- 1Peking University Sixth Hospital, Institute of Mental Health, Beijing, China
- 2National Clinical Research Center for Mental Disorders, Key Laboratory of Mental Health, Ministry of Health, Peking University, Beijing, China
- 3Center for Cognition and Brain Disorders, Hangzhou Normal University, Hangzhou, China
- 4Zhejiang Key Laboratory for Research in Assessment of Cognitive Impairments, Hangzhou, China
Objectives: Executive function (EF) deficits are major impairments in adults with attention deficit/hyperactivity disorder (ADHD). Previous studies have shown that the insula is involved in cognitive and EFs. However, the insula is highly heterogeneous in function, and few studies have focused on functional networks which related to specific insular subregions in adults with ADHD. We explored the functional networks of the insular subregions [anterior insula (AI), mid-insula (MI), and posterior insula (PI)]. Furthermore, their correlations with self-ratings of ecological EFs, including inhibition, shifting, and working memory were investigated.
Methods: Resting-state functional magnetic resonance imaging data in 28 adults with ADHD and 30 matched healthy controls (HCs) were analyzed. The seed-based resting-state functional connectivity (RSFC) of the insular subregions was evaluated. We also investigated their associations with the Behavior Rating Inventory of Executive Function-Adult Version (BRIEF-A) inhibition, working memory, and shifting factor scores.
Results: Compared with HCs, adults with ADHD showed altered RSFC of the AI, with the precuneus, precentral gyrus, and inferior temporal gyrus extended to the middle temporal gyrus, lingual gyrus, and superior occipital gyrus, respectively. There were no significant differences in RSFC of the MI and PI between the two groups. Within the HC group, working memory scores were associated with the RSFC of AI with precuneus and temporal gyrus. However, there was no correlation between these variables in the ADHD group.
Conclusion: The study evaluated RSFC patterns of the insular subregions in adults with ADHD for the first time. Altered RSFC of the AI which is a crucial region of salience network (SN) and part of regions in default mode network (DMN), were detected in adults with ADHD in both results with and without global signal regression (GSR), suggesting that disrupted SN-DMN functional connectivity may be involved in EF impairments in adults with ADHD, especially with respect to working memory. Deficits of the AI which is involved in salient stimuli allocation, might be associated with the pathophysiology of ADHD. The inconsistent results of MI and PI between analyses with and without GSR need further exploration.
Introduction
Attention deficit/hyperactivity disorder (ADHD) is a childhood-onset neurodevelopmental disorder characterized by developmentally inappropriate symptoms of inattention, hyperactivity, and impulsivity (1). ADHD has an estimated worldwide prevalence of 7.2% among children (2) and in approximately 70% of those diagnosed in childhood, the condition persists into adulthood (3). The persistence of ADHD into adulthood is significantly associated with comorbid anxiety, depression, substance dependence, and emotional dysregulation, exerting effects on the individual’s quality of life (4, 5).
Executive function (EF) deficits are major impairments in ADHD. They include inhibition, working memory, shifting, and so on (6–10). On the basis of a recent review, the core EFs include inhibition, working memory, and cognitive flexibility [also called set shifting (11)]. Furthermore, higher-order EFs, such as reasoning, problem solving, and planning, are built on the foundation of the three core EFs (11). Ecological EF may be more predictive of occupational function than the cognitive performance test (12). Hence, we chose the three factors from the Behavior Rating Inventory Executive Function—Adult Version [BRIEF-A (13)], which is used to estimate ecological EFs.
The pathophysiology of EF impairments in patients with ADHD is still unclear. Convergent evidence indicated that EF impairment in ADHD was associated with functional and structural abnormalities in large-scale brain networks consisting of the prefrontal cortex, parietal lobe, occipital cortex, temporal lobe, insula, and limbic regions (14). Recently, Menon hypothesized the triple network model which consists of the central executive network (CEN), salience network (SN), and default mode network [DMN (15)]. The model indicated that abnormalities in the engagement and disentanglement of the three networks play an important role in many psychiatric disorders, such as depression, autism, and schizophrenia (15). Abnormal cognitive control and state switching in ADHD were also found to be related to aberrant connectivity within SN, CEN, DMN, and attention networks (10, 16). Among these brain networks and regions underlying the neuropathologic mechanism of ADHD, the insula is a critical part of SN and involved in multiple dimensions of EF (17–21). The insula plays an important role in cognitive and affective processes (22). Previous studies have found structural and functional abnormalities in the insula in patients with ADHD. Lopez-Larson et al. revealed a reduction in gray matter volume of the anterior part of the bilateral insula in children with ADHD and that the volume of the right anterior insula (AI) was associated with attention problems and inhibition (19). The insula displayed a significantly abnormal activation during various cognitive tasks including during attention (17, 18), decision making (23), state switching (10), inhibition (24, 25), and working memory tasks (26) in patients with ADHD. In addition, recent studies indicated that children with ADHD showed a decreased functional connectivity between the insula and amygdala interacting with emotional regulation (27, 28).
Previous studies have investigated the abnormal function of the entire insula in patients with ADHD. However, the insula is a complicated and functionally heterogeneous structure. Based on previous studies on insular structure and function (29–31), the insula can be partitioned into three major subregions: the AI, mid-insula (MI), and posterior insula (PI). The AI is implicated in cognitive processes such as working memory, decision making, state switching (shifting), socioemotional function, and interoception (32–34). Adults with ADHD also showed impairments in these processes. The MI is correlated with reactions to olfactogustatory stimuli, sensorimotor function, the awareness of body movement (34), and socioemotional functions. The PI is also implicated in sensorimotor function and interoception (34, 35). The aberrant function and structure of the insula have been investigated in patients with psychiatric diseases such as schizophrenia (36–38), mood disorder (39–42), autism spectrum disorder (43), and posttraumatic stress disorder (44, 45). Different insular subregions have different functions, and a systematic exploration of the neural functional connectivity of insular subregions in patients with ADHD has yet to be conducted.
To explore the neural networks alterations of the insular subregions and the mechanism correlated with ecological EF from a broader view, the present study utilized the seed-based resting-state functional connectivity (RSFC) to investigate the functional connectivity patterns of AI, MI, and PI. Then, we explored their correlations with ecological EFs, including inhibition, working memory and shifting factor scores. Based on prior literature, we expected that (a) patients with ADHD compared with healthy controls (HCs) would show significantly altered RSFC between the insular subregions and other regions (especially ACC and regions within CEN and DMN) and (b) altered RSFC in adults with ADHD between the insular subregions and other brain regions would be correlated with inhibition, working memory, and shifting factor scores.
Materials and Methods
Participants
Thirty-five right-handed adults with ADHD were recruited from clinics of Peking University Sixth Hospital and advertisements on the Internet when taking part in cognitive behavioral therapy studies and 30 HCs matched for sex, age, and IQ were enrolled in the study. All of the participants were interviewed, underwent diagnosis and were screened for any potential comorbidities according to exclusion criteria using the Structured Clinical Interview for DSM-IV Axis I Disorders [SCID-I (46)] by a qualified psychiatrist. Conner’s Adult ADHD Diagnostic Interview for DSM-IV (47) was also completed to confirm the diagnosis of ADHD in subjects in the ADHD group. Full-scale IQ measurements were made using the Wechsler Adult Intelligence Scale, Third Edition. All participants met the following criteria: (a) right-handed, (b) no history of head trauma with a loss of consciousness, (c) no history of neurological illness or other severe disease, and (d) no current diagnosis of schizophrenia, severe major depression, clinically significant panic disorder, bipolar disorder, pervasive developmental disorders, or mental retardation (seven participants in the ADHD group were excluded after this review, of whom one had social phobias, one had anorexia nervoasa, two had major depressive disorder, and three had dysthymia disorder); (e) no excessive head movements (>3.0 mm of translation or degrees of rotation in any direction) and (f) a full-scale IQ above 80. A previous or current history of psychiatric disorders, as evidenced in the SCID-I assessment, or neurological disorders resulted in exclusion from the HC groups. Among the 28 adults with ADHD chosen for the final imaging analyses, 21 met the criteria of the inattentive subtype (ADHD-I) and 7 corresponded to the combined subtype (ADHD-C).
Six participants with ADHD had a history of methylphenidate hydrochloride or tomoxetine hydrochloride treatment. Three of the six had stopped the treatment for a long time or had only taken medicine for a short period of time (no >2 months). The other three participants were still being treated with methylphenidate hydrochloride and were required to undergo a washout period of 24 h before the MRI scan. Furthermore, we compared the mean functional connectivity z-scores of the clusters, which showed differences between the ADHD and HC groups for patients without history, patients with the history and the HCs. There was no difference in mean functional connectivity z-scores of 8 clusters between the two ADHD groups. Furthermore, differences were found between patients with a history and the HCs as well as between patients without a history and the HCs. Therefore, we combined patients with and without medication together to compare with the HC for our final results.
Self-administered scales were completed by all of the participants for diagnostic and correlational purposes.
The ADHD Rating Scale-IV [ADHD RS-IV (48)], a 4-point severity scale (scores 1 indicates no ADHD symptoms, 2 indicates symptoms sometimes, 3 indicates symptoms usually, and 4 indicates symptoms always) was also completed by the participants for the evaluation of the severity of ADHD symptoms. This scale contains 18 items that are consistent with the 18 symptoms in DSM, which consists of 9 inattention items and 9 hyperactivity and impulsivity items.
The Mandarin version of the BRIEF-A consists of 75 items based on the EFs (13). The participants were required to assess their performance in daily life on a 3-point Likert scale (1 equals never; 2 equals sometimes; and 3 equals often). A higher score indicates more EF problems in daily life. The BRIEF-A includes nine factors of EFs: initiating, working memory, planning/organizing, organization of material, task-monitoring, self-monitoring, inhibition, shifting, and emotional control. We chose the working memory, shifting, and inhibition factors from the BRIEF-A. Twenty-seven HCs and 25 patients of the ADHD group completed the BRIEF-A.
This study was approved by the Research Ethics Review Board of Peking University Sixth Hospital. All subjects were fully informed about the research before being asked to sign the informed consent form.
MRI Data Acquisition
Magnetic resonance imaging data were acquired using a Siemens Trio 3 T scanner (Siemens, Erlangen, Germany) at the Imaging Center for Brain Research at Beijing Normal University. During the resting-state functional magnetic resonance imaging (rs-fMRI) scanning, participants lay in the supine position and were instructed to remain still and relaxed with their eyes closed and to keep their mind vacant but without falling asleep. A head strap and foam pads were used to minimize head movements. Functional images were acquired using an echo-planar imaging sequence with the following parameters: repetition time (TR) = 2,000 ms, echo time (TE) = 30 ms, flip angle = 90°, thickness/skip = 3.5/0.7 mm, matrix = 64 × 64, field of view (FOV) = 200 mm × 200 mm, 33 axial slices, and 240 volumes. High-resolution T1-weighted anatomical images were acquired with the following parameters: TR = 2,530 ms, TE = 3.39 ms, inversion time = 1,100 ms, flip angle = 7°, 128 slices, slice thickness = 1.33 mm, FOV = 256 mm × 256 mm, and matrix = 256 × 256.
Data Preprocessing
Resting-state fMRI data preprocessing was performed using the Data Processing & Analysis for (Resting-State) Brain Imaging (DPABI) (49). First, we discarded the first 10 volumes. Then, the data were corrected for slice timing and realigned for head motion correction. Next, the structural images were coregistered to the mean functional image. The high-resolution individual T1-weighted images were segmented into gray matter, white matter and cerebrospinal fluid (CSF) by using the “New Segment” method. Subsequently, we performed nuisance signal regression (including 12 derivative motion parameters, global mean signals, white matter, and CSF) to remove the confounding artifacts of head motion and physiological noise (i.e., cardiac and respiratory fluctuations) during the resting state. The functional data were then normalized to the standard Montreal Neurological Institute (MNI) space (resampled voxel size = 3 mm × 3 mm × 3 mm) and spatially smoothed by Diffeomorphic Anatomical Registration Through Exponentiated Lie Algebra (50). The Gaussian kernel full width at half-maximum was 6 mm3. Linear detrending of the time series and temporal bandpass filtering (0.01–0.08 Hz) were conducted. Whether preprocessing should include global signal regression (GSR) is controversial (51–53). We conducted an analysis without the GSR, and the results are shown in the supplementary materials (Table S1 and Figures S2–S4 in Supplementary Material).
Resting-State Functional Connectivity
We selected six spherical seed regions of interest (ROIs) with a radius of 6 mm centered on six MNI coordinates, which were selected based on the previous anatomical and MRI studies (31, 54): the bilateral AI [MNI (x, y, z): left = −32, 16, 6; right = 32, 16, 6], the bilateral MI (MNI: left = −38, 2, 8; right = 38, 2, 8), and the bilateral PI (MNI: left = −39, −15, 1; right = 39, −15, 8). The time course of the ROIs were correlated against all other voxels within the whole brain. Individual RSFC maps of the AI, MI and PI were generated by calculating Pearson’s correlation coefficients between the mean time series of the ROIs and the time series of each voxel in the whole brain. Subject-level correlation maps were then converted to z-value maps using Fisher’s transformation to improve the normality. All figures were displayed using BrainNet Viewer (55). We used the insular subregion masks (k = 3) for further analysis to confirm our results (56). The results comparing the insular subregion masks and spherical ROIs were displayed in the supplementary materials (Figure S5 and Table S2 in Supplementary Material). The results of the differences were very similar to our results.
Head Motion
Head motion was defined by the mean frame-wise displacement (FD), as described Jenkinson et al. (57). The mean FD (Jenkinson) was calculated to estimate the voxel-wise motion differences between the two groups. The ADHD group and HC group did not differ significantly in the mean FD (p = 0.705).
Statistical Analysis
Within-Group Differences in the Connectivity of Insular Subregions
One-sample t-tests were performed on z-maps of each ROI to generated maps showing significant positive and negative RSFC by applying a gray matter mask implemented in DPABI template within each insular subregions in the adults with ADHD and the HC. A p < 0.05 uncorrected indicated that the voxel was significantly correlated with the ROIs. Furthermore, the regions with significant correlations with the ROIs in the two groups were combined to produce a mask for the next analysis.
Between-Group Differences in the Connectivity of the Insular Subregions
Two-sample t-tests were conducted between the ADHD and HC groups by applying masks which obtained from the significant regions of the combined one-sample t-test significant regions. Because of the exploratory nature, the significance of the statistical functional connectivity maps was p < 0.05 multiple comparisons using the voxel-wise non-parametric permutation test (5,000 permutations, no acceleration method) with threshold-free cluster enhancement [TFCE (58)] and family wise error corrected. Furthermore, the cluster size of no fewer than 40 voxels. TFCE is an approach for defining a cluster-like voxel-wise statistic in a way that is more natural and stable than the commonly used approach of an initial cluster-forming hard thresholding and gives generally better sensitivity than other methods (58). Besides, non-parametric permutation test is found to produce nominal results than other (59).
Correlation Calculation
Pearson’s correlation analyses were performed between the RSFC z-values of brain regions showing significant group differences and behavioral performances (working memory, inhibition, and shifting factor scores from the BRIEF) in the ADHD and HC groups. Owing to the exploratory nature of these correlation analyses, the statistical level of significance was set at p < 0.05, uncorrected.
Results
Demographic and Clinical Information
The demographics and clinical characteristics of the ADHD and HC groups were given in Table 1. The adults with ADHD showed significantly higher ADHD RS-IV scores and working memory, inhibition, and shifting factor scores than the adults in the HC group. No differences in sex, age, IQ, and mean FD were detected between the two groups.
Functional MRI Results
One sample t-tests displayed the within-group functional connectivity patterns in the ADHD and HC group (Figure 1; Figure S1 in Supplementary Material, respectively). Within the HC group, the functional connectivity patterns showed similar activity in the right and left hemisphere. A significantly positive functional connectivity with the bilateral AI was shown in the partial cortex, temporal lobe, and superior frontal gyrus. A significant negative functional connectivity with the bilateral AI was located in the occipital gyrus, temporal lobe and prefrontal cortex and limbic lobes. Adults with ADHD showed similar RSFC patterns of the bilateral MI and PI as the HCs.
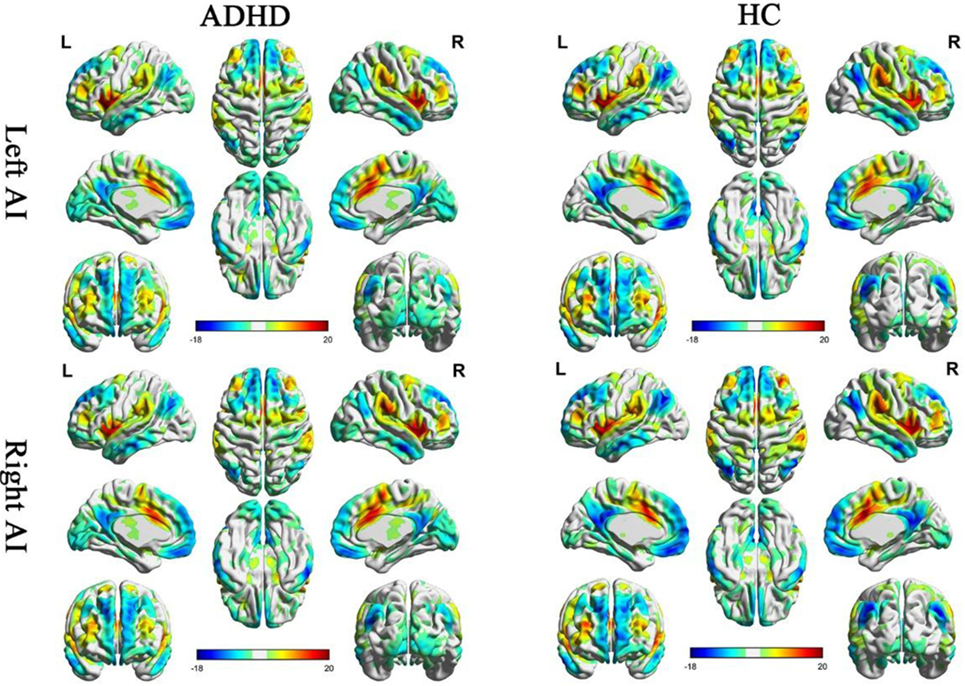
Figure 1. Significant resting-state functional connectivity (RSFC) patterns of the bilateral anterior insula of the attention deficit/hyperactivity disorder (ADHD) and healthy control (HC) groups. The yellow-red colors indicate that the brain regions were positively correlated with the insular subregions and the blue colors indicate that the brain regions were negatively correlated with insular subregions.
Between-Group Differences in the RSFC of the Insular Subregions
Anterior Insula (Table 2)
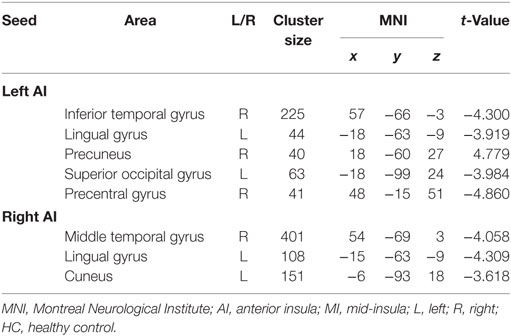
Table 2. Clusters displaying significant group differences in the RSFC of AI between the ADHD and HC groups.
The between-group RSFC with the left AI showed that the left AI had a positive RSFC with the right precuneus in adults with ADHD and a negative RSFC with the right precuneus in those in the HC group. The two groups showed different RSFC direction of the left AI in the right temporal gyrus, left lingual gyrus, left superior occipital gyrus, and right precentral gyrus. Specifically, the HC group displayed a positive RSFC and the patients with ADHD showed a negative RSFC between the AI and these brain regions shown above (Figure 2).
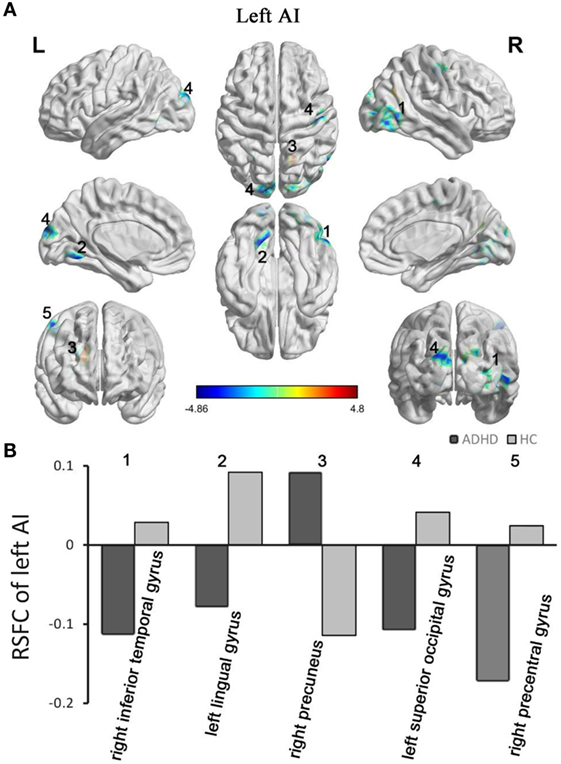
Figure 2. Group differences in RSFC of the left AI. Yellow color (ADHD > HC) indicates an increased functional connectivity with the insular subregions in the ADHD group, and blue color (ADHD < HC) indicates a decreased functional connectivity. (A) The clusters show significant differences in the RSFC of the left AI between the two groups. (B) Mean functional connectivity z-scores in clusters showing significant differences in the RSFC between the ADHD and HC groups. Numbers 1–5 marked in (A) refer to the brain regions in (B). RSFC, resting-state functional connectivity; AI, anterior insula; HC, healthy control; ADHD, attention deficit/hyperactivity disorder.
Adults with ADHD showed negative RSFC of the right AI with right middle temporal gyrus, left cuneus, and left lingual gyrus. However, the HCs showed positive RSFC with right AI in those regions (Figure 3).
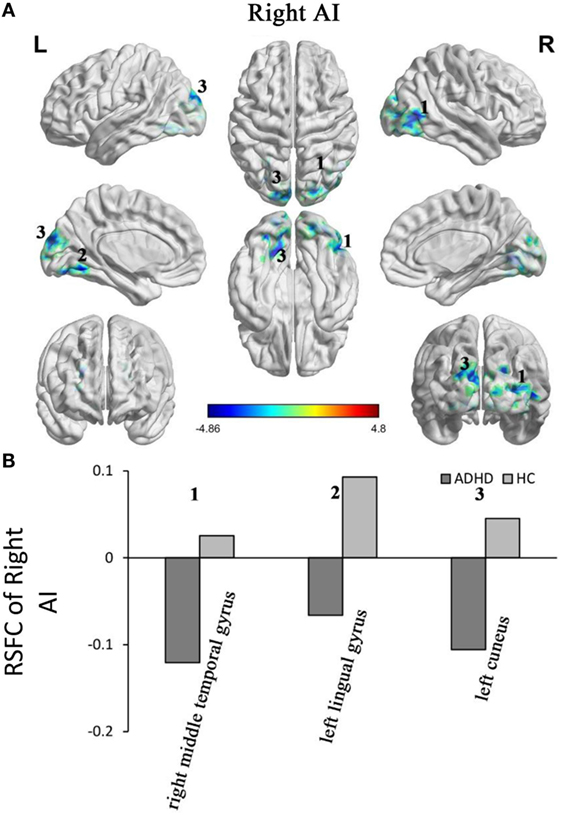
Figure 3. Group differences in the RSFC of the right AI. Yellow color (ADHD > HC) indicates an increased functional connectivity with the insular subregions in the ADHD group and blue color (ADHD < HC) indicates a decreased functional connectivity. (A) The clusters show significant differences in the RSFC of the left AI between the two groups. (B) Mean functional connectivity z-scores in clusters showing significant differences in the RSFC between the ADHD and HC groups. Numbers 1–5 marked in (A) refer to the brain regions in (B). RSFC, resting-state functional connectivity; AI, anterior insula; HC, healthy control; ADHD, attention deficit/hyperactivity disorder.
The results without GSR showed similar with results with GSR in RSFC of AI. Without GSR, bilateral AI with right precuneus showed altered RSFC in adults with ADHD. Patients with ADHD showed positive correlation and HCs showed negative correlation (Table S1 and Figures S2–S4 in Supplementary Material).
MI and PI
The RSFC of the other 4 ROIs, including those of the bilateral MI and PI, displayed no significant difference between the two groups in the results with GSR. There were significant differences between two groups within the results without GSR. Decreased RSFC were detected in adults with ADHD of right MI with right precuneus, left PI with right mid cingulate gyrus, right PI with right inferior temporal gyrus, right PI with right cerebellum and right PI with orbital frontal cortex (Table S1 and Figures S2–S4 in Supplementary Material).
Correlations with the Ecological EF Scores
The RSFC z-values of the brain regions showing significant differences between the two groups were used for further correlation analyses with the shifting, working memory and inhibition factor scores from the BRIEF-A. In the subjects in the HC group which only 27 participants were calculated the correlations, working memory scores were positively associated with the RSFC between the left AI and right precuneus (r = 0.557, p = 0.003). The RSFC of the left AI and right inferior temporal gyrus (r = −0.449, p = 0.032) and that of the left superior occipital gyrus (r = −0.512, p = 0.006) were negatively associated with the working memory scores. The working memory scores also showed a significant negative correlation with the RSFC of the right AI and left cuneus (r = −0.455, p = 0.017). However, there was no correlation between these variables in the ADHD group.
Discussion
This is the first study on the RSFC of the insular subregions and their correlations with ecological EF performance in adults with ADHD. First, compared with the HCs, adults with ADHD displayed general patterns of widespread altered RSFC between the AI and precuneus, occipital cortex, temporal cortex, lingual gyrus, and cuneus in the results with and without GSR. Second, within the HCs, the working memory scores were correlated with the RSFC of the bilateral AI with other brain regions. There were no significant differences of the RSFC of MI and PI in the results with GSR. However, in the results without GSR, patients with ADHD showed decreased positive functional connectivity in precuneus, temporal gyrus, cerebellum with MI and PI compared with HCs. These findings suggest that the overlapped altered functional connectivity of the AI and regions in the DMN in adults with ADHD in with GSR and without GSR may be involved in the aberrant EFs during daily life.
Altered RSFC between the left AI and right precuneus and between the bilateral AI and right medial temporal gyrus extended to the inferior temporal gyrus were observed in adults with ADHD compared with the HCs. Besides, the RSFC AI and right precuneus overlapped in the results with GSR and without GSR. The precuneus and medial temporal gyrus are two critical nodes of the DMN (60, 61) that exhibited consistently found abnormal connectivity and structure in patients with ADHD (10, 62–68). rs-fMRI studies have found decreased negative functional connectivity between the ACC, putamen, and regions in the DMN in ADHD patients (62, 67). According the previous studies, the precuneus is involved in episodic memory retrieval and self-centered mental imagery strategies (69, 70). The temporal lobe plays an important role in mnemonic processes (71). The DMN is involved in internal and external mental processing, such as attention, episodic memory retrieval, envisioning the future, and decision making (71). The abnormal RSFC between the key nodes of the SN and the DMN in patients with ADHD provides support for our prediction. The AI is involved in salience stimuli allocation and DMN disengagement, which play an important role in attention, working memory and higher order cognition (15, 22), and the altered RSFC may suggest that the AI fails to disengage the DMN or disengage it by mistake. Patients with ADHD are often easily distracted by unrelated stimuli (internal or external), which might indicate a misallocation of salience by the AI. This aberrant process uncovered by our results might lead to the EF defects. The results are also in line with recent resting-state studies examining SN abnormality in patients with ADHD (16, 72). Another study found that the improvement of ADHD symptom improvement in adults with ADHD by taking in amphetamine was linked to altered insula-medial prefrontal cortex (a core part of DMN) connectivity (73). These consistent results may suggest that the abnormal salience stimuli allocation procedure mediated by the AI, might be involved in the cognitive and EFs. We supposed that the impairment of the process in which the AI distributes different salient stimuli (internal or external) potentially results in the different subtypes of ADHD. Future studies are needed to verify this hypothesis.
We still identified an altered RSFC of the bilateral AI with left lingual gyrus. The lingual gyrus participates in visual phonological and word processing (74, 75). rs-fMRI and event-related fMRI studies have observed a lingual gyrus abnormality in patients with ADHD (26). Ko et al. identified a higher activation in the lingual area during a working memory dual task, which supports our findings that the lingual gyrus may involve in working memory process. The altered RSFC of bilateral AI with left lingual gyrus did not detect in the results without GSR in patients with ADHD. Future studies should therefore include the reason leading to the inconsistent results with and without GSR.
Our findings identified that the working memory factor scores were positively associated with the RSFC between the left AI and precuneus and that they were negatively correlated with the left AI and right temporal lobe in the HC group. The correlation between the working memory scores and the RSFC of the right AI and right temporal lobe exist with a tendency (p = 0.062). There was no correlation of these variables in the ADHD group. The results identified that participants with a higher RSFC (positive and negative) had better working memory performance during daily life in the HC group. However, these associations between working memory and the RSFC were disrupted in ADHD group. The disrupted correlation might influence the working memory procedure and therefore suggest working memory impairment. Based on the previous studies (15, 22), we speculate that salient stimuli were transferred to the AI and that the AI conveyed altered control signals to other large-scale networks related to working memory resources, involved in the working memory impairment in patients with ADHD.
We also found the altered direction in the RSFC of the left AI with the right precentral gyrus in adults with ADHD compared with the HCs. The precentral gyrus, which is implicated in multiple EFs, such as sustained attention, working memory and response inhibition, and task switching is part of the motor cortex (24, 76–78). We supposed that the abnormal allocation of salient stimuli in the AI is involved an aberrant bottom-up control of the precentral gyrus and then results in attention or EF symptoms. However, there was no correlation between the EF factor scores and the RSFC of the left AI with the right precentral in either of the groups. This discrepancy may be due to differences the experimental conditions (“resting-state” vs. “inhibiting task” or “task switching task”).
We did not identify differences in the RSFC of the MI and PI with the other brain regions between the two groups in the results with GSR. And there were some differences between two groups in the results without GSR. These results may suggest that AI which had overlapped results in two methods deficits play a major role in the ADHD mechanism. However, future studies are needed to replicate these results.
Several studies have raised concerns regarding the anticorrelated networks after GSR (52, 79), and others have deemed the anticorrelated network a true neural signal (80). GSR generally affects between-group analyses in complicated ways. In this situation, there will be distinct results between the with GSR and without GSR analyses. We completed another analysis without GSR, and the between-group difference patterns were altered without GSR. There are some differences between the two groups in the RSFC of the insular subregions (Table S1 and Figures S2–S4 in Supplementary Material). Consistent with previous studies with GSR that found an anticorrelation with the precuneus or posterior cingulate gyrus (63, 67), we found an anticorrelation of the AI and precuneus in the HCs. As shown in the figure, the anticorrelation between the AI and precuneus showed in the results after GSR decreased without GSR. Therefore, our findings should be interpreted with caution.
There are several limitations of this study that should be considered. First, our participants included patients with two subtypes of ADHD (ADHD-I and ADHD-C). To explore fully how the ADHD subtypes moderate such relationships, a separate study with a much larger sample is needed. Furthermore, the IQs of the participants of the two groups in our study were high. Though the IQs of the two groups had no significant statistical differences, a high IQ may cover up some other differences in adults with ADHD. Next, 6 of the 28 participants in the ADHD group had received medication treatment before the assessment. However, after excluding these six patients, the results did not change substantially and our conclusion was not influenced. Finally, the results of the without GSR analyses (Table S1 and Figures S2–S4 in Supplementary Material) showed different pattern with the results using GSR. Therefore, our findings should be interpreted with some caution and need further replication with regard to the abovementioned limitations.
In summary, this study was the first to detect the abnormality of the insular subregions’ functional networks among adults with ADHD and determined that the EF factor scores are associated with the RSFC of the AI with the precuneus and temporal gyrus in the HC group. However, there is no correlation of the EF factor and with the RSFC of the AI in the ADHD group. The normal correlations between the working memory and RSFC of the AI were disrupted in adults with ADHD. The results indicate that disrupted SN-DMN networks might be involved in EF impairment in adults with ADHD, especially working memory. Our findings suggest that deficits of AI particularly, which refer to abnormal salient stimuli allocation, might be involved in working memory impairment in patients with ADHD. The inconsistent results of MI and PI between results with and without GSR need further exploration.
Ethics Statement
This study was approved by the Research Ethics Review Board of Peking University Sixth Hospital. All subjects were fully informed about the research before being asked to sign the informed consent form.
Author Contributions
QZ and LS contributed to the design of the work. QZ, HL, XY, FH, YW, QQ, LS, LL, and YW were involved in the acquisition of the data. QZ, HL, XY, and LS participated in the analysis and interpretation of the data. QZ and LS drafted the work. LL, YZ, QC, and YW revised it critically.
Conflict of Interest Statement
The authors declare that the research was conducted in the absence of any commercial or financial relationships that could be construed as a potential conflict of interest.
Acknowledgments
We thank all the participants in our research.
Funding
This work was supported by the National Natural Sciences Foundation of China (81371496), the National Basic Research Program of China (973 program 2014CB846104), the National Key Technology R&D Program (2015BAI13B01), and the National Natural Sciences Foundation of China (81771479, 81471382, and 81641163).
Supplementary Material
The Supplementary Material for this article can be found online at http://journal.frontiersin.org/article/10.3389/fpsyt.2017.00200/full#supplementary-material.
References
1. American Psychiatric Association. Diagnostic and Statistical Manual of Mental Disorders: Text Revision. 4th ed. Washington, DC: American Psychiatric Association (2000).
2. Thomas R, Sanders S, Doust J, Beller E, Glasziou P. Prevalence of attention-deficit/hyperactivity disorder: a systematic review and meta-analysis. Pediatrics (2015) 135(4):e994–1001. doi:10.1542/peds.2014-3482
3. Karam RG, Breda V, Picon FA, Rovaris DL, Victor MM, Salgado CA, et al. Persistence and remission of ADHD during adulthood: a 7-year clinical follow-up study. Psychol Med (2015) 45(10):2045–56. doi:10.1017/S0033291714003183
4. Moffitt TE, Houts R, Asherson P, Belsky DW, Corcoran DL, Hammerle M, et al. Is adult ADHD a childhood-onset neurodevelopmental disorder? Evidence from a four-decade longitudinal cohort study. Am J Psychiatry (2015) 172(10):967–77. doi:10.1176/appi.ajp.2015.14101266
5. Uchida M, Spencer TJ, Faraone SV, Biederman J. Adult outcome of ADHD: an overview of results from the MGH longitudinal family studies of pediatrically and psychiatrically referred youth with and without ADHD of both sexes. J Atten Disord (2015). doi:10.1177/1087054715604360
6. Alderson RM, Patros CHG, Tarle SJ, Hudec KL, Kasper LJ, Lea SE. Working memory and behavioral inhibition in boys with ADHD: an experimental examination of competing models. Child Neuropsychol (2015) 23(3):255–72. doi:10.1080/09297049.2015.1105207
7. Hart H, Radua J, Nakao T, Mataix-Cols D, Rubia K. Meta-analysis of functional magnetic resonance imaging studies of inhibition and attention in attention-deficit/hyperactivity disorder. JAMA Psychiatry (2013) 70(2):185. doi:10.1001/jamapsychiatry.2013.277
8. Rubia K, Overmeyer S, Taylor E, Brammer M, Williams SCR, Simmons A, et al. Hypofrontality in attention deficit hyperactivity disorder during higher-order motor control: a study with functional MRI. Am J Psychiatry (1999) 156(6):891–6. doi:10.1176/ajp.156.6.891
9. Ma I, van Duijvenvoorde A, Scheres A. The interaction between reinforcement and inhibitory control in ADHD: a review and research guidelines. Clin Psychol Rev (2016) 44:94–111. doi:10.1016/j.cpr.2016.01.001
10. Sidlauskaite J, Sonuga-Barke E, Roeyers H, Wiersema JR. Default mode network abnormalities during state switching in attention deficit hyperactivity disorder. Psychol Med (2015) 46(03):519–28. doi:10.1017/s0033291715002019
11. Diamond A. Executive functions. Annu Rev Psychol (2013) 64(1):135–68. doi:10.1146/annurev-psych-113011-143750
12. Barkley RA, Murphy KR. Impairment in occupational functioning and adult ADHD: the predictive utility of executive function (EF) ratings versus EF tests. Arch Clin Neuropsychol (2010) 25(3):157–73. doi:10.1093/arclin/acq014
13. Roth RM, Isquith PK, Gioia G. Behavioral Rating Inventory of Executive Function – Adult Version (BRIEF-A) Professional Manual. Lutz, FL: Psychological Assessment Resources (2005).
14. Castellanos FX, Proal E. Large-scale brain systems in ADHD: beyond the prefrontal-striatal model. Trends Cogn Sci (2012) 16(1):17–26. doi:10.1016/j.tics.2011.11.007
15. Menon V. Large-scale brain networks and psychopathology: a unifying triple network model. Trends Cogn Sci (2011) 15(10):483–506. doi:10.1016/j.tics.2011.08.003
16. Sidlauskaite J, Sonuga-Barke E, Roeyers H, Wiersema JR. Altered intrinsic organisation of brain networks implicated in attentional processes in adult attention-deficit/hyperactivity disorder: a resting-state study of attention, default mode and salience network connectivity. Eur Arch Psychiatry Clin Neurosci (2016) 266(4):349–57. doi:10.1007/s00406-015-0630-0
17. Bush G, Frazier JA, Rauch SL, Seidman LJ, Whalen PJ, Jenike MA, et al. Anterior cingulate cortex dysfunction in attention-deficit/hyperactivity disorder revealed by fMRI and the counting Stroop. Biol Psychiatry (1999) 45(12):1542–52. doi:10.1016/S0006-3223(99)00083-9
18. Konrad K, Neufang S, Hanisch C, Fink GR, Herpertz-Dahlmann B. Dysfunctional attentional networks in children with attention deficit/hyperactivity disorder: evidence from an event-related functional magnetic resonance imaging study. Biol Psychiatry (2006) 59(7):643–51. doi:10.1016/j.biopsych.2005.08.013
19. Lopez-Larson MP, King JB, Terry J, McGlade EC, Yurgelun-Todd D. Reduced insular volume in attention deficit hyperactivity disorder. Psychiatry Res (2012) 204(1):32–9. doi:10.1016/j.pscychresns.2012.09.009
20. Tian L, Jiang T, Wang Y, Zang Y, He Y, Liang M, et al. Altered resting-state functional connectivity patterns of anterior cingulate cortex in adolescents with attention deficit hyperactivity disorder. Neurosci Lett (2006) 400(1–2):39–43. doi:10.1016/j.neulet.2006.02.022
21. Vasic N, Plichta MM, Wolf RC, Fallgatter AJ, Sosic-Vasic Z, Gron G. Reduced neural error signaling in left inferior prefrontal cortex in young adults with ADHD. J Atten Disord (2014) 18(8):659–70. doi:10.1177/1087054712446172
22. Menon V, Uddin LQ. Saliency, switching, attention and control: a network model of insula function. Brain Struct Funct (2010) 214(5–6):655–67. doi:10.1007/s00429-010-0262-0
23. Ernst M, Kimes AS, London ED, Matochik JA, Eldreth D, Tata S, et al. Neural substrates of decision making in adults with attention deficit hyperactivity disorder. Am J Psychiatry (2003) 160(6):1061–70. doi:10.1176/appi.ajp.160.6.1061
24. Lei D, Du M, Wu M, Chen T, Huang X, Du X, et al. Functional MRI reveals different response inhibition between adults and children with ADHD. Neuropsychology (2015) 29(6):874–81. doi:10.1037/neu0000200
25. Rubia K, Alegria AA, Cubillo AI, Smith AB, Brammer MJ, Radua J. Effects of stimulants on brain function in attention-deficit/hyperactivity disorder: a systematic review and meta-analysis. Biol Psychiatry (2014) 76(8):616–28. doi:10.1016/j.biopsych.2013.10.016
26. Ko CH, Hsieh TJ, Wang PW, Lin WC, Chen CS, Yen JY. The altered brain activation of phonological working memory, dual tasking, and distraction among participants with adult ADHD and the effect of the MAOA polymorphism. J Atten Disord (2015). doi:10.1177/1087054715572609
27. Hulvershorn LA, Mennes M, Castellanos FX, Di Martino A, Milham MP, Hummer TA, et al. Abnormal amygdala functional connectivity associated with emotional lability in children with attention-deficit/hyperactivity disorder. J Am Acad Child Adolesc Psychiatry (2014) 53(3):351–61.e351. doi:10.1016/j.jaac.2013.11.012
28. Yu X, Liu L, Chen W, Cao Q, Zepf FD, Ji G, et al. Integrity of amygdala subregion-based functional networks and emotional lability in drug-naive boys with ADHD. J Atten Disord (2016). doi:10.1177/1087054716661419
29. Augustine JR. The insular lobe in primates including humans. Neurol Res (1985) 7(1):2–10. doi:10.1080/01616412.1985.11739692
30. Deen B, Pitskel NB, Pelphrey KA. Three systems of insular functional connectivity identified with cluster analysis. Cereb Cortex (2010) 21(7):1498–506. doi:10.1093/cercor/bhq186
31. Ichesco E, Schmidt-Wilcke T, Bhavsar R, Clauw DJ, Peltier SJ, Kim J, et al. Altered resting state connectivity of the insular cortex in individuals with fibromyalgia. J Pain (2014) 15(8):815–26.e811. doi:10.1016/j.jpain.2014.04.007
32. Craig AD. How do you feel – now? The anterior insula and human awareness. Nat Rev Neurosci (2009) 10(1):59–70. doi:10.1038/nrn2555
33. Goulden N, Khusnulina A, Davis NJ, Bracewell RM, Bokde AL, McNulty JP, et al. The salience network is responsible for switching between the default mode network and the central executive network: replication from DCM. Neuroimage (2014) 99:180–90. doi:10.1016/j.neuroimage.2014.05.052
34. Kurth F, Zilles K, Fox PT, Laird AR, Eickhoff SB. A link between the systems: functional differentiation and integration within the human insula revealed by meta-analysis. Brain Struct Funct (2010) 214(5–6):519–34. doi:10.1007/s00429-010-0255-z
35. Craig AD. Emotional moments across time: a possible neural basis for time perception in the anterior insula. Philos Trans R Soc Lond B Biol Sci (2009) 364(1525):1933–42. doi:10.1098/rstb.2009.0008
36. Chen X, Duan M, He H, Yang M, Klugah-Brown B, Xu H, et al. Functional abnormalities of the right posterior insula are related to the altered self-experience in schizophrenia. Psychiatry Res (2016) 256:26–32. doi:10.1016/j.pscychresns.2016.09.006
37. Shepherd AM, Matheson SL, Laurens KR, Carr VJ, Green MJ. Systematic meta-analysis of insula volume in schizophrenia. Biol Psychiatry (2012) 72(9):775–84. doi:10.1016/j.biopsych.2012.04.020
38. Takahashi T, Suzuki M, Zhou SY, Hagino H, Tanino R, Kawasaki Y, et al. Volumetric MRI study of the short and long insular cortices in schizophrenia spectrum disorders. Psychiatry Res (2005) 138(3):209–20. doi:10.1016/j.pscychresns.2005.02.004
39. Adleman NE, Fromm SJ, Razdan V, Kayser R, Dickstein DP, Brotman MA, et al. Cross-sectional and longitudinal abnormalities in brain structure in children with severe mood dysregulation or bipolar disorder. J Child Psychol Psychiatry (2012) 53(11):1149–56. doi:10.1111/j.1469-7610.2012.02568.x
40. Liu CH, Jing B, Ma X, Xu PF, Zhang Y, Li F, et al. Voxel-based morphometry study of the insular cortex in female patients with current and remitted depression. Neuroscience (2014) 262:190–9. doi:10.1016/j.neuroscience.2013.12.058
41. Takahashi T, Yucel M, Lorenzetti V, Tanino R, Whittle S, Suzuki M, et al. Volumetric MRI study of the insular cortex in individuals with current and past major depression. J Affect Disord (2010) 121(3):231–8. doi:10.1016/j.jad.2009.06.003
42. Tang LR, Liu CH, Jing B, Ma X, Li HY, Zhang Y, et al. Voxel-based morphometry study of the insular cortex in bipolar depression. Psychiatry Res (2014) 224(2):89–95. doi:10.1016/j.pscychresns.2014.08.004
43. Yamada T, Itahashi T, Nakamura M, Watanabe H, Kuroda M, Ohta H, et al. Altered functional organization within the insular cortex in adult males with high-functioning autism spectrum disorder: evidence from connectivity-based parcellation. Mol Autism (2016) 7:41. doi:10.1186/s13229-016-0106-8
44. Nicholson AA, Sapru I, Densmore M, Frewen PA, Neufeld RWJ, Théberge J, et al. Unique insula subregion resting-state functional connectivity with amygdala complexes in posttraumatic stress disorder and its dissociative subtype. Psychiatry Res (2016) 250:61–72. doi:10.1016/j.pscychresns.2016.02.002
45. Zhang Y, Xie B, Chen H, Li M, Guo X, Chen H. Disrupted resting-state insular subregions functional connectivity in post-traumatic stress disorder. Medicine (2016) 95(27):e4083. doi:10.1097/md.0000000000004083
46. First MB, Spitzer RL, Gibbon M, Williams JB. Structured Clinical Interview for DSM-IV® Axis I Disorders (SCID-I), Clinician Version. Washington, DC: American Psychiatric Publishing (1996).
47. Conners CK, Erhardt D, Sparrow E. Conner’s Adult ADHD Rating Scales: CAARS. Toronto: MHS (1999).
48. DuPaul GPT, Anastopoulos A, Reid R. ADHD Rating Scale-IV: Checklists, Norms, and Clinical Interpretation. New York: Guilford (1998).
49. Yan CG, Wang XD, Zuo XN, Zang YF. DPABI: data processing & analysis for (resting-state) brain imaging. Neuroinformatics (2016) 14(3):339–51. doi:10.1007/s12021-016-9299-4
50. Ashburner J. A fast diffeomorphic image registration algorithm. Neuroimage (2007) 38(1):95–113. doi:10.1016/j.neuroimage.2007.07.007
51. Liu TT, Nalci A, Falahpour M. The global signal in fMRI: nuisance or information? Neuroimage (2017) 150:213–29. doi:10.1016/j.neuroimage.2017.02.036
52. Weissenbacher A, Kasess C, Gerstl F, Lanzenberger R, Moser E, Windischberger C. Correlations and anticorrelations in resting-state functional connectivity MRI: a quantitative comparison of preprocessing strategies. Neuroimage (2009) 47(4):1408–16. doi:10.1016/j.neuroimage.2009.05.005
53. Yang GJ, Murray JD, Repovs G, Cole MW, Savic A, Glasser MF, et al. Altered global brain signal in schizophrenia. Proc Natl Acad Sci U S A (2014) 111(20):7438–43. doi:10.1073/pnas.1405289111
54. Taylor KS, Seminowicz DA, Davis KD. Two systems of resting state connectivity between the insula and cingulate cortex. Hum Brain Mapp (2009) 30(9):2731–45. doi:10.1002/hbm.20705
55. Xia M, Wang J, He Y. BrainNet viewer: a network visualization tool for human brain connectomics. PLoS One (2013) 8(7):e68910. doi:10.1371/journal.pone.0068910
56. Kelly C, Toro R, Di Martino A, Cox CL, Bellec P, Castellanos FX, et al. A convergent functional architecture of the insula emerges across imaging modalities. Neuroimage (2012) 61(4):1129–42. doi:10.1016/j.neuroimage.2012.03.021
57. Jenkinson M, Bannister P, Brady M, Smith S. Improved optimization for the robust and accurate linear registration and motion correction of brain images. Neuroimage (2002) 17(2):825–41. doi:10.1006/nimg.2002.1132
58. Smith SM, Nichols TE. Threshold-free cluster enhancement: addressing problems of smoothing, threshold dependence and localisation in cluster inference. Neuroimage (2009) 44(1):83–98. doi:10.1016/j.neuroimage.2008.03.061
59. Eklund A, Nichols TE, Knutsson H. Cluster failure: why fMRI inferences for spatial extent have inflated false-positive rates. Proc Natl Acad Sci U S A (2016) 113(28):7900–5. doi:10.1073/pnas.1602413113
60. Fransson P. Spontaneous low-frequency BOLD signal fluctuations: an fMRI investigation of the resting-state default mode of brain function hypothesis. Hum Brain Mapp (2005) 26(1):15–29. doi:10.1002/hbm.20113
61. Greicius MD, Krasnow B, Reiss AL, Menon V. Functional connectivity in the resting brain: a network analysis of the default mode hypothesis. Proc Natl Acad Sci U S A (2003) 100(1):253–8. doi:10.1073/pnas.0135058100
62. Cao X, Cao Q, Long X, Sun L, Sui M, Zhu C, et al. Abnormal resting-state functional connectivity patterns of the putamen in medication-naive children with attention deficit hyperactivity disorder. Brain Res (2009) 1303:195–206. doi:10.1016/j.brainres.2009.08.029
63. Castellanos FX, Margulies DS, Kelly C, Uddin LQ, Ghaffari M, Kirsch A, et al. Cingulate-precuneus interactions: a new locus of dysfunction in adult attention-deficit/hyperactivity disorder. Biol Psychiatry (2008) 63(3):332–7. doi:10.1016/j.biopsych.2007.06.025
64. Helps SK, Broyd SJ, James CJ, Karl A, Chen W, Sonuga-Barke EJ. Altered spontaneous low frequency brain activity in attention deficit/hyperactivity disorder. Brain Res (2010) 1322:134–43. doi:10.1016/j.brainres.2010.01.057
65. Oldehinkel M, Beckmann CF, Franke B, Hartman CA, Hoekstra PJ, Oosterlaan J, et al. Functional connectivity in cortico-subcortical brain networks underlying reward processing in attention-deficit/hyperactivity disorder. Neuroimage Clin (2016) 12:796–805. doi:10.1016/j.nicl.2016.10.006
66. Sidlauskaite J, Wiersema JR, Roeyers H, Krebs RM, Vassena E, Fias W, et al. Anticipatory processes in brain state switching—evidence from a novel cued-switching task implicating default mode and salience networks. Neuroimage (2014) 98:359–65. doi:10.1016/j.neuroimage.2014.05.010
67. Sun L, Cao Q, Long X, Sui M, Cao X, Zhu C, et al. Abnormal functional connectivity between the anterior cingulate and the default mode network in drug-naive boys with attention deficit hyperactivity disorder. Psychiatry Res (2012) 201(2):120–7. doi:10.1016/j.pscychresns.2011.07.001
68. Wilson TW, Franzen JD, Heinrichs-Graham E, White ML, Knott NL, Wetzel MW. Broadband neurophysiological abnormalities in the medial prefrontal region of the default-mode network in adults with ADHD. Hum Brain Mapp (2013) 34(3):566–74. doi:10.1002/hbm.21459
69. Cavanna AE, Trimble MR. The precuneus: a review of its functional anatomy and behavioural correlates. Brain (2006) 129(Pt 3):564–83. doi:10.1093/brain/awl004
70. Margulies DS, Vincent JL, Kelly C, Lohmann G, Uddin LQ, Biswal BB, et al. Precuneus shares intrinsic functional architecture in humans and monkeys. Proc Natl Acad Sci U S A (2009) 106(47):20069–74. doi:10.1073/pnas.0905314106
71. Buckner RL, Andrews-Hanna JR, Schacter DL. The brain’s default network: anatomy, function, and relevance to disease. Ann N Y Acad Sci (2008) 1124:1–38. doi:10.1196/annals.1440.011
72. Cai W, Chen T, Szegletes L, Supekar K, Menon V. Aberrant cross-brain network interaction in children with attention-deficit/hyperactivity disorder and its relation to attention deficits: a multisite and cross-site replication study. Biol Psychiatry (2015). doi:10.1016/j.biopsych.2015.10.017
73. Yang Z, Kelly C, Castellanos FX, Leon T, Milham MP, Adler LA. Neural correlates of symptom improvement following stimulant treatment in adults with attention-deficit/hyperactivity disorder. J Child Adolesc Psychopharmacol (2016) 26(6):527–36. doi:10.1089/cap.2015.0243
74. Burton MW, Locasto PC, Krebs-Noble D, Gullapalli RP. A systematic investigation of the functional neuroanatomy of auditory and visual phonological processing. Neuroimage (2005) 26(3):647–61. doi:10.1016/j.neuroimage.2005.02.024
75. Mechelli A, Humphreys GW, Mayall K, Olson A, Price CJ. Differential effects of word length and visual contrast in the fusiform and lingual gyri during reading. Proc Biol Sci (2000) 267(1455):1909–13. doi:10.1098/rspb.2000.1229
76. Dibbets P, Evers EA, Hurks PP, Bakker K, Jolles J. Differential brain activation patterns in adult attention-deficit hyperactivity disorder (ADHD) associated with task switching. Neuropsychology (2010) 24(4):413–23. doi:10.1037/a0018997
77. Suskauer SJ, Simmonds DJ, Fotedar S, Blankner JG, Pekar JJ, Denckla MB, et al. Functional magnetic resonance imaging evidence for abnormalities in response selection in attention deficit hyperactivity disorder: differences in activation associated with response inhibition but not habitual motor response. J Cogn Neurosci (2008) 20(3):478–93. doi:10.1162/jocn.2008.20032
78. Villemonteix T, De Brito SA, Kavec M, Baleriaux D, Metens T, Slama H, et al. Grey matter volumes in treatment naive vs. chronically treated children with attention deficit/hyperactivity disorder: a combined approach. Eur Neuropsychopharmacol (2015) 25(8):1118–27. doi:10.1016/j.euroneuro.2015.04.015
79. Murphy K, Birn RM, Handwerker DA, Jones TB, Bandettini PA. The impact of global signal regression on resting state correlations: are anti-correlated networks introduced? Neuroimage (2009) 44(3):893–905. doi:10.1016/j.neuroimage.2008.09.036
Keywords: attention deficit/hyperactivity disorder, insular subregions, resting-state functional connectivity, executive function, salience network, default mode network
Citation: Zhao Q, Li H, Yu X, Huang F, Wang Y, Liu L, Cao Q, Qian Q, Zang Y, Sun L and Wang Y (2017) Abnormal Resting-State Functional Connectivity of Insular Subregions and Disrupted Correlation with Working Memory in Adults with Attention Deficit/Hyperactivity Disorder. Front. Psychiatry 8:200. doi: 10.3389/fpsyt.2017.00200
Received: 07 July 2017; Accepted: 25 September 2017;
Published: 11 October 2017
Edited by:
Jeffrey Robert Strawn, University of Cincinnati, United StatesReviewed by:
Hilary Marusak, Wayne State University, United StatesWi Hoon Jung, Korea University, South Korea
Copyright: © 2017 Zhao, Li, Yu, Huang, Wang, Liu, Cao, Qian, Zang, Sun and Wang. This is an open-access article distributed under the terms of the Creative Commons Attribution License (CC BY). The use, distribution or reproduction in other forums is permitted, provided the original author(s) or licensor are credited and that the original publication in this journal is cited, in accordance with accepted academic practice. No use, distribution or reproduction is permitted which does not comply with these terms.
*Correspondence: Li Sun, sunlioh@bjmu.edu.cn