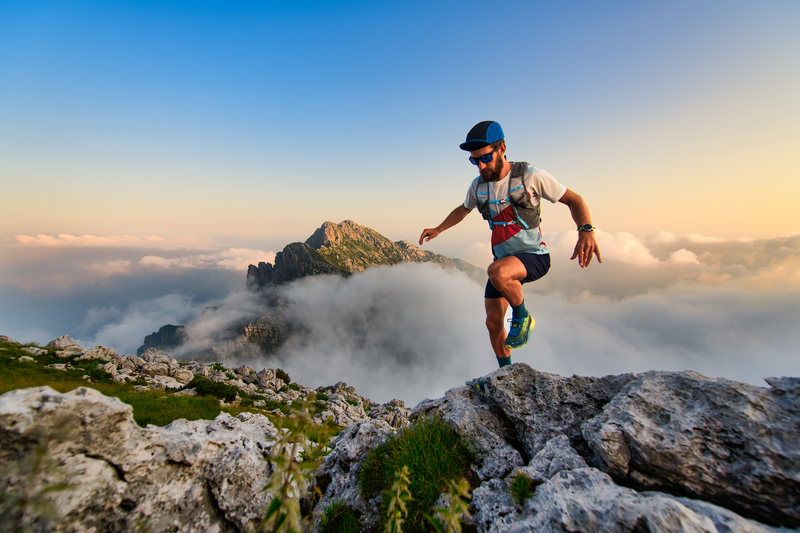
94% of researchers rate our articles as excellent or good
Learn more about the work of our research integrity team to safeguard the quality of each article we publish.
Find out more
ORIGINAL RESEARCH article
Front. Psychiatry , 06 March 2018
Sec. Psychopathology
Volume 9 - 2018 | https://doi.org/10.3389/fpsyt.2018.00067
This article is part of the Research Topic Neural Mechanisms Underlying Internet Gaming Disorder View all 14 articles
With the development of the Internet, an increasing number of adolescents play online game excessively, which leads to adverse effects on individuals and society. Previous studies have demonstrated altered gray-matter volume (GMV) in individuals with Internet gaming disorder (IGD), but the relationship between the tendency to IGD and the GMV across whole brain is still unclear in adolescents. In the present study, anatomical imaging with high resolution was performed on 67 male adolescents who played online game; and Young’s Internet addiction test (IAT) was conducted to test the tendency to IGD. FMRIB Software Library (FSL) was used to calculate the voxel-based correlations between the GMV and the IAT score after controlling for the age and years of education. The GMVs of the bilateral postcentral gyri (postCG), the bilateral precentral gyri (preCG), the right precuneus, the left posterior midcingulate cortex (pMCC), the left inferior parietal lobe (IPL), and the right middle frontal gyrus (MFG) were negatively correlated with the IAT score. The correlation still existed between the IAT score and the GMVs of the bilateral postCG, the left preCG, the left pMCC, and the right MFG after controlling for the total time of playing online game. When the participants were divided into two groups according to the IAT score, the GMVs of these IAT-related brain regions were lower in high IAT score subgroup (IAT score >50) than in low IAT score subgroup (IAT score ≤50). Our results suggested that the GMVs of brain regions involved in sensorimotor process and cognitive control were associated with the IGD tendency. These findings may lead to new targets for preventing and treating the IGD.
In the past decades, the Internet played an important role in our life. However, more and more adolescents surf the Internet and play online game excessively, which result in adverse effects on adolescents themselves and society. An epidemiological study demonstrated that Internet gaming disorder (IGD), a subtype of Internet addiction (IA) (1), was a very common mental health problem among Chinese adolescents (2). Therefore, more and more studies focused on the neuromechanism of IGD and aimed to contribute to the prevention and treatment of IGD.
Structural neuroimaging of brain could be used to investigate brain mechanisms about individual personality traits (3–5). Previous structural studies have found that individuals with IGD had structural abnormalities in gray matter (GM), such as decreased gray-matter volume (GMV) or GM density in multiple cortical and subcortical areas (6–11), and increased GMV in frontal and temporal regions (8, 12). These studies suggested that multiple brain areas in the frontal, temporal, parietal, and subcortical regions such as ventral striatum were associated with IA, which contributed to the understanding of the neuromechanisms of IA. However, the majority of previous studies just focused on the IA or IGD diagnosed by clinical questionnaire such as Internet addiction test (IAT), and compared the differences in behavior and brain function and structure between the IGD individuals and healthy controls. As a matter of fact, not all the individuals who play online game suffer from the IGD (13). Therefore, investigation of the structural correlations in online game players with different levels of tendency to IGD, not only the individuals with IGD diagnosis, is necessary.
Recently, three studies directly focused on the neural associations of the tendency to the IA. Wen and Hsieh (14) explored the relationship between the whole brain functional connections and the level of IA in a group of young adults (19–29 years) and found two networks mainly consisted of frontal regions were correlated to the tendency of IA. Li et al. (15) reported that the structure and functional connectivity of the right dorsolateral prefrontal cortex were positively correlated with the IAT score in a group of healthy young adults (18–27 years). A study by Kühn (16) revealed that the GMV of the brain regions within fronto-striatal network correlated to excessive Internet use assessed by IAT score. Additionally, previous studies have also demonstrated that the GMV changes were related to the online game addiction severity in the IGD subjects. For example, a study by Weng et al. demonstrated that the GMVs of the right orbitofrontal cortex and bilateral insula were positively correlated with the online game addiction severity in the IGD subjects (7). Cai et al. reported increased GMV of nucleus accumbens was associated with the IAT score in the IGD individuals (17). A study by Zhou et al. showed that lower GMV in the right orbitofrontal cortex was related to higher online video gaming addiction severity within the Internet gamers (18). These studies demonstrated that brain structures and functions were associated with the level of IA. However, the relationship between the tendency to IGD and the GMV across whole brain was not yet clearly evaluated in adolescents (14–18 years). The adolescent between 14 and 18 years of age is in a critical period of psychological development and is prone to addiction and adverse effects (19, 20). Many studies regarding the substance addiction paid close attention to adolescents aged from 14 to 18 years (21, 22). A large-sample study demonstrated that the IGD is very common in Chinese elementary and middle school students with a incidence of 22.5% among those students who play online games (2). Therefore, it is more necessary to investigate the brain structural correlations with the tendency to IGD in adolescents (14–18 years).
Furthermore, previous studies demonstrated that long-term online game playing could lead to structural reorganization of the brain in online game players (12, 23, 24). The GMVs in the ventrolateral prefrontal cortex, the dorsolateral prefrontal cortex, the supplementary motor area, and the rostral anterior cingulate cortex were correlated with the duration of online game playing in the adolescents with IA disorder (6, 25). Therefore, whether the duration of online game playing affects the relation between the GMV and the tendency to IGD is worth studying.
In the present study, 67 male adolescents (14–18 years) who played online games were recruited. The voxel-based correlation analysis was conducted to detect the brain regions associated with IAT score before and after controlling for the total time of playing online game. Based on the previous studies, the prefrontal-striatal circuits are closely related to the addiction. Ventral striatum participated in the habit learning and rewarding process involved in addiction (26, 27), and the reduced control effect of prefrontal cortex on rewarding process is one of the mechanisms of addiction (28, 29). Therefore, we hypothesized that the IGD tendency may be associated with the brain regions related to the cognitive control (prefrontal cortex) and the rewarding process (ventral striatum). This study may lead to new targets for preventing and treating the IGD in adolescents.
Sixty seven right-handed adolescents (14–18 years old, average 15.54 ± 0.14) who played online game were recruited in this study. Twenty of 67 participants were the students of a Health School and 47 of 67 participants were the adolescents whose parents took them to a psychiatrist because of possible IGD. All participants received education for 6–12 years, ranging from primary school to senior high school. All of the participants spent more than 80% of the online time on playing online game. Only male adolescents were enrolled in this study because relatively small number of females play online games and suffer from IGD (2, 30). Exclusion criteria included the following: alcohol abuse or drug dependence; existence of any neurologic or psychiatric disease such as insomnia, migraines, tinnitus, and attention deficit hyperactive disorder; history of physical illness such as brain trauma, brain tumor, or epilepsy assessed according to clinical evaluations and medical records; MRI contradiction; and visible abnormalities on conventional MRI. The present study was approved by the Ethical Committee of Tianjin Medical University General Hospital, and all of the participants and their guardians provided written informed consent according to institutional guidelines.
Internet addiction test was used to assess the severity of the tendency to IGD in this study. The IAT consists of 20 items and the answers of these questions were described as 1–5 score (1 = “rarely” to 5 = “always”) (31). The total score of 20 items measures the severity of Internet dependency. The experience of online game playing was assessed via a self-report questionnaire that questioned about the length and amount of playing. The total time of playing online game was calculated as hours per day multiplied by the days of playing online games. Intelligence Quotient (IQ) of all participants was tested using Standard Raven’s Progressive Matrices. The anxiety and depression were texted by using the self-rating anxiety scale (SAS) and the self-rating depression scale (SDS).
Structural images were obtained using a Siemens 3.0 T scanner (Magnetom Verio, Siemens, Erlangen, Germany). A series of 192 contiguous sagittal high-resolution anatomical images were obtained using a three-dimensional T1-weighted volumetric magnetization-prepared rapid gradient-echo sequence with the following parameters: TR = 2000 ms, TE = 2.34 ms, TI = 900 ms, flip angle = 9°, FOV = 256 mm × 256 mm, slice thickness = 1 mm, matrix size = 256 × 256.
All structural images were preprocessed with the VBM8 toolbox1 of the SPM8 (Wellcome Department of Imaging Neuroscience, London, UK)2 running on MATLAB R2010a (Math Works Inc., Sherborn, MA, USA). Three-dimensional geometric correction was performed during reconstructing the images. After that, the individual native images of all participants were segmented into GM, white matter (WM), and cerebral spinal fluid (CSF), and the GM segments were normalized to the Montreal Neurological Institute template by diffeomorphic anatomical registration through exponentiated lie algebra (DARTEL) (32). The registered GM images were then modulated by dividing the Jacobian of the warp field to correct for local expansion or contraction. The isotropic Gaussian kernel of 8-mm full width at half maximum was adopted to smooth the modulated GM images. The mean image of normalized GM from all participants was used to create a GM mask whose threshold was set at a value of 0.3 (pixels with computed GM fraction values >30% were selected). Then the GM mask was used as an explicit mask for the statistical analysis to exclude the pixels with low GM probability values.
Voxel-wise multiple regression analysis was carried out to explore the correlation between the GMV and the IAT score across all participants after controlling for the age and years of education. The non-parametric permutation approach (33) was accomplished by the randomize tool commanded in FMRIB Software Library (FSL)3. The threshold-free cluster enhancement (TFCE) analysis was performed as it combines cluster extent and height into one statistic and does not require an arbitrary choice of a cluster forming threshold (34). The correlation between the GMV and the IAT score was assessed using permutation-based non-parametric testing with 5,000 random permutations. The statistical threshold for significance was defined at P < 0.01. For clarifying whether the duration of online game playing affected the correlation between the GMV and the IAT, Voxel-wise multiple regression analysis was conducted again when adding the total time of playing online game as a nuisance covariate.
Clusters with correlation between the GMV and the IAT score were defined as regions of interest (ROIs), and the average GMV within each ROI was extracted. ROI-based correlation analysis was conducted between the average GMV and the IAT score after controlling for the age and years of education. Then, all of the participants were divided into two subgroups, the high IAT score group (IAT score >50, N = 30) and the low IAT score group (IAT score ≤50, N = 37). The difference in the GMV between the two subgroups was tested by General Linear Model analysis, controlling for the age and years of education. The significance levels were both set at P < 0.05.
Participants had a median score of 46 on the IAT which was used to assess the IGD tendency. Subjects spent average 5.5 h/day on playing online games and lasted for average 56 months. The clinical and demographic characteristics are listed in Table 1.
Voxel-wise correlation analysis revealed that the GMVs of the bilateral postcentral gyri (postCG), the bilateral precentral gyri (preCG), the right precuneus, the left posterior midcingulate cortex (pMCC), the left inferior parietal lobe (IPL), and the right middle frontal gyrus (MFG) were significantly correlated to the IAT score (Figure 1; Table 2). Figure 2 shows the ROI-based correlations between the GMV and the IAT score. After the total time of playing online game was added as a nuisance covariate, the correlation still existed between the IAT and the GMV of the bilateral postCG, the left preCG, the left pMCC, and the right MFG (Figure 3; Table 3).
Figure 1. Brain regions showing negative structural correlates to Internet addiction test (IAT) score in adolescent online game players. The IAT score was negatively correlated to the gray-matter volumes (GMVs) of the bilateral postcentral gyri, the bilateral precentral gyri, the right precuneus, the left posterior mid cingulate cortex, the left inferior parietal lobule, and the right middle frontal gyrus. The numbers below the images are the Montreal Neurological Institute coordinates at z-axis. The colorbar represents the −log p.
Figure 2. Regions-of-interest (ROI)-based correlation analysis between the gray-matter volume (GMV) and the Internet addiction test (IAT) score. The residual was used because the age and years of education were controlled during correlation analysis.
Figure 3. Brain regions showing negative structural correlates to Internet addiction test (IAT) score in adolescent online game players after controlling for the total time of playing online game. The IAT score was negatively correlated to the gray-matter volumes (GMVs) of the bilateral postcentral gyri, the left precentral gyrus, the left posterior mid cingulate cortex, and the right middle frontal gyrus. The numbers below the images are the Montreal Neurological Institute coordinates at z-axis. The colorbar represents the −log p.
Table 3. Regions showed structural correlates to Internet addiction test (IAT) score after controlling for the total time of playing online game.
As seen in Table 4, when the participants were divided into the two subgroups according to the IAT score, the subgroup with high IAT score (IAT score >50) had lower GMV in the seven of eight regions compared with the subgroup with low IAT score group (IAT score ≤50) (P < 0.05).
Table 4. Regions-of-interest (ROI)-based comparisons of the gray-matter volume (GMV) between the two subgroups.
In the present study, the association between the GMV and IGD tendency was evaluated within the whole brain in adolescent online game players. After controlling for the effect of the total time of playing online game, the GMVs of the bilateral postCG, the left preCG, the left pMCC, and the right MFG were still negatively correlated to the IGD tendency. The adolescents with lower GMV in the brain regions related to sensorimotor process and cognitive control had higher IGD tendency.
It was consistent with the hypothesis that the GMV in MFG, as a part of prefrontal cortex involved in cognitive controls (35, 36), was negatively correlated with the IGD tendency. Structural and functional abnormalities were widely reported in individuals with IGD (37–40). For example, less activation in the prefrontal cortex was found in the IA (40). Previous studies demonstrated the lower GM density and GMV in the prefrontal cortex in the IGD individuals (37, 39). Smaller amplitude of low-frequency fluctuation within the right MFG was also revealed in the IGD individuals (41). Abnormal activation in the prefrontal cortex was also found in drug-addicted individuals such as the marijuana users and the abstinent cocaine abusers (42–44). Similar changes in functional connectivity of the prefrontal cortex were revealed in the individuals with alcohol dependence and the individuals with IGD (45, 46). These studies demonstrated that the structural or functional condition of prefrontal cortex was associated with the addiction. In this study, the GMV of the right MFG was negatively correlated to the IAT score, and was lower in the high IAT score subgroup than that in the low IAT score subgroup. Structural abnormality in the right MFG might lead to the impairment of cognitive control in online game players. As a result, the online game players could not control their problematic online game playing and exhibited a higher tendency to the IGD.
Incongruent with the hypothesis, we did not find the GMV of the ventral striatum correlating with the IAT score. The ventral striatum is a critical region related to the addiction, and usually presents abnormal activation in individuals with addiction (26, 27). In our study, we focused on adolescent online game players but not only the IGD individuals, which might be a possible explanation to the negative result of ventral striatum. However, this negative result should be verified in the future study with large sample size.
Unexpectedly, the preCG, postCG, and the pMCC involved in the sensorimotor process showed negative correlations with the IAT score. The preCG played a major role in the motor planning and conducting (47). Adolescence is a critical period of neural development, and is prone to be affected by the environmental factors. Previous studies demonstrated that the alcohol and drug use might change the GMV in the developing brain of adolescents (48). A study showed longer use of the methamphetamine was associated with the GMV reduction in the preCG (49). In our study, the GMV of preCG was lower in the high IAT score subgroup than that in the low IAT score subgroup. Considering prevention and suppression of the action is conceptually associated with the primary motor cortex (50), the decreased GMV of preCG might be related to the IGD tendency. The postCG consists of the primary sensory cortex and is involved in integrating sensory information (24). The negative correlation between the GMV of the postCG and the IAT score means the lower GMV of this region in individuals with higher IAT score. Abnormal function connectivity of the postCG was found in adolescents with IGD (51). The decreased GMV and cortical thickness of the postCG were also revealed, respectively, in heroin users (52) and adolescents with online gaming addiction (53). The impaired postCG may lead to abnormality in receiving, processing, and integrating body-relevant signals and may fail to guide ongoing behavior related to arousal, attention, stress, reward, and conditioning, and finally associated with the addiction (54). In this study, negative structural correlations to the IAT score were also found in the left pMCC. The pMCC exhibits extensive functional connectivity with brain regions involved in the sensorimotor network (55, 56) and has important role in processing sensorimotor integration and motor control (57). The sensorimotor areas not only control the basic aspects of movement but can also shape human behavior (58). The functional properties of sensorimotor network may be relevant for automatized/compulsive behaviors in addiction (59). Sensorimotor cortex impairments were also reported in individuals with cocaine addiction (60, 61) and alcohol ingestion (62). Taken together, the reduction of the GMVs within the preCG, postCG, and the pMCC might have association with the abnormalities of the sensorimotor network, and further associated with the IGD tendency.
In the present study, the negative correlations between the IAT score and the GMVs of the right preCG/postCG, the left IPL, and the right precuneus disappeared after controlling for the effect of the total time of playing online game. The preCG/postCG was involved in sensorimotor process (63); the IPL and the right precuneus were closely related to the visual and intentional processing (64–66). Gaming process requires players to pay full attention to the tiny change in the screen for a long time then injures their visual ability (65), which might have a relationship with the GMV reduction in the visual attention-related regions. Previous studies demonstrated decreased GMV of precuneus (8) and decreased cortical thickness of the IPL (53) in the individuals with online game addiction. Our results indicated that the GMV reduction in some brain regions related to the visual attention and sensorimotor process was influenced by the total time of playing online game, namely had a cumulative effect of playing online game.
Several limitations should be noted in our study. First, although some correlations were revealed between the brain GMV and IAT score, the causality cannot be clarified in this correlation analysis. The observed lower GMV in the adolescents with higher IAT score may be a result of excessive online game playing or a preexisting condition which is sensitive to IGD. Second, the IAT is a subjective questionnaire and more objective methods for evaluating the tendency to IGD are needed. Third, the total time of playing online games was just a probable measure and might be not accurate enough. Fourth, we could not rule out the effect of game genre on the results, which should be considered in the future study. Finally, only male adolescents were recruited in our study. Therefore, the present findings are restricted to male adolescent online game players.
In this study, the structural correlation to the IGD tendency was investigated in a group of adolescent online game players. The GMV of brain regions related to the sensorimotor process and cognitive control were found to be associated with the IAT score. The lower GMV of the regions related to sensorimotor process and cognitive control might attribute to the high IGD tendency, which might lead to new targets for preventing and treating the IGD in adolescents.
The present study was approved by the Ethical Committee of Tianjin Medical University General Hospital, and all of the participants and their guardians provided written informed consent according to institutional guidelines.
NP, YY, XL, and QZ designed research. XQ, XD, GD, YZ, and QZ performed research. YY was involved in the clinical assessment. NP, YZ, GD, and QZ analyzed data. NP, YZ, XL, YY, and QZ wrote the paper.
The authors declare that the research was conducted in the absence of any commercial or financial relationships that could be construed as a potential conflict of interest.
1. Block JJ. Issues for DSM-V: Internet addiction. Am J Psychiatry (2008) 165(3):306–7. doi:10.1176/appi.ajp.2007.07101556
2. Li Y, Zhang X, Lu F, Zhang Q, Wang Y. Internet addiction among elementary and middle school students in China: a nationally representative sample study. Cyberpsychol Behav Soc Netw (2014) 17(2):111–6. doi:10.1089/cyber.2012.0482
3. Hayakawa YK, Sasaki H, Takao H, Mori H, Hayashi N, Kunimatsu A, et al. Structural brain abnormalities in women with subclinical depression, as revealed by voxel-based morphometry and diffusion tensor imaging. J Affect Disord (2013) 144(3):263–8. doi:10.1016/j.jad.2012.10.023
4. Takeuchi H, Taki Y, Sassa Y, Hashizume H, Sekiguchi A, Nagase T, et al. Regional gray and white matter volume associated with Stroop interference: evidence from voxel-based morphometry. Neuroimage (2012) 59(3):2899–907. doi:10.1016/j.neuroimage.2011.09.064
5. Liu F, Guo W, Yu D, Gao Q, Gao K, Xue Z, et al. Classification of different therapeutic responses of major depressive disorder with multivariate pattern analysis method based on structural MR scans. PLoS One (2012) 7(7):e40968. doi:10.1371/journal.pone.0040968
6. Yuan K, Qin W, Wang G, Zeng F, Zhao L, Yang X, et al. Microstructure abnormalities in adolescents with Internet addiction disorder. PLoS One (2011) 6(6):e20708. doi:10.1371/journal.pone.0020708
7. Weng CB, Qian RB, Fu XM, Lin B, Han XP, Niu CS, et al. Gray matter and white matter abnormalities in online game addiction. Eur J Radiol (2013) 82(8):1308–12. doi:10.1016/j.ejrad.2013.01.031
8. Sun Y, Sun J, Zhou Y, Ding W, Chen X, Zhuang Z, et al. Assessment of in vivo microstructure alterations in gray matter using DKI in Internet gaming addiction. Behav Brain Funct (2014) 10:37. doi:10.1186/1744-9081-10-37
9. Ko CH, Hsieh TJ, Wang PW, Lin WC, Yen CF, Chen CS, et al. Altered gray matter density and disrupted functional connectivity of the amygdala in adults with Internet gaming disorder. Prog Neuropsychopharmacol Biol Psychiatry (2015) 57:185–92. doi:10.1016/j.pnpbp.2014.11.003
10. Lin X, Dong G, Wang Q, Du X. Abnormal gray matter and white matter volume in ‘Internet gaming addicts’. Addict Behav (2015) 40:137–43. doi:10.1016/j.addbeh.2014.09.010
11. Zhou Y, Lin FC, Du YS, Qin LD, Zhao ZM, Xu JR, et al. Gray matter abnormalities in Internet addiction: a voxel-based morphometry study. Eur J Radiol (2011) 79(1):92–5. doi:10.1016/j.ejrad.2009.10.025
12. Kuhn S, Gleich T, Lorenz RC, Lindenberger U, Gallinat J. Playing super mario induces structural brain plasticity: gray matter changes resulting from training with a commercial video game. Mol Psychiatry (2014) 19(2):265–71. doi:10.1038/mp.2013.120
13. Przybylski AK, Weinstein N, Murayama K. Internet gaming disorder: investigating the clinical relevance of a new phenomenon. Am J Psychiatry (2017) 174(3):230–6. doi:10.1176/appi.ajp.2016.16020224
14. Wen T, Hsieh S. Network-based analysis reveals functional connectivity related to Internet addiction tendency. Front Hum Neurosci (2016) 10:6. doi:10.3389/fnhum.2016.00006
15. Li W, Li Y, Yang W, Zhang Q, Wei D, Li W, et al. Brain structures and functional connectivity associated with individual differences in Internet tendency in healthy young adults. Neuropsychologia (2015) 70:134–44. doi:10.1016/j.neuropsychologia.2015.02.019
16. Kuhn S, Gallinat J. Brains online: structural and functional correlates of habitual Internet use. Addict Biol (2015) 20(2):415–22. doi:10.1111/adb.12128
17. Cai C, Yuan K, Yin J, Feng D, Bi Y, Li Y, et al. Striatum morphometry is associated with cognitive control deficits and symptom severity in Internet gaming disorder. Brain Imaging Behav (2016) 10(1):12–20. doi:10.1007/s11682-015-9358-8
18. Zhou F, Montag C, Sariyska R, Lachmann B, Reuter M, Weber B, et al. Orbitofrontal gray matter deficits as marker of Internet gaming disorder: converging evidence from a cross-sectional and prospective longitudinal design. Addict Biol (2017). doi:10.1111/adb.12570
19. Ac-Nikolic E, Zaric D, Niciforovic-Surkovic O. Prevalence of Internet addiction among schoolchildren in Novi Sad. Srp Arh Celok Lek (2015) 143(11–12):719–25. doi:10.2298/SARH1512719A
20. Gonzalvez MT, Espada JP, Orgiles M, Morales A, Sussman S. Nicotine dependence as a mediator of project EX’s effects to reduce tobacco use in scholars. Front Psychol (2016) 7:1207. doi:10.3389/fpsyg.2016.01207
21. Colby SM, Nargiso J, Tevyaw TO, Barnett NP, Metrik J, Lewander W, et al. Enhanced motivational interviewing versus brief advice for adolescent smoking cessation: results from a randomized clinical trial. Addict Behav (2012) 37(7):817–23. doi:10.1016/j.addbeh.2012.03.011
22. Thanki D, Domingo-Salvany A, Barrio Anta G, Sanchez Manez A, Llorens Aleixandre N, Suelves JM, et al. The choice of screening instrument matters: the case of problematic cannabis use screening in Spanish population of adolescents. ISRN Addict (2013) 2013:723131. doi:10.1155/2013/723131
23. Kuhn S, Romanowski A, Schilling C, Lorenz R, Morsen C, Seiferth N, et al. The neural basis of video gaming. Transl Psychiatry (2011) 1:e53. doi:10.1038/tp.2011.53
24. Tanaka S, Ikeda H, Kasahara K, Kato R, Tsubomi H, Sugawara SK, et al. Larger right posterior parietal volume in action video game experts: a behavioral and voxel-based morphometry (VBM) study. PLoS One (2013) 8(6):e66998. doi:10.1371/journal.pone.0066998
25. Lee D, Namkoong K, Lee J, Jung YC. Abnormal gray matter volume and impulsivity in young adults with Internet gaming disorder. Addict Biol (2017). doi:10.1111/adb.12552
26. Ashby FG, Turner BO, Horvitz JC. Cortical and basal ganglia contributions to habit learning and automaticity. Trends Cogn Sci (2010) 14(5):208–15. doi:10.1016/j.tics.2010.02.001
27. Volkow ND, Wang GJ, Fowler JS, Tomasi D, Telang F. Addiction: Beyond dopamine reward circuitry. Proc Natl Acad Sci U S A (2011) 108(37):15037–42. doi:10.1073/pnas.1010654108
28. Walter M, Wang Y, Yin Y, Sun Y-W, Zhou Y, Chen X, et al. Decreased prefrontal lobe interhemispheric functional connectivity in adolescents with Internet gaming disorder: a primary study using resting-state fMRI. PLoS One (2015) 10(3):e0118733. doi:10.1371/journal.pone.0118733
29. Koob GF, Volkow ND. Neurocircuitry of addiction. Neuropsychopharmacology (2009) 35(1):217–38. doi:10.1038/npp.2009.110
30. Yu L, Shek DT. Internet addiction in Hong Kong adolescents: a three-year longitudinal study. J Pediatr Adolesc Gynecol (2013) 26(3 Suppl):S10–7. doi:10.1016/j.jpag.2013.03.010
31. Young KS. Caught in the net: how to recognize the signs of Internet addiction – and a winning strategy for recovery. Assessment (1998) 21(6):713–22.
32. Ashburner J. A fast diffeomorphic image registration algorithm. Neuroimage (2007) 38(1):95–113. doi:10.1016/j.neuroimage.2007.07.007
33. Nichols TE, Holmes AP. Nonparametric permutation tests for functional neuroimaging: a primer with examples. Hum Brain Mapp (2002) 15(1):1–25. doi:10.1002/hbm.1058
34. Smith SM, Nichols TE. Threshold-free cluster enhancement: addressing problems of smoothing, threshold dependence and localisation in cluster inference. Neuroimage (2009) 44(1):83–98. doi:10.1016/j.neuroimage.2008.03.061
35. Rd MDA, Cohen JD, Stenger VA, Carter CS. Dissociating the role of the dorsolateral prefrontal and anterior cingulate cortex in cognitive control. Science (2000) 288(5472):1835. doi:10.1126/science.288.5472.1835
36. Yuan P, Raz N. Prefrontal cortex and executive functions in healthy adults: a meta-analysis of structural neuroimaging studies. Neurosci Biobehav Rev (2014) 42(5):180–92. doi:10.1016/j.neubiorev.2014.02.005
37. Wang H, Jin C, Yuan K, Shakir TM, Mao C, Niu X, et al. The alteration of gray matter volume and cognitive control in adolescents with internet gaming disorder. Front Behav Neurosci (2015) 9:64. doi:10.3389/fnbeh.2015.00064
38. Liu GC, Yen JY, Chen CY, Yen CF, Chen CS, Lin WC, et al. Brain activation for response inhibition under gaming cue distraction in Internet gaming disorder. Kaohsiung J Med Sci (2014) 30(1):43–51. doi:10.1016/j.kjms.2013.08.005
39. Choi J, Cho H, Kim JY, Jung DJ, Ahn KJ, Kang HB, et al. Structural alterations in the prefrontal cortex mediate the relationship between Internet gaming disorder and depressed mood. Sci Rep (2017) 7(1):1245. doi:10.1038/s41598-017-01275-5
40. Seok JW, Lee KH, Sohn S, Sohn JH. Neural substrates of risky decision making in individuals with Internet addiction. Aust N Z J Psychiatry (2015) 49(10):923–32. doi:10.1177/0004867415598009
41. Park SY, Kim SM, Roh S, Soh MA, Lee SH, Kim H, et al. The effects of a virtual reality treatment program for online gaming addiction. Comput Methods Programs Biomed (2016) 129:99–108. doi:10.1016/j.cmpb.2016.01.015
42. Bolla K, Ernst M, Kiehl K, Mouratidis M, Eldreth D, Contoreggi C, et al. Prefrontal cortical dysfunction in abstinent cocaine abusers. J Neuropsychiatry Clin Neurosci (2004) 16(4):456. doi:10.1176/jnp.16.4.456
43. Bolla KI, Eldreth DA, Matochik JA, Cadet JL. Neural substrates of faulty decision-making in abstinent marijuana users. Neuroimage (2005) 26(2):480–92. doi:10.1016/j.neuroimage.2005.02.012
44. Bolla KI, Eldreth DA, London ED, Kiehl KA, Mouratidis M, Contoreggi C, et al. Orbitofrontal cortex dysfunction in abstinent cocaine abusers performing a decision-making task. Neuroimage (2003) 19(3):1085. doi:10.1016/S1053-8119(03)00113-7
45. Ge X, Sun Y, Han X, Wang Y, Ding W, Cao M, et al. Difference in the functional connectivity of the dorsolateral prefrontal cortex between smokers with nicotine dependence and individuals with Internet gaming disorder. BMC Neurosci (2017) 18(1):54. doi:10.1186/s12868-017-0375-y
46. Han JW, Han DH, Bolo N, Kim B, Kim BN, Renshaw PF. Differences in functional connectivity between alcohol dependence and Internet gaming disorder. Addict Behav (2015) 41:12–9. doi:10.1016/j.addbeh.2014.09.006
47. Nock NL, Dimitropolous A, Tkach J, Frasure H, von Gruenigen V. Reduction in neural activation to high-calorie food cues in obese endometrial cancer survivors after a behavioral lifestyle intervention: a pilot study. BMC Neurosci (2012) 13:74. doi:10.1186/1471-2202-13-74
48. Squeglia LM, Gray KM. Alcohol and drug use and the developing brain. Curr Psychiatry Rep (2016) 18(5):46. doi:10.1007/s11920-016-0689-y
49. Hall MG, Alhassoon OM, Stern MJ, Wollman SC, Kimmel CL, Perez-Figueroa A, et al. Gray matter abnormalities in cocaine versus methamphetamine-dependent patients: a neuroimaging meta-analysis. Am J Drug Alcohol Abuse (2015) 41(4):290–9. doi:10.3109/00952990.2015.1044607
50. Stinear CM, Coxon JP, Byblow WD. Primary motor cortex and movement prevention: where stop meets go. Neurosci Biobehav Rev (2009) 33(5):662–73. doi:10.1016/j.neubiorev.2008.08.013
51. Zhang JT, Yao YW, Li CS, Zang YF, Shen ZJ, Liu L, et al. Altered resting-state functional connectivity of the insula in young adults with Internet gaming disorder. Addict Biol (2016) 21(3):743–51. doi:10.1111/adb.12247
52. Gardini S, Venneri A. Reduced grey matter in the posterior insula as a structural vulnerability or diathesis to addiction. Brain Res Bull (2012) 87(2–3):205–11. doi:10.1016/j.brainresbull.2011.11.021
53. Yuan K, Cheng P, Dong T, Bi Y, Xing L, Yu D, et al. Cortical thickness abnormalities in late adolescence with online gaming addiction. PLoS One (2013) 8(1):e53055. doi:10.1371/journal.pone.0053055
54. Paulus MP, Stewart JL. Interoception and drug addiction. Neuropharmacology (2014) 76(Pt B):342–50. doi:10.1016/j.neuropharm.2013.07.002
55. Picard N, Strick PL. Motor areas of the medial wall: a review of their location and functional activation. Cereb Cortex (1996) 6(3):342–53. doi:10.1093/cercor/6.3.342
56. Yu C, Zhou Y, Liu Y, Jiang T, Dong H, Zhang Y, et al. Functional segregation of the human cingulate cortex is confirmed by functional connectivity based neuroanatomical parcellation. Neuroimage (2011) 54(4):2571–81. doi:10.1016/j.neuroimage.2010.11.018
57. Wang D, Zhou Y, Zhuo C, Qin W, Zhu J, Liu H, et al. Altered functional connectivity of the cingulate subregions in schizophrenia. Transl Psychiatry (2015) 5:e575. doi:10.1038/tp.2015.69
58. Casartelli L, Chiamulera C. The motor way: clinical implications of understanding and shaping actions with the motor system in autism and drug addiction. Cogn Affect Behav Neurosci (2016) 16(2):191–206. doi:10.3758/s13415-015-0399-7
59. Gremel CM, Lovinger DM. Associative and sensorimotor cortico-basal ganglia circuit roles in effects of abused drugs. Genes Brain Behav (2017) 16(1):71–85. doi:10.1111/gbb.12309
60. Porrino LJ, Lyons D, Smith HR, Daunais JB, Nader MA. Cocaine self-administration produces a progressive involvement of limbic, association, and sensorimotor striatal domains. J Neurosci (2004) 24(14):3554–62. doi:10.1523/JNEUROSCI.5578-03.2004
61. Hanlon CA, Wesley MJ, Roth AJ, Miller MD, Porrino LJ. Loss of laterality in chronic cocaine users: an fMRI investigation of sensorimotor control. Psychiatry Res (2010) 181(1):15–23. doi:10.1016/j.pscychresns.2009.07.009
62. Nikolaou K, Critchley H, Duka T. Alcohol affects neuronal substrates of response inhibition but not of perceptual processing of stimuli signalling a stop response. PLoS One (2013) 8(9):e76649. doi:10.1371/journal.pone.0076649
63. White LE, Andrews TJ, Hulette C, Richards A, Groelle M, Paydarfar J, et al. Structure of the human sensorimotor system. I: morphology and cytoarchitecture of the central sulcus. Cereb Cortex (1997) 7(1):18–30. doi:10.1093/cercor/7.1.18
64. Cavanna AE, Trimble MR. The precuneus: a review of its functional anatomy and behavioural correlates. Brain (2006) 129(Pt 3):564–83. doi:10.1093/brain/awl004
65. Feng Q, Chen X, Sun J, Zhou Y, Sun Y, Ding W, et al. Voxel-level comparison of arterial spin-labeled perfusion magnetic resonance imaging in adolescents with Internet gaming addiction. Behav Brain Funct (2013) 9(1):33. doi:10.1186/1744-9081-9-33
Keywords: Internet gaming addiction, gray-matter volume, Internet addiction test, online game, adolescent
Citation: Pan N, Yang Y, Du X, Qi X, Du G, Zhang Y, Li X and Zhang Q (2018) Brain Structures Associated with Internet Addiction Tendency in Adolescent Online Game Players. Front. Psychiatry 9:67. doi: 10.3389/fpsyt.2018.00067
Received: 29 June 2017; Accepted: 19 February 2018;
Published: 06 March 2018
Edited by:
Matthias Brand, University of Duisburg-Essen, GermanyReviewed by:
Jennifer Kennel, Universitätsklinikum des Saarlandes, GermanyCopyright: © 2018 Pan, Yang, Du, Qi, Du, Zhang, Li and Zhang. This is an open-access article distributed under the terms of the Creative Commons Attribution License (CC BY). The use, distribution or reproduction in other forums is permitted, provided the original author(s) and the copyright owner are credited and that the original publication in this journal is cited, in accordance with accepted academic practice. No use, distribution or reproduction is permitted which does not comply with these terms.
*Correspondence: Xiaodong Li, bHhkODE5OTgxOUAxMjYuY29t;
Quan Zhang, emhhbmdxdWFuMDkxMkAxNjMuY29t
†These authors have contributed equally to this work.
Disclaimer: All claims expressed in this article are solely those of the authors and do not necessarily represent those of their affiliated organizations, or those of the publisher, the editors and the reviewers. Any product that may be evaluated in this article or claim that may be made by its manufacturer is not guaranteed or endorsed by the publisher.
Research integrity at Frontiers
Learn more about the work of our research integrity team to safeguard the quality of each article we publish.