- 1 Scientific Computing and Imaging Institute, University of Utah, Salt Lake City, UT, USA
- 2 Department of Psychiatry, University of North Carolina, Chapel Hill, NC, USA
- 3 Department of Computer Science, University of North Carolina, Chapel Hill, NC, USA
- 4 Biomedical Research Imaging Center, University of North Carolina, Chapel Hill, NC, USA
- 5 Department of Psychology, University of North Carolina, Chapel Hill, NC, USA
The use of structural magnetic resonance imaging (sMRI) and diffusion tensor imaging (DTI) in animal models of neuropathology is of increasing interest to the neuroscience community. In this work, we present our approach to create optimal translational studies that include both animal and human neuroimaging data within the frameworks of a study of post-natal neuro-development in intra-uterine cocaine-exposure. We propose the use of non-invasive neuroimaging to study developmental brain structural and white matter pathway abnormalities via sMRI and DTI, as advanced MR imaging technology is readily available and automated image analysis methodology have recently been transferred from the human to animal imaging setting. For this purpose, we developed a synergistic, parallel approach to imaging and image analysis for the human and the rodent branch of our study. We propose an equivalent design in both the selection of the developmental assessment stage and the neuroimaging setup. This approach brings significant advantages to study neurobiological features of early brain development that are common to animals and humans but also preserve analysis capabilities only possible in animal research. This paper presents the main framework and individual methods for the proposed cross-species study design, as well as preliminary DTI cross-species comparative results in the intra-uterine cocaine-exposure study.
Introduction
In the last decade, research in many psychiatric disorders has focused on a potential onset very early in life (Bale et al., 2010), perhaps even in utero (Brown and Derkits, 2010), before any onset of clinical symptoms (NIMH, 2008). While many neuroimaging research projects have provided novel insights (Kennedy et al., 2003; Shaw et al., 2008; Hazlett et al., 2009; Mosconi et al., 2009; Gilmore et al., 2010), overall progress into the neurobiological etiology of mental illness can be considered slow, as no major breakthrough toward a more complete understanding of a major psychiatric disorder has yet been reported. Main reasons include the necessary time scale to study a neuro-developmental disorder into adolescence, the correspondingly large monetary requirements, the difficulty of rigorously controlling the developmental environment and the need for handling ethical limitations on the collection of human developmental neurobiological data. In contrary to human clinical studies, animal models have several advantages, such as a well controlled environment and access to genetic modifications that allow for the creation of knock-out models (Nieman et al., 2005; Bugos et al., 2009), as well as the typically shorter lifespan of small animals, which provides ample time to study the disease from conception to adulthood. With respect to neuroimaging, rodent imaging has the advantage that scan time is commonly only limited by access to the imaging facility availability; another advantage is the availability of contrast enhancers that can be used in rodents that are not fit for use in human studies (Nieman et al., 2005). These enhancements result in higher resolution scans as well as increased signal to noise ratio (SNR) as compared human neuroimaging. Finally, there are inherent benefits to using rodent MRI models, such as the capability to augment and validate the neuroimaging results with traditional histology (Nieman et al., 2005).
While few experimental tools have shown themselves to be of use for thein-vivo study of neurobiological mechanisms in clinical human neuro-development, magnetic resonance imaging (MRI) has proven itself an invaluable tool for such research. MRI provides a non-invasive tool to probe brain anatomy and function. As it has no known, detectable influence on neuro-development, it allows for repeated longitudinal assessments (Paus et al., 1999; Gilmore et al., 2007; NIMH, 2008). Furthermore, MRI can be applied both in the clinical settings for humans and in animal research, as the MR data can be acquired and processed using similar methodology in both humans and animals as illustrated in this paper. Thus, findings have the potential to directly translate from basic science to clinical science, within reason. Rodent imaging is commonly acquired with specialized coils and high-field scanners (up to 17.4 T), although commercial-grade clinical scanners can be used for studies with less stringent requirements on spatial resolution and SNR (Pfefferbaum et al., 2004; Lee et al., 2006; Mayer et al., 2007; Pillai et al., 2011). The higher field strength and the smaller bore size of such high-field magnet not only allow for sub-millimeter resolution at appropriate SNR, but also provide a more homogeneous static magnetic field (Nieman et al., 2005). Nevertheless, the basic science community has been rather cautious to embrace MRI, as small animal researchers have a number of non-MRI tools at their disposal such as microscopy and electrophysiology that allow for dramatically enhanced neurochemical and anatomical assessment at considerably higher spatial resolution. On the other hand, MRI offers the advantage of assessing undistorted, three-dimensional structural changes (Nieman et al., 2005). Consequently, the use of rodent MRI has increased significantly in the last few years in order to study small animal models of human neuropathology via knock-outs (Badea et al., 2007; Lerch et al., 2008), lesion models (VanCamp et al., 2009; Zhang et al., 2009), as well as exposure models (Fatemi et al., 2009; Fernandes de Abreu et al., 2010; O’Leary-Moore et al., 2010). However, to our knowledge, none have attempted to do both human and rodent imaging in a parallel fashion and compare results across-species directly. Comparisons are usually ad hoc and compare a mouse model against human literature, or human neuroimaging results against mouse model literature. Here, we describe our approach to simultaneously study both aspects. This allows for the analysis to be as comparable as possible between human and rodent study settings. The standard comparison across existing studies usually entails mismatched study paradigms and measurements related to the drug exposure, assessment time points, assessed structures, and tracts, as well as MR protocols. Our proposed approach is designed to maximize the ability to infer findings from the rodent setting to the human one.
The overall focus of our research project is the elucidation of neurobiological and behavioral characteristics and responses of mothers that have used primarily cocaine during pregnancy, and of offspring prenatally exposed to cocaine, that could impact negatively on normal mother–infant interactions. Assessment of neurobiological changes includes structural imaging as a measure in human and animal infants and mothers to assess differences in brain structure and maturation.
In this paper, we present our proposed approach toward a cross-species neuroimaging with synchronized, comparative assessments of rodent pups and human neonates. In the next sections, we will first discuss the general concept of our parallel assessment approach. This approach demands comparative settings with respect to assessment age, MR imaging acquisition sequences as well as the MR image analysis methodology. These aspects are all discussed by presenting data from our ongoing study on intra-uterine cocaine-exposure and its effect on early post-natal brain development. It is also important to note that while this paper focuses on the neuroimaging aspects, our ongoing project devotes similar attention toward physiological, behavioral, and biosample measurements.
Materials and Methods
Study on Post-Natal Brain Development Following Intra-Uterine Cocaine-Exposure
We are illustrating our parallel assessment approach for cross-species neuroimaging studies employing an ongoing multidisciplinary, translational research project that focuses on the elucidation of neurobiological, and behavioral characteristics of offspring prenatally exposed to cocaine. While maternal cocaine use is known to be highly correlated with maternal neglect and poorer mother–infant interactions in both human and animal models, there is little known about the potentially pathologic brain development and the ensuing abnormal physiological/behavioral responses in infants prenatally exposed to cocaine that may in turn impact parenting behaviors of both drug using and non-using mothers.
The project assesses both intra-uterine cocaine-exposure in humans as well as a small animal model using Sprague-Dawley rats. The human part of our study was approved by the institutional review board of the University of North Carolina School of Medicine. All rodent procedures were approved by the institutional animal care and use committee of the University of North Carolina. While characterizing the influence of prenatal cocaine-exposure on post-natal development directly in human infants is the main goal of the project, such a study is nearly impossible within controlled settings. Cocaine abusing mothers often also abuse other drugs such as alcohol and nicotine during pregnancy. Also social environment in both pre and post-natally settings is commonly non-conventional. These factors are all potential confounders for an appropriate analysis. In contrast, prenatal cocaine-exposure in rodent models can be tightly controlled such that effects on the rodent brain can be clearly described.
We focus here on the neuroimaging aspects of this project, where brain structural abnormalities are hypothesized in regions important for the production of relevant sensory stimuli and integration of complex signals. Cocaine-exposed individuals are hypothesized to exhibit increased ventricular size, reduced hypothalamic, limbic, auditory, and olfactory regions, as well as delayed myelination of tracts. Deficits in myelination following long term cocaine-exposure in humans has been a consistent finding, as well as enlarged ventricles and localized white matter (WM) reduction (Moeller et al., 2005; Schlaepfer et al., 2005). Moeller et al. (2005) correlated reduced myelination in the genu and rostral body of the corpus callosum in cocaine-abusers to behavioral scores of impulsivity. This translational, interdisciplinary project aims to better understand how drug abuse affects brain development in the intra-uterine exposed offspring, as well as how the resulting behavioral characteristics may influence neglect. This could result in early and continuing intervention strategies to offset some of the negative consequences of cocaine abuse during and post pregnancy.
General Concept
Magnetic resonance imaging follows a parallel design (see Figure 1) where human subjects are imaged on high-field human scanners (3 T Siemens Trio for adults, 3 T Siemens Allegra for neonates), whereas rodent imaging is using a dedicated high-field animal scanner (9.4 T Bruker). With primary interest in anatomical and WM diffusion imaging, we selected optimal imaging sequences on all scanners in regard to maximum contrast for brain tissue types, fluid, and WM diffusivity. Image processing and analysis methodologies and tools developed by our group are generic with respect to the type of species imaged, and we apply the same processing tools to characterize tissue types, to extract anatomical structures, and to quantify WM integrity properties at each voxel and also for selected fiber tracts of interest. In processing steps that require subject-specific anatomical templates, often in the form of probabilistic, normative brain atlases (Cocosco et al., 1997; Prastawa et al., 2005), we use existing atlases for human brain analysis and generate new atlases for rodent adult and pup analysis. Anatomical structures and WM tracts hypothesized to show changes due to drug abuse or drug exposure can then be compared at the subsequent stage of biostatistical analysis.
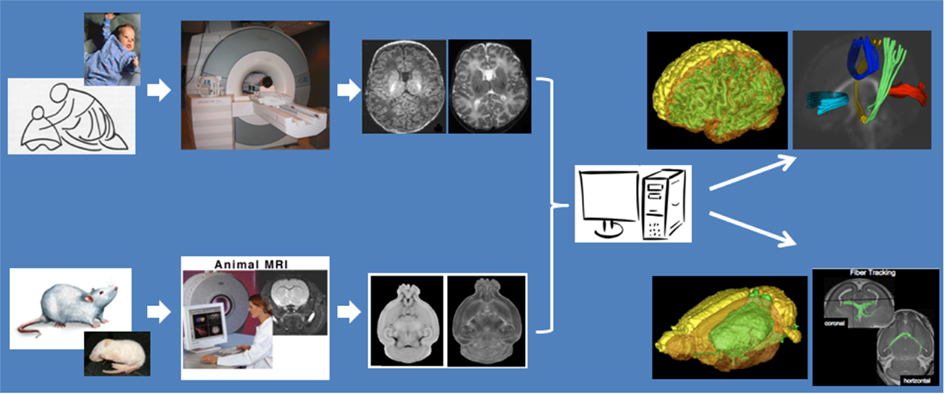
Figure 1. Mother and infant neuroimaging of humans and animals using dedicated scanners, and comparable imaging sequences and image processing methods for inter-species studies.
Subject Age
Assessment of both behavioral and neuroimaging measurements at comparative ages for humans and rodents is quite difficult to establish, as not all brain development processes happen along parallel trajectories. For example, rodent pups are born with their eyes closed and remain so until post-natal day 5–6, resulting in a comparatively slower visual system development. Similar disparate relationships between the two species exist in social systems, learning and memory, stress, and numerous other brain systems. This inconsistency in developmental trajectories a cross-species means that it is important to define a priori the measurements of interest and choose the assessment age in the studied species according to a similar stage in development. In addition to the consideration of developmental stage, we also need to take into account that neuroimaging of human infants in their first year of life is usually restricted to annual or biannual measurements by local institutional review boards (IRB). No such considerations are in place for small animal imaging, which offers thus a chance to capture a more detailed characterization of the neuro-development process with respect to the developmental time scale than is possible in human clinical research studies. In Figure 2 several milestones of post-natal brain development are compared between humans and rats (data from Watson et al., 2006). While several neuro-developmental milestones are mapped across-species well in Figure 2, others clearly are mismatched such as cerebellar growth or olfactory tract myelination.
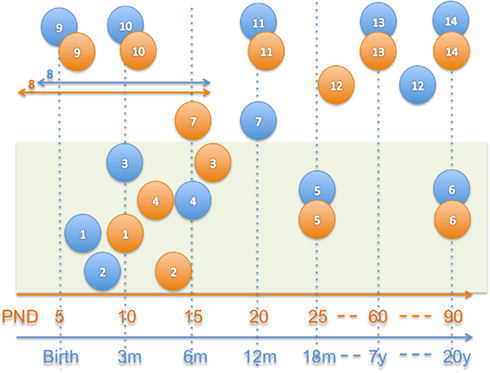
Figure 2. Approximate neuro-development comparison in humans (blue) and rats (orange; Watson et al., 2006). 1–6 = myelination, 1 = myelination onset internal capsule, 2 = onset olfactory tract, 3 = onset anterior commissure, 4 = onset fornix, 5 = 50% myelination of corpus callosum, 6 = mature myelination, 7 = 50% cerebellum size, 8 = peak synaptogenesis period, 9 = maximum brain growth velocity (peak), 10 = cortical dominance established, 11 = mature cerebral metabolism, 12 = adult pattern of slow wave and REM sleep, 13 = adult brain weight, 14 = mature prefrontal cortex.
For our cocaine-exposure study we chose to study auditory, emotional, and olfactory aspects. Consequently, hippocampus, amygdala, and olfactory bulb are some of the main target measures. The human neonate imaging time point was preset at 1–6 weeks of age (median at 3 weeks) by the availability of prior control data from a large study of normal development (Gilmore et al., 2007; Marc et al., 2010). We selected two pup imaging time points, one early time point at post-natal day (PND) five where pups are at a similar neuro-developmental stage for the selected measures to human 1-week-old neonates, as well as a second later time point at PND 14 with myelination stages in the olfactory bulb comparable to human infants at about 8 weeks of age, as well as established cortical dominance comparable to human development at about 12 weeks of age (Bayer et al., 1993; Watson et al., 2006). All image assessments occur during peak synaptogenesis, which ends at PND 16 in rats and 8 months in humans, as well as prior to mature cerebral metabolism, adult sleep patterns, and 50% cerebral myelination. The use of two separate time points as compared to the single human time point also allows us to better characterize the developmental impact of intra-uterine cocaine-exposure in the rat model.
MR Imaging
We chose anatomical and structural imaging protocols on the various high-field MRI scanners which provided optimal contrast for tissue type and fluid, and adequate WM diffusion information This includes high-resolution sequences for T1- and T2-weighted scans, which are mainly used for structural volumetry analysis, as well as for diffusion tensor imaging (DTI) scans, which are used for brain WM connectivity information. Derived DTI property maps focus on the local fiber integrity [fractional anisotropy (FA) and axonal diffusivity (AD)], the local degree of myelination [Zhang et al., 2009; radial diffusivity (RD)] as well as the level of micro-organization [mean diffusivity (MD)].
Animal imaging
Animal imaging is performed on a Bruker 9.4 T horizontal 30 cm MR scanner with a 800 mT/m gradient coil. The system has a 35 mm quad volume coil for transmit and receive. In addition to allowing acquisition of high-resolution images, the available high gradient coil greatly facilitates the proposed DTI. A small animal heating system is integrated with the animal bed. Finally, a MR compatible small animal monitoring system is available to provide the physiological monitoring, including respiration, ECG and body temperature, while animals are being scanned. Several imaging sequences are used to provide high-resolution anatomical as well as DTI images. A high-resolution (minimally 150 μm × 150 μm × 110 μm) 3D RARE DTI sequence with diffusion gradients applied in six non-collinear directions is used to acquire DTI imagery (Cai et al., 2009). Two navigator-echoes are utilized in this sequence to correct for motion artifacts. The total data acquisition time is within 3–4 h. Animals are maintained under ad lib conditions for 24 h prior to imaging. General anesthesia is induced via gas isoflurane (1–2% for induction and 0.75–1% for maintaining anesthesia). The study design includes a total of 165 rat and pup MRI and DTI scans.
Human infant imaging
The high-field 3 T scanning (Siemens 3 T Allegra head-only) of newborns uses parallel imaging (eight-channel receiver array head coil) to provide optimal contrast-to-noise ratio and optimal success rate for motion-free scans of non-sedated newborns. Structural imaging sequences include a turboflash, rapid gradient echo (MP-RAGE) T1-weighted and a turbo spin echo, dual-echo (proton density and T2-weighted). Spatial resolution is 1 mm × 1 mm × 1 mm voxel for T1-weighted images and 1.25 mm × 1.25 mm × 1.5 mm voxel for proton density/T2-weighted images. For diffusion weighted imaging, a single shot echo planar SE DTI imaging sequence is used. Forty-two unique gradient direction images at b = 1000 s/mm2 are acquired and seven images without diffusion gradient (b = 0; Gilmore et al., 2007). The total scan time is about 20 min. At the final stage of the project, 120 children are planned to be enrolled in this study, 60 with prenatal exposure to cocaine and 60 normal controls.
Image Analysis
In this section we will focus on the methods and design of the brain MR image analysis pipelines. As was the case for our selection of the MR imaging sequences, while these pipelines have been developed for our cocaine-exposure projects, they are generally applicable to any human or rodent MR neuroimaging projects. Regional selections for the structural analysis as well as the fiber selection for the DTI analysis are synchronized, such that the findings can be compared between the human and rodent arms of our project.
Structural MRI analysis workflow
Atlas space. A general necessity in neuroimaging is the ability to compare findings across different studies, and for that purpose MR datasets are commonly reoriented to a standard, prior atlas space, usually a variant of the MNI (Mazziotta et al., 2001) atlas space in human neuroimaging and the Paxinos (Paxinos and Franklin, 2001; MacKenzie-Graham et al., 2004) or Waxholm (Johnson et al., 2010) atlas space in rodents. While such atlas spaces are commonly well defined and publicly available for the adult brain, this is not the case for developing subjects. As part of our neuro-developmental projects we generated atlas images that represent an average brain anatomy at every developmental stage of study. In this study, we employed a prior human neonate T1-weighted atlas image (Prastawa et al., 2005; Gilmore et al., 2010), and we computed novel average DTI atlas images for Sprague-Dawley rats at PND five and 14 from 10 post-mortem subject each (see also Figure 3). All these atlases were built using a joint deformable registration of all training datasets into a single, iteratively improving the unbiased average image that has a minimal deformation to all training images, as described in (Joshi et al., 2004; Styner et al., 2009; AtlasWerks1). Prior to the deformable atlas building, one selected training image was rigidly aligned to a prior atlas space (such as the adult MNI space) and then all other training images were affinely registered to it. Furthermore, skull stripping, initialized via the automatic BET2 (Jenkinson et al., 2005) method followed by manual post-processing using ITKSnap2 (Yushkevich et al., 2006), and intensity calibration using pairwise histogram quantile matching via AutoSeg3 (Gouttard et al., 2007) was performed prior to atlas building.
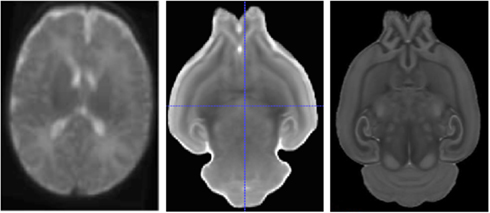
Figure 3. Human neonate T2-weighted atlas (left) and rodent MD atlas at PND 5 (middle) and PND 14 (right).
Atlas priors. On the above mentioned atlas image space, we further define additional prior information that is employed in the subsequent image analysis steps. Such spatial prior information can be encoding both voxel-wise attributes, such as spatial tissue probability maps, and geometric attributes, such as surfaces or WM fiber tracts. In our structural MRI atlases, we define the following voxel-wise attribute maps: (a) probabilistic tissue maps for WM, gray matter (GM), and cerebrospinal fluid (CSF) in humans (Prastawa et al., 2005), for WM and two separate classes of GM (Lee et al., 2009) in rodents; (b) probabilistic or hard label maps for brain structures (hippocampus, amygdala, caudate/putamen/striatum, globus pallidus, and lateral ventricles in both human and rodent atlas); and (c) hard label maps for cortical lobe parcellations (hemispheric definitions of occipital, temporal, parietal, frontal, and prefrontal lobes in both human and rodent atlas). All label definitions (b, c) were established by experts via manual outlining with ITKSnap.
Brain tissue segmentation. Following rigid registration into the atlas space, all MR images are automatically segmented into the major brain tissue regions using an atlas-based expectation maximization (EM) scheme (Van Leemput et al., 1999; Prastawa et al., 2005; see also brain renderings in Figure 1). Human neonate data employed an adapted EM version that allows the separation WM into unmyelinated an myelinated regions (Prastawa et al., 2005). Rodent brain segmentations use the same parameter settings but separate atlases and tissue priors (via ABC4). It is noteworthy that the relative sizes of GM and WM regions is vastly different in the human and rodent brain, e.g., the hippocampus is an order of magnitude larger, measured relative to the overall brain size (Lee et al., 2009). Thus, whole brain tissue measurements are difficult to compare between species and thus are of limited use in cross-species studies. Nevertheless, our brain tissue segmentation step provides an overall segmentation of the brain without the need for skull stripping. In fact, the computed tissue segmentation serves as a mean to strip the skull from the images, a necessary processing step before the regional segmentation step can be computed (Oguz et al., 2011). Manual post-processing is employed if necessary. It further noteworthy that our EM based segmentation method provides an intensity inhomogeneity correction that ensures that the different tissue classes appear homogeneously with similar intensities across the image.
Regional segmentation. In this step, we subdivide the brain into several regions via a deformable fluid registration (Joshi et al., 2004; AtlasWerks/AutoSeg) of the prior atlas (Gouttard et al., 2007; Lee et al., 2009; see Figure 4 for an example). Since the registration method is matching directly the intensities of the atlas image with the intensities in the subject MR images, intensity calibration using pairwise histogram quantile matching (Gouttard et al., 2007) is applied before registration. Using the computed deformable transform, prior regional atlas maps are propagated to the individual image (a) to directly define the subcortical structure measurements and (b) to be combined with the tissue segmentations for cortical, lobar parcellation measurement.
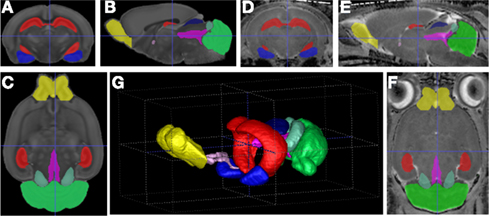
Figure 4. Visualization of the atlas registration based regional segmentation for PND14: (A–C) Orthogonal slice views of the atlas; (D–F) views on an example dataset; (G) 3D-rendering of example segmentation. Red: hippocampus, green: cerebellum, blue: amygdala, yellow: olfactory bulb.
While the same registration method should be employed in human and rodent MRI data, the registration parameters have to be optimized separately for each setting. In general, human brain anatomy exhibits much higher inter-subject variability than small animal models due to the fact that such small animal models do not have any complex cortical folds which are the main contributors to the inter-subject variability in humans; the variability is further reduced due to the fact that the animals are commonly only investigated within a genetically similar population, such as only Sprague-Dawley rats in our study. In order to match major cortical folds in the human settings, registration parameters are chosen to provide a considerably more relaxed deformation model than in the small animal settings. The parameters used in our human study are the default parameters in the AutoSeg package.
Cortical thickness analysis. As a final step in the structural analysis workflow, we compute local cortical thickness measurements using the cortical GM segmentation in humans (Vachet et al., 2011; via GAMBIT5) and the regional neocortex segmentation in rodents (via ShapeWorks6; Lee et al., 2011; Figure 5). While the cortical thickness is estimated from the segmentations in a voxel-wise fashion, it is analyzed on the cortical surface in a surface location wise fashion. For this purpose, a group-wise correspondence is established on the cortical surface (Oguz et al., 2008) using a particle-based entropy optimization over surface location, as well as sulcal depth measurements in human data due to the folded nature of the human cortex (GAMBIT). Cortical thickness measurements at corresponding surface locations are then extracted and statistically analyzed using the statistical analysis toolbox SurfStat7.
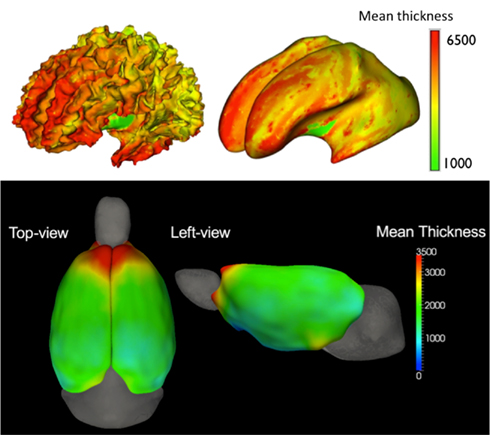
Figure 5. Mean cortical thickness (in μm) in a human 2 year old population (top, seen on the original white matter surface and the corresponding inflated brain surface; Vachet et al., 2011) and an adolescent rat population (bottom, seen from two viewpoints; Lee et al., 2011).
DTI analysis workflow
Prior to any processing, a strict diffusion weighted images (DWI)/DTI quality control (QC), eddy current and motion correction was performed for all DWI scans via DWI/DTI data suffers from inherent low SNR, motion, and eddy current artifacts. These artifacts can be too severe for a correct and stable estimation of the diffusion tensor without strict QC and correction methods. Thus, all datasets are subjected to a strict QC using DTIPrep8 (Liu et al., 2010), which identifies signal corruption artifacts in the DWI, as well as corrects for motion and eddy current artifacts. Skull stripping is performed automatically via tissue segmentation masks from co-registered structural MRI data. Diffusion tensor images are computed by standard weighted least-square estimation.
Regional analysis using structural segmentations. White matter integrity is assessed in our DTI analysis at three levels of scale. The first step analyzes WM properties within larger regions using the previously computed regional segmentation of the structural MRI data. For human DTI datasets, the corresponding T2-weighted structural image is registered with the baseline (b = 0) scan and the regional segmentations are propagated accordingly. We employ affine registration within 3D Slicer9 due to the presence of DTI distortion artifacts. As we compute the regional segmentations directly on the DTI property maps for the rat MRI datasets, no registration is required for this data. Within each region, including both the cortical parcellations as well as the subcortical structures, we compute the mean and median DTI FA, MD, AD, and RD measures for further statistical analysis. While region analysis usually provides stable measurements of both white and GM regions, WM regions are not well represented, as different fiber tracts with likely different developmental maturation trajectories are lumped together. For example, the frontal lobe WM region contains information from fiber tracts such as the cerebrospinal tract, which myelinates early in development mainly during the first year of life (Goodlett et al., 2009), as well as the arcuate fasciculus, which myelinates later.
Voxel-wise analysis via atlas mapping. The second DTI analysis step provides a highly localized voxel-wise analysis (Liu et al., 2009), in contrast to the regional step that lumps together rather large regions. For this voxel-wise analysis, all datasets are mapped into a common reference space using the fluid diffeomorphic registration based atlas building (Joshi et al., 2004) also employed in the structural analysis (via AtlasWerks). This creates an unbiased analysis space, which allows the examination of the whole WM. The discovered findings are often considered for hypothesis generation or as preliminary findings that have to be confirmed in the third DTI analysis step.
Tract-based DTI analysis via atlas mapping. In the third DTI analysis step, we measure WM diffusion properties along selected tracts of interest (see Figure 6). This step thus provides a curvilinear regional analysis at an intermediate scale between the first two analysis steps. Using the atlas space generated in the previous step, Goodlett et al. (2009) developed a population-based analysis scheme that uses fiber tractography in the common atlas space to generate statistics of tract-based diffusion properties across all images in the population. DTI fibers on the DTI altas are computed with standard streamline tractography in 3D Slicer. Measurements of FA mean diffusivity (MD), and axial and radial diffusivity (AD, RD) are extracted at well defined locations along each tract (Fillard et al., 2003; Corouge et al., 2005; Goodlett et al., 2005; via DTITractStat10) to do group comparisons. In our cocaine study, the selected tracts of interest include major tracts such as the motor tract, sensory tract, uncinate fasciculus, fornix, arcuate fasciculus, splenium tract, genu tract, and the inferior longitudinal fasciculus. This fiber based DTI analysis method has previously been used in large clinical studies, such as our ongoing large neonatal MRI study (Gilmore et al., 2007). Figure 6 demonstrates tract-based visualizations of WM diffusion properties based on 67 neonatal subjects in our cocaine study (31 controls, 13 cocaine, and 23 nicotine only subjects (Gerig et al., 2010; Gouttard et al., 2010).
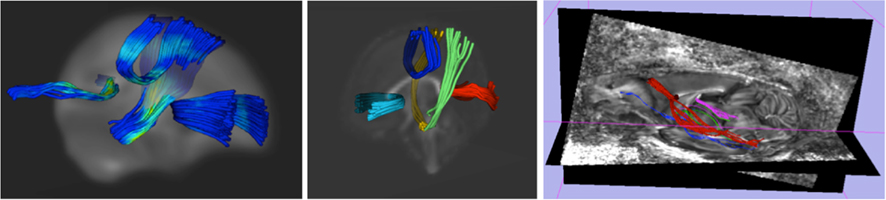
Figure 6. Left/middle: white matter fiber tracts (splenium/red, genu/cyan, mid-corpus callosum/blue and motor tracts/green) obtained from population-based analysis of neonatal DTI with color coding of fractional anisotropy (left) and as multi-colored fiber tracts (middle). Right: genu fiber tract (red) in a rodent DTI scan (anterior commissure = blue, hippocampal commissure = pink).
Preliminary Comparative DTI Analysis
Our proposed cross-species analysis framework is currently being applied to the previously described study on intra-uterine cocaine-exposure and brain development. To highlight the proposed framework, we present preliminary results that focus on the fiber tract-based DTI analysis of the inter-hemispheric genu tract, which connect the left and right hemispheric prefrontal cortices through the corpus callosum. In Figure 6/middle, the human genu tract is highlight in light blue, whereas in Figure 6/right, the rodent genu tract is shown in red including also non-prefrontal projections, which were excluded in the preliminary results presented in Figure 7. The human results were computed from scans of 10 neonates exposed to cocaine intra-uterinely as well as 22 age matched controls. Rodent results were computed from scans of 20 cocaine-exposed Wistar rats at PND 14 and 20 ages matched untreated controls. Figure 7 shows the surprising, though non-significant (trends at p = 0.08 to 0.10) results of heightened FA measures in the corpus callosum region (center section of the profile) of the genu fiber tract in both human and rodent analyses. The similarities of group differences between the human and rodent results are also quite evident.
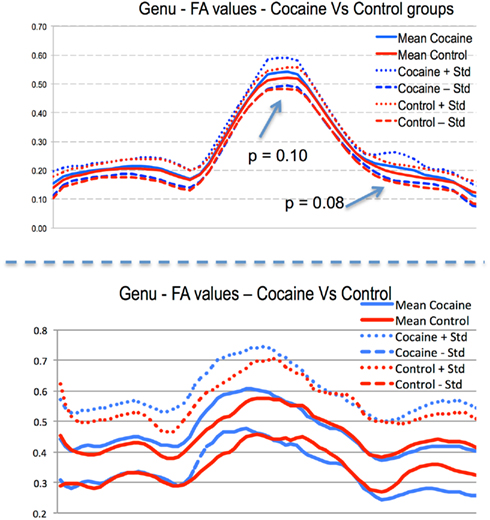
Figure 7. Preliminary DTI–FA profile results of the genu fiber tract in the intra-uterine cocaine-exposure study (plot shows left hemisphere to right hemisphere). Top: human subjects, bottom: rodent subjects. Few locations show statistical trends with surprising similarities across-species in group differences along most of the tract.
Discussion
This report describes the study design of the neuroimaging and image processing setup for a translational animal–human translational project to study effects of drug-abuse in mothers and drug-exposure of infants. It is discussed that the choice of scanners and imaging protocols for 3D anatomical and diffusion imaging is carefully optimized with respect to the type of species and age of subjects. Human adult imaging uses a full-body 3 T scanner, whereas neonatal imaging makes use of a head-only 3 T device which facilitates subject management. Animal imaging requires much higher field strength due to much smaller structures, and this study therefore make use of a 9.4 T special animal research scanner. It is discussed that pulse sequences are optimized on each device to achieve maximum soft tissue contrast and also WM diffusion information. Image processing, on the other hand, uses exactly the same underlying methodological and computational concepts, and can make use of the same toolboxes originally developed for human imaging. We can thus measure brain WM, GM, cortical thickness, size, and shapes of subcortical structures, volume of lobar regions, and WM diffusion in regions and along fiber tracts in animals and humans and in infants and adults. This allows us to capture relevant anatomical and WM property changes from normal due to drug exposure across-species. Comparison across-species is not done at the imaging level, but at the level of statistical analysis of image-derived assessments of brain changes. Information obtained from animal models can thus help us to generate possible hypothesis for human brain maturation and changes as observed in non-invasive MRI and DTI imaging.
It is noteworthy to keep in mind that despite all the efforts toward a cross-species analysis framework, no rodent model of neuro-development will sufficiently characterize the human teratologic impact of drug abuse. Nevertheless, such rodent models provide highly valuable information highlighted as evidenced by the vast literature on this topic. With respect to the framework presented here, we cannot compare the brain morphology between humans and rodents directly, e.g., by combining human and rodent atlases based results in a voxel-wise fashion. Rather we can compare findings indirectly, e.g., reproducible findings of delayed maturation in WM fibers within the genu region of the corpus callosum both in humans and rodents would be a strong indication that such a finding in infants would be due to the cocaine-exposure and not one of the many possible confounding factors. Our framework aims to enhance the confidence in such inferred cross-species findings.
Animal and human capabilities for imaging carry significant differences that make such a parallel approach very attractive and potentially a powerful way to get better insight in brain changes due to drug abuse or exposure but also in other scenarios. Human brains show large individual variability within a population, which presents significant challenges for measurements of comparable regional and functional properties. Rat brains, on the other hand, show very small anatomical variability due to the fact that they can be genetically controlled. Further, human in vivo imaging inherently is limited by safety and patient comfort constraints that affect scan times and repetition of scanning for longitudinal assessment. Choices of spatial resolution and of multi-modal imaging sequences are therefore always limited by the total scan time but also maximum time per image sequence to avoid artifacts due to patient motion. These limitations are even more pronounced in imaging infants since sedation is limited to subjects with indication of pathology, which permits clinical scanning. Animal imaging brings much more flexibility concerning scanning types and times since subjects are imaged under general anesthesia.
Conflict of Interest Statement
The authors declare that the research was conducted in the absence of any commercial or financial relationships that could be construed as a potential conflict of interest.
Acknowledgments
We would like to acknowledge the following funding sources UNC IDDRC HD 03110, NINDS R42 NS059095, NIDA P01 DA022446-01, RC1AA019211. Furthermore, we would like to thanks Fulton Crews, Allan Johnson and Sheryl Moy for their insightful discussion on the topic of cross-species studies and small animal imaging. NICHD P30 HD03110 awarded to J. Piven.
Footnotes
- ^http://www.sci.utah.edu/software/13/370-atlaswerks.html
- ^http://www.itksnap.org
- ^http://www.nitrc.org/projects/autoseg
- ^http://www.nitrc.org/projects/abc/
- ^http://www.nitrc.org/projects/gambit/
- ^http://www.nitrc.org/projects/shapeworks/
- ^http://www.nitrc.org/projects/surfstat/
- ^http://www.nitrc.org/projects/dtiprep
- ^http://www.slicer.org
- ^http://www.nitrc.org/projects/dti_tract_stat/
References
Badea, A., Nicholls, P., Johnson, G., and Wetsel, W. (2007). Neuro-anatomical phenotypes in the reeler mouse. Neuroimage 34, 1363–1374.
Bale, T. J., Baram, T. Z., Brown, A. S., Goldstein, J. M., Insel, T. M., McCarthy, M. M., Nemeroff, C. B., Reyes, T. M., Simerly, R. B., Susser, E. S., and Nestler, E. J. (2010). Early life programming and neuro-developmental disorders. Biol. Psychiatry 68, 314–319.
Bayer, S. A., Altman, J., Russo, R. J., and Zhang, X. (1993). Time-tables of neurogenesis in the human brain based on experimentally determined patterns in the rat. Neurotoxicology 14, 83–144.
Brown, A. S., and Derkits, E. J. (2010). Prenatal infection and schizophrenia: a review of epidemiologic and translational studies. Am. J. Psychiatry 167, 261–280.
Bugos, O., Bhide, M., and Zilka, N. (2009). Beyond the rat models of human neurodegenerative disorders. Cell. Mol. Neurobiol. 29, 859–869.
Cai, Y., Hong, Y. L., McMurray, M. S., Johns, J. M., Lin, W., and An, H. (2009). “High-field diffusion tensor magnetic resonance histology using rapid acquisition with relaxation enhancement (DTI RARE),” in Annual Meeting of the Society for Neuroscience, Chicago.
Cocosco, C. A., Kollokian, V., Kwan, R. S., and Evans, A. C. (1997). BrainWeb: online interface to a 3D MRI simulated brain database. Neuroimage 5, S425.
Corouge, I., Fletcher, T., Joshi, S., Gilmore, J. H., and Gerig, G. (2005). “Fiber tract-oriented statistics for quantitative diffusion tensor MRI analysis,” in Lecture Notes in Computer Science LNCS, Vol. 3749, eds J. S. Duncan and G. Gerig (Palm Springs, CA: Springer Verlag), 131–138.
Fatemi, S. H., Folsom, T., Reutiman, T., Abu-Odeh, D., Mori, S., Huang, H., and Oishi, K. (2009). Abnormal expression of myelination genes and alterations in white matter fractional anisotropy following prenatal viral influenza infection at E16 in mice. Schizophr. Res. 112, 46–53.
Fernandes de Abreu, D., Nivet, E., Baril, N., Khrestchatisky, M., Roman, F., and Féron, F. (2010). Developmental vitamin D deficiency alters learning in C57Bl/6J mice. Behav. Brain Res. 208, 603–608.
Fillard, P., Gilmore, J., Lin, W., and Gerig, G. (2003). “Quantitative analysis of white matter fiber properties along geodesic paths,” in Lecture Notes in Computer Science LNCS #2879, eds R. E. Ellis and T. M. Peters (Montreal, CA: Springer), 16–23.
Gerig, G., Grewen, K., Gouttard, S., Gilmore, J., Bitaud, E., and Johns, J. (2010). “Prenatal cocaine effects on neonatal white matter development,” in Annual Meeting of the Society for Neuroscience, San Diego, CA.
Gilmore, J., Kang, C., Evans, D., Wolfe, H., Smith, J. K., Lieberman, J. A., Lin, W., Hamer, R., Styner, M., and Gerig, G. (2010). Prenatal and neonatal brain structure and white matter maturation in children at high risk for schizophrenia. Am. J. Psychiatry 167, 1083–1091.
Gilmore, J. H., Lin, W., Corouge, I., Vetsa, Y. S. K., Smith, J. K., Kang, C., Gu, H., Hamer, R. M., Lieberman, J. A., and Gerig, G. (2007). Early postnatal development of corpus callosum and corticospinal white matter assessed with quantitative tractography. AJNR Am. J. Neuroradiol. 28, 1789–1795.
Goodlett, C., Corouge, I., Jomier, M., and Gerig, G. (2005). “A Quantitative DTI Fiber Tract Analysis Suite,” The Insight Journal, Vol. ISC/NAMIC/MICCAI Workshop on Open-Source Software, Palm Springs, CA.
Goodlett, C. B., Thomas, Fletcher, P. T., Gilmore, J. H., and Gerig, G. (2009). Group analysis of DTI fiber tract statistics with application to neurodevelopment. Neuroimage 45(Supp. 1), S133–S142.
Gouttard, S., Grewen, K., Gerig, G., Gilmore, J., Bitaud, E., and Johns, J. (2010). “Prenatal cocaine effects on infant brain development,” in Annual Meeting of the Society for Neuroscience, San Diego.
Gouttard, S., Styner, M., Joshi, S., Smith, R. G., Cody, H., and Gerig, G. (2007). “Subcortical structure segmentation using probabilistic atlas priors,” in Proceedings in SPIE Medical Imaging, San Diego, CA.
Hazlett, H., Poe, M., Lightbody, A., Gerig, G., Macfall, J. R., Ross, A. K., Provenzale, J., Martin, A., Reiss, A., and Piven, J. (2009). Teasing apart the heterogeneity of autism: same behavior, different brains in toddlers with fragile X syndrome and autism. J. Neurodev. Disord. 1, 81–90.
Jenkinson, M., Pechaud, M., and Smith, S. (2005). “BET2: MR-based estimation of brain, skull and scalp surfaces,” in Eleventh Annual Meeting of the Organization for Human Brain Mapping, Toronto, CA.
Johnson, G. A., Badea, A., Brandenburg, J., Cofer, G., Fubara, B., Liu, S., and Nissanov, J. (2010). Waxholm space: an image-based reference for coordinating mouse brain research. Neuroimage 53, 365–372.
Joshi, S., Davis, B., Jomier, M., and Gerig, G. (2004). Unbiased diffeomorphic atlas construction for computational anatomy. Neuroimage 23, S151–S160.
Kennedy, D., Haselgrove, C., and McInerney, S. (2003). MRI-based morphometric of typical and atypical brain development. Ment. Retard. Dev. Disabil. Res. Rev. 9, 155–160.
Lee, F. K. H., Fang, M., Antonio, G. E., Yeung, D. K. W., Chan, E. T. Y., Zhang, L., Yew, D. T., and Ahuja, A. T. (2006). Dti of rodent brains in vivo using a 1.5t clinical MR scanner. J. Magn. Reson. Imaging 23, 747–751.
Lee, J., Jomier, J., Aylward, S., Tyszka, M., Moy, S., Lauder, J., and Styner, M. (2009). Evaluation of atlas based mouse brain segmentation. Proc. SPIE 7259, 137–148.
Lee, J., Ehlers, C., Crews, F., Niethammer, M., Budin, F., Paniagua, B., Sulik, K., Johns, J., Styner, M., and Oguz, I. (2011). “Automatic cortical thickness analysis on rodent brain,” in SPIE Medical Imaging: Image Processing, Orlando, FL, 7962, 7962481–79624811.
Lerch, J., Carroll, J., Dorr, A., Spring, S., Evans, A., Hayden, M., Sled, J., and Henkelman, R. (2008). Cortical thickness measured from MRI in the YAC128 mouse model of Huntington’s disease. Neuroimage 41, 243–251.
Liu, Z., Wang, Y., Gerig, G., Gouttard, S., and Styner, M. (2010). “Quality control of diffusion weighted images. Medical imaging 2010: advanced PACS-based imaging informatics and therapeutic applications,” in Proceedings of SPIE, San Diego, CA.
Liu, Z., Zhu, H., Marks, B., Katz, L., Goodlett, C. B., Gerig, G., and Styner, M. (2009). “Voxel-wise group analysis of DTI,” in IEEE International Symposium on Biomedical Imaging, Orlando, FL.
MacKenzie-Graham, A., Lee, E., Dinov, I. D., Bota, M., Shattuck, D., Ruffins, S., Yuan, H., Konstantinidis, F., Pitiot, A., Ding, Y., Hu, G., Jacobs, R., and Toga, A. (2004). A multimodal, multidimensional atlas of the C57BL/6J mouse brain. J. Anat. 204, 93–102.
Marc, C., Vachet, C., Gerig, G., Blocher, J., Gilmore, J., and Styner, M. (2010). “Changes of MR and DTI appearance in early human brain development,” in SPIE Medical Imaging: Image Processing, San Diego, CA.
Mayer, D., Zahr, N. M., Adal-steinsson, E., Rutt, B., Sullivan, E. V., and Pfefferbaum, A. (2007). In vivo fiber tracking in the rat brain on a clinical 3t MRI system using a high strength insert gradient coil. Neuroimage 35, 1077–1085.
Mazziotta, J., Toga, A., Evans, A., Fox, P., Lancaster, J., Zilles, K., Woods, R., Paus, T., Simpson, G., Pike, B., Holmes, C., Collins, L., Thompson, P., MacDonald, D., Iacoboni, M., Schormann, T., Amunts, K., Palomero-Gallagher, N., Geyer, S., Parsons, L., Narr, K., Kabani, N., Le Goualher, G., Boomsma, D., Cannon, T., Kawashima, R., and Mazoyer, B. (2001). A probabilistic atlas and reference system for the human brain: International Consortium for Brain Mapping (ICBM). Philos. Trans. R. Soc. Lond. B Biol. Sci. 356, 1293–1322.
Moeller, F. G., Hasan, K. M., Steinberg, J. L., Kramer, L. A., Dougherty, D. M., Santos, R. M., Valdes, I., Swann, A. C., Barratt, E. S., and Narayana, P. A. (2005). Reduced anterior corpus callosum white matter integrity is related to increased impulsivity and reduced discriminability in cocaine-dependent subjects: diffusion tensor imaging. Neuropsychopharmacology 30, 610–617.
Mosconi, M. W., Hazlett, H., Poe, M. D., Gerig, G., Smith, R., and Piven, J. (2009). Longitudinal study of amygdala volume and joint attention in 2- to 4-year-old children with autism. Arch. Gen. Psychiatry 66, 509–516.
Nieman, B. J., Bock, N. A., Bishop, J., Chen, X. J., Sled, J. G., Rossant, J., and Henkelman, R. M. (2005). Magnetic resonance imaging for detection and analysis of mouse phenotypes. NMR Biomed. 18, 447–468.
NIMH. (2008). “Transformative neurodevelopmental research in mental illness,” in National Advisory Mental Health Council Workgroup, Bethesda, MD.
Oguz, I., Cates, J., Fletcher, T., Whitaker, R., Cool, D., Aylward, S., and Styner, M. (2008). “Cortical correspondence using entropy-based particle systems and local features,” in IEEE International Symposium on Biomedical Imaging, Paris.
Oguz, I., Lee, J., Budin, F., Rumple, A., McMurray, M., Ehlers, C., Crews, F., Johns, J., and Styner, M. (2011). “Automatic skull-stripping of rat MRI/DTI scans and atlas building,” in SPIE Medical Imaging: Image Processing, Orlando, FL, 7962, 7962251–7962257.
O’Leary-Moore, S. K., Parnell, S. E., Godin, E., Dehart, D., Ament, J., Khan, A., Johnson, G. A., Styner, M. A., and Sulik, K. (2010). Magnetic resonance microscopy-based analyses of the brains of normal and ethanol-exposed fetal mice. Birth Defects Res. A Clin. Mol. Teratol. 88, 953–964.
Paus, T., Zijdenbos, A., Worsley, K., Collins, D. L., Blumenthal, J., Giedd, J. N., Rapoport, J. L., and Evans, A. C. (1999). Structural maturation of neural pathways in children and adolescents: in vivo study. Science 283, 1908–1911.
Paxinos, G., and Franklin, K. B. J. (2001). The Mouse Brain in Stereotaxic Coordinates, 2nd Edn, San Diego: Academic Press.
Pfefferbaum, A., Adal-steinsson, E., and Sullivan, E. V. (2004). In vivo structural imaging of the rat brain with a 3-t clinical human scanner. J. Magn. Reson. Imaging 20, 779–785.
Pillai, D., Heidemann, R., Kumar, P., Shanbhag, N., Lanz, T., Dittmar, M., Sandner, B., Beier, C., Weidner, N., Greenlee, M., Schuierer, G., Bogdahn, U., and Schlachetzki, F. (2011). Comprehensive small animal imaging strategies on a clinical 3 T dedicated head MR-scanner; adapted methods and sequence protocols in cns pathologies. PLoS ONE 6, e16091. doi: 10.1371/journal.pone.0016091
Prastawa, M., Gilmore, J. H., Lin, W., and Gerig, G. (2005). Automatic segmentation of MR images of the developing newborn brain. Med. Image Anal. 9, 457–466.
Schlaepfer, T. E., Lancaster, E., Heidbreder, R., Strain, E. C., Kosel, M., Fisch, H. U., and Pearlson, G. D. (2005). Decreased frontal white-matter volume in chronic substance abuse. J. Neuropsychopharmacol. 9, 1–7.
Shaw, P., Kabani, N., Lerch, J. P., Eckstrand, K., Lenroot, R., Gogtay, N., Greenstein, D., Clasen, L., Evans, A., Rapoport, J. L., Giedd, J., and Wise, S. (2008). Neurodevelopmental trajectories of the human cerebral cortex. J. Neurosci. 28, 3586–3594.
Styner, M. A., Joshi, S., Overstreet, D. H., Mc Murray, M. S., and Johns, J. M. (2009). “Unbiased population atlas building of early postnatal rat development at PND 5 and 14 for atlas based analysis,” in Annual Meeting of the Society for Neuroscience, Chicago.
Vachet, C., Hazlett, H. C., Niethammer, M., Oguz, I., Cates, J., Whitaker, R., Piven, J., and Styner, M. (2011). “Group-wise automatic mesh-based analysis of cortical thickness,” in Medical Imaging 2011: Image Processing, 796227.
Van Leemput, K., Maes, F., Vandermeulen, D., and Suetens, P. (1999). Automated model-based tissue classification of MR images of the brain. IEEE Trans. Med. Imaging 18, 897–908.
VanCamp, N., Blockx, I., Verhoye, M., Casteels, C., Coun, F., Leemans, A., Sijbers, J., Baekelandt, V., Van Laere, K., and Van der Linden, A. (2009). Dti in a rat model of Parkinson’s disease after lesioning of the nigrostriatal tract. NMR Biomed. 22, 697–706.
Watson, R. E., DeSesso, J. M., Hurtt, M. E., and Cappon, G. D. (2006). Postnatal growth and morphological development of the brain: a species comparison. Birth Defects Res. B Dev. Reprod. Toxicol. 77, 471–484.
Yushkevich, P. A., Piven, J., Hazlett, H. C., Smith, R. G., Ho, S., Gee, J. C., and Gerig, G. (2006). User-guided 3D active contour segmentation of anatomical structures: significantly im-proved efficiency and reliability. Neuroimage 31, 1116–1128.
Keywords: small animal imaging, neonatal neuroimaging, drug abuse, brain segmentation, white matter pathways, magnetic resonance imaging, diffusion tensor imaging
Citation: Gerig G, Oguz I, Gouttard S, Lee J, An H, Lin W, McMurray M, Grewen K, Johns J and Styner MA (2011) Synergy of image analysis for animal and human neuroimaging supports translational research on drug abuse. Front. Psychiatry 2:53. doi: 10.3389/fpsyt.2011.00053
Received: 18 February 2011;
Accepted: 11 September 2011;
Published online: 03 October 2011.
Edited by:
Linda Mayes, Yale University, USACopyright: © 2011 Gerig, Oguz, Gouttard, Lee, An, Lin, McMurray, Grewen, Johns and Styner. This is an open-access article subject to a non-exclusive license between the authors and Frontiers Media SA, which permits use, distribution and reproduction in other forums, provided the original authors and source are credited and other Frontiers conditions are complied with.
*Correspondence: Martin Andreas Styner, Neuro Image Research and Analysis Laboratory, Department of Psychiatry, University of North Carolina, Chapel Hill, NC 27599-7160, USA. e-mail: styner@cs.unc.edu