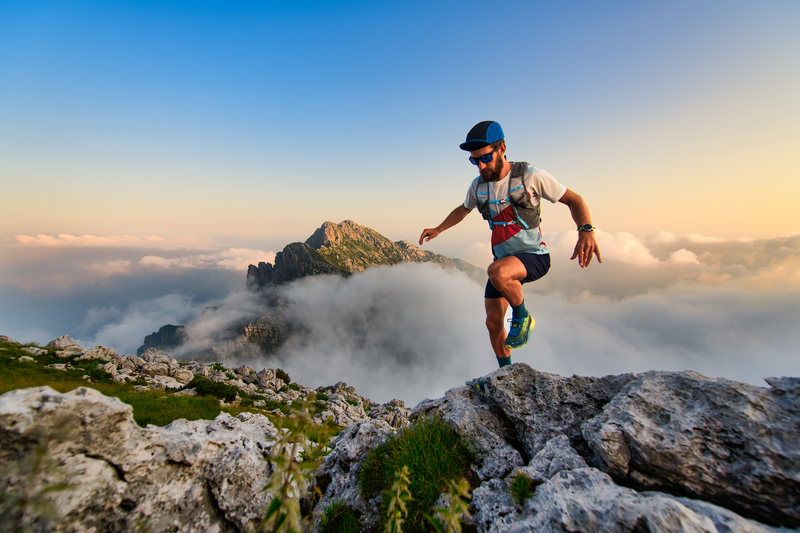
94% of researchers rate our articles as excellent or good
Learn more about the work of our research integrity team to safeguard the quality of each article we publish.
Find out more
REVIEW article
Front. Psychiatry , 25 March 2014
Sec. Schizophrenia
Volume 5 - 2014 | https://doi.org/10.3389/fpsyt.2014.00030
This article is part of the Research Topic Neuropsychopharmacology of Psychosis: Relation of Brain Signals, Cognition and Chemistry View all 20 articles
Computational modeling of functional brain networks in fMRI data has advanced the understanding of higher cognitive function. It is hypothesized that functional networks mediating higher cognitive processes are disrupted in people with schizophrenia. In this article, we review studies that applied measures of functional and effective connectivity to fMRI data during cognitive tasks, in particular working memory fMRI studies. We provide a conceptual summary of the main findings in fMRI data and their relationship with neurotransmitter systems, which are known to be altered in individuals with schizophrenia. We consider possible developments in computational neuropsychiatry, which are likely to further our understanding of how key functional networks are altered in schizophrenia.
Schizophrenia is a severe psychiatric disorder, which is initially manifested through positive symptoms including delusions, hallucinations, and disorganized thoughts. As the illness progresses negative symptoms such as avolition, alogia, and apathy may occur. Prior to diagnosis of illness, cognitive deficits can occur and illness progression can also be associated with cognitive deficits (1, 2). It is widely established that such cognitive deficits are considered a core symptom of schizophrenia (3) and are associated with reductions in working memory performance. Working memory deficits are one of the main neurocognitive impairments found in subjects with first episode schizophrenia (FES) (4, 5) and in people with established schizophrenia (EST) (6). Similar deficits also occur in individuals at high risk of schizophrenia [HR; Ref. (2)]. Furthermore, recent evidence has been presented, which indicates a relationship between severity of working memory deficits and the severity of negative symptoms (7). The severity of working memory deficits that is evident at the first episode of schizophrenia can predict the quality of life at the established stage of the illness (8, 9).
Two major neurotransmitter circuits have been implicated in clinical and cognitive symptoms in subjects with schizophrenia: these are the dopamine and glutamate neurotransmitter circuits. Evidence has been presented for separate alterations/disruptions of dopamine and glutamate as well as an interactive role between both neurotransmitters1. The two main neurobiological hypotheses in schizophrenia are based on the theories of altered dopaminergic transmission (“dopamine hypothesis of schizophrenia”) and altered glutamatergic transmission (“glutamate hypothesis of schizophrenia”). It is thought that both dopamine and glutamate modulate the dorsolateral prefrontal cortex (DLPFC) and in schizophrenia alter the performance in cognitive processes such as in working memory (10–13). Such work supports the notion of schizophrenia as a brain disorder. FMRI and positron emission tomography (PET) findings of altered functional activation and functional connectivity (FC) during working memory have been reported in people with schizophrenia when they are compared to healthy controls (14, 15). Furthermore, PET studies have presented evidence for indirect markers of altered dopamine transmission, which was correlated with working memory performance (2, 16). Alterations of indirect measures of glutamate concentrations have been reported by proton magnetic resonance spectroscopy (MRS) studies (17).
One subfield within the emerging field of computational neuropsychiatry is based on modeling fMRI networks and the evidence of (i) altered dopaminergic and/or glutamatergic transmission in (ii) cognitive function (i.e., working memory) in people with schizophrenia. Therefore, the objectives are the investigation of impaired cognitive function mediated by large-scale networks in combination with underlying neurobiological circuits such as dopamine and glutamate. Researchers in computational neuropsychiatry examine and model altered cognitive brain function in terms of functionally integrated regions [i.e., effective connectivity (EC)] (18), which may be mediated by genetic factors and neurotransmitter circuits (19–21). Mechanistic responses can be inferred from the computational modeling of cognitive brain function where the localized brain function is monitored through the BOLD response (22). This modeling approach allows computational neuropsychiatry to further our understanding of the neurobiological processes, which underlie altered cognitive brain function in individuals with schizophrenia. Thus, advancing our knowledge of schizophrenia as a cognitive brain network disorder.
In this review, we summarize fMRI findings in verbal/numeric working memory2 in context of (i) the understanding of schizophrenia as a cognitive brain disorder (from clinical and cognitive neurosciences) and (ii) the understanding of schizophrenia as a cognitive brain network disorder (from computational neuropsychiatry). We discuss these sets of findings in context of the dopamine and the glutamate hypotheses of schizophrenia. We consider two key research questions for the discussion of each set of findings:
(i) To what extent do these sets of findings support the dopamine hypothesis and/or the glutamate hypothesis in subjects with schizophrenia?
(ii) Do the findings from computational neuropsychiatry lead to a better understanding of schizophrenia than that obtained from clinical and cognitive neurosciences?
The review is structured as followed: first, the dopamine and glutamate hypotheses of schizophrenia are summarized (Section Schizophrenia as a Brain Disorder). Second, exemplary findings of verbal/numeric working memory deficits from fMRI studies in subjects with schizophrenia are summarized. These findings are discussed in context of the dopamine hypothesis and the glutamate hypothesis of schizophrenia (Section Schizophrenia as a Cognitive Brain Disorder). Third, we present a brief introduction to computational neuropsychiatry. We provide examples from computational neuropsychiatry and the application to the investigation of cognitive brain large-scale networks in people with schizophrenia3. Finally, we consider current methodological limitations of the methods (Section From Computational Neuropsychiatry Towards Schizophrenia as a Cognitive Brain Network Disorder). We outline potential future influences of computational advances in schizophrenia that may shape our understanding of schizophrenia with the aim of developing more effective treatments for the disorder (Section Understanding of Schizophrenia).
Neurobiological research into alterations of dopaminergic and/or glutamatergic neurotransmission has paved the way for the understanding of schizophrenia as a disorder of the brain. The dopamine hypothesis posits that dopamine function is altered in schizophrenia and that this dysfunction may be the pathophysiological pathway leading to clinical and cognitive symptoms (23, 24). The glutamate hypothesis proposes that the altered dopaminergic dysfunction may be secondary to aberrant glutamatergic dysregulation, which may contribute to clinical and cognitive symptoms in schizophrenia (25–27).
The origin of the dopamine hypothesis of schizophrenia is based on the discovery of antipsychotic drugs by Delay et al. (28) in 1952. Carlsson and Lindqvit reported the first findings of an effect of antipsychotic drugs on the metabolism of dopamine (29). The dopamine hypothesis posits that alterations of dopaminergic receptors may underlie the clinical symptoms of schizophrenia (30). Over last three decades, the dopamine hypothesis of schizophrenia has undergone reformulations in light of newly available preclinical and clinical findings. Here, we consider the three main hypotheses: (i) the “dopamine receptor hypothesis,” (ii) the “modified dopamine hypothesis of schizophrenia,” and (iii) the “dopamine hypothesis: version III.”
The dopamine receptor hypothesis goes back to studies reporting antipsychotics affecting the affinity of dopamine receptors (31–33). Further evidence for the hypothesis was presented with increased synaptic monoamine levels during the induction of psychotic symptoms (34). The focus of this hypothesis rests on the excess of dopamine receptors. Thus, the clinical treatment is aimed at blocking the dopamine D2 subtype of the dopamine receptors (35).
The modified dopamine hypothesis of schizophrenia has been formulated to integrate new findings (36). Preclinical and clinical studies (i.e., post-mortem, metabolite, and dopamine receptor neuroimaging studies) have advanced the understanding of relationships between affinity and occupancy of D2 and D1 subtypes of the dopamine receptors and regional specificity (37). Furthermore, it was assumed that findings of altered regional dopaminergic receptor function from preclinical and indirect clinical studies could be linked to clinical symptomatology in schizophrenia (36). The hypothesis suggests that “hypofrontality,” as measured with reduced regional cerebral blood flow (rCBF) in the PFC may indicate low dopamine levels in the PFC (36). Findings from preclinical lesion studies proposed that prefrontal “hypodopaminergia” lead to striatal “hyperdopaminergia” (38, 39). In addition, it is hypothesized that prefrontal “hypodopaminergia” could cause negative symptoms, whereas striatal “hyperdopaminergia” could lead to positive symptoms (36).
The dopamine hypothesis: version III synthesizes published findings on dopamine and its potential role in schizophrenia from the main fields into one unifying hypothesis. The hypothesis aims to provide a framework for findings from developments in clinical research into genetic (risk) factors, environmental risk factors, neurochemical and neuroimaging studies, and preclinical studies, which may be related to increased presynaptic striatal dopaminergic function in schizophrenia (23). The hypothesis is comprised of four components: (i) The interaction of “hits” such as fronto-temporal dysfunction, genes, stress, and drugs may lead to striatal dopamine dysregulation (i.e., increased presynaptic dopamine synthesis capacity) and therefore to psychosis. (ii) It is hypothesized that the primary dopaminergic dysfunction is located at the presynaptic dopaminergic level instead of the D2 receptor level. (iii) The hypothesis assumes that the dopamine dysregulation combined with cultural and societal factors could lead to future clinical diagnosis of “psychosis” rather than schizophrenia. (iv) It is proposed that the dopamine dysfunction could change the perception and judgment of stimuli (possibly through aberrant salience), which could result in cognitive deficits (40, 41).
Recent meta-analyses, which examined markers of striatal dopamine alterations in schizophrenia, reported evidence of different types of elevated dopamine dysfunction. Supporting evidence for the dopamine hypothesis has been shown by increased striatal presynaptic dopaminergic function in medication-free or medication-naïve patients with schizophrenia contrasted to healthy controls (42) and increased striatal dopamine synthesis capacity (43). Contradictory findings have however been reported by Fusar-Poli and Meyer-Lindenberg (44), who found no difference in striatal dopamine active transporter density between patients with schizophrenia and healthy controls.
In summary, while both the dopamine receptor hypothesis and the modified dopamine hypothesis of schizophrenia have their origins in the neurobiological investigation of the mode of action of antipsychotics, the dopamine hypothesis: version III aims at integrating advances in research of schizophrenia into one unifying dopamine hypothesis. The scope of understanding of dopaminergic dysregulation has become more defined, ranging from the whole brain perspective, through the perspective of regional specificity between (DL)PFC and striatum, to the current perspective of elevated presynaptic striatal dopaminergic function. The development of the dopamine hypothesis over the three versions has helped shape the understanding of schizophrenia as a brain disorder.
The origin of the glutamate hypothesis of schizophrenia was based on the discovery of psychotomimetic effects of ketamine and phencyclidine, which elicited psychotic symptoms in healthy people. Symptoms such as delusions and hallucinations experienced by healthy individuals were compared to positive symptoms seen in FES (45, 46). The glutamate hypothesis postulates a mechanistic process of altered interacting glutamatergic and/or dopaminergic neurotransmitter circuitries implicated in the pathophysiology of clinical and cognitive symptoms in schizophrenia (47–50). In this review, we consider three models of the glutamate hypothesis with relevance to the investigation of altered working memory function in people with schizophrenia: (i) the “N-Methyl-d-aspartate acid (NMDA) receptor hypofunction model” of schizophrenia, (ii) the “acute ketamine model,” and (iii) the “dysconnection hypothesis” of schizophrenia.
The NMDA receptor hypofunction model of schizophrenia posits that the subtype of the glutamate receptor is implicated in multiple pathological brain mechanisms of schizophrenia ranging across cellular, chemical, and neuronal levels (51–54). It has been proposed that NMDA receptor hypofunction could underlie the pathophysiology of negative and cognitive symptoms in schizophrenia (29, 51, 55, 56). Clinical trials with agents modulating NMDA receptor in addition to treatment with first-generation antipsychotics (FGA; such as chlorpromazine, haloperidol, perphenazine) and second-generation antipsychotics (SGA; such as clozapine and olanzapine) presented supporting evidence for amelioration of negative and cognitive symptoms (51, 57, 58). Evidence for the involvement of NMDA receptor hypofunction through interactions among different neurotransmitters such as γ-aminobutyric acid (GABAergic) interneurons (51) and dopamine (59, 60) has also been reported.
Evidence for the glutamate hypothesis in humans is based on clinical studies with ketamine in healthy subjects. Results suggest that glutamatergic alterations could explain the pathophysiological mechanisms resulting in positive symptoms predominantly experienced by FES and those with first episode psychosis (FEP) (45, 61). While findings from ketamine injection studies have aided the understanding of glutamatergic signaling in the development of delusions and hallucinations, evidence for altered glutamatergic transmission in negative and cognitive symptoms is scarce. FMRI findings from ketamine studies in healthy subjects propose that altered glutamatergic signaling could be implicated in working memory (12, 45, 62). These findings are in keeping with evidence from glutamatergic animal models, which report aberrant working memory function after the inhibition of glutamatergic receptors (63–66).
The dysconnection hypothesis of schizophrenia posits that altered NMDA receptor-mediated synaptic plasticity may be the underlying pathophysiological mechanism in individuals with schizophrenia (20, 21, 67). The authors propose that altered synaptic plasticity may explain both clinical symptoms and cognitive deficits in people with schizophrenia neurobiologically by altered NMDA receptor neuromodulation. Therefore, the dysconnection hypothesis synthesizes neurobiological findings (i.e., dopamine as one of the main neuromodulators leading to aberrant NMDA receptor function) with clinical and cognitive neuroscientific findings (i.e., cognitive impairment) in individuals with schizophrenia. One of the main objectives of the dysconnection hypothesis is to offer a new approach and therefore new interpretation of neurophysiological and neuroimaging data. This may be used to assist in the understanding of altered cognitive function in people with schizophrenia. For functional neuroimaging data, the biophysical modeling approach of dynamic causal modeling [DCM; Ref. (18)] has been proposed to infer biophysical processes (namely, NMDA receptor-dependent synaptic plasticity) underlying the blood-oxygen-level-dependent (BOLD) responses. In addition, the authors provide arguments that the development of positive symptoms such as delusions can be explained by a “failure of self-monitoring mechanism” or “corollary discharge” (20). Abnormal EC findings from EEG and fMRI studies across a range of cognitive tasks in subjects with schizophrenia in contrast to healthy controls have been reported (68–70). These lead to a new insight into altered connectivity above those provided by FC studies, which are formulated under different theoretical frameworks, specifically DCM findings enable the inference of biophysical processes underlying neural responses (18, 19, 71).
In summary, the three hypotheses, the NMDA receptor hypofunction model, the acute ketamine model, and the dysconnection hypothesis, have motivated researchers to investigate biophysical circuit processes implicated in glutamatergic and dopaminergic interaction in negative symptoms and cognitive function in schizophrenia. These circuit mechanisms are thought to underlie altered working memory function in schizophrenia. Research on the NMDA receptor hypofunction model has its roots in the pharmacological examination of antipsychotics, the development of new agents, and its effects on clinical and cognitive symptoms in preclinical and clinical research in schizophrenia. The focus of researchers examining the acute ketamine model and the dysconnection hypothesis lies on elucidating proposed neurobiological processes of blockade of NMDA receptor underlying altered cognitive brain function in schizophrenia. The study designs of both versions differ in the investigation of (i) the pharmacological effect of ketamine on altered cognitive brain function and clinical symptomatology in healthy controls (the acute ketamine model) and (ii) altered synaptic plasticity during altered cognitive brain function in subjects with schizophrenia. Despite the different approaches, researchers of both versions of the glutamate hypothesis share the common aim of increasing our insight into schizophrenia by the translation of neurobiological knowledge from basic research to clinical research in schizophrenia. Furthermore, researchers share the common methodological approach of large-scale network analysis of fMRI data. Taken together, development over the three versions of the glutamate hypothesis of schizophrenia have presented promising evidence for shaping the understanding of schizophrenia as a cognitive brain network disorder.
Clinical and cognitive neuroscience studies have applied in vivo neuroimaging techniques of fMRI, PET, and single-photon emission computed tomography (SPECT) to assess neurobiological processes that underlie working memory function in people with schizophrenia. Techniques such as PET and SPECT use injections of positron-emitting radionuclide as tracer (for PET) or gamma-emitting radionuclide as tracer (for SPECT) in the living brain. Although these nuclear medical imaging techniques are non-invasive they require the administration of tracers. FMRI provides non-invasive in vivo imaging, which measures brain function by means of the BOLD response (72).
In the last two decades, the fields of clinical and cognitive neurosciences merged to provide a multidisciplinary approach to research into schizophrenia. This approach has created the notion of schizophrenia as a cognitive brain disorder (15, 73, 74).
Working memory tasks were initially investigated with fMRI in healthy subjects (75–78). These initial findings led to the use of fMRI as a tool for examining neurobiological markers that could be related to working memory performance. The examination of working memory function was extended to individuals with schizophrenia.
Reported findings of brain function during working memory (among several domains and components of working memory tasks) in healthy controls have led to the understanding that dopamine modulates working memory in healthy controls (79–81). This evidence of dopaminergic involvement in working memory was extended by the findings of altered dopaminergic modulation in schizophrenia (74, 82). Subsequently, converging findings were reported that regions such as DLPFC, anterior cingulate cortex (ACC), and parietal cortex (PC) are activated in working memory in both healthy controls and in subjects with schizophrenia (83–86). However, in those with schizophrenia, these regions exhibit increased or reduced functional activations and FC between prefrontal and parietal regions as well as between prefrontal and temporal regions in contrast to healthy controls. Alterations in FC occur at all stages of the illness (87, 88): (i) in HR subjects (89); (ii) in FES and FEP (90), and (iii) in subjects with EST (91).
Systematic reviews and meta-analyses of working memory fMRI studies in people with schizophrenia do not report consistent findings (92–95). Some studies report increased activation of the DLPFC, commonly referred to as “hyperfrontality,” however, others report decreased activation or “hypofrontality.” This picture of differing functional activation in terms of the direction, extent, and/or pattern of BOLD responses was attributed to the variation of domains and components of working memory tasks (92–95). Also it was considered that methodological factors in the applied analyses would contribute to these variations in functional activation (93, 95, 96). In addition, differences in medication could contribute to variation in the reported functional activation between studies.
Here, we review exemplary fMRI studies using the numeric or verbal “N-back” task in subjects with EST and healthy controls, which reported functional activation and FC findings (Table 1). The reviewed studies present group differences between subjects with schizophrenia and healthy controls. In functional activation studies, evidence was reported for increased activation in DLPFC, PFC, ventral PFC, medial frontal gyrus, and AC during high working memory load in subjects with EST (89, 97–101). However, reduced activation in prefrontal regions, such as ventral PFC, DLPFC, AC, and parietal regions was found during high working memory load in subjects with EST (97, 98, 102). One study in FES found a reduction of activation in inferior frontal gyrus (IFG), superior frontal gyrus, and AC during high working memory load (103). We note three factors, which contributed to difficulties in comparing the findings across the reviewed studies: (i) missing information of phase of schizophrenia (100), (ii) heterogeneous groups of subjects with EST (97, 101, 103), and (iii) limited information on antipsychotic treatment (89, 97–101, 103). Fundamentally, none of the functional activation findings was interpreted in context of the dopamine or glutamate hypothesis. The lack of a clear understanding in terms of neural activation and pathophysiological mechanism suggests there is a need for studies examining wider prefrontal circuitry underlying working memory deficits in schizophrenia (93, 95).
Table 1. Understanding of schizophrenia as a cognitive brain disorder – I summary of main findings in verbal/numeric working memory.
Functional connectivity studies applied voxel-based seed approaches to the BOLD response (89, 100, 103), with the exception of one study, which applied an ROI-to-ROI approach (101). Despite equivalent methodological approaches, the FC findings are not entirely comparable due to the use of different seed locations. Findings of reduced connectivity involving subregions of the PFC were found in FES and EST. Reduced FC findings in subjects with schizophrenia and EST were reported in the majority of studies: (i) Reduced prefrontal–parietal4 FC in subjects with schizophrenia (100); (ii) Reduced prefrontal–hippocampal, prefrontal–striatal, and within-PFC FC in EST (89); and (iii) Reduced parieto-prefrontal FC and between putamen and ventrolateral PFC in EST (101). Further evidence for reduced FC between medial frontal gyrus and putamen was found in FES (103). In contrast to most studies that report reduced FC in the early and late phases of the illness, increased FC between the ventral PFC and posterior PC was shown in subjects with schizophrenia (100). The findings of both reduced and increased FC between subregions of the PFC and the posterior PC may be related to variations in behavioral response to task load for subjects with schizophrenia (100). Similar difficulties in comparing the FC findings among the studies are present as in the comparison of the functional activation studies due to unclear and missing information regarding the illness phase, diagnosis, and medication treatment. Similarly, no reference is made to the dopamine or glutamate hypothesis in interpreting the FC findings.
In summary, findings presented by FC studies during the “N-back” task have paved the way for the understanding of large-scale functional networks in working memory. Furthermore, the insight of brain alterations in subjects with schizophrenia has advanced with FC from individually activated regions to connectivity between brain regions. The perspective of circuit-based neurobiology and cognitive brain function opens the doors for translational research from preclinical and clinical research in schizophrenia. However, FC is limited as the connection assessments are based upon regional correlations and this approach does not allow inferences of directions or causality between connected regions (18).
Positron emission tomography and SPECT imaging in schizophrenia research are used to investigate indirect markers of dopamine measures such as D2/3 receptors, presynaptic dopaminergic function, dopamine synthesis capacity, dopamine release, and dopamine transporters. Three [H215O] PET studies consistently reported reduced rCBF in DLPFC and PC in verbal/numeric “2-back” in subjects with EST in contrast to healthy controls (104–106). Reduced prefrontal–hippocampal FC findings in subjects with schizophrenia in contrast to healthy controls (105, 106) confirmed the hypothesis of reduced functional connections in working memory. Correlational PET studies provided support for dopaminergic alterations and measures of the “2-back” task in subjects with schizophrenia (2, 16).
In summary, fMRI and PET studies in the field of clinical and cognitive neurosciences have been used to investigate brain function during working memory in people with schizophrenia (Figure 1). Both fMRI and PET findings have advanced the understanding of altered working memory performance and brain function in subjects with schizophrenia. This has led to better insight into the interaction between altered working memory function and experimental/clinical factors (such as cognitive domains of working memory function, performance level, phases of illness, clinical symptomatology, and effects of antipsychotic medication) in individuals with schizophrenia.
Figure 1. Understanding of schizophrenia as a cognitive brain disorder – verbal/numeric “N-back” task. afMRI; bPET, positron emission tomography.
The role of the DLPFC in working memory deficits has been associated with glutamatergic alterations and more specifically in dopamine–glutamate interactions (10, 50, 51). Furthermore, it has been reported that ketamine, a NMDA receptor antagonist, can induce psychosis-like symptoms in healthy subjects (45). Here, we briefly summarize the main functional activation and FC findings of fMRI studies on the spatial “N-back” task in the context of the glutamate hypothesis of schizophrenia (Table 2).
Table 2. Schizophrenia as a cognitive brain disorder II – summary of main findings in spatial working memory.
Anticevic et al. (12) presented ketamine-induced reduced functional activation in task-activated regions (such as the DLPFC and the precuneus) and task-deactivated regions of the default-mode network (DMN). In addition, the combination of a spiking local-circuit model of performance during the spatial “N-back” task and the functional activation findings revealed that the modulation of ketamine alters the association between the task-activated and the task-deactivated networks. Finally, it was shown that ketamine modulates FC between the fronto-parietal and DMN networks. In a recent study, Driesen et al. (62) provided further support for ketamine-induced reduced prefrontal FC during the spatial “N-back” task. Two FC approaches with the same seed regions were employed, seed-based FC and global-based connectivity (GBC), which revealed both decreased FC within the DLPFC. The seed-based analysis resulted in reduced FC between DLPFC and middle frontal gyrus [MFG, IFG, and insula (among other regions) under ketamine in contrast to saline]. The GBC analysis showed decreased FC of the DLPFC under ketamine.
In summary, these studies on altered spatial working memory function inform on the glutamate hypothesis, through the acute ketamine model (Figure 2). In this, they have advanced the understanding of NMDA receptor-modulated brain function in healthy subjects.
Clinical and cognitive neurosciences have advanced the understanding of altered working memory function in subjects with schizophrenia. FMRI studies in working memory among other neuroimaging and electrophysiological techniques, have reported on functional activation and FC findings in subjects with schizophrenia. Both findings of functional activation and FC revealed methodological, cognitive, and clinical factors related to our understanding of altered working memory function in patients with schizophrenia. In particular, FC findings mark the beginning of the notion of “disconnection” and “dysconnection” (20, 21, 67, 107) in working with people with schizophrenia. FC is defined as the statistical association or dependency among two or more anatomically distinct time-series (107). FC findings cannot be interpreted in terms of causal effects between connected regions and thus, does not allow for a mechanistic inference of the BOLD responses.
The modeling of functional large-scale networks5 during working memory function in schizophrenia could provide mechanistic explanations for altered brain function in individuals with schizophrenia. The advantage of modeling functional large-scale networks in terms of EC over FC is that inferences can be drawn on mechanistic processes, which are not directly observable in the BOLD response.
Marr proposes a theoretical framework for computational research on the brain on three levels (1976). At the first level, researchers should aim to gain knowledge of the high-level computations of the brain such as working memory (“computational level”). At the next level, the testing of the brain’s methods and algorithms for the high-level working memory function is led by hypotheses derived from the acquired knowledge and testing how appropriate an algorithm such as Bayesian inference is for modeling the working memory brain function (“algorithmic level”). Finally, when an algorithm is found, which is valid and more likely than alternative algorithms to predict known brain function/behavior, then the investigation of the biological implementation can be pursued (“physical level”).
Computational neuropsychiatry is an emerging field within computational neuroscience. Computational neuropsychiatry aims to provide an explanatory bridge between altered cognitive function and neurobiological mechanisms associated with the development of mental illness (108, 109, Huys, unreferred preprint). Computational neuropsychiatry in humans has been defined by outlining a set of components, which include biophysical modeling and computational modeling (109). Different types of computational models at different neural levels are used dependent on the study hypothesis (108, Huys, unreferred preprint).
Connectionist and neural network models in working memory/cognitive control in subjects with schizophrenia have added to our understanding of both the brain function and the neurobiological mechanism underlying working memory (74, 76). The strength of these models is based on the translational link between human brain function (i.e., functional activation) and preclinical neurobiological evidence (namely, dopaminergic modulation) during working memory.
Following on from the work of Cohen and Braver, evidence for the understanding of schizophrenia as a cognitive network disorder has been presented by both preclinical studies (8, 10, 110–113) and human FC studies in working memory (89, 100, 101, 103). Recent studies examining biophysical mechanisms underlying altered functional large-scale networks aim to bridge the gap between the human functional network used in working memory and the preclinical neurobiological processes. Examples of such computational neuropsychiatric studies, including EC during working memory in subjects with schizophrenia, are reviewed. In this, we focus on DCM studies investigating the numeric/verbal “N-back” task in subjects with schizophrenia and healthy controls. This is considered in the context of the dopamine and glutamate hypotheses of schizophrenia. Both neurobiological hypotheses have contributed to the formulation of research objectives in computational neuropsychiatry (114) and the development of computational modeling techniques of fMRI data in subjects with schizophrenia (20).
Dynamic causal modeling for fMRI has been introduced as a method to provide insight into the notion of “functional integration” during cognitive performance. “Functional integration” has been advanced from the historic notion of “functional specialization” (115), which is defined by context-dependent interactions among different brain regions (18, 116–118).
Dynamic causal modeling has been described as a biophysical modeling of neuronal dynamic processes (18, 19)6 , which can be used as a method for the computation of synaptic plasticity from fMRI task-based studies (20, 21). Together biophysical modeling and Bayesian inference analysis form the framework for DCM (71, 117, 118). Thus, DCM is a modeling approach, which combines defined network models (i.e., hypotheses) with Bayesian inversion methods (19, 117). Specifically, DCM assesses inter-regional EC through assessment of experimentally induced changes (18) and therefore allows for mechanistic inferences from neuronal function.
Bilinear DCM infers dynamics at the neuronal level by translating modeled neuronal responses into predicted BOLD measurements (18). Non-linear DCM for fMRI (71, 119) is an advanced approach for increasing the biological plausibility of DCMs by the means of modeling “gain modulation” (i.e., non-linear modulation of neuronal connections) (19, 117, 118). In non-linear DCM, the modulation of connection strengths by experimental inputs is supplemented by direct modulation of neural activity in one or more network regions (18, 119). The computations for gating in neural networks use the multiplicative computation of non-linear modulation (120, 121). Accordingly, non-linear DCM can be used for inferring that the strength of a connection is modulated by activity of other neuronal populations (119, 122).
Findings of altered effective connectivity during working memory in subjects with schizophrenia. The first DCM studies in healthy controls described large-scale networks in working memory and a similar task [continuous performance test; (123–125)]. A recent study in healthy controls built the linkage between EC results and underlying dopaminergic modulation of large-scale networks comprising of the DLPFC and PC during verbal memory performance (126).
To date four DCM studies have examined the verbal/numeric “N-back” task in subjects with schizophrenia using bilinear DCM (127–130) (Table 3). These provide novel insights into reduced task-dependent EC and increased task-independent EC measures through modeling large-scale networks in schizophrenia.
Table 3. Schizophrenia as a cognitive brain network disorder II – summary of main findings in verbal/numeric working memory – neuroimaging and biophysical modeling.
In the first study, increased fronto-temporal intrinsic connectivity was found to be associated with increased functional activation of the superior temporal gyrus (STG) during the numeric “N-back” task in the subjects at the prodromal and at the acutely psychotic stage of schizophrenia in contrast to the healthy controls. This suggests a potential marker for vulnerability to the disorder (127). Furthermore, progressively decreased intrinsic connectivity between the STG and the MFG in subjects at-risk mental state (ARMS) and FES subjects in contrast to the healthy controls was reported. This finding suggested that functional activation may resemble increased task-independent EC between the PFC and the STG. However, the results of the study are not comparable to other DCM studies because (i) only one model was examined and (ii) the biological plausibility of the EC measures is not clearly accessible. No reference to the dopamine or glutamate hypotheses was made.
The second study investigated the working memory-dependent modulatory effect for the prefrontal–parietal connectivity in subjects with EST and healthy subjects during the numeric “N-back” task (128). The large-scale networks included the right DLPFC, the PC, and the visual cortex with bidirectional connection between all regions. The main finding was decreased task-dependent EC from the DLPFC to the PC in the subjects with EST. Thus, this finding could resemble evidence for the glutamate hypothesis of schizophrenia, specifically the NMDA receptor hypofunction model and the dysconnection hypothesis.
The third study examined possible vulnerability markers for psychosis from the verbal “N-back” task in ARMS subjects, FES subjects, and healthy subjects (129). This study examined reduced task-dependent EC measures as well as relationships between connectivity parameters and antipsychotic medication received by subjects. In this study, EC in interhemispheric large-scale networks between the bilateral superior parietal lobes (SPL) and the bilateral MFG was assessed. This study reported novel findings of progressively decreased working memory and induced modulation of connectivity between the MFG and the SPL (from healthy subjects to ARMS). Additionally, further decreased EC of modulatory effects were observed in non-medicated subjects with FEP contrasted to healthy controls. Evidence for amelioration of reduced EC between the MFG and the SPL in subjects with FES, who received SGA medication, could reflect alterations of dopaminergic regulation of NMDA receptor-dependent synaptic plasticity of fronto-parietal connections. However, this interpretation is limited by the lack of a control group of FES who are treated with different types of antipsychotic medication. These findings across different subpopulations of schizophrenia together with the effect of antipsychotic medication may reflect support for the NMDA receptor hypofunction model and the dysconnection hypothesis.
In the fourth study, Zhang et al. (130) explored EC measures in terms of possible neurobiological markers in groups of subjects with schizophrenia with high or low suicide risk and contrasted these with healthy controls during the verbal “N-back” task. The large-scale networks were defined by unidirectional and bidirectional connections between the two regions of the medial PFC and PC as well as working memory effects on these regions. This pilot study presented novel findings in subjects with schizophrenia at suicidal risk in terms of increased intrinsic connectivity from the PC to the MFG in both groups with FES (in comparison to healthy controls). This finding was interpreted as a possible association to schizophrenia, in which increased intrinsic connectivity from the MFG to the PC in the subjects with high risk of suicide could reflect vulnerability of suicide. However, the results are not directly comparable to the other DCM studies because of the study population, which focused on the issue of suicide. The findings were also not interpreted in context of the dopamine or glutamate hypotheses.
We highlight main experimental and methodological limitations in the four DCM studies, which impede the comparability of findings (please see Table 3 for details). The main experimental limitation focuses on the discrepancies between the different patient subpopulations. Two studies analyzed working memory fMRI data of subjects with ARMS and FES in comparison to healthy controls (127, 129), whereas one study modeled scans from subjects with EST (128). Zhang et al. (130) reported findings of a unique patient population of FES with high and low suicidal risk. In terms of methodological issues, one limitation lies in different definitions of model spaces for the large-scale networks, despite equivalence in the experimental tasks. Another limitation is that the reviewed DCM studies employed deterministic DCM for the comparison of the models. Deterministic models can predict processes perfectly if all inputs are known (131). However, at this early stage of employing biophysical modeling approaches to human brain function, we do not have a full understanding of the brain responses to working memory. Future studies may employ stochastic DCM as an extension (117, 118, 132). A further limitation is that different DCM versions were applied across the four studies, which impede the comparability of the findings. The priors are differently defined in the used DCM versions, which give rise to a variation in model evidence between the studies (117). Thus, it is possible that discrepancies in EC findings could be due to the prior definition and may not be solely due to differences in performance, brain function, or clinical aspects of subjects with schizophrenia. Lastly, a general limitation of DCM for fMRI is that maximally 10 regions within a large-scale network can be modeled. This simplification results in difficulties of biophysical modeling of tasks, which are likely to encompass more than ten regions. Furthermore, not only the definition of different regions and different numbers of regions but also different modulatory inputs result in further extensions to the model space. Such model spaces are difficult to validate and analyze.
The four DCM studies presented evidence for increased task-dependent EC and increased task-independent EC findings during verbal/numeric working memory in subjects with schizophrenia. We discuss these EC findings in context of (i) the dopamine and glutamate hypothesis and (ii) FC findings during verbal/numeric working memory in subjects with schizophrenia.
The four studies modeled large-scale networks during the “N-back” task in subjects with schizophrenia. However, only two out of these four studies consider their DCM results in the light of biophysical processes (128, 129). The findings of reduced EC (namely, the effect of task-modulation) of the prefrontal–parietal connection in subjects with schizophrenia in contrast to healthy controls were interpreted biophysically and linked to the NMDA receptor hypofunction model and the dysconnection hypothesis (128, 129). Both studies reported reduced EC findings of the prefrontal–parietal connection during working memory, however, these findings need to be considered carefully due to different experimental designs (i.e., patient subpopulations, antipsychotic medication treatment of FGA and SGA) and methodological implementation (i.e., model space, DCM settings, and inference techniques).
Three of the DCM studies reported altered EC findings of the prefrontal–parietal and parieto-prefrontal connections during the “N-back” task in subjects with schizophrenia in contrast to healthy controls. Deserno et al. (128) and Schmidt et al. (129) presented reduced EC (effect of task-modulation) of the prefrontal–parietal connection in subjects with schizophrenia in contrast to healthy controls, whereas Zhang et al. (130) found increased EC (intrinsic connectivity) of the parietal–prefrontal connection. The reduced task-dependent EC findings are in keeping with reduced FC findings of these connections, although increased FC between a different prefrontal subregion and the PC was reported (100).
The study by Crossley et al. (127) reported increased EC (intrinsic connectivity) of the prefrontal–temporal connection in subjects at HR and FES (in contrast to healthy controls). Reduced FC of the prefrontal–temporal connection during the “N-back” task in subjects with schizophrenia has been previously reported in PET studies (105, 106). However, the regions within the PFC and temporal region differ between the studies.
Findings of altered effective connectivity during verbal fluency in subjects with schizophrenia. Here, we discuss bilinear and non-linear DCM studies, which have assessed large-scale networks during verbal fluency [namely, the Hayling sentence completion task (HSCT)] in subjects with schizophrenia and healthy controls (Table 4). One bilinear DCM study in healthy controls investigated the task-dependent modulation of response initiation and response suppression in EC between left hemispheric temporal and prefrontal regions (133). The main finding was a difference in connection strength of the modulatory effect in response initiation and response suppression.
Table 4. Schizophrenia as a cognitive brain network disorder II – summary of main findings in verbal fluency – neuroimaging and biophysical modeling.
Two clinical bilinear DCM studies have investigated EC measures during the HSCT in HR subjects and healthy controls: (i) Subjects at high clinical risk of schizophrenia [ARMS; Ref. (134)] and (ii) subjects at high familial risk of schizophrenia (135). Allen et al. (134) investigated increased fronto-temporal EC (intrinsic connectivity) as a potential measure of vulnerability of developing schizophrenia. Two main findings were reported: firstly, no significant effect of task-dependent modulation on the fronto-temporal connection between ARMS subjects and healthy controls was revealed. Secondly, ARMS subjects displayed increased intrinsic connectivity between the ACC and the MTG in comparison to healthy controls. Furthermore, the Bayesian model selection (BMS) approach revealed that the same network was equally likely to explain the given HSCT fMRI data in both the ARMS subjects and the healthy controls. No reference to the glutamate hypothesis was made.
Dauvermann et al. (135) modeled EC measures in a similar version of the HSCT that was used by Allen et al. (134). This study was conducted in subjects at high familial risk of schizophrenia and healthy subjects. The results reported by Allen et al. (134) of a similar large-scale network in both HR subjects and healthy controls was replicated7. This finding was also confirmed by Dauvermann et al. (135), when the group of HR subjects was subdivided into high risk subjects without transient psychotic symptoms (referred to as HR−), high risk subjects with transient psychotic symptoms (referred to as HR+) and high risk subjects who subsequent to scanning developed schizophrenia [referred to as HRill; please see Ref. (136, 137)]. Comparability between these two studies is limited due to differences in the model space. The model space in Dauvermann et al. (135) includes the IPS and the mediodorsal thalamus, which are not incorporated in the model space by Allen et al. (134). In addition, endogenous connections and task-dependent modulations were accordingly changed [Ref. (135); Table 4]. There was no reference to the glutamate hypothesis of schizophrenia.
Limitations of bilinear DCM have been addressed through the development of non-linear DCM for fMRI (119). This method was applied in the genetic high risk study reported by Dauvermann et al. (135). The progress from the bilinear DCM to the non-linear DCM as reported by Dauvermann is based on the biophysical modeling of connection strength with non-linear modulation during the HSCT response. The authors show that relative to healthy controls there is reduced connection strength with non-linear modulation of the thalamo-cortical connection during the HSCT in HR+ subjects and a further reduction in this connection strength in HRill subjects (135). The authors suggest that reduced gain control may underlie the reduced strength in the thalamo-cortical connection. Furthermore, the findings of reduced connection strength with non-linear modulation of the thalamo-cortical connection could reflect altered glutamatergic neurotransmission, which may underlie a disruption of synaptic plasticity in this thalamo-cortical connection [Ref. (135); Table 4]. Thus, the findings were interpreted in context of the NMDA receptor hypofunction model and the dysconnection hypothesis.
Evidence from brain function in working memory in subjects with schizophrenia at the level of functional large-scale networks (i.e., clinical and cognitive neurosciences) and neurobiological mechanisms in working memory in animal models of schizophrenia (preclinical neurobiological research) in combination with computational neuroscientific approaches has informed and enabled research in computational neuropsychiatry.
Exemplary DCM studies in subjects with schizophrenia have reported both increased and reduced EC findings during cognition in subjects with schizophrenia in contrast to healthy controls. These studies applied DCM as a biophysical modeling approach to functional large-scale networks, which enabled the interpretation of EC findings on the basis of the glutamate hypothesis of schizophrenia, namely the NMDA receptor hypofunction model and the dysconnection hypothesis (128, 129, 135). We emphasize that the findings support not only the glutamate hypothesis but also the dopamine hypothesis. Dopamine is a neuromodulator that may crucially affect glutamate-induced synaptic plasticity. Synaptic plasticity may be involved in a regulation of dopamine synthesis and release via other neurotransmitter systems. Specifically for non-linear effects, it has been shown that dopamine acts as a neuromodulator mediating postsynaptic gain (74, 138).
In a recent study, it has been reported that the combination of the DCM analysis of numerical “N-back” task in EST (128) and generative embedding resulted in the dissection of three subgroups of EST based on the mechanistically inferred DCM findings (139). This exemplary study showed that DCM can be applied as a generative model of large-scale networks in individuals with schizophrenia. In summary, DCM is a promising approach for modeling synaptic plasticity; nevertheless in its current form it cannot reflect the full complexity in the processing required for the implementation of tasks such as working memory (Figure 3).
Figure 3. Understanding of schizophrenia as a cognitive brain network disorder – verbal/numeric “N-back” task.
Our understanding of schizophrenia is in continuous development and with more preclinical and clinical findings being published this understanding will advance further. A critical aspect of this understanding is the facilitation of multidisciplinary approach between preclinical and clinical research in schizophrenia.
The original understanding of schizophrenia as a brain disorder stems from observational clinical work, which led onto preclinical investigation. Over time, the knowledge of alterations of cellular, chemical, and molecular mechanisms has increased: (i) findings of dopaminergic and glutamatergic modulation of working memory (and clinical features) in animal models of schizophrenia contributed to form the understanding of schizophrenia as a cognitive brain disorder; (ii) findings of neurotransmitter circuit systems, mainly dopaminergic and glutamatergic systems, were found to modulate working memory in animal models of schizophrenia in combination with computational studies (140), which plays a role in shaping the understanding of schizophrenia as a cognitive network disorder.
Understanding of schizophrenia has not only been shaped by preclinical research but also by clinical research in subjects with schizophrenia, which has been and continues to be illuminated by preclinical neurobiological and computational work. The field of clinical and cognitive neurosciences has contributed to forming our understanding of schizophrenia as a cognitive brain disorder. Importantly, the multidisciplinary field of computational neuropsychiatry (preclinical neurobiology, clinical and cognitive neurosciences, and computational psychiatry) has allowed for progress in our understanding of schizophrenia as a cognitive brain network disorder.
The use of computational neuropsychiatric research in developing our understanding of schizophrenia as a cognitive brain network disorder is at an early stage. Here, we focused on FC and EC studies (DCM studies) during the verbal/numeric “N-back” task in subjects with schizophrenia and healthy controls. We discuss these FC and EC findings in context of two key research questions. Consideration of these questions was seen as a means to inform future schizophrenia research in the fields of clinical and cognitive neurosciences and/or computational neuropsychiatry:
Studies reported both increased and reduced FC during the “N-back” task in subjects with schizophrenia in contrast to healthy controls. These findings have introduced the notion of human large-scale networks underlying brain function during working memory. The FC correlational analyses do not allow for the inference of directions or weights of in functional connections. Thus, from FC findings it is not practical to draw inferences on neurobiological causal processing.
Studies, which applied DCM as a biophysical modeling approach to functional large-scale networks, showed that reduced EC findings could be interpreted in context of the NMDA receptor hypofunction model and the dysconnection hypothesis.
In summary, FC findings cannot be interpreted in context of the dopamine or glutamate hypothesis. For EC findings, the computational neuropsychiatric approach of modeling large-scale networks requires biophysically plausible networks, which are hypothesis-driven from neurobiological and cognitive neuroscience in subjects with schizophrenia. EC findings have been interpreted in the context of the glutamate hypothesis and the dopamine hypothesis.
Functional connectivity findings from cognitive and clinical neuroscience have contributed to the understanding of schizophrenia as a cognitive brain disorder. The analysis of altered working memory at the level of large-scale networks has advanced our knowledge of cognitive function in humans. However, it is not wholly understood what altered FC during cognition neurobiologically means in schizophrenia. EC findings from computational neuropsychiatry, here specifically modeling functional large-scale networks with DCM, have shown indications of linkage between clinical network-based working memory (large-scale networks) and preclinical neurotransmitter modulation of cognitive function. Altered synaptic plasticity during working memory can be interpreted with dopaminergic and glutamatergic mechanisms. We emphasize that the interpretation of altered neurotransmitter circuits should be considered carefully because the DCM method is likely to underestimate the processing complexity in neurobiological circuits. Nonetheless, a strength of DCM lies in interpretation of altered synaptic plasticity based on the inference of mechanistic information.
The consideration of schizophrenia as a cognitive brain network disorder from computational neuropsychiatry offers a holistic view of schizophrenia. Computational neuropsychiatry seeks to bridge the gap between neurobiology and cognitive and clinical neurosciences in subjects with schizophrenia. It is hoped that this research will enhance our understanding of schizophrenia, clinical treatment, and improve outcome in people with schizophrenia.
The reviewed findings in biophysical modeling of functional large-scale networks are promising. In order to reach the objective of predicting and improving clinical treatment in subjects with schizophrenia, longitudinal study designs, and the combination of subfields within computational neuropsychiatry should be pursued.
We consider computational neuropsychiatric research areas for the combination of biophysical modeling of functional large-scale networks and other computational (neuro)psychiatric approaches, which are of clinical relevance for subjects with schizophrenia, for example:
• Neurotransmitter systems
• Behavior
• Clinical symptoms
• Effects of antipsychotic medication
• Clinical outcome.
We suggest specific study designs, which may increase our understanding for developing clinical treatment for subjects with schizophrenia:
(i) Combination of biophysical modeling of functional large-scale networks with computation, for example:
(a) Brain function and brain circuit model (12);
(b) Brain function and behavior (141);
(c) Brain function and effect of antipsychotic medication:
(ii) Combination of biophysical modeling of functional large-scale networks with multimodal neuroimaging study designs, for example:
(a) FMRI and EEG/magnetoencephalography study designs;
(b) FMRI and transcranial magnetic stimulation study designs (142);
(c) FMRI and MRS study designs;
(d) FMRI and PET study designs;
(iii) Combination of biophysical modeling of functional large-scale networks and computational modeling for the investigation of clinical (sub)groups, for example:
(a) Associative learning (143, 144);
(b) Machine learning approach (139, 145);
(c) Reinforcement learning (109).
Findings of modeling functional large-scale networks contribute to shaping the understanding of schizophrenia as a cognitive brain network disorder. The combination of computational neuropsychiatric areas may bring researchers closer to the common long-term objectives of developing a diagnostic tool for schizophrenia along with the development of more effective treatments.
Maria R. Dauvermann, Neil Roberts, Stephen M. Lawrie, and Thomas W. J. Moorhead have received financial support from Pfizer (formerly Wyeth) in relation to imaging studies of people with schizophrenia. Stephen M. Lawrie has done consultancy work for Roche Pharmaceuticals in connection with a possible new treatment for schizophrenia. Stephen M. Lawrie has also received honoraria for lectures, chairing meetings, and consultancy work from Janssen in connection with brain imaging and therapeutic initiatives for psychosis.
We acknowledge Vincent Valton for his help. Maria R. Dauvermann and Thomas W. J. Moorhead are supported by Dr Mortimer and Theresa Sackler Foundation. Heather C. Whalley is supported by Royal Society Dorothy Hodgkin Fellowship.
AC/ACC, anterior cingulate/anterior cingulate cortex; ARMS, at-risk mental state; BMS, Bayesian model selection; BOLD response, blood-oxygen-level dependent response; D1 receptor, D1 subtype of the dopamine receptor; D2 receptor, D2 subtype of the dopamine receptor; D2/3 receptors, D2/3 subtype of the dopamine receptor; DCM, Dynamic Causal Modeling; DMN, default-mode network; DLPFC, dorsolateral prefrontal cortex; EC, effective connectivity; EST, subjects with EST; FC, functional connectivity; FEP, subjects with first episode psychosis; FES, subjects with first episode schizophrenia; GABA, γ-aminobutyric acid; GBC, global-based connectivity; HR, subjects at high risk of schizophrenia; HR+, subjects at high familial risk of schizophrenia with transient psychotic symptoms; HR−, subjects at high familial risk of schizophrenia without transient psychotic symptoms; HRill, subjects at high familial risk of schizophrenia who subsequent to scanning developed schizophrenia; HSCT, Hayling sentence completion task IFG inferior frontal gyrus; MFG, middle frontal gyrus; MRS, magnetic resonance spectroscopy; NMDA, N-Methyl-d-aspartate acid; PC, parietal cortex; PET, positron emission tomography; rCBF, regional cerebral blood flow; SPECT, single-photon emission computed tomography; SPL, superior parietal lobe; STG, superior temporal gyrus.
1. MacDonald AW, Schulz SC. What we know: findings that every theory of schizophrenia should explain. Schizophr Bull (2009) 35:493–508. doi: 10.1093/schbul/sbp017
2. Fusar-Poli P, Howes OD, Allen P, Broome M, Valli I, Asselin MC, et al. Abnormal frontostriatal interactions in people with prodromal signs of psychosis: a multimodal imaging study. Arch Gen Psychiatry (2010) 67(7):683–91. doi:10.1001/archgenpsychiatry.2010.77
3. Gold JM. Cognitive deficits as treatment targets in Schizophrenia. Schizophr Res (2004) 72(1):21–8. doi:10.1016/j.schres.2004.09.008
4. Seidman LJ, Giuliano AJ, Meyer EC, Addington J, Cadenhead KS, Cannon TD, et al. Neuropsychology of the prodrome to psychosis in the NAPLS consortium: relationship to family history and conversion to psychosis. Arch Gen Psychiatry (2010) 67:578–88. doi:10.1001/archgenpsychiatry.2010.66
5. Kim HS, Shin NY, Jang JH, Kim E, Shim G, Park HY, et al. Social cognition and neurocognition as predictors of conversion to psychosis in individuals at ultra-high risk. Schizophr Res (2011) 130:170–5. doi:10.1016/j.schres.2011.04.023
6. Genevsky A, Garrett CT, Alexander PP, Vinogradov S. Cognitive training in schizophrenia: a neuroscience-based approach. Dialogues Clin Neurosci (2010) 12(3):416–21.
7. Bora E, Murray RM. Meta-analysis of cognitive deficits in ultra-high risk to psychosis and first-episode psychosis: do the cognitive deficits progress over, or after the onset of psychosis? Schizophr Bull (2013). doi:10.1093/schbul/sbt085
8. Seshadri S, Zeledon M, Sawa A. Synapse-specific contributions in the cortical pathology of schizophrenia. Neurobiol Dis (2013) 53:26–35. doi:10.1016/j.nbd.2013.01.009
9. Kukla M, Davis LW, Lysaker PH. Cognitive behavioral therapy and work outcomes: correlates of treatment engagement and full and partial success in schizophrenia. Behav Cogn Psychother (2013). doi:10.1017/S1352465813000428
10. Tanaka S. Dopaminergic control of working memory and its relevance to schizophrenia: a circuit dynamics perspective. Neuroscience (2006) 139:153–71. doi:10.1016/j.neuroscience.2005.08.070
11. Tan HY, Callicott JH, Weinberger DR. Dysfunctional and compensatory prefrontal cortical systems, genes and the pathogenesis of schizophrenia. Cereb Cortex (2007) 17:i171–81. doi:10.1093/cercor/bhm069
12. Anticevic A, Gancsos M, Murray JD, Repovs G, Driesen NR, Ennis DJ, et al. NMDA receptor function in large-scale anticorrelated neural systems with implications for cognition and schizophrenia. Proc Natl Acad Sci U S A (2012) 109:16720–5. doi:10.1073/pnas.1208494109
13. Volk DW, Lewis DA. Prefrontal cortical circuits in schizophrenia. Curr Top Behav Neurosci (2010) 4:485–508. doi:10.1007/7854_2010_44
14. Kim JJ, Kwon JS, Park HJ, Youn DH, Kang MS, Kim MS, et al. Functional disconnection between the prefrontal and parietal cortices during working memory processing in schizophrenia: a [15(O)]H20 PET study. Am J Psychiatry (2003) 160(5):919–23. doi:10.1176/appi.ajp.160.5.919
15. Honey GD, Fletcher PC. Investigating principles of human brain function underlying working memory: what insights from schizophrenia? Neuroscience (2006) 139:59–71. doi:10.1016/j.neuroscience.2005.05.036
16. Abi-Dargham A, Mawlawi O, Lombardo I, Gil R, Martinez D, Huang Y, et al. Prefrontal dopamine D1 receptors and working memory in schizophrenia. J Neurosci (2002) 22(9):3708–19.
17. Poels EM, Kegeles LS, Kantrowitz JT, Javitt DC, Lieberman JA, Abi-Dargham A, et al. Glutamatergic abnormalities in schizophrenia: a review of proton MRS findings. Schizophr Res (2014) 152(2–3):325–32. doi:10.1016/j.schres.2013.12.013
18. Friston KJ, Harrison L, Penny W. Dynamic causal modelling. Neuroimage (2003) 19:1273–302. doi:10.1016/S1053-8119(03)00202-7
19. Friston KJ, Dolan RJ. Computational and dynamic models in neuroimaging. Neuroimage (2010) 52:752–65. doi:10.1016/j.neuroimage.2009.12.068
20. Stephan KE, Friston KJ, Frith CD. Dysconnection in schizophrenia: from abnormal synaptic plasticity to failures of self-monitoring. Schizophr Bull (2009) 35:509–27. doi:10.1093/schbul/sbn176
21. Stephan KE, Baldeweg T, Friston KJ. Synaptic plasticity and dysconnection in schizophrenia. Biol Psychiatry (2006) 59:929–39. doi:10.1016/j.biopsych.2005.10.005
22. Stephan KE, Mathys C. Computational approaches to psychiatry. Curr Opin Neurobiol (2014) 25:85–92. doi:10.1016/j.conb.2013.12.007
23. Howes OD, Kapur S. The dopamine hypothesis of schizophrenia: version III – the final common pathway. Schizophr Bull (2009) 35:549–62. doi:10.1093/schbul/sbp006
24. Qi Z, Miller GW, Voit EO. Computational modeling of synaptic neurotransmission as a tool for assessing dopamine hypotheses of schizophrenia. Pharmacopsychiatry (2010) 43(Suppl 1):S50–60. doi:10.1055/s-0030-1248317
25. Coyle JT. NMDA receptor and schizophrenia: a brief history. Schizophr Bull (2012) 38(5):920–6. doi:10.1093/schbul/sbs076
26. Coyle JT, Basu A, Benneyworth M, Balu D, Konopaske G. Glutamatergic synaptic dysregulation in schizophrenia: therapeutic implications. Handb Exp Pharmacol (2012) 2012:267–95. doi:10.1007/978-3-642-25758-2_10
27. Kantrowitz JT, Javitt DC. N-methyl-d-aspartate (NMDA) receptor dysfunction or dysregulation: the final common pathway on the road to schizophrenia? Brain Res Bull (2010) 83:108–21. doi:10.1016/j.brainresbull.2010.04.006
28. Delay J, Deniker P, Harl JM. Therapeutic use in psychiatry of phenothiazine of central elective action (4560 RP). Ann Med Psychol (Paris) (1952) 110(2):112–7.
30. Emilien G, Maloteaux JM, Geurts M, Owen MJ. Dopamine receptors and schizophrenia: contribution of molecular genetics and clinical neuropsychology. Int J Neuropsychpharmacol (1999) 2(3):197–227. doi:10.1017/S1461145799001479
31. Seeman P, Lee T. Antipsychotic drugs: direct correlation between clinical potency and presynaptic action on dopamine neurons. Science (1975) 188:1217–9. doi:10.1126/science.1145194
32. Creese I, Burt DR, Snyder SH. Dopamine receptor binding predicts clinical and pharmacological potencies of antischizophrenic drugs. Science (1976) 192:481–3. doi:10.1126/science.3854
33. Seeman P, Lee T, Chau-Wong M, Wong K. Antipsychotic drug doses and neuroleptic/dopamine receptors. Nature (1976) 261:77–9. doi:10.1038/261717a0
34. Lieberman AN, Goldstein M, Gopinathan G, Nophytides A. D-1 and D-2 agonists in Parkinson’s disease. Can J Neurol Sci (1987) 14(3 Suppl):466–73.
35. Snyder SH. The dopamine hypothesis of schizophrenia: focus on the dopamine receptor. Am J Psychiatry (1976) 133(2):197–202.
36. Davis KL, Kahn RS, Ko G, Davidson M. Dopamine in schizophrenia: a review and reconceptualization. Am J Psychiatry (1991) 148:1474–86.
37. Kapur S, Seeman P. Does fast dissociation from the dopamine D(2) receptor explain the action of atypical antipsychotics? A new hypothesis. Am J Psychiatry (2001) 158:360–9. doi:10.1176/appi.ajp.158.3.360
38. Pycock CJ, Kerwin RW, Carter CJ. Effect of lesion of cortical dopamine terminals on subcortical dopamine receptors in rats. Nature (1980) 286:74–6. doi:10.1038/286074a0
39. Scatton B, Worms P, Lloyd KG, Bartholini G. Cortical modulation of striatal function. Brain Res (1982) 232(2):331–43. doi:10.1016/0006-8993(82)90277-3
40. Heinz A. Dopaminergic dysfunction in alcoholism and schizophrenia – psychopathological and behavioral correlates. Eur Psychiatry (2002) 17(1):9–16. doi:10.1016/S0924-9338(02)00628-4
41. Kapur S. Psychosis as a state of aberrant salience: a framework linking biology, phenomenology, and pharmacology in schizophrenia. Am J Psychiatry (2003) 160(1):13–23. doi:10.1176/appi.ajp.160.1.13
42. Howes OD, Kambeitz J, Kim E, Stahl D, Slifstein M, Abi-Dargham A, et al. The nature of dopamine dysfunction in schizophrenia and what this means for treatment. Arch Gen Psychiatry (2012) 69(8):776–86. doi:10.1001/archgenpsychiatry.2012.169
43. Fusar-Poli P, Meyer-Lindenberg A. Striatal presynaptic dopamine in schizophrenia, part I: meta-analysis of dopamine active transporter (DAT) density. Schizophr Bull (2013) 39(1):22–32. doi:10.1093/schbul/sbr111
44. Fusar-Poli P, Meyer-Lindenberg A. Striatal presynaptic dopamine in schizophrenia, part II: meta-analysis of [(18)F/(11)C]-DOPA PET studies. Schizophr Bull (2013) 39(1):33–42. doi:10.1093/schbul/sbr180
45. Krystal JH, Karper LP, Seibyl JP, Freeman GK, Delaney R, Bremner JD, et al. Subanesthetic effects of the noncompetitive NMDA antagonist, ketamine, in humans. Psychotomimetic, perceptual, cognitive, and neuroendocrine responses. Arch Gen Psychiatry (1994) 51(3):199–214. doi:10.1001/archpsyc.1994.03950030035004
46. Abi-Saab WM, D’Souza DC, Moghaddam B, Krystal JH. The NMDA antagonist model for schizophrenia: promise and pitfalls. Pharmacopsychiatry (1998) 31(Suppl 2):104–9. doi:10.1055/s-2007-979354
47. Luby E, Cohen B, Rosenbaum G, Gottlieb J, Kelley J. Study of a new schizophrenomimetic drugs. AMA Arch Neurol Psychiatry (1959) 81:363–9. doi:10.1001/archneurpsyc.1959.02340150095011
48. Carlsson A, Waters N, Waters S, Carlsson ML. Network interactions in schizophrenia – therapeutic implications. Brain Res Brain Res Rev (2000) 31(2–3):342–9. doi:10.1016/S0165-0173(99)00050-8
49. Farber NB, Kim SH, Dikranian K, Jiang XP, Heinkel C. Receptor mechanisms and circuitry underlying NMDA antagonist neurotoxicity. Mol Psychiatry (2002) 7:32–43. doi:10.1038/sj.mp.4000912
50. Javitt DC. Glutamate and schizophrenia: phencyclidine, N-methyl-d-aspartate receptors, and dopamine-glutamate interactions. Int Rev Neurobiol (2007) 78:69–108. doi:10.1016/S0074-7742(06)78003-5
51. Coyle JT. Glutamate and schizophrenia: beyond the dopamine hypothesis. Cell Mol Neurobiol (2006) 26:365–84. doi:10.1007/s10571-006-9062-8
52. Coyle JT, Balu D, Benneyworth M, Basu A, Roseman A. Beyond the dopamine receptor: novel therapeutic targets for treating schizophrenia. Dialogues Clin Neurosci (2010) 12(3):359–82.
53. Moghaddam B, Krystal JH. Capturing the angel in “angel dust”: twenty years of translational neuroscience studies of NMDA receptor antagonists in animals and humans. Schizophr Bull (2012) 38:942–9. doi:10.1093/schbul/sbs075
54. Javitt DC, Zukin SR, Heresco-Levy U, Umbricht D. Has an angel shown the way? Etiological and therapeutic implications of the PCP/NMDA model of schizophrenia. Schizophr Bull (2012) 38:958–66. doi:10.1093/schbul/sbs069
55. Javitt DC. Glutamatergic theories of schizophrenia. Isr J Psychiatry Relat Sci (2010) 47(1):4–16.
56. Goff DC, Coyle JT. The emerging role of glutamate in the pathophysiology and treatment of schizophrenia. Am J Psychiatry (2001) 158:1367–77. doi:10.1176/appi.ajp.158.9.1367
57. Heresco-Levy U, Ermilov M, Shimoni J, Shapira B, Silipo G, Javitt DC. Placebo-controlled trial of D-cycloserine added to conventional neuroleptics, olanzapine, or risperidone in schizophrenia. Am J Psychiatry (2002) 159(3):480–2. doi:10.1176/appi.ajp.159.3.480
58. Lane HY, Chang YC, Liu YC, Chiu CC, Tsai GE. Sarcosine or D-serine add-on treatment for acute exacerbation of schizophrenia: a randomized, double-blind, placebo-controlled study. Arch Gen Psychiatry (2005) 62(11):1196–204. doi:10.1001/archpsyc.62.11.1196
59. Tzschentke TM. Pharmacology and behavioral pharmacology of the mesocortical dopamine system. Prog Neurobiol (2001) 63(3):241–320. doi:10.1016/S0301-0082(00)00033-2
60. Sesack SR, Carr DB. Selective prefrontal cortex inputs to dopamine cells: implications for schizophrenia. Physiol Behav (2002) 77(4–5):513–7. doi:10.1016/S0031-9384(02)00931-9
61. Krystal JH, D’Souza DC, Karper LP, Bennett A, Abi-Dargham A, Abi-Saab D, et al. Interactive effects of subanesthetic ketamine and haloperidol in healthy humans. Psychopharmacology (1999) 145(2):193–204. doi:10.1007/s002130051049
62. Driesen NR, McCarthy G, Bhagwagar Z, Bloch MH, Calhoun VD, D’Souza DC, et al. The impact of NMDA receptor blockade on human working memory-related prefrontal function and connectivity. Neuropsychopharmacology (2013) 38(13):2613–22. doi:10.1038/npp.2013.170
63. Moghaddam B, Adams B, Verma A, Daly D. Activation of glutamatergic neurotransmission by ketamine: a novel step in the pathway from NMDA receptor blockade to dopaminergic and cognitive disruptions associated with the prefrontal cortex. J Neurosci (1997) 17:2921–7.
64. Timofeeva OA, Levin ED. Glutamate and nicotinic receptor interactions in working memory: importance for the cognitive impairment of schizophrenia. Neuroscience (2011) 195:21–36. doi:10.1016/j.neuroscience.2011.08.038
65. Fitzgerald PJ. The NMDA receptor may participate in widespread suppression of circuit level neural activity, in addition to a similarly prominent role in circuit level activation. Behav Brain Res (2012) 230:291–8. doi:10.1016/j.bbr.2012.01.057
66. Arnsten AF, Wang MJ, Paspalas CD. Neuromodulation of thought: flexibilities and vulnerabilities in prefrontal cortical network synapses. Neuron (2012) 76:223–39. doi:10.1016/j.neuron.2012.08.038
67. Stephan KE, Penny WD, Daunizeau J, Moran RJ, Friston KJ. Bayesian model selection for group studies. Neuroimage (2009) 46:1004–17. doi:10.1016/j.neuroimage.2009.03.025
68. Dima D, Roiser JP, Dietrich DE, Bonnemann C, Lanfermann H, Emrich HM, et al. Understanding why patients with schizophrenia do not perceive the hollow-mask illusion using dynamic causal modelling. Neuroimage (2009) 46(4):1180–6. doi:10.1016/j.neuroimage.2009.03.033
69. Dima D, Dietrich DE, Dillo W, Emrich HM. Impaired top-down processes in schizophrenia: a DCM study of ERPs. Neuroimage (2010) 52(3):824–32. doi:10.1016/j.neuroimage.2009.12.086
70. Wagner G, Koch K, Schachtzabel C, Schultz CC, Gaser C, Reichenbach JR, et al. Structural basis of the fronto-thalamic dysconnectivity in schizophrenia: a combined DCM-VBM study. Neuroimage Clin (2013) 3:95–105. doi:10.1016/j.nicl.2013.07.010
71. Stephan KE, Friston KJ. Analyzing effective connectivity with fMRI. Wiley Interdiscip Rev Cogn Sci (2010) 1:446–59. doi:10.1002/wcs.58
72. Ogawa S, Lee TM, Kay AR, Tank DW. Brain magnetic resonance imaging with contrast dependent on blood oxygenation. Proc Natl Acad Sci U S A (1990) 87:9868–72. doi:10.1073/pnas.87.24.9868
73. Cohen JD, Servan-Schreiber D. A theory of dopamine function and its role in cognitive deficits in schizophrenia. Schizophr Bull (1993) 19:85–104. doi:10.1093/schbul/19.1.85
74. Braver TS, Barch DM, Cohen JD. Cognition and control in schizophrenia: a computational model of dopamine and prefrontal function. Biol Psychiatry (1999) 46:312–28. doi:10.1016/S0006-3223(99)00116-X
75. Cohen JD, Servan-Schreiber D. Context, cortex, and dopamine: a connectionist approach to behavior and biology in schizophrenia. Psychol Rev (1992) 99:45–77. doi:10.1037/0033-295X.99.1.45
76. Cohen JD, Braver TS, O’Reilly RC. A computational approach to prefrontal cortex, cognitive control and schizophrenia: recent developments and current challenges. Philos Trans R Soc Lond B Biol Sci (1996) 351:1515–27. doi:10.1098/rstb.1996.0138
77. Smith EE, Jonides J. Storage and executive processes in the frontal lobes. Science (1999) 283:1657–61. doi:10.1126/science.283.5408.1657
78. Collette F, Van der Linden M. Brain imaging of the central executive component of working memory. Neurosci Biobehav Rev (2002) 26:105–25. doi:10.1016/S0149-7634(01)00063-X
79. Braver TS, Cohen JD, Nystrom LE, Jonides J, Smith EE, Noll DC. A parametric study of prefrontal cortex involvement in human working memory. Neuroimage (1997) 5:49–62. doi:10.1006/nimg.1996.0247
80. Cohen JD, Braver TS, Brown JW. Computational perspectives on dopamine function in prefrontal cortex. Curr Opin Neurobiol (2002) 12:223–9. doi:10.1016/S0959-4388(02)00314-8
81. Cools R, Gibbs SE, Miyakawa A, Jagust W, D’Esposito M. Working memory capacity predicts dopamine synthesis capacity in the human striatum. J Neurosci (2008) 28(5):1208–12. doi:10.1523/JNEUROSCI.4475-07.2008
82. Hazy TE, Frank MJ, O’Reilly RC. Banishing the homunculus: making working memory work. Neuroscience (2006) 139:105–18. doi:10.1016/j.neuroscience.2005.04.067
83. Cole MW, Schneider W. The cognitive control network: integrated cortical regions with dissociable functions. Neuroimage (2007) 37:343–60. doi:10.1016/j.neuroimage.2007.03.071
84. Lenartowicz A, McIntosh AR. The role of anterior cingulate cortex in working memory is shaped by functional connectivity. J Cogn Neurosci (2005) 17(7):1026–42. doi:10.1162/0898929054475127
85. Woodward TS, Cairo TA, Ruff CC, Takane Y, Hunter MA, Ngan ET. Functional connectivity reveals load dependent neural systems underlying encoding and maintenance in verbal working memory. Neuroscience (2006) 139:317–25. doi:10.1016/j.neuroscience.2005.05.043
86. Gazzaley A, Nobre AC. Top-down modulation: bridging selective attention and working memory. Trends Cogn Sci (2012) 16:129–35. doi:10.1016/j.tics.2011.11.014
87. Broome MR, Matthiasson P, Fusar-Poli P, Woolley JB, Johns LC, Tabraham P, et al. Neural correlates of executive function and working memory in the ‘at-risk mental state’. Br J Psychiatry (2009) 194:25–33. doi:10.1192/bjp.bp.107.046789
88. Liemburg EJ, Knegtering H, Klein HC, Kortekaas R, Aleman A. Antipsychotic medication and prefrontal cortex activation: a review of neuroimaging findings. Eur Neuropsychopharmacol (2012) 22:387–400. doi:10.1016/j.euroneuro.2011.12.008
89. Rasetti R, Sambataro F, Chen Q, Callicott JH, Mattay VS, Weinberger DR. Altered cortical network dynamics: a potential intermediate phenotype for schizophrenia and association with ZNF804A. Arch Gen Psychiatry (2011) 68:1207–17. doi:10.1001/archgenpsychiatry.2011.103
90. Barch DM, Carter CS, Braver TS, Sabb FW, MacDonald A III, Noll DC, et al. Selective deficits in prefrontal cortex function in medication-naive patients with schizophrenia. Arch Gen Psychiatry (2001) 58:280–8. doi:10.1001/archpsyc.58.3.280
91. Potkin SG, Turner JA, Brown GG, McCarthy G, Greve DN, Glover GH, et al. Working memory and DLPFC inefficiency in schizophrenia: the FBIRN study. Schizophr Bull (2009) 35:19–31. doi:10.1093/schbul/sbn162
92. Brown GG, Thompson WK. Functional brain imaging in schizophrenia: selected results and methods. Curr Top Behav Neurosci (2010) 4:181–214. doi:10.1007/7854_2010_54
93. Manoach DS. Prefrontal cortex dysfunction during working memory performance in schizophrenia: reconciling discrepant findings. Schizophr Res (2003) 60:285–98. doi:10.1016/S0920-9964(02)00294-3
94. Wager TD, Smith EE. Neuroimaging studies of working memory: a meta-analysis. Cogn Affect Behav Neurosci (2003) 3:255–74. doi:10.3758/CABN.3.4.255
95. Glahn DC, Ragland JD, Abramoff A, Barrett J, Laird AR, Bearden CE, et al. Beyond hypofrontality: a quantitative meta-analysis of functional neuroimaging studies of working memory in schizophrenia. Hum Brain Mapp (2005) 25(1):60–9. doi:10.1002/hbm.20138
96. van Snellenberg JX, Torres IJ, Thornton AE. Functional neuroimaging of working memory in schizophrenia: task performance as a moderating variable. Neuropsychology (2006) 20(5):497–510. doi:10.1037/0894-4105.20.5.497
97. Callicott JH, Bertolino A, Mattay VS, Langheim FJ, Duyn J, Coppola R, et al. Physiological dysfunction of the dorsolateral prefrontal cortex in schizophrenia revisited. Cereb Cortex (2000) 10:1078–92. doi:10.1093/cercor/10.11.1078
98. Callicott JH, Mattay VS, Verchinski BA, Marenco S, Egan MF, Weinberger DR. Complexity of prefrontal cortical dysfunction in schizophrenia: more than up or down. Am J Psychiatry (2003) 160:2209–15. doi:10.1176/appi.ajp.160.12.2209
99. Thermenos HW, Goldstein JM, Buka SL, Poldrack RA, Koch JK, Tsuang MT, et al. The effect of working memory performance on functional MRI in schizophrenia. Schizophr Res (2005) 74(2–3):19–94. doi:10.1016/j.schres.2004.07.021
100. Tan HY, Sust S, Buckholtz JW, Mattay VS, Meyer-Lindenberg A, Egan MF, et al. Dysfunctional prefrontal regional specialization and compensation in schizophrenia. Am J Psychiatry (2006) 163(11):1969–77. doi:10.1176/appi.ajp.163.11.1969
101. Quidé Y, Morris RW, Shepherd AM, Rowland JE, Green MJ. Task-related fronto-striatal functional connectivity during working memory performance in schizophrenia. Schiozphr Res (2013) 150(2–3):468–75. doi:10.1016/j.schres.2013.08.009
102. Perlstein WM, Carter CS, Noll DC, Cohen JD. Relation of prefrontal cortex dysfunction to working memory and symptoms in schizophrenia. Am J Psychiatry (2001) 158(7):1105–13. doi:10.1176/appi.ajp.158.7.1105
103. Guerrero-Pedraza A, McKenna PJ, Gomar JJ, Sarró S, Salvador R, Amann B, et al. First-episode psychosis is characterized by failure of deactivation but not by hypo- or hyperfrontality. Psychol Med (2012) 42(1):73–84. doi:10.1017/S0033291711001073
104. Carter CS, Perlstein W, Ganguli R, Brar J, Mintun M, Cohen JD. Functional hypofrontality and working memory dysfunction in schizophrenia. Am J Psychiatry (1998) 155(9):1285–7.
105. Meyer-Lindenberg A, Poline JB, Kohn PD, Holt JL, Egan MF, Weinberger DR, et al. Evidence for abnormal cortical functional connectivity during working memory in schizophrenia. Am J Psychiatry (2001) 158(11):1809–17. doi:10.1176/appi.ajp.158.11.1809
106. Meyer-Lindenberg AS, Olsen RK, Kohn PD, Brown T, Egan MF, Weinberger DR, et al. Regionally specific disturbance of dorsolateral prefrontal-hippocampal functional connectivity in schizophrenia. Arch Gen Psychiatry (2005) 62(4):379–86. doi:10.1001/archpsyc.62.4.379
107. Friston KJ, Frith CD. Schizophrenia: a disconnection syndrome? Clin Neurosci (1995) 3(2):89–97.
108. Huys QJ, Moutoussis M, Williams J. Are computational models of any use to psychiatry? Neural Netw (2011) 24:544–51. doi:10.1016/j.neunet.2011.03.001
109. Montague PR, Dolan RJ, Friston KJ, Dayan P. Computational psychiatry. Trends Cogn Sci (2012) 16:72–80. doi:10.1016/j.tics.2011.11.018
110. Lewis DA, Moghaddam B. Cognitive dysfunction in schizophrenia: convergence of gamma-aminobutyric acid and glutamate alterations. Arch Neurol (2006) 63:1372–6. doi:10.1001/archneur.63.10.1372
111. Lewis DA, Gonzalez-Burgos G. Neuroplasticity of neocortical circuits in schizophrenia. Neuropsychopharmacology (2008) 33:141–65. doi:10.1038/sj.npp.1301563
112. Gonzalez-Burgos G, Hashimoto T, Lewis DA. Alterations of cortical GABA neurons and network oscillations in schizophrenia. Curr Psychiatry Rep (2010) 12:335–44. doi:10.1007/s11920-010-0124-8
113. Volk DW, Eggan SM, Lewis DA. Alterations in metabotropic glutamate receptor 1 alpha and regulator of G protein signaling 4 in the prefrontal cortex in schizophrenia. Am J Psychiatry (2010) 167:1489–98. doi:10.1176/appi.ajp.2010.10030318
114. Schwartz TL, Sachdeva S, Stahl SM. Glutamate neurocircuitry: theoretical underpinnings in schizophrenia. Front Pharmacol (2012) 3:195. doi:10.3389/fphar.2012.00195
115. Friston K. Beyond phrenology: what can neuroimaging tell us about distributed circuitry? Annu Rev Neurosci (2002) 25:221–50. doi:10.1146/annurev.neuro.25.112701.142846
116. McIntosh AR. Towards a network theory of cognition. Neural Netw (2000) 13:861–70. doi:10.1016/S0893-6080(00)00059-9
117. Daunizeau J, David O, Stephan KE. Dynamic causal modelling: a critical review of the biophysical and statistical foundations. Neuroimage (2011) 58:312–22. doi:10.1016/j.neuroimage.2009.11.062
118. Daunizeau J, Preuschoff K, Friston KJ, Stephan KE. Optimizing experimental d esign for comparing models of brain function. PLoS Comput Biol (2011) 7:e1002280. doi:10.1371/journal.pcbi.1002280
119. Stephan KE, Kasper L, Harrison LM, Daunizeau J, den Ouden HE, Breakspear M, et al. Nonlinear dynamic causal models for fMRI. Neuroimage (2008) 42:649–62. doi:10.1016/j.neuroimage.2008.04.262
120. Salinas E, Sejnowski TJ. Gain modulation in the central nervous system: where behavior, neurophysiology, and computation meet. Neuroscientist (2001) 7:430–40. doi:10.1177/107385840100700512
121. Volman V, Levine H, Sejnowski TJ. Shunting inhibition controls the gain modulation mediated by asynchronous neurotransmitter release in early development. PLoS Comput Biol (2010) 6:e1000973. doi:10.1371/journal.pcbi.1000973
122. Stephan KE, Harrison LM, Kiebel SJ, David O, Penny WD, Friston KJ. Dynamic causal models of neural system dynamics: current state and future extensions. J Biosci (2007) 32:129–44. doi:10.1007/s12038-007-0012-5
123. Tana MG, Montin E, Cerutti S, Bianchi AM. Exploring cortical attentional system by using fMRI during a continuous performance test. Comput Intell Neurosci (2010) 2010:329213. doi:10.1155/2010/329213
124. Wang L, Liu X, Guise KG, Knight RT, Ghajar J, Fan J. Effective connectivity of the fronto-parietal network during attentional control. J Cogn Neurosci (2010) 22:543–53. doi:10.1162/jocn.2009.21210
125. Brazdil M, Mikl M, Marecek R, Krupa P, Rektor I. Effective connectivity in target stimulus processing: a dynamic causal modeling study of visual oddball task. Neuroimage (2007) 35:827–35. doi:10.1016/j.neuroimage.2006.12.020
126. Tan HY, Chen AG, Kolachana B, Apud JA, Mattay VS, Callicott JH, et al. Effective connectivity of AKT1-mediated dopaminergic working memory networks and pharmacogenetics of anti-dopaminergic treatment. Brain (2012) 135:1436–45. doi:10.1093/brain/aws068
127. Crossley NA, Mechelli A, Fusar-Poli P, Broome MR, Matthiasson P, Johns LC, et al. Superior temporal lobe dysfunction and frontotemporal dysconnectivity in subjects at risk of psychosis and in first-episode psychosis. Hum Brain Mapp (2009) 30:4129–37. doi:10.1002/hbm.20834
128. Deserno L, Sterzer P, Wustenberg T, Heinz A, Schlagenhauf F. Reduced prefrontal-parietal effective connectivity and working memory deficits in schizophrenia. J Neurosci (2012) 32:12–20. doi:10.1523/JNEUROSCI.3405-11.2012
129. Schmidt A, Smieskova R, Aston J, Simon A, Allen P, Fusar-Poli P, et al. Brain connectivity abnormalities predating the onset of psychosis: correlation with the effect of medication. JAMA Psychiatry (2013) 70(9):903–12. doi:10.1001/jamapsychiatry.2013.117
130. Zhang H, Wei X, Tao H, Mwansisya TE, Pu W, He Z, et al. Opposite effective connectivity in the posterior cingulate and medial prefrontal cortex between first-episode schizophrenic patients with suicide risk and healthy controls. PLoS One (2013) 8:e63477. doi:10.1371/journal.pone.0063477
131. Dayan P, Abbot LF. Theoretical Neuroscience: Computational and Mathematical Modeling of Neural Systems. Cambridge, MA: The MIT Press (2000).
132. Li B, Daunizeau J, Stephan KE, Penny W, Hu D, Friston K. Generalised filtering and stochastic DCM for fMRI. Neuroimage (2011) 58:442–57. doi:10.1016/j.neuroimage.2011.01.085
133. Allen P, Mechelli A, Stephan KE, Day F, Dalton J, Williams S, et al. Fronto-temporal interactions during overt verbal initiation and suppression. J Cogn Neurosci (2008) 20:1656–69. doi:10.1162/jocn.2008.20107
134. Allen P, Stephan KE, Mechelli A, Day F, Ward N, Dalton J, et al. Cingulate activity and fronto-temporal connectivity in people with prodromal signs of psychosis. Neuroimage (2010) 49:947–55. doi:10.1016/j.neuroimage.2009.08.038
135. Dauvermann MR, Whalley HC, Romaniuk L, Valton V, Owens DG, Johnstone EC, et al. The application of nonlinear dynamic causal modelling for fMRI in subjects at high genetic risk of schizophrenia. Neuroimage (2013) 73:16–29. doi:10.1016/j.neuroimage.2013.01.063
136. Whalley HC, Simonotto E, Flett S, Marshall I, Ebmeier KP, Owens DG, et al. FMRI correlates of state and trait effects in subjects at genetically enhanced risk of schizophrenia. Brain (2004) 127:478–90. doi:10.1093/brain/awh070
137. Whalley HC, Simonotto E, Marshall I, Owens DG, Goddard NH, Johnstone EC, et al. Functional disconnectivity in subjects at high genetic risk of schizophrenia. Brain (2005) 128:2097–108. doi:10.1093/brain/awh556
138. Friston KJ, Shiner T, FitzGerald T, Galea JM, Adams R, Brown H, et al. Dopamine, affordance and active inference. PLoS Comput Biol (2012) 8(1):e1002327. doi:10.1371/journal.pcbi.1002327
139. Brodersen KJ, Deserno L, Schlagenhauf F, Lin Z, Penny WD, Buhmann JM, et al. Dissecting psychiatric spectrum disorders. Neuroimage Clin (2013) 4:98–111. doi:10.1016/j.nicl.2013.11.002
140. Durstewitz D, Seamans JK. The computational role of dopamine D1 receptors in working memory. Neural Netw (2002) 15(4–6):561–72. doi:10.1016/S0893-6080(02)00049-7
141. Murray JD, Anticevic A, Gancsos M, Ichinose M, Corlett PR, Krystal JH, et al. Linking microcircuit dysfunction to cognitive impairment: effects of disinhibition associated with schizophrenia in a cortical working memory model. Cereb Cortex (2012) 24(4):859–72. doi:10.1093/cercor/bhs370
142. D’Ardenne K, Eshel N, Luka J, Lenartowicz A, Nystrom LE, Cohen JD. Role of prefrontal cortex and the midbrain dopamine system in working memory updating. Proc Natl Acad Sci U S A (2012) 109:19900–9. doi:10.1073/pnas.1116727109
143. den Ouden HE, Friston KJ, Daw ND, McIntosh AR, Stephan KE. A dual role for prediction error in associative learning. Cereb Cortex (2009) 19(5):1175–85. doi:10.1093/cercor/bhn161
144. den Ouden HE, Daunizeau J, Roiser J, Friston KJ, Stephan KE. Striatal prediction error modulates cortical coupling. J Neurosci (2010) 30(9):3210–9. doi:10.1523/JNEUROSCI.4458-09.2010
145. Brodersen KH, Schofield TM, Leff AP, Ong CS, Lomakina EI, Buhmann JM, et al. Generative embedding for model-based classification of fMRI data. PLoS Comput Biol (2011) 7(6):e1002079. doi:10.1371/journal.pcbi.1002079
146. Stephan KE, Penny WD, Moran RJ, den Ouden HE, Daunizeau J, Friston KJ. Ten simple rules for dynamic causal modeling. Neuroimage (2010) 49(4):3099–109. doi:10.1016/j.neuroimage.2009.11.015
147. Penny WD, Stephan KE, Mechelli A, Friston KJ. Comparing dynamic causal models. Neuroimage (2004) 22(3):1157–72. doi:10.1016/j.neuroimage.2004.03.026
148. Penny WD, Stephan KE, Daunizeau J, Rosa MJ, Friston KJ, Schofield TM, et al. Comparing families of dynamic causal models. PLoS Comput Biol (2010) 6(3):e1000709. doi:10.1371/journal.pcbi.1000709
149. Lisman JE, Coyle JT, Green RW, Javitt DC, Benes FM, Heckers S, et al. Circuit-based framework for understanding neurotransmitter and risk gene interactions in schizophrenia. Trends Neurosci (2008) 31:234–42. doi:10.1016/j.tins.2008.02.005
Dynamic causal modeling is general framework for model-based assessment of competing theories about neuronal circuits (18, 146). In particular, DCM is a generic Bayesian system identification technique, which allows for inference on “hidden” neurophysiological mechanisms that generated observed measures, such as blood-oxygen-level-dependent signal in functional magnetic resonance imaging (fMRI) or evoked responses measured with electroencephalography (EEG). The principle idea thereby is to formulate a simplified model of neuronal population responses (z) and combine this with a modality-specific forward model (λ) such that one can predict the measurement (y) that would arise from any particular neuronal circuit (18).
In DCM for fMRI, the dynamics of the neural states underlying regional BOLD response are modeled by a bilinear differential equation that describes how the neural states (x) change over time (t) as a function of endogenous inter-regional connections (matrix A), modulatory effects on these connections (matrix B), and direct (driving) inputs (matrix C) (Eq. A1) (18, 122). The endogenous connections represent coupling strengths in the absence of input uj to the system, whereas the modulatory effects represent task-specific alterations in this connectivity.
The bilinear state equation has subsequently been extended by a non-linear term, where the modulation of connection strengths by experimental inputs is supplemented by direct modulation with neural activity in one or more regions (119). In other words, non-linear DCMs allow addressing how the connection between two neuronal units is gated by activity in other units. This is of particular interest as gating processes represent a key mechanism for many neurobiological processes and thus increasing the biological realism of non-linear compared to bilinear DCMs. To this end, compared with the bilinear state equation, the new term in the non-linear equations are the D matrices (Eq. A2), which encode how the n regions gate connections in the system.
Bayesian model selection is an essential procedure of DCM studies as it can be used to test competing hypotheses (different DCMs) about the neural mechanisms generating the data. BMS rests on comparing the evidence of a predefined set of models (the model space). The model evidence is the probability of observing the empirical data, given a model, and represents a principled measure of model quality, derived from probability theory (147, 148). Concretely, it represents the mean predicted data under random sampling from the model’s priors or, alternatively, a principled measure of the balance between model fit and model complexity. A random-effects BMS approach has been suggested for group studies, which is capable of quantifying the degree of heterogeneity in a population while being extremely robust to potential outliers (20, 67). The probability that one model is more likely than any other model, given the group data, can be expressed by the exceedance probability (φk) of each model:
After inference on the most likely network architecture underlying a specific neural process, one can compare the parameter estimates of the most likely model obtained from BMS (winning model) for between-group inferences. However, statistical comparison of model parameter estimates across groups is only valid if those estimates stem from the same model. Given that different models may be found to be optimal across groups, Bayesian model averaging (BMA) has been recommended as standard approach for clinical DCM studies (146). BMA averages posterior parameter estimates over models, weighted by the posterior model probabilities (148). Thus, models with a low posterior probability contribute little to the estimation of the marginal posterior. In brief, BMS and BMA are central components of DCM studies to infer on neural mechanisms at the neural system level and on specific model parameters across groups, respectively (146).
In non-linear DCM analysis, the connection strengths between selected nodes are assessed for activity-dependent modulation of the reciprocal neuronal projections by the introduction of gating mechanisms. Non-linear DCM is applied to the models identified as winning models from the application of bilinear state equation. The bilinear model and the non-linear models differ only in the introduction of gating mechanisms such as a parametric response in the tested functional task. Such gating mechanisms are applied to nodal connections, which are expected to explain the variation in subject response to the functional task. The appropriate placement of the gating input is assessed through the application of model space partitioning and family inference. The exceedance probabilities of the models are compared and the non-linear models, which provide higher exceedance probabilities than the bilinear models are identified as winning models.
Keywords: computational neuropsychiatry, schizophrenia, fMRI, dynamic causal modeling, cognition, neurotransmitter, dopamine, glutamate
Citation: Dauvermann MR, Whalley HC, Schmidt A, Lee GL, Romaniuk L, Roberts N, Johnstone EC, Lawrie SM and Moorhead TWJ (2014) Computational neuropsychiatry – schizophrenia as a cognitive brain network disorder. Front. Psychiatry 5:30. doi: 10.3389/fpsyt.2014.00030
Received: 04 September 2013; Accepted: 10 March 2014;
Published online: 25 March 2014.
Edited by:
Stefan Borgwardt, University of Basel, SwitzerlandReviewed by:
Philip R. Corlett, Yale School of Medicine, USACopyright: © 2014 Dauvermann, Whalley, Schmidt, Lee, Romaniuk, Roberts, Johnstone, Lawrie and Moorhead. This is an open-access article distributed under the terms of the Creative Commons Attribution License (CC BY). The use, distribution or reproduction in other forums is permitted, provided the original author(s) or licensor are credited and that the original publication in this journal is cited, in accordance with accepted academic practice. No use, distribution or reproduction is permitted which does not comply with these terms.
*Correspondence: Maria R. Dauvermann, Division of Psychiatry, Royal Edinburgh Hospital, University of Edinburgh, Kennedy Tower, Morningside Park, Edinburgh EH10 5HF, UK e-mail:bS5yLmRhdXZlcm1hbm5Ac21zLmVkLmFjLnVrLA==bWFyaWEuZGF1dmVybWFubkBjaGlsZHJlbnMuaGFydmFyZC5lZHU=
Disclaimer: All claims expressed in this article are solely those of the authors and do not necessarily represent those of their affiliated organizations, or those of the publisher, the editors and the reviewers. Any product that may be evaluated in this article or claim that may be made by its manufacturer is not guaranteed or endorsed by the publisher.
Research integrity at Frontiers
Learn more about the work of our research integrity team to safeguard the quality of each article we publish.