- Department of Psychiatry, University of Texas Southwestern Medical Center, Dallas, TX, USA
Refinements in the methods of diagnosis for psychiatric disorders are critically needed. These new methods should be based on objectively measured brain characteristics that provide clinically useful information. Studying the brain with respect to psychiatric disorders, however, faces numerous challenges. Utilizing techniques learned in other areas of medicine to deal with symptoms that lead to complex disorders can provide insight into improving diagnostic models in psychiatry. Specifically, many areas of medicine use objective measures of an organ’s function or characteristic to guide clinical management of particular subjective complaints. In psychiatry, an objectively measured brain characteristic that provides clinically useful information is proposed to be that person’s “phronotype.” Important requirements to developing phronotypes are discussed. Identifying phronotypes in psychiatry will require a specific investigative approach that must be grounded in rigorous scientific methodology. Successfully developing such markers will have a profound impact on clinical care, clinical research, basic science research, and most importantly the lives of those suffering from these illnesses.
There is a critical need for new methods to diagnose psychiatric illnesses (Insel and Wang, 2009). These new methods must be based on disease models that enable clinicians to achieve better outcomes for their patients and researchers to develop more effective treatments. Thus, the purpose of these models is to improve patient outcome and that is how they should be evaluated. Our current model of diagnosis involves acquiring a group of signs and symptoms that the patient either describes or is observed experiencing. This results in a syndrome based diagnosis, but not necessarily an etiological based one. Occasionally objective measures such as laboratory testing of blood or neuroimaging are acquired, but these tests are only used to rule out other causes – not to refine the psychiatric diagnosis. If the current method of diagnosis provided a system in which illnesses could be effectively identified and treated, further refinement of the diagnostic model would not be a critical issue. Unfortunately, however, recent large scale studies have demonstrated that even in optimal treatment settings, a significant portion of our patients do not get better and/or do not stay well (Lieberman et al., 2005; Perlis et al., 2006; Rush et al., 2006). Although the current psychiatric diagnostic system was an important step in helping psychiatry move forward as a medical specialty, new models of these illnesses are necessary to advance psychiatric medicine (Nestler et al., 2002; Hyman and Fenton, 2003).
Developing a new diagnostic method or system should involve building a brain-based model of psychiatric illnesses. All models are over simplifications of reality and are thus at some level inaccurate. But much like a road map is an over simplification of a system of roads, the map is still a very useful tool with which to navigate. In a similar manner, brain based models of psychiatric illnesses do not necessarily have to capture all of the complexity of the illness in order to have value. The crucial question becomes – does the model improve the care of the patient? The current model of psychiatric illness that categorizes diagnoses by a series of signs and symptoms has been reasonably successful at enabling physicians to care for their patients. To improve our outcomes, however, a model of illness that moves beyond the syndrome level to the level of organ dysfunction is needed.
Psychiatry faces a number of significant obstacles to developing a diagnostic model based on organ dysfunction. For most of our psychiatric illnesses, there are no clear neuropathologic changes that are diagnostic even at autopsy. There are numerous reasons for this lack of knowledge, but several are important to point out. One, the brain is surrounded by bone, skin, and tissues that make evaluating the organ very difficult. Two, the function of the brain as it relates to psychiatric illnesses cannot be easily assessed like the function of the lungs, heart, or kidneys. This is partly related to the first point, but more fundamentally by the nature of the functions that the brain performs. Three, the brain is not an organ that can be ethically biopsied for routine study. Thus, the study of human brain tissue is limited to post-mortem or post-operative specimens in a very select group of patients. Both types of samples for various reasons are limited in what type of questions can be investigated. Four, attempts to identify neuropathologic markers have generally grouped patients based on syndrome diagnoses and determined what is different about the brains (e.g., structure and/or function) of a group of patients and some control group. This strategy makes the fundamental assumption that patients with similar symptoms have a similar disease (i.e., neuropathology). There is a growing body of evidence, however, that this is not likely to be the case. Also, this method does not allow assessments to be made about individuals, only groups of individuals. Five, psychiatric illnesses are very complex disorders that result from interactions of genetic, epigenetic, developmental, neuronal, environmental, and cultural factors. Six, there exists no clear animal models of many of our psychiatric diseases that encompass the full illness. Although there are animal models used to study psychiatric diseases, these often focus on some aspect of the illness that appears to be similar in humans and animals. Whether the neuropathology that result in the human and animal symptoms are similar is not known – largely because we do not have brain based models of psychiatric diseases in humans. These above factors have made the development of brain-based models of psychiatric illnesses very challenging.
Other areas of medicine (e.g., cardiology, pulmonology) have addressed the problem of disease complexity at the symptom level (e.g., chest pain, shortness of breath) by measuring the function of the organ of interest (e.g., cardiac stress test, pulmonary function test – PFT). This enables the diagnoses to be based on organ pathology. These etiologic based diagnostic systems provide measures that aid in diagnosis, a metric to assess outcome, and a means to develop a targeted approach for treatment development. A similar approach should be taken with psychiatric diseases.
As an example, take the syndrome of major depressive disorder (MDD). Currently, when a patient presents with the complaint of depressive symptoms, information is acquired regarding current and past symptoms (e.g., problems with mood, sleep, concentration, appetite, anxiety, mania, psychosis, etc.) and other history (e.g., substance use, medical problems, medications, family history, etc.). These are put together to make a syndrome diagnosis (e.g., MDD) (DSM-IV, 1994). The diagnosis is reliable in that most clinicians would make the same diagnosis, and it does provide information related to treatment (i.e., a group of patients with similar symptoms have been found to be more likely to respond to various antidepressant treatments than to placebo treatments). This has enabled clinicians to successfully treat a majority of patients with MDD. Unfortunately, for a substantial portion of patients suffering with MDD, however, their symptoms are not relieved with standard treatments. Even for those who eventually do respond, it may take multiple treatment trials resulting in prolonged morbidity before the successful treatment is chosen. Thus, a better means to categorize patients who present with depressive symptoms is needed. Again, other areas of medicine have accomplished this by measuring the functional output of the organ of interest. Similarly, patients with psychiatric diseases could be classified by some brain attribute or function. The term “phronotype” is proposed to indicate an objectively measured brain characteristic that provides clinically useful information. The term is derived from phon-o- from the Ancient Greek phontis1 meaning thought or brain, and type from the Ancient Greek typos meaning model or type. Thus, an individual’s objectively measured functional brain output that provided clinically relevant information would be that person’s phronotype.
The functional output of the brain as it relates to psychiatric diseases, however, is not easily measured. One measure is the observable characteristics of the illness or phenotype. The word is derived from the Ancient Greek phainein meaning to display and typos meaning model or type (The American Heritage Dictionary, 1982). In psychiatry, this is generally regarded as the symptoms of the illnesses and is thought to be the resulting interaction of the person’s genotype and environment. The genotype is defined as the genetic constitution of an individual. The term comes from the Greek genos meaning race plus typos meaning model or type (The American Heritage Dictionary, 1982). In an attempt to define an intermediate “type,” the term endophenotype was coined as an intermediate link between observable characteristics and genetics. Gottesman and Gould (2003) discuss the endophenotype concept for psychiatry in a very nice review. Their criteria for a characteristic or finding to be an endophenotype include: one, is associated with the illness in the population; two, is heritable; three, is state independent; four, co-segregates with illness within families; and five, is found in a higher percentage of an affected person’s family members who do not have the illness than in the general population. Thus, the focus of the endophenotype is finding a direct link between the genotype and observable behavior and not on defining a brain function or characteristic. This is in contrast to the phronotype, which is only concerned with a brain attribute (e.g., function) that provides clinical value. Defining phronotypes of current psychiatric diagnoses may or may not relate to the underlying genetics of psychiatric disorders, but that would not be their focus during development. Future studies could address the relationship between phronotypes and genotypes.
Although the distinctions between phronotype and both genotype and phenotype are relatively clear, the differentiation of a phenotype and endophenotype is less obvious. The concepts are similar in that both would be associated with the illness in the population, but there are several key differences. The fundamental difference is the heritability of the brain marker. For an endophenotype, the trait must be heritable, co-segregate with illness within families, and appear in unaffected family members at a higher percentage than the general population. Conversely, a phronotype is a functional measure that is independent of heritability. Using the PFT as an example of a functional measure of the lungs, a child with reactive airway disease (RAD) who has a PFT result indicative of obstructive airway disease may or may not have relatives with a similar result. The clinically pertinent point is that the child has the objective organ function characteristic that will guide treatment – regardless of whether his RAD was the result of genetic, environmental, or some combination of factors. Another important distinction is that an endophenotype is state independent (i.e., a trait), while a phronotype can be related to either the state or trait – as long as it provides clinically relevant information.
A classification system based on phronotypes would have radical clinical and research implications as well as provide a pathway to reach the National Institute of Mental Health’s strategic goal of personalized medicine. Following strategies from other areas of medicine offers a guide to developing phronotypes in psychiatry. As a starting point, testing differences in the function of the organ (i.e., the brain) in which the symptoms (e.g., depressive, psychotic, anxious symptoms) originate makes the most sense. The testing methodology must also be safe, able to be implemented in clinical care, and provide reliable information at the individual level. For a clinical diagnostic test to be meaningful, it must have validity at the individual level (Kozel and Trivedi, 2008). Also, in assessing the functional state of an organ, having a measure of the organ in a dynamic state generally provides the most sensitive and specific diagnostic information. One unique aspect to developing a diagnostic test in patients with psychiatric disorders is that the illness itself impacts on the patient’s level of effort in performing a test. A diagnostic test dealing with psychiatric disorders should ideally be independent of the patient’s effort.
Developing a diagnostic brain test to define phronotypes requires a specific approach. Most studies assessing brain function as it relates to psychiatric syndromes compare a group of people with a particular diagnosis and some control group – usually healthy controls. Conversely, to develop a phronotype diagnostic system, patients with a particular syndrome would have functional brain information acquired and then treated with an intervention. Functional brain differences at the individual level that distinguish those that had a particular clinical response and those that did not are determined. These findings would then need to be tested a priori on various independent groups of patients with that particular syndrome to determine how accurately the phronotype distinguished the patients as responders versus non-responders. The functional brain “marker” (if validated) would provide a phronotype diagnosis that provided more information on likelihood of response for a particular treatment than a purely syndrome based diagnosis. This is analogous to a patient presenting with the complaint of shortness of breath and a physician ordering a PFT. The result of the PFT (e.g., obstructive, restrictive, or normal pattern) enables the physician to choose a treatment or obtain additional testing to refine the diagnosis even further. Similarly, a patient with depressive symptoms could undergo a test of brain function that has been validated to predict clinical outcome. Depending on the result, a treatment would be chosen or further testing order. Thus, the focus of developing the phronotype is not to distinguish syndrome versus health, but to provide information regarding treatment choice within a syndrome (see Figure 1).
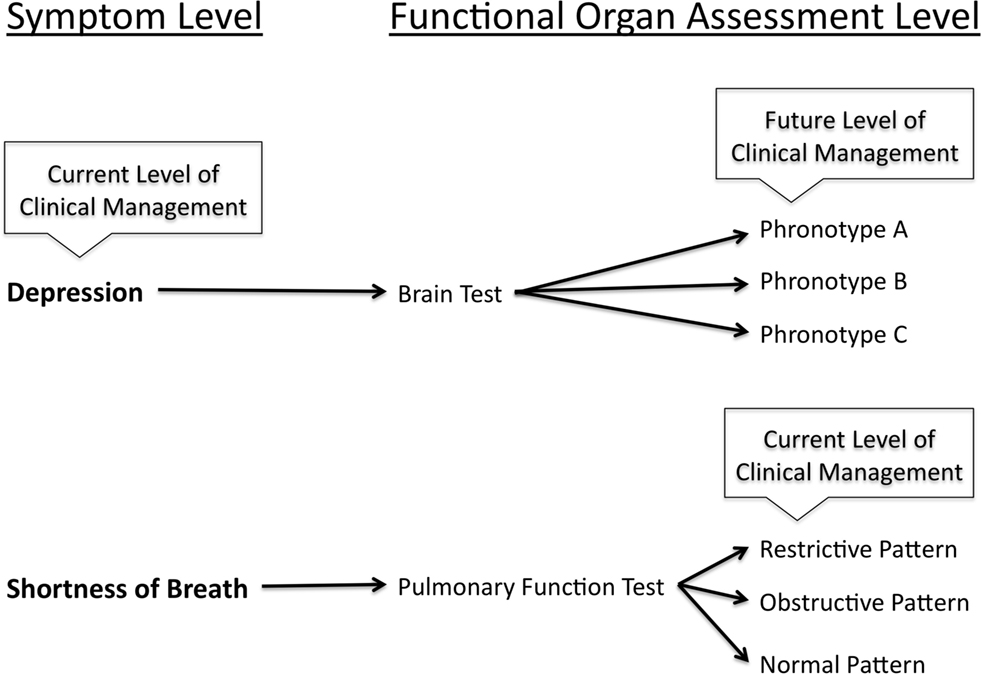
Figure 1. Outlines the role that phronotypes will play in the management of psychiatric disorders. As a simplified example, the symptoms of depression are compared with the symptoms of shortness of breath. For depressive symptoms (Depression), there currently exists no object test that can refine diagnosis to improve outcome. Conversely, the symptom of shortness of breath can be better characterized with a pulmonary function test (PFT). The results of the PFT enable the physician to more effectively target treatments and/or further evaluation.
Developing phronotypes requires a safe and reliable method to measure brain function. Although the brain is not an easily accessed organ, functional measures of brain activity have been developed. This has led to an explosion of research investigating the brain – especially as it relates to psychiatric disorders. New methods of imaging the brain as well as developing new analysis methods of older technologies for assessing brain function are rapidly progressing. Various investigators have used functional neuroimaging measures (Mayberg, 2003; Dougherty and Rauch, 2007; Phillips et al., 2008; Savitz and Drevets, 2009; Smith and Jakobsen, 2009) and electroencephalography (EEG) (Iosifescu, 2008) to compare responders and non-responders in an attempt to identify predictors of treatment response. Using EEG patterns of brain activity has shown promise predicting outcome better than a syndrome based diagnosis in MDD (Leuchter et al., 2009a,b) and attention-deficit/hyperactivity disorder (ADHD) (Hermens et al., 2005; Sangal and Sangal, 2006; Arns et al., 2008). Despite the elegant work produced, however, we still do not have an objective brain test that aids in clinical decision making (i.e., a more effective diagnostic model).
Lessons learned from other areas of medicine can provide some clues to developing such a diagnostic model based on phronotypes. Measuring the organ while it is functioning often provides the most sensitive information. For the brain, much like the heart, even during the “resting state,” there is considerable activity. Measuring the heart during a resting state (e.g., electrocardiogram) can provide useful information as well as in an activated state (e.g., cardiac stress test). Similarly, functional brain measures can be acquired during non-task periods (resting state) or in an “activated state.” There are a number of technologies that measure non-task brain function including EEG, positron emission tomography (PET), single photon emission computed tomography (SPECT), functional magnetic resonance imaging (fMRI), and functional near infrared spectroscopy (fNIRS). Non-task fMRI sometimes referred to resting state fMRI can be used to assess brain function in a number of ways including measuring connectivity between regions (Biswal et al., 1995). These connectivity measures can be compared to likelihood of treatment outcome to determine which provides clinically useful information. As an example, a recent study has provided preliminary evidence that connectivity measures acquired from non-task fMRI between the dorsal anterior cingulate gyrus and subcallosal cortex are correlated with and could predict treatment outcome in MDD (F.A. Kozel, personal communication).
Assessing brain function during an activated state requires the brain to be activated and a means to measure the functional brain changes (e.g., EEG, PET, SPECT, fMRI, fNIRS, etc.). The brain can be activated by various means including: one, performing a task (e.g., cognitive or emotional paradigms); two, with sensory stimulation (e.g., show flashing lights); or three, with direct brain stimulation (e.g., transcranial magnetic stimulation). As previously mentioned, the problem with tasks in psychiatric disorders is that a hallmark of many of our syndromes is the impact on the patient’s level of effort. Thus, specific tasks have the potential confound of effort that can be difficult to tease apart from changes due to pathology. Conversely, peripheral stimulation does not require patient effort and produces functional brain changes – but only in specific regions of the brain. These primary sensory regions of the brain are, however, not likely to be helpful in developing psychiatric phronotypes. In order to stimulate specific portions of the brain without patient effort, direct brain stimulation is required. An example of a technology that can safely stimulate the brain is transcranial magnetic stimulation (TMS) (George and Belmaker, 2007). TMS involves using rapidly alternating current in a coil to induce magnetic fields. These magnetic fields pass from the coil relatively unimpeded to the brain and induce electrical fields that can depolarize neurons (e.g., stimulating the thumb area of the motor cortex can make the thumb twitch) (Hallett, 2007). When TMS is provided repeatedly at a specific frequency, it is referred to as repetitive TMS (rTMS). Thus, rTMS is a safe and non-invasive technology to activate a focal portion of the cortex that does not require any effort on the part of the patient. Combining rTMS with a non-invasive functional brain measure offers the promise of developing a test to determine phronotypes within psychiatric syndromes. Specific portions of the cortex can be non-invasively stimulated and functional status measured. In addition, measuring the changes in other areas of the brain during stimulation can assess the functional connectivity status of the region being stimulated. Because the functional brain changes induced by rTMS can be altered depending on the TMS parameters being chosen (e.g., frequency), various aspects of brain inhibitory (e.g., 1 Hz) and excitatory (e.g., 10 Hz) function can be assessed in an individual. Thus, combining non-invasive brain stimulation with neuroimaging and testing the relationship to clinical outcome offers a means to determine which are clinically useful.
Further testing is required to determine if and how these technologies can better identify which patients are likely to respond to a particular treatment (i.e., phronotypes). Combining minimally invasive brain stimulation with neuroimaging or using non-task fMRI, however, offers the potential to provide research that will have direct clinical benefits in the near future. Importantly, these are only two examples of a number of different technologies that could provide brain measures that could successfully serve as phronotypes.
Developing phronotypes in psychiatry – regardless of the technology to be used – will require a specific scientific approach that has a different focus than much of the current research paradigms. The focus will be on separating individuals within a syndrome versus separating between a syndrome and health. Measures will be acquired at the level of the individual patient, and models will be empirically tested to determine if they accurately predict treatment outcome. In order for a model to have value, it must provide testable hypotheses and have some utility. Because we do not currently have neuropathologic findings for most psychiatric disorders to guide our diagnostic models, treatment outcome (i.e., endpoint upon which the model is tested) will need to drive our classification system. Having a brain measure that indicates likelihood of treatment response will provide valuable and much needed guidance to clinicians as well as help direct future research. Investigators doing basic science research can design specific animal models based on the objective phronotypes in humans to develop a greater understanding of the brain basis of these diseases and test new treatments. In addition, the ability to better categorize patients with phronotypes will have other positive consequences. Psychiatry can progress from a diagnostic nomenclature that uses terms that have become non-specific in common usage (e.g., depressed, manic, panic, anxious, and schizophrenic – often incorrectly used for dissociative identity disorder) to a nomenclature that is precise and emphasizes the brain dysfunction in these disorders. Moving away from terms that are often used to describe normal variations in emotion or behavior will help emphasize that these conditions are severe medical illnesses. Also, the ability to focus the pathology on the brain dysfunction versus the patient could help combat the stigma associated with mental illnesses.
In summary, phronotypes are objectively measured brain characteristics that provide clinically useful information. Developing phronotypes in psychiatry will require a specific research approach that focuses on an individual’s brain attributes and their relationship to clinical outcome. The value of the models being developed need to be judged based on empiric results of studies that test whether the models provide clinical advantage. These phronotypes have the potential to improve the lives of people who suffer from psychiatric disorders with respect to many aspects of their illness.
Financial Disclosures
Past Research and/or Salary Support: National Institute of Mental Health K23 NIMH 5 K23 MH070897-02, Role: PI; NIH/NCRR 5 UL1 RR024982-02 Packer (PI) Role: Pilot Study PI; Neuronetics Grant-in-kind support for supplies and use of equipment; the Defense Academy for Credibility Assessment (formerly the Department of Defense Polygraph Institute), Cephos Corp., Stanley Medical Research Institute, Cyberonics (Treatment studies D01, D02, D04, AN01) 2001-2005; Glaxo Smith Kline (Interleaved TMS-fMRI) 2002-2003
Paid Advisory/Consulting: None
Speaking: None
Equity Holdings (exclude mutual funds): None
Royalty/patent, other income: patents pending as an inventor through the Medical University of South Carolina on fMRI Detection of Deception, Guided rTMS Inhibition of Deception, Optimizing VNS dose with rTMS Other: 2004 Monthly Case Discussion Group Leader x1 Sponsored by Astra Zeneca; Unpaid scientific consultant to Cephos Corp.
Conflict of Interest Statement
The author declares that the research was conducted in the absence of any commercial or financial relationships that could be construed as a potential conflict of interest.
Acknowledgments
Dr. Kozel was supported by a K23 from the NIMH (5K23MH070897). The author acknowledges the assistance of Ms Cenir de Meira Arruda regarding the development of the word phronotype, Dr. Carol Tamminga for comments on the manuscript, and Ms. Jeanne McCurdy for editorial support. The views expressed in this article are those of the author and do not necessarily reflect the official policy or position of the National Institute of Mental Health, the National Institutes of Health, or the U.S. Government.
Footnote
- ^Phrono – Aeschylus uses the word “phrontis” that translated as “brain” in “Agamemnon” v. 165. Only the root phron- is used with the addition of one vowel (o) to make the connection with the next consonant.
References
Arns, M., Gunkelman, J., Breteler, M., and Spronk, D. (2008). EEG phenotypes predict treatment outcome to stimulants in children with ADHD. J. Integr. Neurosci. 7, 421–438.
Biswal, B., Yetkin, F. Z., Haughton, V. M., and Hyde, J. S. (1995). Functional connectivity in the motor cortex of resting human brain using echo-planar MRI. Magn. Reson. Med. 34, 537–541.
Dougherty, D. D., and Rauch, S. L. (2007). Brain correlates of antidepressant treatment outcome from neuroimaging studies in depression. Psychiatr. Clin. North Am. 30, 91–103.
DSM-IV. (1994). Diagnostic and Statistical Manual of Mental Disorders: DSM-IV, 4th Edn. Washington, D.C.: American Psychiatric Association.
George, M. S., and Belmaker, R. H. (2007). Transcranial Magnetic Stimulation in Clinical Psychiatry. Washington, D.C.: American Psychiatric Publishing, Inc.
Gottesman, I. I., and Gould, T. D. (2003). The endophenotype concept in psychiatry: etymology and strategic intentions. Am. J. Psychiatry 160, 636–645.
Hermens, D. F., Cooper, N. J., Kohn, M., Clarke, S., and Gordon, E. (2005). Predicting stimulant medication response in ADHD: evidence from an integrated profile of neuropsychological, psychophysiological and clinical factors. J. Integr. Neurosci. 4, 107–121.
Hyman, S. E., and Fenton, W. S. (2003). Medicine. What are the right targets for psychopharmacology? Science 299, 350–351.
Insel, T. R., and Wang, P. S. (2009). The STAR*D trial: revealing the need for better treatments. Psychiatr. Serv. 60, 1466–1467.
Iosifescu, D. V. (2008). Prediction of response to antidepressants: is quantitative EEG (QEEG) an alternative? CNS Neurosci. Ther. 14, 263–265.
Kozel, F. A., and Trivedi, M. H. (2008). Developing a neuropsychiatric functional brain imaging test. Neurocase 14, 54–58.
Leuchter, A. F., Cook, I. A., Gilmer, W. S., Marangell, L. B., Burgoyne, K. S., Howland, R. H., Trivedi, M. H., Zisook, S., Jain, R., Fava, M., Iosifescu, D., and Greenwald, S. (2009a). Effectiveness of a quantitative electroencephalographic biomarker for predicting differential response or remission with escitalopram and bupropion in major depressive disorder. Psychiatry Res. 169, 132–138.
Leuchter, A. F., Cook, I. A., Marangell, L. B., Gilmer, W. S., Burgoyne, K. S., Howland, R. H., Trivedi, M. H., Zisook, S., Jain, R., McCracken, J. T., Fava, M., Iosifescu, D., and Greenwald, S. (2009b). Comparative effectiveness of biomarkers and clinical indicators for predicting outcomes of SSRI treatment in Major Depressive Disorder: results of the BRITE-MD study. Psychiatry Res. 169, 124–131.
Lieberman, J. A., Stroup, T. S., McEvoy, J. P., Swartz, M. S., Rosenheck, R. A., Perkins, D. O., Keefe, R. S., Davis, S. M., Davis, C. E., Lebowitz, B. D., Severe, J., and Hsiao, J. K. (2005). Effectiveness of antipsychotic drugs in patients with chronic schizophrenia. N. Engl. J. Med. 353, 1209–1223.
Mayberg, H. S. (2003). Modulating dysfunctional limbic-cortical circuits in depression: towards development of brain-based algorithms for diagnosis and optimised treatment. Br. Med. Bull. 65, 193–207.
Nestler, E. J., Barrot, M., DiLeone, R. J., Eisch, A. J., Gold, S. J., and Monteggia, L. M. (2002). Neurobiology of depression. Neuron 34, 13–25.
Perlis, R. H., Ostacher, M. J., Patel, J. K., Marangell, L. B., Zhang, H., Wisniewski, S. R., Ketter, T. A., Miklowitz, D. J., Otto, M. W., Gyulai, L., Reilly-Harrington, N. A., Nierenberg, A. A., Sachs, G. S., and Thase, M. E. (2006). Predictors of recurrence in bipolar disorder: primary outcomes from the Systematic Treatment Enhancement Program for Bipolar Disorder (STEP-BD). Am. J. Psychiatry 163, 217–224.
Phillips, M. L., Travis, M. J., Fagiolini, A., and Kupfer, D. J. (2008). Medication effects in neuroimaging studies of bipolar disorder. Am. J. Psychiatry 165, 313–320.
Rush, A. J., Trivedi, M. H., Wisniewski, S. R., Nierenberg, A. A., Stewart, J. W., Warden, D., Niederehe, G., Thase, M. E., Lavori, P. W., Lebowitz, B. D., McGrath, P. J., Rosenbaum, J. F., Sackeim, H. A., Kupfer, D. J., Luther, J., and Fava, M. (2006). Acute and longer-term outcomes in depressed outpatients requiring one or several treatment steps: a STAR*D report. Am. J. Psychiatry 163, 1905–1917.
Sangal, R. B., and Sangal, J. M. (2006). Attention-deficit/hyperactivity disorder: use of cognitive evoked potential (P300) to predict treatment response. Clin. Neurophysiol. 117, 1996–2006.
Savitz, J., and Drevets, W. C. (2009). Bipolar and major depressive disorder: neuroimaging the developmental-degenerative divide. Neurosci. Biobehav. Rev. 33, 699–771.
Keywords: diagnosis, disease models, psychiatry, phronotype, biomarkers
Citation: Kozel FA (2010) Identifying phronotypes in psychiatry. Front. Psychiatry 1:141. doi: 10.3389/fpsyt.2010.00141
Received: 01 September 2010;
Accepted: 27 September 2010;
Published online: 20 October 2010
Edited by:
Zafiris J. Daskalakis, University of Toronto, CanadaReviewed by:
Dennis J. L. G. Schutter, Utrecht University, NetherlandsAristotle Voineskos, Centre for Addiction and Mental Health, Canada
Copyright: © 2010 Kozel. This is an open-access article subject to an exclusive license agreement between the authors and the Frontiers Research Foundation, which permits unrestricted use, distribution, and reproduction in any medium, provided the original authors and source are credited.
*Correspondence: F. Andrew Kozel, Department of Psychiatry, University of Texas Southwestern Medical Center, 5323 Harry Hines Boulevard, Dallas, TX 75390-9119, USA. e-mail: andrew.kozel@utsouthwestern.edu