. doi: 10.1177/2167702614560746
19. Borkovec TD, Sides JK. The contribution of relaxation and expectancy to fear reduction via graded, imaginal exposure to feared stimuli. Behav Res Ther (1979) 17(6):529–40. doi: 10.1016/0005-7967(79)90096-2
20. Heimberg RG, Becker RE, Goldfinger K, Vermilyea JA. Treatment of social phobia by exposure, cognitive restructuring and homework assignments. J Nerv Mental Dis (1985) 173(4):236–45. doi: 10.1097/00005053-198504000-00006
21. Wiederhold BK, Jang DP, Gevirtz RG, Kim SI, Kim I-Y, Wiederhold MD. The treatment of fear of flying: a controlled study of imaginal and virtual reality graded exposure therapy. IEEE Trans Inf Technol Biomed (2002) 6(3):218–23. doi: 10.1109/TITB.2002.802378
22. Rentz TO, Powers MB, Smits JA, Cougle JR, Telch MJ. Active-imaginal exposure: Examination of a new behavioral treatment for cynophobia (dog phobia). Behav Res Ther (2003) 41(11):1337–53. doi: 10.1016/S0005-7967(03)00041-X
23. Foa EB, Steketee G, Turner RM, Fischer SC. Effects of imaginal exposure to feared disasters in obsessive-compulsive checkers. Behav Res Ther (1980) 18(5):449–55. doi: 10.1016/0005-7967(80)90010-8
24. Abramowitz JS. Variants of exposure and response prevention in the treatment of obsessive-compulsive disorder: A meta-analysis. Behav Ther (1996) 27(4):583–600. doi: 10.1016/S0005-7894(96)80045-1
25. Vogel PA, Stiles TC, Götestam KG. Adding cognitive therapy elements to exposure therapy for obsessive compulsive disorder: A controlled study. Behav Cognit Psychother (2004) 32(3):275–90. doi: 10.1017/S1352465804001353
26. Tarrier N, Pilgrim H, Sommerfield C, Faragher B, Reynolds M, Graham E, et al. A randomized trial of cognitive therapy and imaginal exposure in the treatment of chronic posttraumatic stress disorder. J Consulting Clin Psychol (1999) 67(1):13. doi: 10.1037/0022-006X.67.1.13
27. Foa EB, Keane TM, Friedman MJ, Cohen JA. Effective treatments for PTSD: practice guidelines from the International Society for Traumatic Stress Studies. New York, NY Guilford Press (2008). doi: 10.1037/e517322011-216
28. Smucker MR, Dancu CV. (1999). Cognitive-behavioral treatment for adult survivors of childhood trauma: Imagery rescripting and reprocessing. Ed. Aronson Jason. Northvale, NJ: Jason Aronson.
29. Ohanian V. Imagery rescripting within cognitive behavior therapy for bulimia nervosa: An illustrative case report. Int J Eating Disord (2002) 31(3):352–7. doi: 10.1002/eat.10044
30. Arntz A, Tiesema M, Kindt M. Treatment of PTSD: A comparison of imaginal exposure with and without imagery rescripting. J Behav Ther Exp Psychiatry (2007) 38(4):345–70. doi: 10.1016/j.jbtep.2007.10.006
31. Holmes EA, Butler G. Imagery and the self following childhood trauma: Observations concerning the use of drawings and external images. In: Imagery and the Threatened Self. London: Routledge (2009). p. 176–90.
32. Arntz A, Sofi D, van Breukelen G. Imagery Rescripting as treatment for complicated PTSD in refugees: A multiple baseline case series study. Behav Res Ther (2013) 51(6):274–83. doi: 10.1016/j.brat.2013.02.009
33. Arbuthnott KD, Arbuthnott DW, Rossiter L. Guided imagery and memory: implications for psychotherapists. J Counseling Psychol (2001) 48(2):123. doi: 10.1037/0022-0167.48.2.123
34. Arbuthnott KD, Geelen CB, Kealy KL. Phenomenal characteristics of guided imagery, natural imagery, and autobiographical memories. Memory Cognit (2002) 30(4):519–28. doi: 10.3758/BF03194953
35. Fors EA, Sexton H, Götestam KG. The effect of guided imagery and amitriptyline on daily fibromyalgia pain: a prospective, randomized, controlled trial. J Psychiatric Res (2002) 36(3):179–87. doi: 10.1016/S0022-3956(02)00003-1
36. Holmes EA, Mathews A. Mental imagery in emotion and emotional disorders. Clin Psychol Rev (2010) 30(3):349–62. doi: 10.1016/j.cpr.2010.01.001
37. Holmes EA, Arntz A, Smucker MR. Imagery rescripting in cognitive behaviour therapy: Images, treatment techniques and outcomes. J Behav Ther Exp Psychiatry (2007) 38(4):297–305. doi: 10.1016/j.jbtep.2007.10.007
38. Stopa L. Imagery Rescripting Across Disorders: A Practical Guide. Cognitive and Behavioral Practice. (2011) 4(18):421–3.
39. Grunert BK, Weis JM, Smucker MR, Christianson HF. Imagery rescripting and reprocessing therapy after failed prolonged exposure for post-traumatic stress disorder following industrial injury. J Behav Ther Exp Psychiatry (2007) 38(4):317–28. doi: 10.1016/j.jbtep.2007.10.005
40. Holmes EA, Lang TJ, Deeprose C. Mental imagery and emotion in treatment across disorders: Using the example of depression. Cognit Behav Ther (2009) 38(S1):21–8. doi: 10.1080/16506070902980729
41. Morina N, Deeprose C, Pusowski C, Schmid M, Holmes EA. Prospective mental imagery in patients with major depressive disorder or anxiety disorders. J Anxiety Disord (2011) 25(8):1032–7. doi: 10.1016/j.janxdis.2011.06.012
42. Fink J, Pflugradt E, Stierle C, Exner C. Changing disgust through imagery rescripting and cognitive reappraisal in contamination-based obsessive-compulsive disorder. J Anxiety Disord (2018) 54:36–48. doi: 10.1016/j.janxdis.2018.01.002
43. Ochsner KN, Gross JJ. The cognitive control of emotion. Trends Cogn Sci (2005) 9(5):242–9. doi: 10.1016/j.tics.2005.03.010
44. Gross JJ, Thompson RA. Emotion regulation: Conceptual foundations. In Gross J. J. (Ed.), Handbook of emotion regulation. New York, NY: Guilford. (2007) pp. 3–24.
45. Smits JA, Julian K, Rosenfield D, Powers MB. Threat reappraisal as a mediator of symptom change in cognitive-behavioral treatment of anxiety disorders: A systematic review. J Consulting Clin Psychol (2012) 80(4):624. doi: 10.1037/a0028957
46. Zilverstand A, Parvaz MA, Goldstein RZ. Neuroimaging cognitive reappraisal in clinical populations to define neural targets for enhancing emotion regulation. A systematic review. Neuroimage (2017) 151:105–16. doi: 10.1016/j.neuroimage.2016.06.009
47. Gross JJ, John OP. Individual differences in two emotion regulation processes: implications for affect, relationships, and well-being. J Personality Social Psychol (2003) 85(2):348. doi: 10.1037/0022-3514.85.2.348
48. Moore SA, Zoellner LA, Mollenholt N. Are expressive suppression and cognitive reappraisal associated with stress-related symptoms? Behav Res Ther (2008) 46(9):993–1000. doi: 10.1016/j.brat.2008.05.001
49. Haga SM, Kraft P, Corby E-K. Emotion regulation: antecedents and well-being outcomes of cognitive reappraisal and expressive suppression in cross-cultural samples. J Happiness Stud (2009) 10(3):271–91. doi: 10.1007/s10902-007-9080-3
50. Davis M. The role of the amygdala in fear and anxiety. Ann Rev Neurosci (1992) 15(1):353–75. doi: 10.1146/annurev.ne.15.030192.002033
51. Adolphs R, Tranel D, Damasio H, Damasio AR. Fear and the human amygdala. J Neurosci (1995) 15(9):5879–91. doi: 10.1523/JNEUROSCI.15-09-05879.1995
52. LeDoux J. The emotional brain, fear, and the amygdala. Cell Mol Neurobiol (2003) 23(4-5):727–38. doi: 10.1023/A:1025048802629
53. Weiskrantz L. Behavioral changes associated with ablation of the amygdaloid complex in monkeys. J Comp Physiol Psychol (1956) 49(4):381. doi: 10.1037/h0088009
54. Blanchard DC, Blanchard RJ. Innate and conditioned reactions to threat in rats with amygdaloid lesions. J Comp Physiol Psychol (1972) 81(2):281. doi: 10.1037/h0033521
55. Royet J-P, Plailly J, Delon-Martin C, Kareken DA, Segebarth C. fMRI of emotional responses to odors:: influence of hedonic valence and judgment, handedness, and gender. Neuroimage (2003) 20(2):713–28. doi: 10.1016/S1053-8119(03)00388-4
56. Small DM, Gregory MD, Mak YE, Gitelman D, Mesulam MM, Parrish T. Dissociation of neural representation of intensity and affective valuation in human gustation. Neuron (2003) 39(4):701–11. doi: 10.1016/S0896-6273(03)00467-7
57. Wicker B, Keysers C, Plailly J, Royet J-P, Gallese V, Rizzolatti G. Both of us disgusted in My insula: the common neural basis of seeing and feeling disgust. Neuron (2003) 40(3):655–64. doi: 10.1016/S0896-6273(03)00679-2
58. Blair R, Morris JS, Frith CD, Perrett DI, Dolan RJ. Dissociable neural responses to facial expressions of sadness and anger. Brain (1999) 122(5):883–93. doi: 10.1093/brain/122.5.883
59. Drexler K, Schweitzer JB, Quinn CK, Gross R, Ely TD, Muhammad F, et al. Neural activity related to anger in cocaine-dependent men: a possible link to violence and relapse. Am J Addict (2000) 9(4):331–9. doi: 10.1080/105504900750047382
60. Kober H, Barrett LF, Joseph J, Bliss-Moreau E, Lindquist K, Wager TD. Functional grouping and cortical–subcortical interactions in emotion: a meta-analysis of neuroimaging studies. Neuroimage (2008) 42(2):998–1031. doi: 10.1016/j.neuroimage.2008.03.059
61. Lindquist KA, Wager TD, Kober H, Bliss-Moreau E, Barrett LF. The brain basis of emotion: a meta-analytic review. Behav Brain Sci (2012) 35(3):121–43. doi: 10.1017/S0140525X11000446
62. Barrett LF. Variety is the spice of life: A psychological construction approach to understanding variability in emotion. Cognit Emotion (2009) 23(7):1284–306. doi: 10.1080/02699930902985894
63. Lindquist KA, Barrett LF. A functional architecture of the human brain: emerging insights from the science of emotion. Trends Cogn Sci (2012) 16(11):533–40. doi: 10.1016/j.tics.2012.09.005
64. Liberzon I, Phan KL, Decker LR, Taylor SF. Extended amygdala and emotional salience: a PET activation study of positive and negative affect. Neuropsychopharmacology (2003) 28(4):726–33. doi: 10.1038/sj.npp.1300113
65. Cunningham WA, Brosch T. Motivational salience: amygdala tuning from traits, needs, values, and goals. Curr Directions Psychol Sci (2012) 21(1):54–9. doi: 10.1177/0963721411430832
66. Phan KL, Wager T, Taylor SF, Liberzon I. Functional neuroanatomy of emotion: a meta-analysis of emotion activation studies in PET and fMRI. Neuroimage (2002) 16(2):331–48. doi: 10.1006/nimg.2002.1087
67. Murphy FC, Nimmo-Smith I, Lawrence AD. Functional neuroanatomy of emotions: a meta-analysis. Cognit Affective Behav Neurosci (2003) 3(3):207–33. doi: 10.3758/CABN.3.3.207
68. Wager TD, Phan KL, Liberzon I, Taylor SF. Valence, gender, and lateralization of functional brain anatomy in emotion: a meta-analysis of findings from neuroimaging. Neuroimage (2003) 19(3):513–31. doi: 10.1016/S1053-8119(03)00078-8
69. Storbeck J, Clore GL. On the interdependence of cognition and emotion. Cognit Emotion (2007) 21(6):1212–37. doi: 10.1080/02699930701438020
70. Duncan S, Barrett LF. Affect is a form of cognition: A neurobiological analysis. Cognit Emotion (2007) 21(6):1184–211. doi: 10.1080/02699930701437931
71. Squire LR, Zola-Morgan S. The medial temporal lobe memory system. Science (1991) 253(5026):1380–6. doi: 10.1126/science.1896849
72. Dolcos F, LaBar KS, Cabeza R. Interaction between the amygdala and the medial temporal lobe memory system predicts better memory for emotional events. Neuron (2004) 42(5):855–63. doi: 10.1016/S0896-6273(04)00289-2
73. Squire LR, Stark CE, Clark RE. The medial temporal lobe. Annu Rev Neurosci (2004) 27:279–306. doi: 10.1146/annurev.neuro.27.070203.144130
74. Eichenbaum H, Yonelinas AP, Ranganath C. The medial temporal lobe and recognition memory. Annu Rev Neurosci (2007) 30:123–52. doi: 10.1146/annurev.neuro.30.051606.094328
75. Saarimäki H, Gotsopoulos A, Jääskeläinen IP, Lampinen J, Vuilleumier P, Hari R, et al. Discrete neural signatures of basic emotions. Cereb Cortex (2015) 26(6):2563–73. doi: 10.1093/cercor/bhv086
76. Hendler T, Rotshtein P, Hadar U. Emotion–perception interplay in the visual cortex:”the eyes follow the heart”. Cell Mol Neurobiol (2001) 21(6):733–52. doi: 10.1023/A:1015156222101
77. Rotshtein P, Malach R, Hadar U, Graif M, Hendler T. Feeling or features: different sensitivity to emotion in high-order visual cortex and amygdala. Neuron (2001) 32(4):747–57. doi: 10.1016/S0896-6273(01)00513-X
78. Bradley MM, Sabatinelli D, Lang PJ, Fitzsimmons JR, King W, Desai P. Activation of the visual cortex in motivated attention. Behav Neurosci (2003) 117(2):369–80. doi: 10.1037/0735-7044.117.2.369
79. Holmes EA, Mathews A. Mental imagery and emotion: a special relationship? Emotion (2005) 5(4):489. doi: 10.1037/1528-3542.5.4.489
80. Pearson J, Naselaris T, Holmes EA, Kosslyn SM. Mental imagery: functional mechanisms and clinical applications. Trends Cogn Sci (2015) 19(10):590–602. doi: 10.1016/j.tics.2015.08.003
81. D’Esposito M, Detre JA, Aguirre GK, Stallcup M, Alsop DC, Tippet LJ, et al. A functional MRI study of mental image generation. Neuropsychologia (1997) 35(5):725–30. doi: 10.1016/S0028-3932(96)00121-2
82. Goebel R, Khorram-Sefat D, Muckli L, Hacker H, Singer W. The constructive nature of vision: direct evidence from functional magnetic resonance imaging studies of apparent motion and motion imagery. Eur J Neurosci (1998) 10(5):1563–73. doi: 10.1046/j.1460-9568.1998.00181.x
83. Knauff M, Kassubek J, Mulack T, Greenlee MW. Cortical activation evoked by visual mental imagery as measured by fMRI. Neuroreport (2000) 11(18):3957–62. doi: 10.1097/00001756-200012180-00011
84. Ishai A, Haxby JV, Ungerleider LG. Visual imagery of famous faces: effects of memory and attention revealed by fMRI. Neuroimage (2002) 17(4):1729–41. doi: 10.1006/nimg.2002.1330
85. Cui X, Jeter CB, Yang D, Montague PR, Eagleman DM. Vividness of mental imagery: individual variability can be measured objectively. Vision Res (2007) 47(4):474–8. doi: 10.1016/j.visres.2006.11.013
86. Reddy L, Tsuchiya N, Serre T. Reading the mind’s eye: Decoding category information during mental imagery. NeuroImage (2010) 50(2):818–25. doi: 10.1016/j.neuroimage.2009.11.084
87. Goldenberg G, Muellbacher W, Nowak A. Imagery without perception—a case study of anosognosia for cortical blindness. Neuropsychologia (1995) 33(11):1373–82. doi: 10.1016/0028-3932(95)00070-J
88. Bridge H, Harrold S, Holmes EA, Stokes M, Kennard C. Vivid visual mental imagery in the absence of the primary visual cortex. J Neurol (2012) 259(6):1062–70. doi: 10.1007/s00415-011-6299-z
89. Salas Riquelme CE, Radovic D, Castro O, Turnbull OH. Internally and externally generated emotions in people with acquired brain injury: preservation of emotional experience after right hemisphere lesions. Front Psychol (2015) 6(101). doi: 10.3389/fpsyg.2015.00101
90. LeDoux J (1998). The emotional brain: The mysterious underpinnings of emotional life. NewYork, NY: Simon and Schuster.
91. Rosen JB, Schulkin J. From normal fear to pathological anxiety. Psychol Rev (1998) 105(2):325. doi: 10.1037/0033-295X.105.2.325
92. Öhman A, Flykt A, Esteves F. Emotion drives attention: detecting the snake in the grass. J Exp Psychol General (2001) 130(3):466. doi: 10.1037/0096-3445.130.3.466
93. Öhman A. The role of the amygdala in human fear: automatic detection of threat. Psychoneuroendocrinology (2005) 30(10):953–8. doi: 10.1016/j.psyneuen.2005.03.019
94. Lang PJ, Melamed BG, Hart J. A psychophysiological analysis of fear modification using an automated desensitization procedure. J Abnormal Psychol (1970) 76(2):220–34. doi: 10.1037/h0029875
95. Hunt M, Fenton M. Imagery rescripting versus in vivo exposure in the treatment of snake fear. J Behav Ther Exp Psychiatry (2007) 38(4):329–44. doi: 10.1016/j.jbtep.2007.09.001
96. Ochsner KN, Silvers JA, Buhle JT. Functional imaging studies of emotion regulation: a synthetic review and evolving model of the cognitive control of emotion. Ann N Y Acad Sci (2012) 1251(1):E1–E24. doi: 10.1111/j.1749-6632.2012.06751.x
97. Arntz A, de Groot C, Kindt M. Emotional memory is perceptual. J Behav Ther Exp Psychiatry (2005) 36(1):19–34. doi: 10.1016/j.jbtep.2004.11.003
98. Botzung A, Denkova E, Manning L. Experiencing past and future personal events: Functional neuroimaging evidence on the neural bases of mental time travel. Brain Cognit (2008) 66(2):202–12. doi: 10.1016/j.bandc.2007.07.011
99. Gerlach KD, Spreng RN, Madore KP, Schacter DL. Future planning: default network activity couples with frontoparietal control network and reward-processing regions during process and outcome simulations. Social Cognit Affective Neurosci (2014) 9(12):1942–51. doi: 10.1093/scan/nsu001
100. Benoit RG, Schacter DL. Specifying the core network supporting episodic simulation and episodic memory by activation likelihood estimation. Neuropsychologia (2015) 75:450–7. doi: 10.1016/j.neuropsychologia.2015.06.034
101. Hackmann A, Holmes E. Reflecting on imagery: a clinical perspective and overview of the special issue of memory on mental imagery and memory in psychopathology. Memory (2004) 12(4):389–402. doi: 10.1080/09658210444000133
102. Whalley MG, Kroes MC, Huntley Z, Rugg MD, Davis SW, Brewin CR. An fMRI investigation of posttraumatic flashbacks. Brain Cognit (2013) 81(1):151–9. doi: 10.1016/j.bandc.2012.10.002
103. Aviezer H, Bentin S, Dudarev V, Hassin RR. The automaticity of emotional face-context integration. Emotion (2011) 11(6):1406. doi: 10.1037/a0023578
104. Hassin RR, Aviezer H, Bentin S. Inherently ambiguous: Facial expressions of emotions, in context. Emotion Rev (2013) 5(1):60–5. doi: 10.1177/1754073912451331
105. Maren S, Phan KL, Liberzon I. The contextual brain: implications for fear conditioning, extinction and psychopathology. Nat Rev Neurosci (2013) 14:417. doi: 10.1038/nrn3492
106. Engen H, Kanske P, Singer T. The neural component-process architecture of endogenously generated emotion. Social Cognit Affective Neurosci (2016) 12(2):197–211. doi: 10.1093/scan/nsw108
107. Stawarczyk D, Jeunehomme O, D’argembeau A. Differential contributions of default and dorsal attention networks to remembering thoughts and external stimuli from real-life events. Cereb Cortex (2017) 28(11):4023–35. doi: 10.1093/cercor/bhx270
108. Beauregard M, Lévesque J, Bourgouin P. Neural correlates of conscious self-regulation of emotion. J Neurosci (2001) 21(18):RC165. doi: 10.1523/JNEUROSCI.21-18-j0001.2001
109. Ochsner KN, Bunge SA, Gross JJ, Gabrieli JD. Rethinking feelings: an FMRI study of the cognitive regulation of emotion. J Cognit Neurosci (2002) 14(8):1215–29. doi: 10.1162/089892902760807212
110. Buhle JT, Silvers JA, Wager TD, Lopez R, Onyemekwu C, Kober H, et al. Cognitive reappraisal of emotion: a meta-analysis of human neuroimaging studies. Cereb Cortex (2014) 24(11):2981–90. doi: 10.1093/cercor/bht154
111. Ochsner KN, Gross JJ. Cognitive emotion regulation: Insights from social cognitive and affective neuroscience. Curr Directions Psychol Sci (2008) 17(2):153–8. doi: 10.1111/j.1467-8721.2008.00566.x
112. Etkin A, Egner T, Kalisch R. Emotional processing in anterior cingulate and medial prefrontal cortex. Trends Cogn Sci (2011) 15(2):85–93. doi: 10.1016/j.tics.2010.11.004
113. Kamiya J. The first communications about operant conditioning of the EEG. J Neurother (2011) 15(1):65–73. doi: 10.1080/10874208.2011.545764
114. Nunez P, Silberstein R, Cadusch P, Wijesinghe R, Westdorp A, Srinivasan R. A theoretical and experimental study of high resolution EEG based on surface Laplacians and cortical imaging. Electroencephalogr Clin Neurophysiol (1994) 90(1):40–57. doi: 10.1016/0013-4694(94)90112-0
115. Babilonia F, Cincottia F, Carduccia F, Rossinid PM, Babilonia C. Spatial enhancement of EEG data by surface Laplacian estimation: the use of magnetic resonance imaging-based head models. Clin Neurophysiol (2001) 112(724):727. doi: 10.1016/S1388-2457(01)00494-1
116. Burle B, Spieser L, Roger C, Casini L, Hasbroucq T, Vidal F. Spatial and temporal resolutions of EEG: Is it really black and white? A scalp current density view. Int J Psychophysiol (2015) 97(3):210–20. doi: 10.1016/j.ijpsycho.2015.05.004
117. Keynan JN, Cohen A, Jackont G, Green N, Goldway N, Davidov A, et al. Electrical fingerprint of the amygdala guides neurofeedback training for stress resilience. Nat Hum Behav (2019) 3(1):63–73. doi: 10.1038/s41562-018-0484-3
118. Auer D, Auer L. Functional magnetic resonance imaging in clinical neuroscience: Current state and future prospects. In: Minimally Invasive Techniques for Neurosurgery. Berlin, Heidelberg: Springer (1998). p. 237–42. doi: 10.1007/978-3-642-58731-3_40
119. de Charms R, Maeda F, Glover G, Ludlow D, Pauly J, Soneji D, et al. Control over brain activation and pain learned by using real-time functional MRI. Proc Natl Acad Sci U S A (2005) 102(51):18626–31. doi: 10.1073/pnas.0505210102
120. Subramanian L, Hindle JV, Johnston S, Roberts MV, Husain M, Goebel R, et al. Real-time functional magnetic resonance imaging neurofeedback for treatment of Parkinson’s disease. J Neurosci (2011) 31(45):16309–17. doi: 10.1523/JNEUROSCI.3498-11.2011
121. Subramanian L, Morris MB, Brosnan M, Turner DL, Morris HR, Linden DEJ. Functional Magnetic Resonance Imaging Neurofeedback-guided Motor Imagery Training and Motor Training for Parkinson’s Disease: Randomized Trial. Front Behav Neurosci (2016) 10:111. doi: 10.3389/fnbeh.2016.00111
122. Papoutsi M, Weiskopf N, Langbehn D, Reilmann R, Rees G, Tabrizi SJ. Stimulating neural plasticity with real-time fMRI neurofeedback in Huntington’s disease: A proof of concept study. Hum Brain Mapp (2018) 39(3):1339–53. doi: 10.1002/hbm.23921
123. Johnston S, Linden DEJ, Healy D, Goebel R, Habes I, Boehm SG. Upregulation of emotion areas through neurofeedback with a focus on positive mood. Cognit Affective Behav Neurosci (2011) 11(1):44–51. doi: 10.3758/s13415-010-0010-1
124. Linden DEJ, Habes I, Johnston SJ, Linden SC, Tatineni R, Subramanian L, et al. Real-time self-regulation of emotion networks in patients with depression. PloS one (2012) 7(6):e38115. doi: 10.1371/journal.pone.0038115
125. Mehler DMA, Sokunbi MO, Habes I, Barawi K, Subramanian L, Range M, et al. Targeting the affective brain-a randomized controlled trial of real-time fMRI neurofeedback in patients with depression. Neuropsychopharmacology (2018) 43(13):2578. doi: 10.1038/s41386-018-0126-5
126. Young KD, Siegle GJ, Zotev V, Phillips R, Misaki M, Yuan H, et al. Randomized clinical trial of real-time fMRI amygdala neurofeedback for major depressive disorder: effects on symptoms and autobiographical memory recall. Am J Psychiatry (2017b) 174(8):748–55. doi: 10.1176/appi.ajp.2017.16060637
127. Gerin MI, Fichtenholtz H, Roy A, Walsh CJ, Krystal JH, Southwick S, et al. Real-Time fMRI Neurofeedback with War Veterans with Chronic PTSD: A Feasibility Study. Front Psychiatry (2016) 7:111. doi: 10.3389/fpsyt.2016.00111
128. Nicholson AA, Rabellino D, Densmore M, Frewen PA, Paret C, Kluetsch R, et al. The neurobiology of emotion regulation in posttraumatic stress disorder: amygdala downregulation via real-time fMRI neurofeedback. Hum Brain Mapp (2017) 38(1):541–60. doi: 10.1002/hbm.23402
129. Misaki M, Phillips R, Zotev V, Wong CK, Wurfel BE, Krueger F, et al. Real-time fMRI amygdala neurofeedback positive emotional training normalized resting-state functional connectivity in combat veterans with and without PTSD: a connectome-wide investigation. Neuroimage Clin (2018) 20:543–55. doi: 10.1016/j.nicl.2018.08.025
130. Nicholson AA, Rabellino D, Densmore M, Frewen PA, Paret C, Kluetsch R, et al. Intrinsic connectivity network dynamics in PTSD during amygdala downregulation using real-time fMRI neurofeedback: A preliminary analysis. Hum Brain Mapp (2018) 39(11):4258–75. doi: 10.1002/hbm.24244
131. Zweerings J, Pflieger EM, Mathiak KA, Zvyagintsev M, Kacela A, Flatten G, et al. Impaired Voluntary Control in PTSD: Probing Self-Regulation of the ACC With Real-Time fMRI. Front Psychiatry (2018) 9:219. doi: 10.3389/fpsyt.2018.00219
132. Zilverstand A, Sorger B, Sarkheil P, Goebel R. fMRI neurofeedback facilitates anxiety regulation in females with spider phobia. Front Behav Neurosci (2015) 9:148. doi: 10.3389/fnbeh.2015.00148
133. Scheinost D, Stoica T, Saksa J, Papademetris X, Constable RT, Pittenger C, et al. Orbitofrontal cortex neurofeedback produces lasting changes in contamination anxiety and resting-state connectivity. Transl Psychiatry (2013) 3:e250. doi: 10.1038/tp.2013.24
134. Hartwell KJ, Prisciandaro JJ, Borckardt J, Li X, George MS, Brady KT. Real-time fMRI in the treatment of nicotine dependence: a conceptual review and pilot studies. Psychol Addict Behav (2013) 27(2):501–9. doi: 10.1037/a0028215
135. Fovet T, Jardri R, Linden DEJ. Current Issues in the Use of fMRI-Based Neurofeedback to Relieve Psychiatric Symptoms. Curr Pharm Des (2015) 21(23):3384–94. doi: 10.2174/1381612821666150619092540
136. Karch S, Keeser D, Hümmer S, Paolini M, Kirsch V, Karali T, et al. Modulation of Craving Related Brain Responses Using Real-Time fMRI in Patients with Alcohol Use Disorder. PLoS One (2015) 10(7):e0133034. doi: 10.1371/journal.pone.0133034
137. Kirsch M, Gruber I, Ruf M, Kiefer F, Kirsch P. Real-time functional magnetic resonance imaging neurofeedback can reduce striatal cue-reactivity to alcohol stimuli. Addict Biol (2016) 21(4):982–92. doi: 10.1111/adb.12278
138. Cox WM, Subramanian L, Linden DEJ, Lührs M, McNamara R, Playle R, et al. Neurofeedback training for alcohol dependence versus treatment as usual: study protocol for a randomized controlled trial. Trials (2016) 17(1):480. doi: 10.1186/s13063-016-1607-7
139. Gerchen MF, Kirsch M, Bahs N, Halli P, Gerhardt S, Schäfer A, et al. The SyBil-AA real-time fMRI neurofeedback study: protocol of a single-blind randomized controlled trial in alcohol use disorder. BMC Psychiatry (2018) 18(1):12. doi: 10.1186/s12888-018-1604-3
140. Emmert K, Kopel R, Sulzer J, Brühl AB, Berman BD, Linden DEJ, et al. Meta-analysis of real-time fMRI neurofeedback studies using individual participant data: How is brain regulation mediated? Neuroimage (2016) 124:806–12. doi: 10.1016/j.neuroimage.2015.09.042
141. Skottnik L, Sorger B, Kamp T, Linden DEJ, Goebel R. Success and failure of controlling the real-time functional magnetic resonance imaging neurofeedback signal are reflected in the striatum. Brain Behav (2019) 9(3):e01240. doi: 10.1002/brb3.1240
142. Young KD, Zotev V, Phillips R, Misaki M, Yuan H, Drevets WC, et al. Real-time FMRI neurofeedback training of amygdala activity in patients with major depressive disorder. PloS One (2014) 9(2):e88785. doi: 10.1371/journal.pone.0088785
143. Paret C, Kluetsch R, Ruf M, Demirakca T, Hoesterey S, Ende G, et al. Down-regulation of amygdala activation with real-time fMRI neurofeedback in a healthy female sample. Front Behav Neurosci (2014) 8(299). doi: 10.3389/fnbeh.2014.00299
144. Herwig U, Lutz J, Scherpiet S, Scheerer H, Kohlberg J, Opialla S, et al. Training emotion regulation through real-time fMRI neurofeedback of amygdala activity. NeuroImage (2019) 184:687–96. doi: 10.1016/j.neuroimage.2018.09.068
145. Marchesotti S, Martuzzi R, Schurger A, Blefari ML, del Millán JR, Bleuler H, et al. Cortical and subcortical mechanisms of brain-machine interfaces. Hum Brain Mapp (2017) 38(6):2971–89. doi: 10.1002/hbm.23566
146. Paret C, Zähringer J, Ruf M, Gerchen MF, Mall S, Hendler T, et al. Monitoring and control of amygdala neurofeedback involves distributed information processing in the human brain. Hum Brain Mapp (2018) 39(7):3018–31. doi: 10.1002/hbm.24057
147. Sitaram R, Ros T, Stoeckel L, Haller S, Scharnowski F, Lewis-Peacock J, et al. Closed-loop brain training: the science of neurofeedback. Nat Rev Neurosci (2017) 18(2):86. doi: 10.1038/nrn.2016.164
148. Shibata K, Watanabe T, Sasaki Y, Kawato M. Perceptual learning incepted by decoded fMRI neurofeedback without stimulus presentation. Science (2011) 334(6061):1413–5. doi: 10.1126/science.1212003
149. Taschereau-Dumouchel V, Cortese A, Chiba T, Knotts J, Kawato M, Lau H. Towards an unconscious neural reinforcement intervention for common fears. Proc Natl Acad Sci (2018a) 115(13):3470–5. doi: 10.1073/pnas.1721572115
150. Greer SM, Trujillo AJ, Glover GH, Knutson B. Control of nucleus accumbens activity with neurofeedback. NeuroImage (2014) 96:237–44. doi: 10.1016/j.neuroimage.2014.03.073
151. Paret C, Kluetsch R, Zaehringer J, Ruf M, Demirakca T, Bohus M, et al. Alterations of amygdala-prefrontal connectivity with real-time fMRI neurofeedback in BPD patients. Social Cognit Affective Neurosci (2016a) 11(6):952–60. doi: 10.1093/scan/nsw016
152. Paret C, Ruf M, Gerchen MF, Kluetsch R, Demirakca T, Jungkunz M, et al. fMRI neurofeedback of amygdala response to aversive stimuli enhances prefrontal–limbic brain connectivity. Neuroimage (2016b) 125:182–8. doi: 10.1016/j.neuroimage.2015.10.027
153. Koush Y, Meskaldji D-E, Pichon S, Rey G, Rieger SW, Linden DEJ, et al. Learning control over emotion networks through connectivity-based neurofeedback. Cereb Cortex (2015) 27(2):1193–202. doi: 10.1093/cercor/bhv311
154. Zhao Z, Yao S, Li K, Sindermann C, Zhou F, Zhao W, et al. Real-Time Functional Connectivity-Informed Neurofeedback of Amygdala-Frontal Pathways Reduces Anxiety. Psychother Psychosom (2019) 88(1):5–15. doi: 10.1159/000496057
155. Young KD, Misaki M, Harmer CJ, Victor T, Zotev V, Phillips R, et al. Real-Time Functional Magnetic Resonance Imaging Amygdala Neurofeedback Changes Positive Information Processing in Major Depressive Disorder. Biol Psychol (2017a) 82(8), 578–586. doi: 10.1016/j.biopsych.2017.03.013
156. Ihssen N, Sokunbi MO, Lawrence AD, Lawrence NS, Linden DEJ. Neurofeedback of visual food cue reactivity: a potential avenue to alter incentive sensitization and craving. Brain Imaging Behav (2016) 11(3):915–24. doi: 10.1007/s11682-016-9558-x
157. Sokunbi MO, Linden DEJ, Habes I, Johnston S, Ihssen N. Real-time fMRI brain-computer interface: development of a “motivational feedback” subsystem for the regulation of visual cue reactivity. Front Behav Neurosci (2014) 8:392. doi: 10.3389/fnbeh.2014.00392
158. De Putter LM, Van Yper L, Koster EH. Obsessions and compulsions in the lab: A meta-analysis of procedures to induce symptoms of obsessive-compulsive disorder. Clin Psychol Rev (2017) 52:137–47. doi: 10.1016/j.cpr.2017.01.001
159. Taschereau-Dumouchel V, Liu KY, Lau H. Unconscious Psychological Treatments for Physiological Survival Circuits. Curr Opin Behav Sci (2018b) 24:62–8. doi: 10.1016/j.cobeha.2018.04.010
160. Yoo S-S, Freeman DK, McCarthy JJ III, Jolesz FA. Neural substrates of tactile imagery: a functional MRI study. Neuroreport (2003) 14(4):581–5. doi: 10.1097/00001756-200303240-00011
161. Zatorre RJ, Halpern AR. Mental Concerts: Musical Imagery and Auditory Cortex. Neuron (2005) 47(1):9–12. doi: 10.1016/j.neuron.2005.06.013
162. Daselaar SM, Porat Y, Huijbers W, Pennartz CMA. Modality-specific and modality-independent components of the human imagery system. NeuroImage (2010) 52(2):677–85. doi: 10.1016/j.neuroimage.2010.04.239
163. Schmidt TT, Blankenburg F. The somatotopy of mental tactile imagery. Front Hum Neurosci (2019) 13:10. doi: 10.3389/fnhum.2019.00010
164. Engen H, Kanske P, Singer T. Endogenous emotion generation ability is associated with the capacity to form multimodal internal representations. Psychother Psychosom (2018) 8(1):1953. doi: 10.1038/s41598-018-20380-7
165. Sato H, Kawahara J.-i. Selective bias in retrospective self-reports of negative mood states. Anxiety Stress Coping (2011) 24(4):359–67. doi: 10.1080/10615806.2010.543132
166. Mill A, Realo A, Allik J. Retrospective ratings of emotions: the effects of age, daily tiredness, and personality. Front Psychol (2016) 6:2020ss. doi: 10.3389/fpsyg.2015.02020
167. Ros T, Enriquez-Geppert S, Zotev V, Young K, Wood G, Whitfield-Gabrieli S, et al. Consensus on the reporting and experimental design of clinical and cognitive-behavioural neurofeedback studies. PsyArXiv. (2019) doi: 10.31234/osf.io/nyx84
168. Haller S, Birbaumer N, Veit R. Real-time fMRI feedback training may improve chronic tinnitus. Eur Radiol (2010) 20(3):696–703. doi: 10.1007/s00330-009-1595-z
169. Zotev V, Krueger F, Phillips R, Alvarez RP, Simmons WK, Bellgowan P, et al. Self-regulation of amygdala activation using real-time fMRI neurofeedback. PloS One (2011) 6(9):e24522. doi: 10.1371/journal.pone.0024522
170. Berman BD, Horovitz SG, Hallett M. Modulation of functionally localized right insular cortex activity using real-time fMRI-based neurofeedback. Front Hum Neurosci (2013) 7:638. doi: 10.3389/fnhum.2013.00638
171. Garrison K, Santoyo J, Davis J, Thornhill T, Kerr C, Brewer J. Effortless awareness: using real time neurofeedback to investigate correlates of posterior cingulate cortex activity in meditators’ self-report. Front Hum Neurosci (2013) 7:440. doi: 10.3389/fnhum.2013.00440
172. McDonald AR, Muraskin J, Dam NTV, Froehlich C, Puccio B, Pellman J, et al. The real-time fMRI neurofeedback based stratification of default network regulation neuroimaging data repository. NeuroImage (2017) 146:157–70. doi: 10.1016/j.neuroimage.2016.10.048
173. Skouras S, Scharnowski F. The effects of psychiatric history and age on self-regulation of the default mode network. NeuroImage (2019) 198:150–9.
174. Hwang H-J, Kim K-H, Jung Y-J, Kim D-W, Lee Y-H, Im C-H. An EEG-based real-time cortical functional connectivity imaging system. Med Biol Eng Comp (2011) 49(9):985–95. doi: 10.1007/s11517-011-0791-6
175. Pineda J, Juavinett A, Datko M. Self-regulation of brain oscillations as a treatment for aberrant brain connections in children with autism. Med Hypotheses (2012) 79(6):790–8. doi: 10.1016/j.mehy.2012.08.031
176. Liew S-L, Rana M, Cornelsen S, Fortunato de Barros Filho M, Birbaumer N, Sitaram R, et al. Improving motor corticothalamic communication after stroke using real-time fMRI connectivity-based neurofeedback. Neurorehabilitation Neural Repair (2016) 30(7):671–5. doi: 10.1177/1545968315619699
177. Yamashita A, Hayasaka S, Kawato M, Imamizu H. Connectivity neurofeedback training can differentially change functional connectivity and cognitive performance. Cereb Cortex (2017) 27(10):4960–70. doi: 10.1093/cercor/bhx177
178. Perronnet L, Lécuyer A, Mano M, Bannier E, Lotte F, Clerc M, et al. Unimodal versus bimodal EEG-fMRI neurofeedback of a motor imagery task. Front Hum Neurosci (2017) 11:193. doi: 10.3389/fnhum.2017.00193
179. Fenoy AJ, Simpson RK. Risks of common complications in deep brain stimulation surgery: management and avoidance. J Neurosurg (2014) 120(1):132–9. doi: 10.3171/2013.10.JNS131225
180. Zangen A, Roth Y, Voller B, Hallett M. Transcranial magnetic stimulation of deep brain regions: evidence for efficacy of the H-coil. Clin Neurophysiol (2005) 116(4):775–9. doi: 10.1016/j.clinph.2004.11.008
181. Roth Y, Amir A, Levkovitz Y, Zangen A. Three-dimensional distribution of the electric field induced in the brain by transcranial magnetic stimulation using figure-8 and deep H-coils. J Clin Neurophysiol (2007) 24(1):31–8. doi: 10.1097/WNP.0b013e31802fa393
182. Bersani FS, Minichino A, Enticott PG, Mazzarini L, Khan N, Antonacci G, et al. Deep transcranial magnetic stimulation as a treatment for psychiatric disorders: a comprehensive review. Eur Psychiatry (2013) 28(1):30–9. doi: 10.1016/j.eurpsy.2012.02.006
183. Schlaepfer TE, Cohen MX, Frick C, Kosel M, Brodesser D, Axmacher N, et al. Deep brain stimulation to reward circuitry alleviates anhedonia in refractory major depression. Neuropsychopharmacology (2008) 33(2):368. doi: 10.1038/sj.npp.1301408
184. Bewernick BH, Hurlemann R, Matusch A, Kayser S, Grubert C, Hadrysiewicz B, et al. Nucleus accumbens deep brain stimulation decreases ratings of depression and anxiety in treatment-resistant depression. Biol Psychiatry (2010) 67(2):110–6. doi: 10.1016/j.biopsych.2009.09.013
185. Ruff CC, Blankenburg F, Bjoertomt O, Bestmann S, Freeman E, Haynes J-D, et al. Concurrent TMS-fMRI and psychophysics reveal frontal influences on human retinotopic visual cortex. Curr Biol (2006) 16(15):1479–88. doi: 10.1016/j.cub.2006.06.057
186. Ruff CC, Bestmann S, Blankenburg F, Bjoertomt O, Josephs O, Weiskopf N, et al. Distinct causal influences of parietal versus frontal areas on human visual cortex: evidence from concurrent TMS–fMRI. Cereb Cortex (2007) 18(4):817–27. doi: 10.1093/cercor/bhm128
187. Baeken C, De Raedt R, Van Schuerbeek P, Vanderhasselt M-A, De Mey J, Bossuyt A, et al. Right prefrontal HF-rTMS attenuates right amygdala processing of negatively valenced emotional stimuli in healthy females. Behav Brain Res (2010) 214(2):450–5. doi: 10.1016/j.bbr.2010.06.029
188. De Raedt R, Leyman L, Baeken C, Van Schuerbeek P, Luypaert R, Vanderhasselt M-A, et al. Neurocognitive effects of HF-rTMS over the dorsolateral prefrontal cortex on the attentional processing of emotional information in healthy women: an event-related fMRI study. Biological psychology (2010) 85(3):487–95. doi: 10.1016/j.biopsycho.2010.09.015
189. Zwanzger P, Steinberg C, Rehbein MA, Bröckelmann A-K, Dobel C, Zavorotnyy M, et al. Inhibitory repetitive transcranial magnetic stimulation (rTMS) of the dorsolateral prefrontal cortex modulates early affective processing. Neuroimage (2014) 101:193–203. doi: 10.1016/j.neuroimage.2014.07.003
190. Conson M, Errico D, Mazzarella E, Giordano M, Grossi D, Trojano L. Transcranial electrical stimulation over dorsolateral prefrontal cortex modulates processing of social cognitive and affective information. PLoS One (2015) 10(5):e0126448. doi: 10.1371/journal.pone.0126448
191. Feeser M, Prehn K, Kazzer P, Mungee A, Bajbouj M. Transcranial direct current stimulation enhances cognitive control during emotion regulation. Brain Stimulation (2014) 7(1):105–12. doi: 10.1016/j.brs.2013.08.006
192. De Raedt R, Vanderhasselt M-A, Baeken C. Neurostimulation as an intervention for treatment resistant depression: From research on mechanisms towards targeted neurocognitive strategies. Clin Psychol Rev (2015) 41:61–9. doi: 10.1016/j.cpr.2014.10.006
193. Lisanby SH, Kinnunen LH, Crupain MJ. Applications of TMS to therapy in psychiatry. J Clin Neurophysiol (2002) 19(4):344–60. doi: 10.1097/00004691-200208000-00007
194. Rasmussen KG. Evidence for electroconvulsive therapy efficacy in mood disorders. In: Electroconvulsive and Neuromodulation Therapies. Cambridge: Cambridge University Press (2009). p. 109–23. doi: 10.1017/CBO9780511576393.007
195. Donse L, Padberg F, Sack AT, Rush AJ, Arns M. Simultaneous rTMS and psychotherapy in major depressive disorder: Clinical outcomes and predictors from a large naturalistic study. Brain Stimul (2018) 11(2):337–45. doi: 10.1016/j.brs.2017.11.004
196. Potter SM, El Hady A, Fetz EE. Closed-loop neuroscience and neuroengineering. Front Neural Circuits (2014) 8:115. doi: 10.3389/fncir.2014.00115
197. Widge AS, Dougherty DD, Moritz CT. Affective brain-computer interfaces as enabling technology for responsive psychiatric stimulation. Brain-Comput Interfaces (2014) 1(2):126–36. doi: 10.1080/2326263X.2014.912885
Keywords: mental imagery, emotion-regulation, psychotherapy, neuromodulation, neurofeedback, real-time fMRI, brain stimulation
Citation: Skottnik L and Linden DEJ (2019) Mental Imagery and Brain Regulation—New Links Between Psychotherapy and Neuroscience. Front. Psychiatry 10:779. doi: 10.3389/fpsyt.2019.00779
Received: 30 June 2019; Accepted: 30 September 2019;
Published: 30 October 2019.
Edited by:
Gary P. Brown, University of London, United KingdomReviewed by:
Tibor Auer, Royal Holloway, University of London, United KingdomCopyright © 2019 Skottnik and Linden. This is an open-access article distributed under the terms of the Creative Commons Attribution License (CC BY). The use, distribution or reproduction in other forums is permitted, provided the original author(s) and the copyright owner(s) are credited and that the original publication in this journal is cited, in accordance with accepted academic practice. No use, distribution or reproduction is permitted which does not comply with these terms.
*Correspondence: David Linden, ZGF2aWQubGluZGVuQG1hYXN0cmljaHR1bml2ZXJzaXR5Lm5s
Disclaimer: All claims expressed in this article are solely those of the authors and do not necessarily represent those of their affiliated organizations, or those of the publisher, the editors and the reviewers. Any product that may be evaluated in this article or claim that may be made by its manufacturer is not guaranteed or endorsed by the publisher.
Research integrity at Frontiers
Learn more about the work of our research integrity team to safeguard the quality of each article we publish.