- 1 Department of Psychiatry, University of Texas Southwestern Medical Center, Dallas, TX, USA
- 2 Department of Psychiatry and Behavioral Science and the Center for Molecular and Behavioral Neuroscience, Meharry Medical College, Nashville, TN, USA
- 3 Advanced Imaging Research Center, University of Texas Southwestern Medical Center, Dallas, TX, USA
- 4 Division of Biostatistics, Department of Clinical Sciences, University of Texas Southwestern Medical Center, Dallas, TX, USA
- 5 Department of Family and Community Medicine, University of Texas Southwestern Medical Center, Dallas, TX, USA
Identifying biosignatures to assess the probability of response to an antidepressant for patients with major depressive disorder (MDD) is critically needed. Functional connectivity MRI (fcMRI) offers the promise to provide such a measure. Previous work with fcMRI demonstrated that the correlation in signal from one region to another is a measure of functional connectivity. In this pilot work, a baseline non-task fcMRI was acquired in 14 adults with MDD who were free of all medications. Participants were then treated for 8 weeks with an antidepressant and then clinically re-evaluated. Probabilistic anatomic regions of interest (ROI) were defined for 16 brain regions (eight for each hemisphere) previously identified as being important in mood disorders. These ROIs were used to determine mean time courses for each individual’s baseline non-task fcMRI. The correlations in time courses between 16 brain regions were calculated. These calculated correlations were considered to signify measures of functional connectivity. The degree of connectivity for each participant was correlated with treatment outcome. Among 13 participants with 8 weeks follow-up data, connectivity measures in several regions, especially the subcallosal cortex, were highly correlated with treatment outcome. These connectivity measures could provide a means to evaluate how likely a patient is to respond to an antidepressant treatment. Further work using larger samples is required to confirm these findings and to assess if measures of functional connectivity can be used to predict differential outcomes between antidepressant treatments.
Introduction
Major depressive disorder (MDD) is a serious brain syndrome that causes considerable morbidity and mortality. There are a number of treatments available for MDD, but no single treatment works for everyone. In addition, patients not responding to the first antidepressant may respond to a second antidepressant (Rush et al., 2006c; Trivedi et al., 2006a,b). Clinicians currently have no way to determine which treatment option, if any, is most likely to get their patient better. Therefore, a method to identify brain biomarkers that predict treatment response is critically needed. This has been proposed to signify a patient’s phronotype (Kozel, 2010).
Functional neuroimaging has been used in various ways to investigate treatment response in MDD (Dougherty and Rauch, 2007). Most studies have looked at group differences at baseline and/or after treatment with a focus on regional differences in the level of brain activity. A growing body of literature, however, supports the concept that psychiatric illnesses are the result of neuropathology at the level of the brain circuit and not just regional differences (Andreasen, 1997; Phillips et al., 2003; Ressler and Mayberg, 2007; Taylor and Liberzon, 2007). This neuropathology can be revealed as altered connectivity between regions of the brain. Functional connectivity between brain regions can be assessed using functional connectivity MRI (fcMRI) during non-task (resting) periods (Biswal et al., 1995, 1997; Lowe et al., 1998; Xiong et al., 1999; Cordes et al., 2000; Greicius et al., 2003; Peltier et al., 2003; Beckmann et al., 2005). Alterations in connectivity, as measured by fcMRI, have been used to study a number of brain illnesses including MDD (Irwin et al., 2004; Anand et al., 2005a; Greicius et al., 2007; Siegle et al., 2007). In addition, the effect of antidepressants on brain connectivity measures for patients with MDD has been investigated in a few studies (Anand et al., 2005b, 2007). Although measures of regional brain connectivity have been used in several studies to investigate MDD, to the best of our knowledge, brain connectivity measures that were determined from non-task (resting) fcMRI to predict treatment outcome for an individual have not been reported.
In this study, non-task BOLD fcMRI was used to identify potential biological markers of treatment outcomes in patients with MDD. A critically important aspect of this study was the focus on the neurobiological markers at the individual level. For a clinical diagnostic test to be meaningful, the measured value must be valid for an individual patient and not require a group of patients to determine the value (Kozel and Trivedi, 2007). This individually measured value then needs to be tested in a group of participants to determine if the biomarker has clinical meaning (e.g., the blood pressure of an individual patient is measured in a group of patients to determine if the biomarker has clinical significance). In this initial study, connectivity values between brain regions were measured by determining the correlation between time courses of the various brain regions for each individual. These functional connectivity measures of the various brain regions were assessed to determine the most robust markers associated with treatment outcome.
Materials and Methods
Overview
Participants with MDD were screened, clinically evaluated, and underwent MRI scanning. Immediately after the scan, they were started on an antidepressant medication outside the study. The participant’s clinical physician or collaborating study protocol determined the type of medication and dosing. After 8 weeks of antidepressant medication, the participants were again clinically evaluated to assess for changes in depressive symptoms.
Participants
Adult males and females of all races were recruited from the community using fliers, electronic message boards, and collaborating physicians in primary care. In addition, participants were recruited from other ongoing clinical treatment studies at the University of Texas Southwestern Medical Center. Inclusion criteria included the ability to provide informed consent; a diagnosis of non-psychotic MDD; a 17-item Hamilton Rating Scale for Depression (HRSD-17; Hamilton, 1960) score greater than or equal to 14; age 18–50 years; be cognitively intact; and the ability to read, speak, and understand English. Exclusion criteria included a history of psychiatric illness except MDD, Generalized Anxiety Disorder, Social Phobia, or Specific Phobia. History of DSM-IV defined alcohol or substance abuse was allowed as long as diagnostic criteria were not met in the last 6 months; while for alcohol or substance dependence the criteria could not have been present within the last year. The greater time required for dependence versus abuse was due to the presumed greater impact on brain function of substance dependence versus abuse. Other exclusion criteria included clinically unsafe to participate in a research trial, diagnosis of an organic brain disease, serious unstable medical illness, history of serious head injury, unsafe or unable to have an MRI, previous inability to tolerate MRI, taking medication(s) with presumed effects on cerebral blood flow within 2 weeks or five half-lives – whichever is longer, intake of other medications within five half-lives, women who are pregnant or breast feeding, or women of child-bearing potential who are not using an acceptable form of birth control.
Study Procedures
The study protocol was approved by the Institutional Review Board at the University of Texas Southwestern Medical Center. For those interested in participating in the study, an initial brief telephone screen was performed. Those who passed the phone screen were scheduled for Visit 1.
Visit 1 – screen and clinical assessment
Written informed consent was obtained prior to performing any study procedures. Participants completed self-reports regarding demographic information, prior antidepressant medication history, an Annett handedness scale (Annett, 1970), the Quick Inventory of Depressive Symptomatology: Self-Rated (QIDS-SR; Rush et al., 2003, 2006b; Trivedi et al., 2004), and an MRI safety form. A trained and certified clinical rater completed the HRSD-17 (Hamilton, 1960), the clinician-rated Inventory of Depressive Symptomatology (IDS-C; Rush et al., 1996; Trivedi et al., 2004), the Hamilton Anxiety Rating Scale (HARS; Hamilton, 1959), and the Mini Mental State Evaluation (MMSE; Folstein et al., 1975). A physician performed the Structured Clinical Interview for DSM-IV (SCID) Axis I Disorders (First et al., 1995), a medical history, and a physical exam. Also, urine was obtained to screen for drugs of abuse as well as to test females of child-bearing potential for pregnancy. For those who passed the screening phase, Visit 2 was scheduled.
Visit 2 – MRI
Subjects were again screened to ensure that they were safe to enter the MRI environment and subsequently underwent MRI scanning. Once subjects completed the scanning portion of the study, they immediately started an antidepressant medication as part of other collaborative studies or clinical care.
Visit 3 – clinical assessment
After 8 weeks of treatment outside of the study, subjects presented for Visit 3 in order to be evaluated for clinical response to treatment. The IDS-C, QIDS-SR, HRSD, HARS, and antidepressant treatment history over the preceding 8 weeks were obtained.
Treatment Outcome
The a priori primary treatment outcome was defined as the percent change in the QIDS-SR from baseline to week 8 (Trivedi et al., 2004; Rush et al., 2006a,b). The QIDS-SR was chosen as the primary outcome measure because of its ability to be easily integrated into clinical practice and established psychometric properties (Trivedi et al., 2004; Rush et al., 2006a,b).
MRI Scanning
Participants were placed in the MRI with hearing protection and foam padding around their head in order to reduce movement during the scanning. Images were acquired using a research-dedicated 3T Philips Achieva scanner (Philips Medical System, Netherlands) with an eight-channel SENSE head coil. A high-resolution T1-weighted structural scan was obtained using a 3D MPRAGE sequence with one hundred sixty 1-mm slices and a 256 × 256 matrix. Participants were then instructed to hold still, keep their eyes open, and focus on a cross in the middle of the screen. The echo-planar imaging non-task fcMRI scan was obtained with the parameters of TR 2000 ms, 44 slices, 3 mm slices, FOV 220 × 132 × 220, matrix 64 × 64, with resulting voxel dimension of 3.4375 mm ×3 mm × 3.4375 mm. There were 240 time points with a total scan time of 502 s (8 min 22 s) that included dummy and saturation scans.
MRI Analysis to Measure Functional Connectivity
The non-task EPI and structural images were exported from the Philips workstation and converted to NIfTI format using dcm2nii1. Using FMRIB Software Library (FSL; Jenkinson et al., 2002; Smith et al., 2004) 4.1.22, the structural images were reoriented to the standard position using fslswapdim and then skull stripped using BET2 (Smith, 2002). Using FEAT (Jenkinson et al., 2002; Smith et al., 2004) in FSL, the non-task fcMRI data were then motion corrected, spatial smoothed with 6 mm, high-pass filtered with setting of 100 s, registered using the participant’s structural image (6 DOF) and then to the MNI152_T1_2mm_brain (using 12 DOF) using non-linear normalization. These preprocessed functional data were used to determine the mean time courses for the various structures.
To create the brain masks of the 16 regions of interest (ROI masks), standardized templates available in FSL were used. The subcortical structures were obtained by thresholding the structures in the Harvard–Oxford subcortical atlas by 60% using the fslmaths function. The cortical structures were obtained by thresholding the structures in the Harvard–Oxford cortical atlas by 60% using the fslmaths function as well. The cortical masks were then split between left and right. The masks were then converted to the same dimensions as the preprocessed fcMRI data using FLIRT (Jenkinson et al., 2002) with a 7-DOF global rescale. Using the preprocessed fcMRI data as the reference and the standard MNI152_T1_2mm_brain as the input, the 16 masks for each individual were then shadow-registered. The positioning of the resulting masks on the preprocessed fcMRI data was visually confirmed to be reasonable. The resulting masks for the left and right hemispheres included the amygdala, the hippocampus, the anterior cingulate gyrus, the posterior cingulate gyrus, the medial frontal cortex, the orbitofrontal cortex, the middle frontal cortex, and the subcallosal cortex (Fitzgerald et al., 2008).
Using the created ROI masks and the preprocessed fcMRI data, mean time courses for the 16 regions of the brain were determined using the fslmeants function in FSL. The resulting time courses of each structure for each participant were entered into SAS, version 9.2 (Statistical Analysis Systems Institute, Cary, NC, USA). Measures of connectivity were then determined by estimating the Spearman rank-order correlation coefficient between the time course of all ROIs for each individual (120 measures of connectivity were estimated in total). Thus the level of assessment (i.e., connectivity value as determined by correlation coefficient) was based on the individual’s results, not a group of individuals.
Although all participants were aware that they were receiving active treatment, the extent of treatment response was variable. This corresponds to a clinical situation. The focus of this study was to investigate whether brain biomarkers could be predictive treatment outcome and not whether the participants would respond to a particular treatment. Therefore, the investigators were blind to degree of treatment response while calculating brain region connectivity values.
Data Analysis
Brain region functional connectivity related to treatment outcome
A simple correlation analysis (using the Spearman rank-order correlation coefficient) was conducted to assess the relationship between each of the 120 pre-treatment (baseline) brain region connectivity measures and the percent change in QIDS-SR (treatment outcome). The main purpose of using Spearman’s rho (i.e., at the individual level and group level) was to provide resistant (robust) estimates in the presence of any potential outliers detected in the sample data (with any slight departure from normality). Thus for an individual participant, connectivity measures from BOLD signal time courses between two ROIs were robust. Additionally at the group level, determinations of correlation between connectivity measures and treatment outcome were robust as well using Spearman’s rho.
We performed all of the statistical analyses using SAS software, version 9.2. The level of significance for all tests was set at α = 0.05 (two-tailed) and, because of the exploratory nature of this pilot study, p-values were left unadjusted for multiple testing.
Results
Participants
Seventeen adults (14 females) with mean age of 35.3 years (SD 7.5, range 22–48 years) were enrolled. There were 10 Caucasians, 5 African-Americans, and 2 Hispanic participants. Two subjects failed the screening and one failed to show for the MRI visit. There with 14 subjects scanned with 13 having follow-up clinical evaluations at 8 weeks. The one participant with no follow-up data moved from the area after the scan and decided to discontinue from the study. Of the 13 participants (11 right handed, 10 female, mean age 33.7, SD 7.4, range 22–48 years) with follow-up data, 10 took bupropion SR 150 mg twice a day as part of a clinical trial, two took escitalopram 20 mg once a day, and one took aripiprazole 5 mg once a day. Seven of the 13 who were evaluated at 8 weeks met criteria for response (greater than or equal to 50% improvement in the QIDS-SR from baseline to week 8).
Baseline Functional Connectivity Related to Treatment Outcome
The degree of connectivity between several regions was robustly related to treatment outcome (percent change in QIDS-SR; see Table 1). The connectivity between left subcallosal cortex with the left anterior cingulate cortex (see Figure 1) demonstrated the strongest correlation; the magnitude of negative correlation between subcallosal cortex and the anterior cingulate cortex was associated with the degree of treatment response (see Figure 2). Interestingly, of the 15 most significant correlations between structures (of 120 possible), 11 involve the subcallosal cortex (six left, five right hemisphere). Thus, the connectivity measures from the baseline fcMRI scans provide an objective measure that is related to treatment outcome and possibly predictive. As an example, choosing a connectivity value of less than 0.1 for the left subcallosal cortex to the left anterior cingulate as a predictor of treatment response, 11 of the 13 participants (85% accuracy) would have had their treatment outcome correctly ascertained prior to treatment. A one-sample test here of the binomial proportion (H0: Proportion = 0.5) revealed rejection of the null hypothesis (two-sided exact test, p = 0.02).
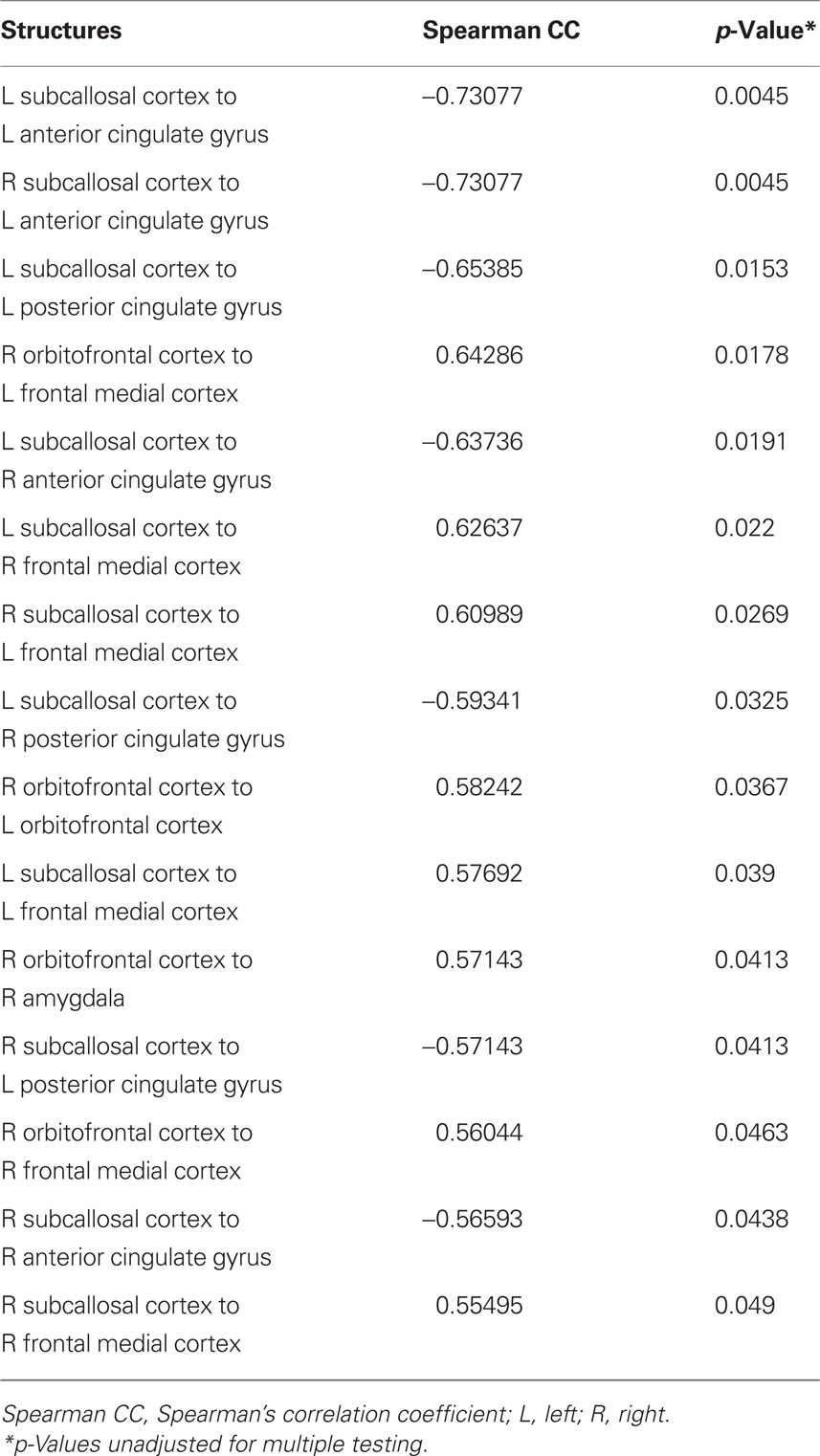
Table 1. Spearman correlation coefficients between brain region connectivity measures and the percent change in QIDS-SR (treatment outcome).
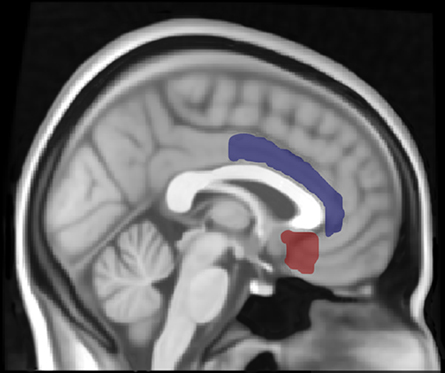
Figure 1. Location of left subcallosal cortex (red) and left anterior cingulate gyrus (blue) regions of interest (ROI). The thresholded ROIs are displayed using Mricron with the structural T1-weighted Montreal Neurological Institute (MNI) 1 mm template in FSL.
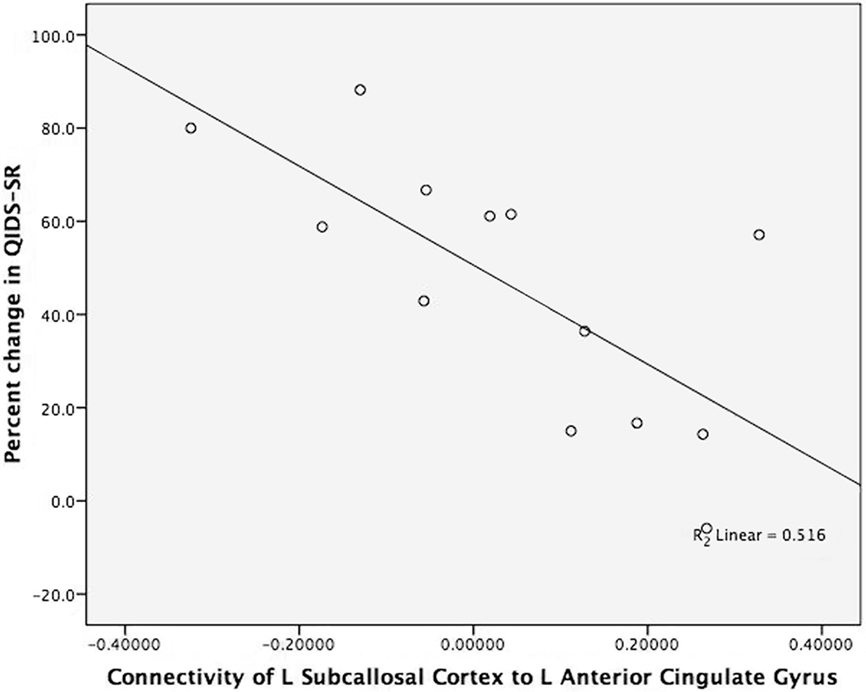
Figure 2. Brain connectivity and antidepressant response. The figure displays the strong negative correlation between connectivity of the left subcallosal cortex to the left anterior cingulate gyrus and treatment outcome (percent change in QIDS-SR). The x-axis is the brain connectivity for the left subcallosal cortex (L_SC) to left anterior cingulate gyrus (L_ACG). The y-axis is the percent change in QIDS-SR score from baseline to 8 weeks of treatment (percent change in QIDS-SR). The two participants’ coordinates for L_SC to L ACG who took escitalopram are (−0.130, 88.2) and (0.188, 16.7). The participant’s coordinates for L_SC to L_ACG who took aripiprazole are (0.019, 61.1).
Discussion
This study demonstrates the potential to use functional connectivity measures derived from non-task fcMRI scans in order to provide critically needed assistance to clinicians. The connectivity of both subcallosal cortices to the left anterior cingulate gyrus were strongly correlated with treatment outcome. The preponderance of subcallosal cortex connectivity values that strongly correlated with treatment outcome demonstrated a non-random pattern. This suggests a critical role for the subcallosal cortex’s connectivity in treatment outcome. This is especially interesting as the subcallosal cortical region has been implicated in antidepressant treatment response for medications (Mayberg et al., 1997; Kennedy et al., 2001, 2007), cognitive behavioral therapy (Siegle et al., 2006; Kennedy et al., 2007), cingulotomy (Dougherty et al., 2003), and transcranial magnetic stimulation (Teneback et al., 1999).
Using this connectivity approach has a number of advantages when developing a clinically useful diagnostic test (Fox and Greicius, 2010). The technology of fcMRI is widely available and can be tolerated by the majority of people. The test provides an objective measure that does not rely on patient effort, reading a particular language, or any task. Because the MRI scanner can acquire multiple other forms of information (structural, perfusion, diffusion tensor imaging for structural connectivity, spectroscopy, etc.), future developments may involve acquiring information simultaneously from diverse methods and integrating this information into a treatment predictive model that is more robust.
The development of objective measures to help guide treatment decisions could also provide important insights into the neurobiology of mood disorders and patients’ response to treatment. This knowledge could guide treatment development and move the field toward a more individualized approach to treatment.
Although this study demonstrates the potential of this technique to provide an objective measure at the individual level to predict treatment outcome in MDD, further work is clearly required to determine whether the technique can provide clinically useful information. A much broader sample of participants with mood disorders will need to be tested using models that are defined a priori. Various treatment modalities will need to be tested which would offer a unique opportunity to develop treatment algorithms based on objective measures. The ultimate goal is to develop diagnostic models that improve clinical outcome. As such, these models should be tested to assess whether they improve treatment outcome.
In addition to a broader sample of the population, future studies should address if changes in the analysis method could improve treatment prediction. The choice of ROIs was based on the need for a clinical diagnostic test to have a standard methodology that could be incorporated into routine practice. The ROIs chosen encompassed regions that were potentially functionally distinct as well as containing different tissue types such as gray and white matter. Whether refining these ROIs would improve treatment prediction is something that should be empirically tested in future studies. Also, factoring out certain signals (e.g., global brain changes) in the time course may improve the treatment prediction as well. These changes would also need to be tested empirically, however, because they may degrade the signal in an unexpected way that actually would reduce the clinical utility of the measure. The important endpoint to use in assessing any changes would need to be impact on treatment prediction and not theoretical considerations (Kozel, 2010).
Several limitations in the study design should be considered when interpreting these data. First, the sample size is small. Although the sample size does bring the generalizability of these findings into question, it does demonstrate that the effect seen – if real – was robust. This is important because the magnitude of these effects make them more plausible choices as clinically relevant treatment predictors. The limited number and non-uniformity of treatments that were assessed may have introduced some confounds that we are not able to predict. The lack of a placebo arm and randomization makes it difficult to tease apart whether the results were related directly to the antidepressant treatment or as a function of non-specific clinical improvement over time. Finally, to identify whether structures’ connectivity values were significantly correlated with treatment outcome, a statistical threshold of p < 0.05 unadjusted for multiple testing was used. The rationale for using an unadjusted p-value was that the focus of this pilot study was to identify brain structures that could be incorporated into future research. This more definitive research would develop brain-based models of treatment response using specific a priori defined regions. Thus, the important test of significance ultimately is not whether particular regions are significantly correlated to each other, but whether the future models built on the functional connectivity values can improve treatment prediction and clinical outcome.
In conclusion, objective measures of connectivity derived from non-task fcMRI scans could provide critically needed assistance to clinicians. Developing these objective measures would significantly advance the field of psychiatry and potentially lead to novel treatment development. Future work is required to test the benefit of this approach.
Conflict of Interest Statement
F. Andrew Kozel, M.D., M.S.C.R.
Past Research and/or Salary Support: The National Institute of Mental Health K23 NIMH 5 K23 MH070897-02, Role: PI NIH/NCRR 5 UL1 RR024982-02 Packer (PI) Role: Pilot Study PI; Neuronetics Grant-in-kind support for supplies and use of equipment; the Defense Academy for Credibility Assessment (formerly the Department of Defense Polygraph Institute), Cephos Corp., Stanley Medical Research Institute, Cyberonics (Treatment studies D01, D02, D04, AN01) 2001–2005; Glaxo Smith Kline (Interleaved TMS–fMRI) 2002–2003.
Paid Advisory/Consulting: None.
Speaking: None.
Equity Holdings (exclude mutual funds): None.
Royalty/patent, other income: patents pending as an inventor through the Medical University of South Carolina on fMRI Detection of Deception, Guided rTMS Inhibition of Deception, Optimizing VNS dose with rTMS.
Other: 2004 Monthly Case Discussion Group Leader x1 Sponsored by Astra Zeneca; Unpaid scientific consultant to Cephos Corp.
Uma Rao, M.D.
Present Research Support: The National Institutes of Health (RO1 DA17805, RO1 MH68391), and Brain and Behavior Research Endowed Chair at Meharry Medical College.
Past Research Support: The National Institutes of Health (RO1 DA14037, RO1 DA15131, RO1 DA17804, RO1 MH62424), and Sarah M. and Charles E. Seay Endowed Chair in Child Psychiatry at UT Southwestern Medical Center.
Paid Advisory/Consulting: None.
Speaking: None.
Equity Holdings (exclude mutual funds): None.
Royalty/Patent, Other Income: None.
Other: None.
Hanzhang Lu, Ph.D.
Present Research support: National Institute of Mental Health R01 MH084021-02, Role: PI.
Past Research and/or Salary Support: National Institute of Neurological Disorder and Stroke R21 NS054916.
Paid Advisory/Consulting: None.
Speaking: None.
Equity Holdings (exclude mutual funds): None.
Royalty/patent, other income: None.
Other: None.
Paul A. Nakonezny, Ph.D.
Dr. Nakonezny has no disclosure to report or conflict of interest that could have inappropriately influenced, or be perceived to influence, this work.
Bruce Grannemann, M.A.
Present Research support: National Institutes of Health (1R18 HS017189-01), PI-Trivedi: Using Information Technology to Provide Measurement Based Care for Chronic Illness. Role: Data Management and Statistical Analysis.
Past Research and/or Salary Support: National Institute of Mental Health (1-R01-MH067692-01), PI-Trivedi. Treatment with Exercise Augmentation for Depression (TREAD). Role: Data Management and Statistical Analysis.
National Institute of Mental Health (R01MH064062-01A2), PI-Trivedi: Computerized Decision Support System for Depression. Role: Data Management and Statistics Analysis.
Paid Advisory/Consulting: None.
Speaking: None.
Equity Holdings (exclude mutual funds): None.
Royalty/Patent, other income: None.
Tamara McGregor, M.D.
Dr. McGregor has no disclosures or conflicts of interest to report.
Paul E. Croarkin, M.D.
Dr. Croarkin receives research and training support from Grant Number UL1RR024982, titled, “North and Central Texas Clinical and Translational Science Initiative” (Milton Packer, M.D., PI) from the National Center for Research Resources (NCRR), a component of the National Institutes of Health (NIH), and has received a Neuronetics grant-in-kind for supplies and use of equipment. He has received research support from the Stanley Medical Research Institute and the National Alliance for Research of Schizophrenia and Depression. Dr. Croarkin has also received salary support for research from Biobehavioral Diagnostics Inc. and Somerset Inc.
Kimberly S. Mapes, B.A.
Ms Mapes has no disclosures or conflicts of interest to report.
Carol Tamminga, M.D.
(Past year; 04/2010)
• Acadia Pharmaceuticals, Inc. – Advisory Board, drug development <$10,000
• Intracellular Therapies (ITI, Inc.) – Advisory Board, drug development >$10,000
• Lundbeck, Inc. – Ad hoc consultant and speaker >$10,000
• International Congress on Schizophrenia Research – Organizer; Unpaid volunteer
• American Psychiatric Association – Deputy Editor >$10,000
• Finnegan Henderson Farabow Garrett and Dunner, LLP – Expert Witness >$10,000
• PureTech Ventures – <$10,000
Madhukar H. Trivedi, M.D.
Madhukar H. Trivedi, M.D. is a consultant to or on speaker bureaus for Abbott Laboratories, Inc., Abdi Ibrahim, Akzo (Organon Pharmaceuticals Inc.), AstraZeneca, Bristol–Myers Squibb Company, Cephalon, Inc., Cyberonics Inc., Eli Lilly and Company, Evotec, Fabre Kramer Pharmaceuticals, Inc., Forest Pharmaceuticals, GlaxoSmithKline, Janssen Pharmaceutica Products, LP, Johnson & Johnson PRD, Meade Johnson, Medtronic, Neuronetics, Otsuka Pharmaceuticals, Parke–Davis Pharmaceuticals, Inc., Pfizer Inc., Sepracor, SHIRE Development, Solvay Pharmaceuticals, VantagePoint, and Wyeth-Ayerst Laboratories. He receives research support from the Agency for Healthcare Research and Quality (AHRQ), Corcept Therapeutics, Inc., Cyberonics, Inc., Merck, National Alliance for Research in Schizophrenia and Depression, National Institute of Mental Health, National Institute on Drug Abuse, Novartis, Pharmacia and Upjohn, Predix Pharmaceuticals (Epix), Solvay Pharmaceuticals, Inc., and Targacept.
Acknowledgments
This project was supported with pilot study funding to Dr. Kozel as part of the “North and Central Texas Clinical and Translational Science Initiative” grant number UL1RR024982 (Milton Packer, M.D., PI) from the National Center for Research Resources (NCRR), a component of the National Institutes of Health (NIH) and NIH Roadmap for Medical Research. Dr. Kozel was supported by a Mentored Patient-Oriented Research Career Development Award grant number 5K23MH070897 (F. Andrew Kozel, PI) from the National Institute of Mental Health (NIMH). Dr. Lu was supported by NIH grant R01 MH084021. The authors acknowledge the editorial support of Ms Jeanne McCurdy. The views expressed in this article are those of the authors and do not necessarily reflect the official policy or position of the NIMH, the NCRR, the NIH, or the U.S. Government.
Footnotes
References
Anand, A., Li, Y., Wang, Y., Gardner, K., and Lowe, M. J. (2007). Reciprocal effects of antidepressant treatment on activity and connectivity of the mood regulating circuit: an FMRI study. J. Neuropsychiatry Clin. Neurosci. 19, 274–282.
Anand, A., Li, Y., Wang, Y., Wu, J., Gao, S., Bukhari, L., Mathews, V. P., Kalnin, A., and Lowe, M. J. (2005a). Activity and connectivity of brain mood regulating circuit in depression: a functional magnetic resonance study. Biol. Psychiatry 57, 1079–1088.
Anand, A., Li, Y., Wang, Y., Wu, J., Gao, S., Bukhari, L., Mathews, V. P., Kalnin, A., and Lowe, M. J. (2005b). Antidepressant effect on connectivity of the mood-regulating circuit: an FMRI study. Neuropsychopharmacology 30, 1334–1344.
Andreasen, N. C. (1997). Linking mind and brain in the study of mental illnesses: a project for a scientific psychopathology. Science 275, 1586–1593.
Annett, M. (1970). A classification of hand preference by association analysis. Br. J. Psychol. 61, 303–321.
Beckmann, C. F., DeLuca, M., Devlin, J. T., and Smith, S. M. (2005). Investigations into resting-state connectivity using independent component analysis. Philos. Trans. R. Soc. Lond. B Biol. Sci. 360, 1001–1013.
Biswal, B., Yetkin, F. Z., Haughton, V. M., and Hyde, J. S. (1995). Functional connectivity in the motor cortex of resting human brain using echo-planar MRI. Magn. Reson. Med. 34, 537–541.
Biswal, B. B., Van Kylen, J., and Hyde, J. S. (1997). Simultaneous assessment of flow and BOLD signals in resting-state functional connectivity maps. NMR Biomed. 10, 165–170.
Cordes, D., Haughton, V. M., Arfanakis, K., Wendt, G. J., Turski, P. A., Moritz, C. H., Quigley, M. A., and Meyerand, M. E. (2000). Mapping functionally related regions of brain with functional connectivity MR imaging. AJNR Am. J. Neuroradiol. 21, 1636–1644.
Dougherty, D. D., and Rauch, S. L. (2007). Brain correlates of antidepressant treatment outcome from neuroimaging studies in depression. Psychiatr. Clin. North Am. 30, 91–103.
Dougherty, D. D., Weiss, A. P., Cosgrove, G. R., Alpert, N. M., Cassem, E. H., Nierenberg, A. A., Price, B. H., Mayberg, H. S., Fischman, A. J., and Rauch, S. L. (2003). Cerebral metabolic correlates as potential predictors of response to anterior cingulotomy for treatment of major depression. J. Neurosurg. 99, 1010–1017.
First, M., Spitzer, R., Williams, J., and Gibbon, M. (1995). Structured Clinical Interview for DSM-IV (SCID). Washington, DC: American Psychiatric Press.
Fitzgerald, P. B., Laird, A. R., Maller, J., and Daskalakis, Z. J. (2008). A meta-analytic study of changes in brain activation in depression. Hum. Brain Mapp. 29, 683–695.
Folstein, M. F., Folstein, S. E., and McHugh, P. R. (1975). “Mini-mental State.” A practical method for grading the cognitive state of patients for the clinician. J. Psychiatr. Res. 12, 189–198.
Fox, M. D., and Greicius, M. (2010). Clinical applications of resting state functional connectivity. Front. Syst. Neurosci. 4:19. doi: 10.3389/fnsys.2010.00019
Greicius, M. D., Flores, B. H., Menon, V., Glover, G. H., Solvason, H. B., Kenna, H., Reiss, A. L., and Schatzberg, A. F. (2007). Resting-state functional connectivity in major depression: abnormally increased contributions from subgenual cingulate cortex and thalamus. Biol. Psychiatry 62, 429–437.
Greicius, M. D., Krasnow, B., Reiss, A. L., and Menon, V. (2003). Functional connectivity in the resting brain: a network analysis of the default mode hypothesis. Proc. Natl. Acad. Sci. U.S.A. 100, 253–258.
Irwin, W., Anderle, M. J., Abercrombie, H. C., Schaefer, S. M., Kalin, N. H., and Davidson, R. J. (2004). Amygdalar interhemispheric functional connectivity differs between the non-depressed and depressed human brain. Neuroimage 21, 674–686.
Jenkinson, M., Bannister, P., Brady, M., and Smith, S. (2002). Improved optimization for the robust and accurate linear registration and motion correction of brain images. Neuroimage 17, 825–841.
Kennedy, S. H., Evans, K. R., Kruger, S., Mayberg, H. S., Meyer, J. H., McCann, S., Arifuzzman, A. I., Houle, S., and Vaccarino, F. J. (2001). Changes in regional brain glucose metabolism measured with positron emission tomography after paroxetine treatment of major depression. Am. J. Psychiatry 158, 899–905.
Kennedy, S. H., Konarski, J. Z., Segal, Z. V., Lau, M. A., Bieling, P. J., McIntyre, R. S., and Mayberg, H. S. (2007). Differences in brain glucose metabolism between responders to CBT and venlafaxine in a 16-week randomized controlled trial. Am. J. Psychiatry 164, 778–788.
Kozel, F. A. (2010). Identifying phronotypes in psychiatry. Front. Psychiatry 1:141. doi: 10.3389/fpsyt.2010.00141
Kozel, F. A., and Trivedi, M. H. (2007). Developing a neuropsychiatric functional brain imaging test. Neurocase 14, 54–58..
Lowe, M. J., Mock, B. J., and Sorenson, J. A. (1998). Functional connectivity in single and multislice echoplanar imaging using resting-state fluctuations. Neuroimage 7, 119–132.
Mayberg, H. S., Brannan, S. K., Mahurin, R. K., Jerabek, P. A., Brickman, J. S., Tekell, J. L., Silva, J. A., McGinnis, S., Glass, T. G., Martin, C. C., and Fox, P. T. (1997). Cingulate function in depression: a potential predictor of treatment response. Neuroreport 8, 1057–1061.
Peltier, S. J., Polk, T. A., and Noll, D. C. (2003). Detecting low-frequency functional connectivity in fMRI using a self-organizing map (SOM) algorithm. Hum. Brain Mapp. 20, 220–226.
Phillips, M. L., Drevets, W. C., Rauch, S. L., and Lane, R. (2003). Neurobiology of emotion perception II: implications for major psychiatric disorders. Biol. Psychiatry 54, 515–528.
Ressler, K. J., and Mayberg, H. S. (2007). Targeting abnormal neural circuits in mood and anxiety disorders: from the laboratory to the clinic. Nat. Neurosci. 10, 1116–1124.
Rush, A. J., Bernstein, I. H., Trivedi, M. H., Carmody, T. J., Wisniewski, S., Mundt, J. C., Shores-Wilson, K., Biggs, M. M., Woo, A., Nierenberg, A. A., and Fava, M. (2006a). An evaluation of the Quick Inventory of Depressive Symptomatology and the Hamilton Rating Scale for Depression: a sequenced treatment alternatives to relieve depression trial report. Biol. Psychiatry 59, 493–501.
Rush, A. J., Carmody, T. J., Ibrahim, H. M., Trivedi, M. H., Biggs, M. M., Shores-Wilson, K., Crismon, M. L., Toprac, M. G., and Kashner, T. M. (2006b). Comparison of self-report and clinician ratings on two inventories of depressive symptomatology. Psychiatr. Serv. 57, 829–837.
Rush, A. J., Trivedi, M. H., Wisniewski, S. R., Stewart, J. W., Nierenberg, A. A., Thase, M. E., Ritz, L., Biggs, M. M., Warden, D., Luther, J. F., Shores-Wilson, K., Niederehe, G., and Fava, M. (2006c). Bupropion-SR, sertraline, or venlafaxine-XR after failure of SSRIs for depression. N. Engl. J. Med. 354, 1231–1242.
Rush, A. J., Gullion, C. M., Basco, M. R., Jarrett, R. B., and Trivedi, M. H. (1996). The Inventory of Depressive Symptomatology (IDS): psychometric properties. Psychol. Med. 26, 477–486.
Rush, A. J., Trivedi, M. H., Ibrahim, H. M., Carmody, T. J., Arnow, B., Klein, D. N., Markowitz, J. C., Ninan, P. T., Kornstein, S., Manber, R., Thase, M. E., Kocsis, J. H., and Keller, M. B. (2003). The 16-Item Quick Inventory of Depressive Symptomatology (QIDS), clinician rating (QIDS-C), and self-report (QIDS-SR): a psychometric evaluation in patients with chronic major depression. Biol. Psychiatry 54, 573–583.
Siegle, G. J., Carter, C. S., and Thase, M. E. (2006). Use of FMRI to predict recovery from unipolar depression with cognitive behavior therapy. Am. J. Psychiatry 163, 735–738.
Siegle, G. J., Thompson, W., Carter, C. S., Steinhauer, S. R., and Thase, M. E. (2007). Increased amygdala and decreased dorsolateral prefrontal BOLD responses in unipolar depression: related and independent features. Biol. Psychiatry 61, 198–209.
Smith, S. M., Jenkinson, M., Woolrich, M. W., Beckmann, C. F., Behrens, T. E., Johansen-Berg, H., Bannister, P. R., De Luca, M., Drobnjak, I., Flitney, D. E., Niazy, R. K., Saunders, J., Vickers, J., Zhang, Y., De Stefano, N., Brady, J. M., and Matthews, P. M. (2004). Advances in functional and structural MR image analysis and implementation as FSL. Neuroimage 23(Suppl. 1), S208–S219.
Taylor, S. F., and Liberzon, I. (2007). Neural correlates of emotion regulation in psychopathology. Trends Cogn. Sci. 11, 413–418.
Teneback, C. T., Nahas, Z., Speer, A. M., Molloy, M., Stallings, L. E., Spicer, K. M., Risch, S. C., and George, M. S. (1999). Changes in prefrontal cortex and paralimbic activity in depression following two weeks of daily left prefrontal TMS. J. Neuropsychiatry Clin. Neurosci. 11, 426–435.
Trivedi, M. H., Fava, M., Wisniewski, S. R., Thase, M. E., Quitkin, F., Warden, D., Ritz, L., Nierenberg, A. A., Lebowitz, B. D., Biggs, M. M., Luther, J. F., Shores-Wilson, K., and Rush, A. J. (2006a). Medication augmentation after the failure of SSRIs for depression. N. Engl. J. Med. 354, 1243–1252.
Trivedi, M. H., Rush, A. J., Wisniewski, S. R., Nierenberg, A. A., Warden, D., Ritz, L., Norquist, G., Howland, R. H., Lebowitz, B., McGrath, P. J., Shores-Wilson, K., Biggs, M. M., Balasubramani, G. K., and Fava, M. (2006b). Evaluation of outcomes with citalopram for depression using measurement-based care in STAR*D: implications for clinical practice. Am. J. Psychiatry 163, 28–40.
Trivedi, M. H., Rush, A. J., Ibrahim, H. M., Carmody, T. J., Biggs, M. M., Suppes, T., Crismon, M. L., Shores-Wilson, K., Toprac, M. G., Dennehy, E. B., Witte, B., and Kashner, T. M. (2004). The Inventory of Depressive Symptomatology, Clinician Rating (IDS-C) and Self-Report (IDS-SR), and the Quick Inventory of Depressive Symptomatology, Clinician Rating (QIDS-C) and Self-Report (QIDS-SR) in public sector patients with mood disorders: a psychometric evaluation. Psychol. Med. 34, 73–82.
Keywords: major depressive disorder, antidepressant, functional MRI, treatment prediction, connectivity, non-task fcMRI
Citation: Kozel FA, Rao U, Lu H, Nakonezny PA, Grannemann B, McGregor T, Croarkin PE, Mapes KS, Tamminga CA and Trivedi MH (2011) Functional connectivity of brain structures correlates with treatment outcome in major depressive disorder. Front. Psychiatry 2:7. doi:10.3389/fpsyt.2011.00007
Received: 22 December 2010;
Accepted: 17 February 2011;
Published online: 08 March 2011.
Edited by:
Paul Fitzgerald, Monash University, AustraliaReviewed by:
John Hart, University of Texas at Dallas, USAJerome Joseph Maller, Monash Alfred Psychiatry Research Centre, Australia
Copyright: © 2011 Kozel, Rao, Lu, Nakonezny, Grannemann, Mcgregor, Croarkin, Mapes, Tamminga and Trivedi. This is an open-access article subject to an exclusive license agreement between the authors and Frontiers Media SA, which permits unrestricted use, distribution, and reproduction in any medium, provided the original authors and source are credited.
*Correspondence: F. Andrew Kozel, Department of Psychiatry, University of Texas Southwestern Medical Center, 5323 Harry Hines Boulevard Dallas, TX 75390-9119, USA. e-mail: andrew.kozel@utsouthwestern.edu