- 1Australian Centre for Ecogenomics, School of Chemistry and Molecular Biosciences, The University of Queensland, Brisbane, QLD, Australia
- 2Department of Biochemistry and Microbiology, Rutgers University, New Brunswick, NJ, United States
Dinoflagellates are a group of diverse protists with complex genomes whose gene expression regulation mechanisms remain little known. RNA editing is a post-transcriptional regulatory mechanism of gene expression utilized by diverse species, and has been described primarily in the plastid and mitochondrial genomes of dinoflagellates. Its role in post-transcriptional regulation in the nuclear genomes of dinoflagellates remains largely unexplored. Here, integrating genome and transcriptome data from two dinoflagellate taxa in a comparative analysis, we identified 10,486 and 69,953 putative RNA editing sites in the nuclear genomes of the coral symbiont, Durusdinium trenchii CCMP2556 and the free-living bloom-forming taxon, Prorocentrum cordatum CCMP1329. We recovered all 12 possible types of RNA edits, with more edits representing transitions than transversions. In contrast to other eukaryotes, we found a dominance of A-to-T transversion in non-coding regions, many of which were condition-specific. Overall, the RNA editing sites implicate 7.5% of D. trenchii genes and 13.2% of P. cordatum genes. Some sites (1.5% in D. trenchii and more-substantially 62.3% in P. cordatum) were edited at significantly different frequencies in distinct growth conditions. The distribution of editing types and locations exhibited conserved patterns between the two phylogenetically distant species. Interestingly, A-to-T editing within the untranslated regions appear to be associated with upregulation of the edited genes in response to heat stress. These results lend support to the hypothesis that RNA editing is a key molecular mechanism that underpins regulation of gene expression in dinoflagellates.
1 Introduction
Dinoflagellates are a group of diverse protists that range from free-living bloom-forming microalgae, parasites of crustaceans, to photosymbionts that critically sustain the health of biodiverse coral reefs. Despite their ecological importance, molecular genetic studies of dinoflagellates have long been challenged by their large, complex genomes that exhibit highly idiosyncratic characteristics compared to genomes of other protists and eukaryotes (Lin, 2011; Wisecaver and Hackett, 2011). Recently available genomes of dinoflagellate taxa revealed remarkable sequence and structural divergence (González-Pech et al., 2021; Dougan et al., 2022b; Shah et al., 2024), further complicating the effort in developing dinoflagellates as models (Ishida et al., 2023).
Importantly, the mechanisms of molecular regulation and their functional roles in stress response in dinoflagellates remain little known. DNA-binding transcription factors (TFs) are prevalent in eukaryote genomes, most constituting 4–8% of total proteins (Babu et al., 2004; Vaquerizas et al., 2009). In dinoflagellates, putative TFs showed a preferential binding to RNA molecules instead of DNA (Zaheri and Morse, 2021), and proteins containing DNA-binding domains account < 0.3% of all proteins (Bayer et al., 2012; Beauchemin et al., 2012). These results suggest that RNA-based post-transcriptional regulation is more prevalent in dinoflagellates than the canonical regulation via TFs.
RNA editing is a key mechanism of post-transcriptional regulation, in which an RNA sequence is altered after transcription, leading to changes in its regulatory properties and/or the resulting protein sequence. Described in diverse lineages of life (Liu et al., 2017; Chu and Wei, 2020; Nie et al., 2020; Birk et al., 2023), RNA editing has been associated with organismal adaptation to changing environmental conditions (Garrett and Rosenthal, 2012; Birk et al., 2023), whereas its deregulation associated with diseases (Jain et al., 2019; Li et al., 2022); substitutional edits are largely transitions, with the most common type from adenosine (A) to inosine (I; a mimic of guanosine [G]) (Nishikura, 2006; Duan et al., 2023). RNA editing has been described in dinoflagellates, primarily among transcripts of genes encoded in the plastid and mitochondrial genomes (Lin et al., 2002; Zauner et al., 2004; Wang and Morse, 2006; Howe et al., 2008; Zhang et al., 2008; Dang and Green, 2009; Mungpakdee et al., 2014; Shoguchi et al., 2020), up to nine of the 12 possible editing types (i.e. from any one RNA base to another). The most frequent editing types in the organellar genomes are A-to-G and T-to-C (Zhang et al., 2008; Mungpakdee et al., 2014), supporting the notion that most RNA edits represent transitions as observed in other eukaryotes. Some edits are known to remove in-frame stop codons to facilitate gene expression, and to reduce AT bias of mitochondrial encoded transcripts (Lin et al., 2002; Zhang et al., 2008; Waller and Jackson, 2009).
RNA editing in nuclear genes of dinoflagellates was described in the symbiotic species of Symbiodinium microadriaticum, implicating 1.6% of nuclear-encoded genes, and all 12 possible editing types (Liew et al., 2017). Although more transitions than transversions were observed overall with the most frequent type as C-to-T, the second most frequent edit is the transversion of A-to-T (Liew et al., 2017). This result presents the first clue of a distinct pattern of editing type in nuclear genomes of dinoflagellates compared to their organellar genomes and to other eukaryote genomes. However, variation among biological replicates were not considered in the study (Liew et al., 2017), and the distribution of editing errors was modelled as a binomial distribution, which could lead to false positives of RNA edits (Heinrich et al., 2012; Piechotta et al., 2017). These technical limitations (see also Ramaswami et al. (2013)) have now been resolved (Piechotta et al., 2017; Piechotta et al., 2022), enabling more-accurate identification of RNA editing sites. Given the extensive genomic divergence among dinoflagellates (Stephens et al., 2018; González-Pech et al., 2021; Shah et al., 2023), the conservation of RNA editing pattern among nuclear-encoded genes of these taxa and the roles of RNA editing in regulating gene expression remain little known.
Traditionally, RNA editing sites were determined individually based on PCR validation (Sommer et al., 1991), which is not practical for genome-wide analysis (Ramaswami and Li, 2016). High-throughput transcriptome data provides a useful platform for rapid first-pass screening for RNA editing by identifying sites where a nucleotide base differs between the transcriptome (e.g. RNA-Seq) data and the assembled genome sequences (i.e. the RNA-DNA difference, or RDD). However, 90% of RNA editing sites reported in the first such study (Li et al., 2011) were later found to be false positives (Schrider et al., 2011; Kleinman et al., 2012). These false positives may arise from single-nucleotide polymorphisms in the genome, and/or incorrect mapping of RNA-Seq reads onto pseudogenes or adjacent introns of a gene, due to inadequate resolution of intron/exon junctions. In addition, the reverse transcription of mRNA to cDNA (routine in RNA-Seq data generation) commonly involves first-strand cDNA synthesis primed with random hexamers, which may introduce further mismatch errors in the sequencing data. For these reasons, more-sophisticated statistical modelling and filtering approaches, such as JACUSA (Piechotta et al., 2017), were developed to minimize false positives in the identification of RNA editing sites using high-throughput sequencing data, particularly in distinguishing genome-sequence polymorphisms from RNA editing sites. Combining genome sequencing reads and the RNA-Seq reads derived from the same source strain/isolate, JACUSA first identifies and excludes putative sites of genome-sequence polymorphism. Coverage of genome versus RNA-Seq reads across the RDD sites is modelled following a distribution based on empirical Bayesian estimates, for which statistically significant RNA editing sites are identified using likelihood ratio tests against a probability vector expected at random. False positives arose from incorrect read mapping and reverse transcription errors are further minimized using a sophisticated filtering approach (Piechotta et al., 2017). Based on simulation of 60,000 random non-overlapping sites, JACUSA yielded the highest recovery of true positives at 95.9%, outperforming other tools including SAMtools/BCFtools (Li, 2011), REDItools (Picardi and Pesole, 2013) and MuTect (Cibulskis et al., 2013). The use of robust statistical models testing in JACUSA also enables the detection of RNA editing sites with low read-coverage and/or variant frequency, compared to the otherwise arbitrarily defined thresholds (e.g. ≥ 10 reads coverage and ≥ 10% variant frequency) used in other tools (Wang et al., 2013).
Here, using high-quality genome and transcriptome data from two distantly related taxa, we assess the evolutionary conservation and functional role of RNA editing sites in dinoflagellates. We targeted the thermotolerant coral symbiont Durusdinium trenchii CCMP2556 (Order Suessiales) and the free-living, bloom-forming Prorocentrum cordatum CCMP1329 (Order Prorocentrales), for which the relevant data are available. D. trenchii is found in tropical coral reefs (Cunning and Baker, 2020), whereas P. cordatum is a potential toxin producer found in open oceans globally (Seebens et al., 2016; Khanaychenko et al., 2019). Using independently generated transcriptome data of these isolates from distinct growth conditions related to heat stress, we assess condition-specific editing of RNAs, and its implications on differential gene expression.
2 Materials and methods
2.1 Genome and transcriptome data
For Durusdinium trenchii CCMP2556, the assembled genome and predicted protein-coding genes were obtained from Dougan et al. (2022a), and the assembled transcriptomes from Bellantuono et al. (2019). All relevant sequence reads were retrieved from NCBI, respectively for genome (BioProject PRJEB66001) and transcriptome (BioProject PRJNA508937) data. For Prorocentrum cordatum, the assembled genome, transcriptomes, and predicted protein-coding genes were acquired from Dougan et al. (2023), whereas the relevant sequence reads were retrieved from NCBI (BioProject PRJEB54915). See Tables 1, 2, and Supplementary Table 1 for detail.
2.2 Identification of RNA editing sites
We adopted a conservative approach (Piechotta et al., 2022) to identify putative RNA editing sites using a combination of genome and transcriptome data, specifically to tease apart the genomic heterogeneity (or polymorphism) and the actual edited RNAs as observed in the transcripts (i.e., the RNA-Seq data). An observed nucleotide variation in the RNA-Seq reads but not in genome-sequence reads, satisfying a stringent requirement indicating statistical significance, is considered a putative RNA edited site. To do this, 25% of all genome-sequence reads (randomly sampled) were mapped to the final genome assembly using bwa-mem v0.7.17 (Li, 2013) (https://github.com/lh3/bwa). RNA-Seq reads from each sample (3 replicates; 6 conditions for P. cordatum and 4 conditions for D. trenchii) were mapped to the genome assembly separately with HISAT2 v2.2.1 (Kim et al., 2019) using default parameters (‐‐no-discordant) and a HGFM index that was built using known exons and splice sites from the predicted gene models of dinoflagellates. This step is particularly important because non-canonical alternative splice sites have been described in dinoflagellate genes (Shoguchi et al., 2013; González-Pech et al., 2021), and the accurate inference of intron/exon junctions enhances the accuracy of identified RNA editing sites. PCR duplicates were marked by MarkDuplicates implemented in Picard v2.23.8 (https://broadinstitute.github.io/picard/). For each condition, mapping of RNA-Seq reads was then compared against the mapped genome-sequence reads using JACUSA v2.0.1 (Piechotta et al., 2022) (call-2 -F 1024 -P2 RF-FIRSTSTRAND -s -a D,Y,H:condition=1). Specifically, the genome and RNA sequencing data were modelled independently using Dirichlet-Multinomial distribution that accounts for overdispersion, a common phenomenon when the observed variance is higher than the theoretically expected variants. The null hypothesis posits that the genome sequencing and RNA-Seq datasets originate from the same distribution. A test statistic score (Piechotta et al., 2017; Piechotta et al., 2022) was calculated to quantify the likelihood of these two datasets originating from different distributions; a larger score indicates higher precision but lower sensitivity, while a score of 0 indicates identical distributions. Setting a high score threshold prioritizes precision over sensitivity. Following the authors’ recommendation, in a stringent approach, we consider an RNA edited site to be statistically significant only if it meets all five requirements: (a) a score > 1.15 (empirical threshold that maximizes overall accuracy); (b) coverage of genome reads > 10; (c) coverage of RNA reads from each sample > 5; (d) number of putative editing type is < 2; and (e) the editing site is present in all three replicates. Impact of each RNA editing events was assessed using snpEff v5.1 (Cingolani et al., 2012).
2.3 Identification of differentially edited sites
For each RNA editing site identified in this study, we assessed whether the edited frequency is significantly different between two conditions using JACUSA v2.0.1 (Piechotta et al., 2022) (call-2 -F 1024 -P2 RF-FIRSTSTRAND -s -a D,Y). In this analysis, we focus on distinct RNA edited sites based on their loci on the genome sequences. For D. trenchii, we compared (a) free-living (28°C + 34°C) versus in hospite (28°C + 34°C), and (b) 28°C (free-living + in hospite) versus 34°C (free-living + in hospite). For P. cordatum, we compared (a) 20°C (exponential + stationary) versus 26°C (exponential + stationary), (b) 20°C (exponential + stationary) versus 30°C (exponential + stationary), (c) 26°C (exponential + stationary) versus 30°C (exponential + stationary), and (d) exponential (20°C + 26°C + 30°C) versus stationary (20°C + 26°C + 30°C). Similar to our approach for identifying RNA editing sites (above), we assessed the likelihood of RNA-Seq reads from two distinct conditions to have originated from the same underlying read-base distribution, based on the test statistic score in JACUSA (Piechotta et al., 2017). Here, the results were filtered by requiring a score > 1.56 as recommended by the authors of JACUSA.
2.4 Analysis of correlation between RNA editing and gene expression
To assess the correlation between RNA editing and gene expression, we first focused on RNA editing sites that were located within P. cordatum genes for which normalised RNA-Seq read count varied significantly among different conditions, as indicated by a coefficient of variation greater than 0.5. For each RNA editing site, we calculated the repeated measures correlation (Bakdash and Marusich, 2017) between the editing frequency of the site and the normalised expression of the corresponding gene. The editing frequency of the site was defined as the number of RNA-Seq reads supporting the edited base divided by the total number of reads mapped to the site, whereas the normalised expression of genes was calculated using the median of ratios method with DESeq2 (Love et al., 2014). We adjusted the p-values from the repeated measures correlation for multiple tests using the false discovery rate (FDR) method, and considered the correlation with adjusted p-value < 0.05 as statistically significant.
3 Results
3.1 Distribution of RNA editing types and locations are conserved in nuclear genomes of dinoflagellates
Integrating the corresponding genome and transcriptome data for each taxon using a conservative approach and a set of stringent criteria (see Materials and Methods), we identified 10,486 high-confident putative RNA editing sites in D. trenchii (Figure 1A) and 69,953 in P. cordatum (Figure 1B), implicating all 12 possible types. The 6.7-fold higher number of putative RNA editing sites in P. cordatum than in D. trenchii may be due to the ~4.6-fold larger genome size of P. cordatum (Table 1), and the higher read coverage associated with this dataset (Supplementary Table 1).
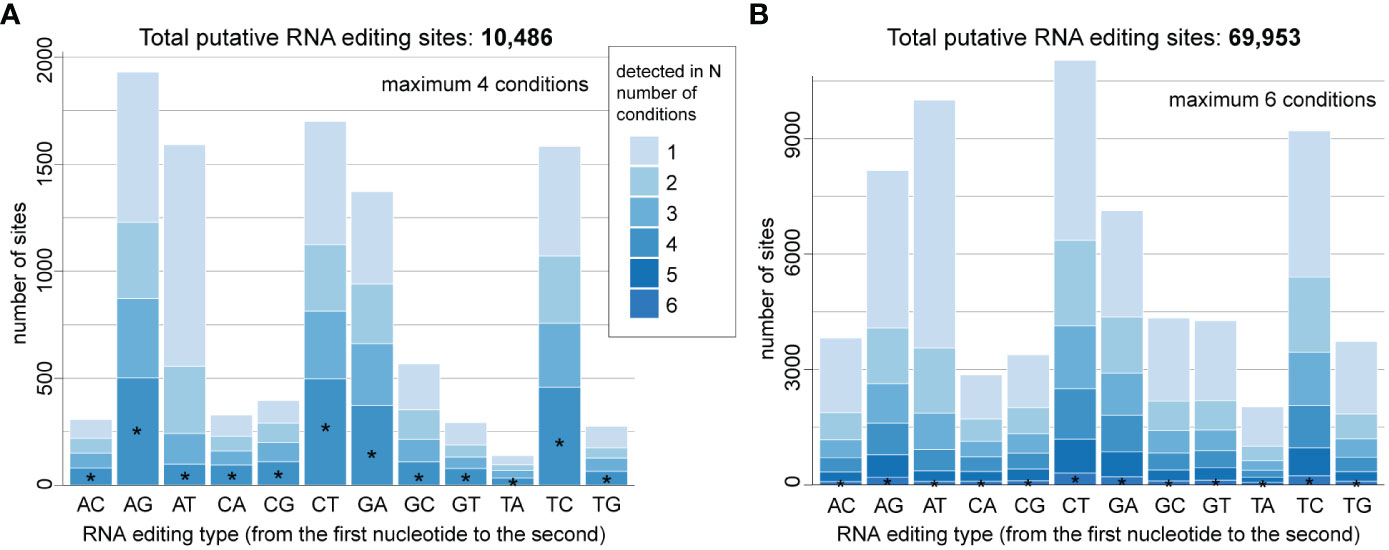
Figure 1 Distribution of overall RNA editing types in (A) D. trenchii and (B) P. cordatum, identified from combined RNA-Seq datasets generated from distinct growth conditions. Those detected in all four conditions are common editing types in D. trenchii. Those detected in all six conditions are common editing types in P. cordatum. The proportion of these common editing types is shown as the bottom portion of each stacked bar, marked with an asterisk.
Most edits represent transitions (i.e. A-to-G, C-to-T, G-to-A, and T-to-C) in both taxa (Figures 1A, B); the most common edit is A-to-G (1,930; 18.4%) for D. trenchii, and C-to-T (11,040; 15.9%) for P. cordatum. As similarly observed in nuclear genome of S. microadriaticum for which 19% of RNA edits represent the A-to-T transversion (Liew et al., 2017), we found substantial RNA edits to be A-to-T (i.e. 1,591 [15.2%] in D. trenchii and 10,003 [14.3%] in P. cordatum). A smaller percentage of edits (i.e. 23.9% in D. trenchii and 2.42% in P. cordatum) were detected in all growth conditions; these represent instances of common RNA edits. RNA edits detected in only one condition represent 38.3% of edits in D. trenchii and 47.8% in P. cordatum; this result lends support to the notion of condition-specific RNA editing (Liew et al., 2017) as a molecular response to distinct growth conditions including heat stress. Interestingly, A-to-T transversion is the most prevalent among condition-specific edits (i.e. 25.8% in D. trenchii and 19.3% in P. cordatum), suggesting their role in molecular response to changing environments is stronger than the other editing types.
We classified all putative RNA edits based on their loci relative to the annotated structural features of the genome sequences: intergenic, untranslated region (UTR), exon (synonymous substitution site), exon (non-synonymous substitution site), and intron (Figure 2A). Among these five classes, edits in intergenic regions were the most common, accounting for 32.7% of all editing sites in D. trenchii and 39.6% in P. cordatum (Figure 2A); these edits may reflect editing in regulatory non-coding elements, such as long non-coding RNAs (lncRNAs), or unannotated mRNAs. Regulation of lncRNAs that is mediated by RNA editing has been documented in humans, for which edited RNAs are known to alter the minimal free energy of lncRNA secondary structures (Gong et al., 2016), thereby controlling their functionality (Novikova et al., 2012). The functional role of intergenic RNA editing remains to be investigated, particularly on how the edits impact lncRNA expression, which in turn affect expression of protein-coding genes. Nevertheless, based on these data, we observed a prevalence of RNA editing in coding regions in both genomes. The coding sequences harbour 52% RNA editing sites (i.e. both synonymous and non-synonymous substitution sites) in D. trenchii and 35.5% in P. cordatum, whereas these sequences account for only 5.3% and 2.7% of bases respectively in the corresponding genome (Supplementary Table 2).
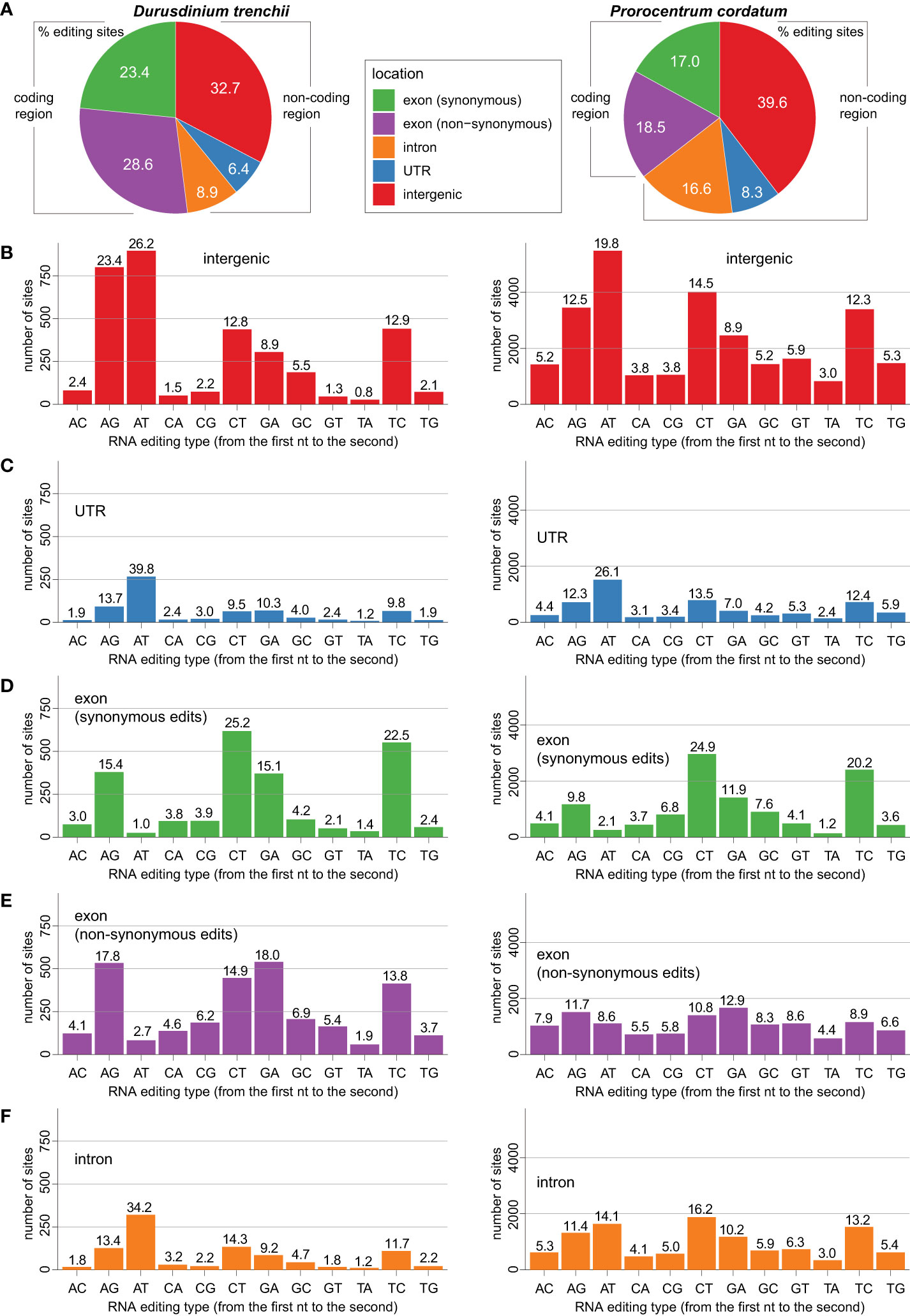
Figure 2 Distribution of RNA editing types recovered in genomic locations of D. trenchii (left) and P. cordatum (right), showing for (A) overall genomic features, (B) intergenic region, (C) UTR, (D) exon (synonymous edits), (E) exon (non-synonymous edits), and (F) intron.
In both genomes, we observed common RNA editing types that are prevalent in distinct structural genomic features. The A-to-T editing was the most frequent type in intergenic (Figure 2B) and UTR regions (Figure 2C), whereas C-to-T and T-to-C editing were more prevalent among synonymous sites in the exons (Figure 2D); no consistent patterns were observed among non-synonymous sites in the exons (Figure 2E) and among introns (Figure 2F). The tendency for A-to-T edits was the strongest in the UTR regions. For instance in D. trenchii (Figure 2C), the percentage of A-to-T edits (39.8%) was approximately three-fold greater than the second-most dominant editing type, A-to-G (13.7%).
In total, we identified 7,061 and 42,268 RNA edits within protein-coding genes, implicating 4,164 (7.5% of total 55,799) genes in D. trenchii and 11,312 (13.2% of total 85,849) genes in P. cordatum (Figure 3A; Table 1). The earlier study of S. microadriaticum (Liew et al., 2017) revealed that the distribution of edited sites across genes was not uniform, with majority (77.1%) of edited sites occurred in only 1.6% of all genes. We observed a similar trend in our results, with 49.2% of edited sites in D. trenchii and 52.3% in P. cordatum occurred in 1.6% of all genes in each corresponding genome. Many edited genes (71.0% for D. trenchii and 47.8% for P. cordatum) had only one RNA edit, whereas the greatest number of edited sites in a single gene was 32 (D. trenchii) and 86 (P. cordatum). The edited genes appear to exhibit greater expression than the non-edited genes (Supplementary Figure 1). We further observed a tendency for locations of RNA editing sites to cluster together in D. trenchii (Figure 3B) and P. cordatum (Figure 3C) based on the distance between an edit to the next; this tendency is statistically significant (p < 2.2×10-16, Wilcoxon signed-rank test) in both cases when compared to the scenario we expect by chance (based on 10,000 Monte Carlo simulated distributions), lending support to observations in the S. microadriaticum study (Liew et al., 2017).
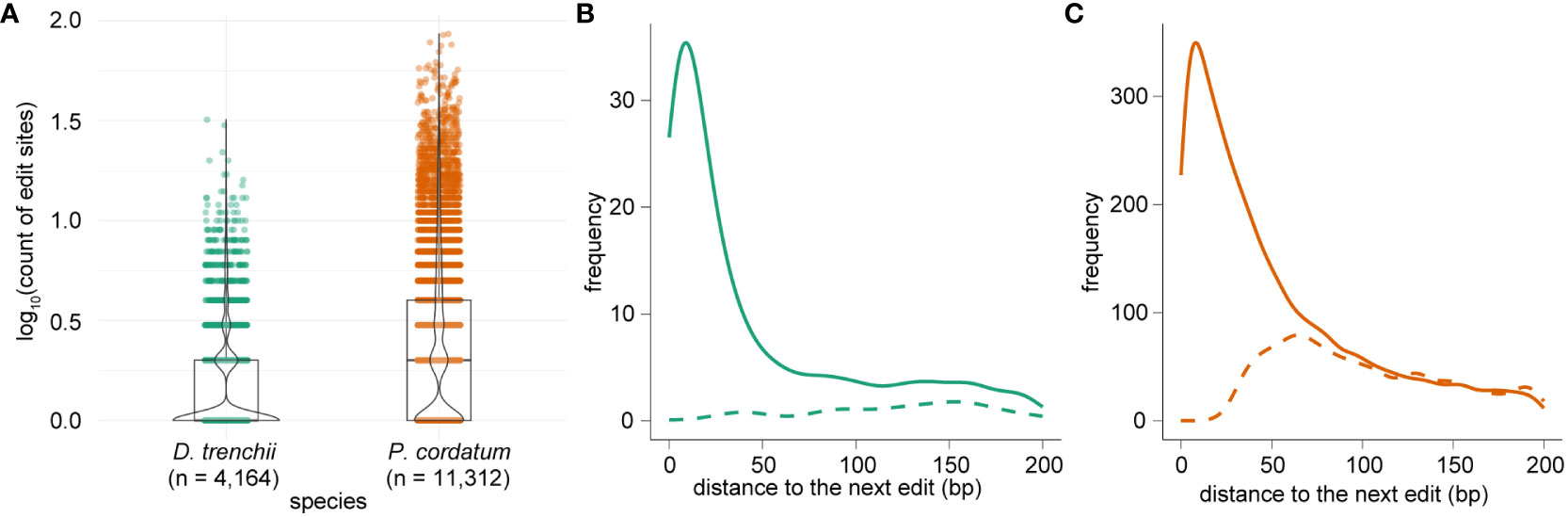
Figure 3 RNA edited sites per gene in dinoflagellates, showing (A) the number of sites per gene in logarithmic scale for both D. trenchii and P. cordatum, and the distribution of distances to neighbouring edits in (B) D. trenchii and (C) P. cordatum, in which each solid line represents the observed distribution, and a dashed line represents the expected distribution generated from 10,000 Monte Carlo simulations.
3.2 Differential mRNA editing in distinct growth conditions
Editing of mRNAs (i.e. in the UTR, intron, and the synonymous and non-synonymous sites of exon) have the potential to alter both their sequences and eventual expression. The frequency of mRNA editing at a site can modulate as a molecular response to changing conditions. In general, we recovered distinct numbers of differentially edited (DE) sites in the two datasets: 61 (0.6% of 10,486) in response to lifestyle and/or temperature in D. trenchii, compared to 43,591 (62.3% of 69,953) in response to growth phase and/or temperature in P. cordatum (Table 3). As a more-specific example, during a heat stress response, we identified 35 distinct DE sites (28°C versus 34°C) in D. trenchii, compared to 23,573 (20°C versus 26°C) in P. cordatum. Fewer DE sites in D. trenchii may be due in part to the fact that the naturally thermotolerant D. trenchii did not elicit strong molecular responses specific to heat stress (Bellantuono et al., 2019), and/or whole genome duplication known in this species (Dougan et al., 2022a) resulted in a smaller proportion of uniquely mapped reads (Supplementary Table 1). The 23,573 sites identified for P. cordatum provide a strong statistical power for comparative analysis; we assessed if the editing frequency at these sites reflects the differential responses between any two growth conditions. If the general editing machinery is activated or shutdown (Rieder et al., 2015), we expect to see a consistent pattern of either increased or decreased editing at the DE sites, i.e., either almost all sites (~100%) would have an increased edited frequency, or almost none (~0%) of them would. In all comparisons, we observed 46.9% to 51.1% of the DE sites to show an increased editing frequency, supporting the notion that mRNA editing in dinoflagellates is condition-specific (Liew et al., 2017).
In S. microadriaticum (Liew et al., 2017), edited sites within differentially edited genes were predominantly associated with non-synonymous substitutions of a non-polar amino acid. Among the DE sites we identified in this study, 28 of 61 (45.9%) in D. trenchii were associated with non-synonymous substitutions, compared to 8,057 of 43,591 (18.5%) in P. cordatum. We adapted the approach by Liew et al. (2017) to assess more specifically the effect of non-synonymous substitution associated with all DE sites, specifically the substitution of an amino acid for another, independently for D. trenchii (Figure 4A) and for P. cordatum (Figure 4B). No clear pattern was observed for D. trenchii, likely due to the small number of DE sites. For P. cordatum, we observed a prevalence of amino acid changes from a non-polar side chain to another, similar to S. microadriaticum (Liew et al., 2017); the most common change was from alanine (Ala) to valine (Val) at 199 sites (Figure 4B).
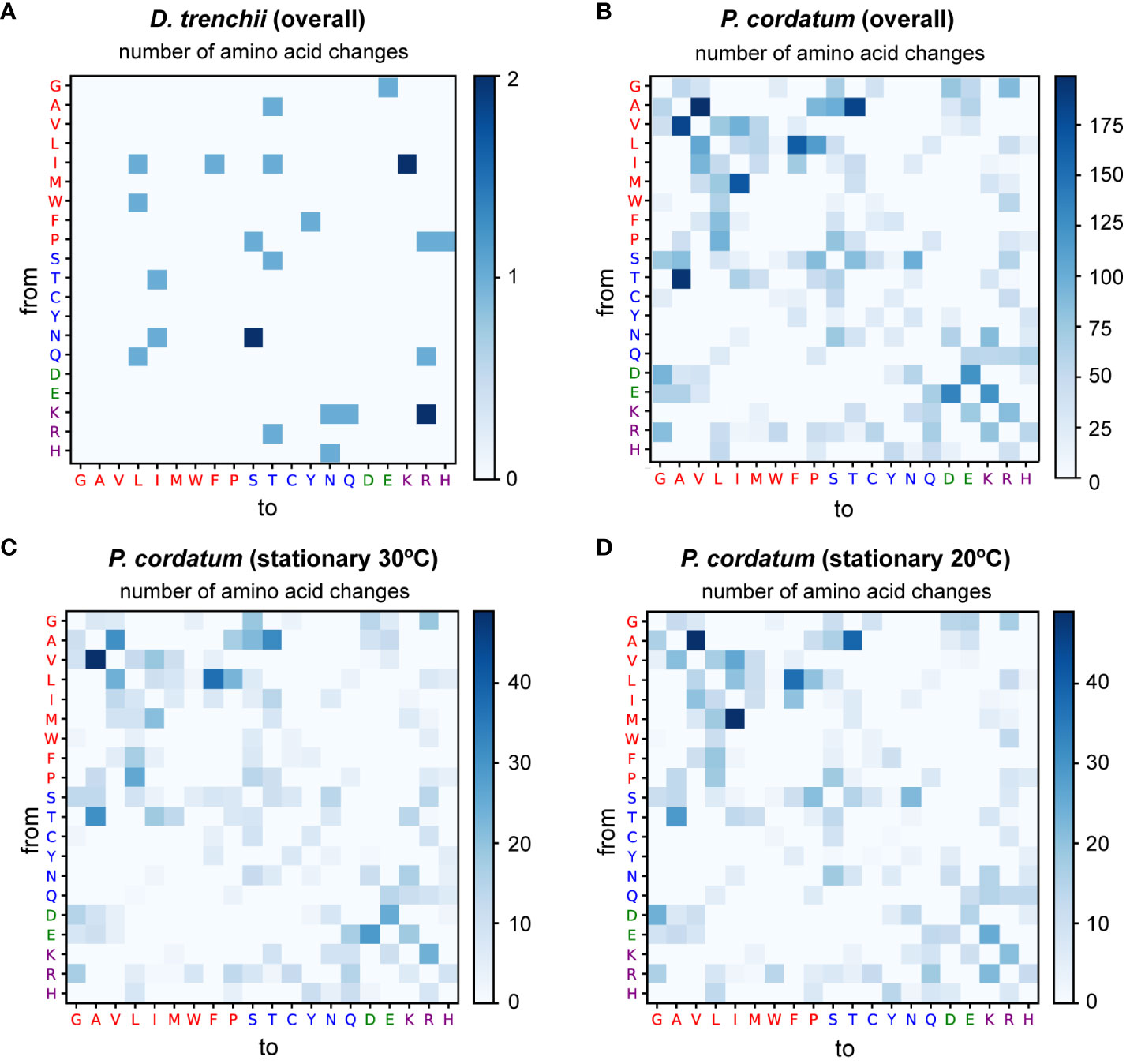
Figure 4 Substitution of amino acids caused by differential mRNA editing events, shown independently for (A) D. trenchii overall, (B) P. cordatum overall, and P. cordatum during stationary phase at (C) 30°C, and (D) 20°C. The y-axis represents the original amino acid, while the x-axis represents the post-edited amino acid. Each amino acid is denoted by its single-letter code, coloured based on the property of its side chain, with non-polar residues shown in red, polar uncharged in blue, acidic in green, and basic in purple.
These results reveal the tendency for the non-synonymous substitutions introduced by mRNA editing to preserve the physicochemical property of the amino acids, and thus the protein structure, to mitigate alterations that lead to non-functional proteins. Moreover, the near-symmetric matrix shown in Figure 4B indicates that the frequency of DE sites resulting in the change from one amino acid to another is similar regardless of the direction of change. Interestingly, the direction of amino acid change differs in response to heat stress. For instance, in our analysis of RNA edits for P. cordatum during stationary phase, Val-to-Ala changes (49) were more frequent than Ala-to-Val (31) at 30°C (Figure 4C), whereas the reverse trend was observed at 20°C (21 Val-to-Ala, and 49 Ala-to-Val; Figure 4D); these changes implicate 143 genes that encode functions including transmembrane proteins and transporters (Supplementary Table 3). This observation lends support to the notions that protein sequences of thermophiles tend to have alanine in place of valine in their homologs among the mesophiles (Vogt et al., 1997), and that Val-to-Ala changes improve thermal stability of transmembrane proteins (Kulandaisamy et al., 2021). For instance, a P. cordatum gene that putatively encodes ceramide synthase (a homolog of longevity assurance gene) harbours a T-to-C editing site at the second codon position resulting in the Val-to-Ala change, with ~10-fold higher editing frequency during stationary growth phase at 30°C than at 20°C (Figure 5). The gene homolog in yeast is known to promote programmed cell death by establishing a lateral diffusion barrier in the nuclear envelope, thereby accumulating aging factors (Megyeri et al., 2019). The P. cordatum gene may play a similar role under heat stress, as programmed cell death is common in unicellular organisms to enhance genetic and population fitness (Bidle, 2016). Although greater editing frequency was observed in cells grown at 30°C, only 1.7–2.7% of mapped transcripts among the three replicates exhibit the T-to-C edit (Figure 5). This observation may reflect heterogeneity of transcriptomes from the bulk RNA-Seq analysis, which can be further investigated using single-cell transcriptome analysis.
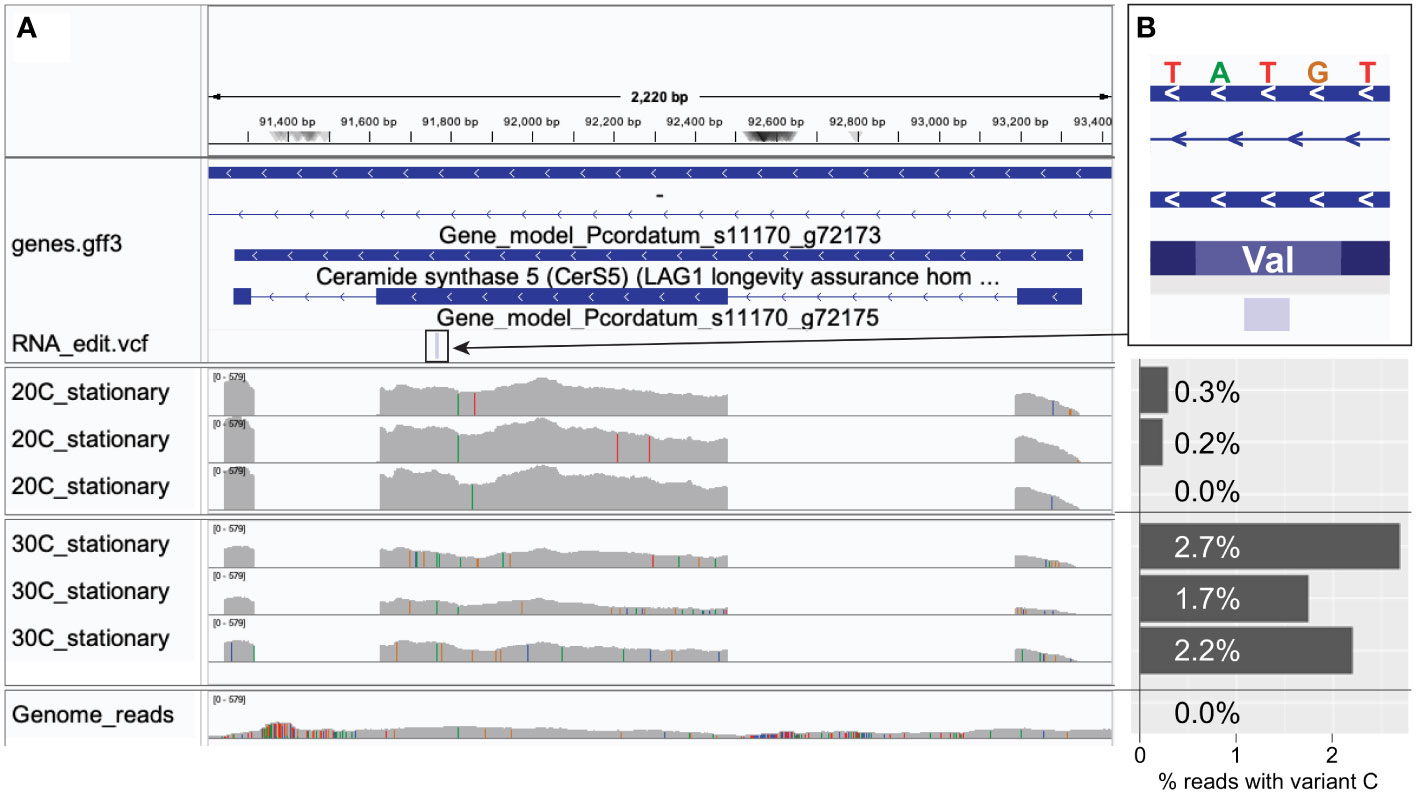
Figure 5 An example of differentially edited site, showing a T-to-C edit relative to the P. cordatum genome, in cells extracted during stationary growth phase at 30°C versus 20°C. (A) Coverage of mapped genome versus RNA-Seq reads along the implicated gene that putatively encodes ceramide synthase (a homolog of longevity assurance gene). (B) Zoomed inset (at 5 bp resolution) centred at the RNA editing site showing T (in the genome) at the second position of the codon of valine, with the percentage of mapped reads that exhibit editing at that position (with C instead of T) shown as a bar chart for each RNA-Seq sample and for the genome reads.
3.3 Correlation of mRNA editing to gene expression
To assess the potential correlation of mRNA editing to gene expression, we focus on the large number of DE sites we identified in P. cordatum; these data provide a strong statistical power for comparative analysis. We first assessed the correlation of mRNA edits to gene expression in the six growth conditions (Table 2). Among the 53,595 mRNA edits harboured by 14,700 genes that exhibit highly variable expression (coefficient of variation > 0.5), we identified 4,309 edited sites (8.0% of 53,595) for which the edit frequency was positively correlated (repeated measures correlation (Bakdash and Marusich, 2017), FDR < 0.05) with the expression of the corresponding transcripts.
The distribution of correlation coefficients differed by their genomic region (Figure 6A) and type of mRNA edit (Figure 6B). The strongest correlation to gene expression was observed in edits within the UTR regions (repeated measures correlation, FDR < 0.05, mean coefficient = 0.56), and among the A-to-T edits (FDR < 0.05, mean coefficient = 0.55); this observation suggests that editing in the UTR and/or the A-to-T editing type are associated with up-regulation of gene expression. Because the A-to-T transversion is also dominant among edits observed in UTR regions (Figure 2C), we further assessed whether the observed association is solely due to editing in UTR regions, A-to-T editing, or both. We found that gene expression was positively correlated (Fisher’s exact test, p < 0.01; Supplementary Table 4) to A-to-T editing consistently in all editing classes (reflecting genomic regions), and within the UTR regions, to multiple editing types, i.e. A-to-T, A-to-G, G-to-A, C-to-T, and T-to-C (Fisher’s exact test, p <0.01; Supplementary Table 5). These results suggest that editing in the UTR and the A-to-T editing type are associated with the up-regulation of gene expression independently. These edits may modulate gene expression by altering the binding efficiency of the mRNAs with other regulatory elements such as microRNAs (Shang et al., 2023), and/or changing the secondary structure of mRNAs (Ruchika and Tsukahara, 2021), although the mechanisms that underpin these associations remain to be investigated.
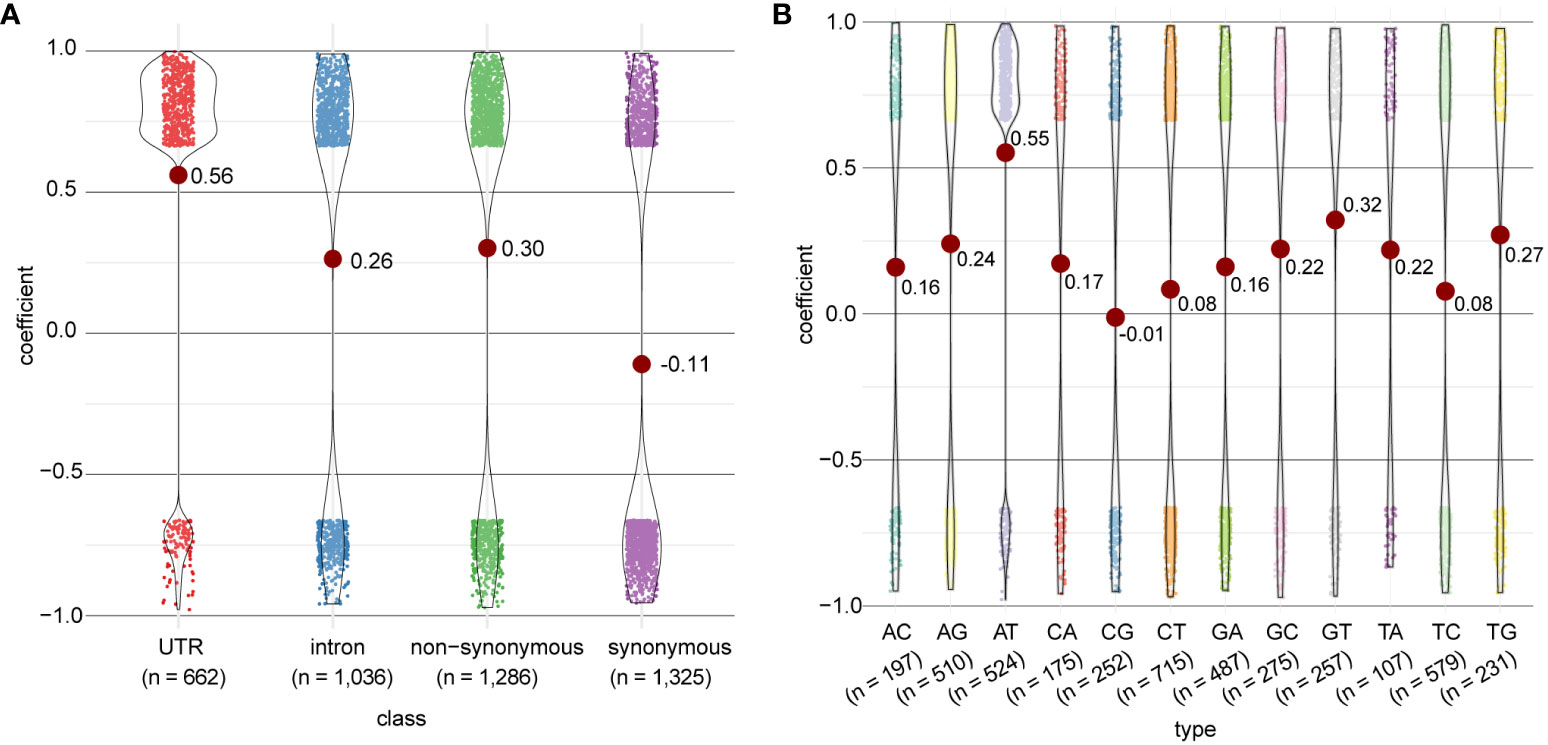
Figure 6 Distribution of correlation coefficients between mRNA edits and the associated gene expression in P. cordatum, based on (A) distinct genomic regions and (B) type of RNA edit. Each dot represents an RNA editing event for which the editing frequency is significantly correlated to gene expression (repeated measures correlation, FDR <0.05). Mean values of the coefficient are shown.
4 Discussion
The prevalence of A-to-T transversion among the RNA edits we observed in dinoflagellates are in stark contrast to findings in other eukaryotes, in which A-to-T commonly constitutes < 5% of RNA edits (Li et al., 2011; Wang et al., 2020). Our approach using JACUSA (Piechotta et al., 2017; Piechotta et al., 2022) rely on sequencing depth of genome and transcriptome data independently as supporting evidence to tease apart single nucleotide polymorphism in the genome (i.e. genomic heterogeneity) versus an edited transcript (see Materials and Methods). A potential concern of this approach is that rare genomic polymorphisms may have escaped detection, causing a false positive detection of an RNA edit when compared against an assembled transcript, i.e. a base difference in the transcript is an outcome of genomic nucleotide polymorphism rather than an actual editing event. If the large number of A-to-T transversions reflect this technical bias, the genome-sequence coverage implicating these sites is expected to be significantly lower than those of the other editing sites. We observed no significant biases in genome-sequence coverage across sites implicating the 12 editing types in both genomes (Supplementary Figure 2). In combination with the earlier observation in S. microadriaticum based on a different approach (Liew et al., 2017), the prevalence of A-to-T edits is unlikely due to technical biases, and reflect a distinct editing feature in the nuclear genomes of dinoflagellates. The abundance of A-to-T edits we identified in the intergenic and UTR regions likely explain why few A-to-T edits were identified in the earlier studies of plastid and mitochondrial genomes in dinoflagellates (Lin et al., 2002; Zauner et al., 2004; Wang and Morse, 2006; Howe et al., 2008; Zhang et al., 2008; Dang and Green, 2009; Mungpakdee et al., 2014; Shoguchi et al., 2020), which largely focused on protein-coding sequences.
Although the genome data we used are of reasonably high quality, and the transcriptome data we analysed are extensive (especially for P. cordatum), we cannot dismiss the fact that some of the de novo assembled genome sequences could be misassembled, which may affect structural annotation of gene and genome features (Chen et al., 2020). The P. cordatum genome assembly (Dougan et al., 2023) was derived from combined PacBio long-read and Illumina short-read data using MaSuRCA, whereas the D. trenchii genome assembly (Dougan et al., 2022a) was derived from 10X Genomics linked reads, assembled using Supernova, the assembler specifically designed for these data. Both assemblies represent haploid genomes, thus the impact of genomic heterozygosity on biasing our results is expected to be minimal. The difference in data-generation strategy may contribute in part to the variable contiguity (i.e. N50 scaffold length of 349.2 Kb for P. cordatum and 774.3 Kb for D. trenchii; Table 1). The transcriptome data we used in this analysis were generated from the same isolates using RNA-Seq technology, for which polyadenylated RNAs were specifically selected for reverse transcription during preparation of sequencing libraries, which also include a step of DNAse treatment to rid of DNA in the samples. As such, RNA-Seq reads that did not map onto coding sequence regions of the genomes likely reflect transcribed non-coding RNAs (Chen et al., 2024), not DNA contamination in the samples. Trimming was not performed on these sequence reads, because JACUSA explicitly accounts for base-quality scores when estimating read-base distributions (Piechotta et al., 2017).
Although we incorporate information of known intron/exon splice signal in our analysis (see Materials and Methods) to minimize incorrect read mapping in these regions (Ramaswami and Li, 2016), we cannot dismiss false positives with absolute certainty. For instance, differences between transcripts of multiple near-identical genes that may not be resolved fully in the genome. In addition, some instances of RNA base modification, e.g. N6-methyladenosine and pseudouridine (Li et al., 2016; Schaefer et al., 2017), may affect the reverse transcription of mRNA to cDNA during the preparation of sequencing library for RNA-Seq, which may in turn manifest as an RNA edit during analysis. Nevertheless, the putative RNA editing sites we identified provide a useful resource for future research to experimentally validate the functional roles of these edits on regulating gene expression, e.g. via targeted PCR amplification.
This study presents a comprehensive analysis of RNA editing in the nuclear genomes of dinoflagellates. The pattern of RNA editing types in the distinct regions of nuclear genomes appears to be evolutionarily conserved, and underscores the important yet under-explored functional role of RNA editing in molecular regulation in dinoflagellates.
Data availability statement
The datasets presented in this study can be found in online repositories. The names of the repository/repositories and accession number(s) can be found in the article/Supplementary Material.
Author contributions
YC: Conceptualization, Formal analysis, Investigation, Methodology, Visualization, Writing – original draft, Writing – review & editing. KED: Supervision, Writing – review & editing. DB: Funding acquisition, Writing – review & editing. CXC: Conceptualization, Funding acquisition, Supervision, Visualization, Writing – review & editing.
Funding
The author(s) declare financial support was received for the research, authorship, and/or publication of this article. This research was supported by The University of Queensland Research Training Program scholarship (YC), the Australian Research Council grant DP19012474 awarded to CXC and DB, and the Australian Academy of Science Thomas Davies Grant for Marine, Soil, and Plant Biology awarded to CXC. DB was also supported by NSF grant NSF-OCE 1756616 and a NIFA-USDA Hatch grant (NJ01180).
Acknowledgments
This project is supported by high-performance computing facilities at the National Computational Infrastructure (NCI) National Facility systems through the NCI Merit Allocation Scheme (Project d85) awarded to CXC, the University of Queensland Research Computing Centre, and computing facility at the Australian Centre for Ecogenomics, School of Chemistry and Molecular Biosciences at The University of Queensland.
Conflict of interest
The authors declare that the research was conducted in the absence of any commercial or financial relationships that could be construed as a potential conflict of interest.
The author(s) declared that they were an editorial board member of Frontiers, at the time of submission. This had no impact on the peer review process and the final decision.
Publisher’s note
All claims expressed in this article are solely those of the authors and do not necessarily represent those of their affiliated organizations, or those of the publisher, the editors and the reviewers. Any product that may be evaluated in this article, or claim that may be made by its manufacturer, is not guaranteed or endorsed by the publisher.
Supplementary material
The Supplementary Material for this article can be found online at: https://www.frontiersin.org/articles/10.3389/frpro.2024.1320917/full#supplementary-material
References
Babu M. M., Luscombe N. M., Aravind L., Gerstein M., Teichmann S. A. (2004). Structure and evolution of transcriptional regulatory networks. Curr. Opin. Struct. Biol. 14, 283–291. doi: 10.1016/j.sbi.2004.05.004
Bakdash J. Z., Marusich L. R. (2017). Repeated measures correlation. Front. Psychol. 8. doi: 10.3389/fpsyg.2017.00456
Bayer T., Aranda M., Sunagawa S., Yum L. K., DeSalvo M. K., Lindquist E., et al. (2012). Symbiodinium transcriptomes: genome insights into the dinoflagellate symbionts of reef-building corals. PLoS One 7, e35269. doi: 10.1371/journal.pone.0035269
Beauchemin M., Roy S., Daoust P., Dagenais-Bellefeuille S., Bertomeu T., Letourneau L., et al. (2012). Dinoflagellate tandem array gene transcripts are highly conserved and not polycistronic. Proc. Natl. Acad. Sci. U. S. A 109, 15793–15798. doi: 10.1073/pnas.1206683109
Bellantuono A. J., Dougan K. E., Granados-Cifuentes C., Rodriguez-Lanetty M. (2019). Free-living and symbiotic lifestyles of a thermotolerant coral endosymbiont display profoundly distinct transcriptomes under both stable and heat stress conditions. Mol. Ecol. 28, 5265–5281. doi: 10.1111/mec.15300
Bidle K. D. (2016). Programmed cell death in unicellular phytoplankton. Curr. Biol. 26, R594–R607. doi: 10.1016/j.cub.2016.05.056
Birk M. A., Liscovitch-Brauer N., Dominguez M. J., McNeme S., Yue Y., Hoff J. D., et al. (2023). Temperature-dependent RNA editing in octopus extensively recodes the neural proteome. Cell 186, 2544–2555. doi: 10.1016/j.cell.2023.05.004
Chen Y., Dougan K. E., Nguyen Q., Bhattacharya D., Chan C. X. (2024). Genome-wide transcriptome analysis reveals the diversity and function of long non-coding RNAs in dinoflagellates. NAR Genom. Bioinform. 6, lqae016. doi: 10.1093/nargab/lqae016
Chen Y., González-Pech R. A., Stephens T. G., Bhattacharya D., Chan C. X. (2020). Evidence that inconsistent gene prediction can mislead analysis of dinoflagellate genomes. J. Phycol 56, 6–10. doi: 10.1111/jpy.12947
Chu D., Wei L. (2020). Reduced C-to-U RNA editing rates might play a regulatory role in stress response of Arabidopsis. J. Plant Physiol. 244, 153081. doi: 10.1016/j.jplph.2019.153081
Cibulskis K., Lawrence M. S., Carter S. L., Sivachenko A., Jaffe D., Sougnez C., et al. (2013). Sensitive detection of somatic point mutations in impure and heterogeneous cancer samples. Nat. Biotechnol. 31, 213–219. doi: 10.1038/nbt.2514
Cingolani P., Platts A., Wang le L., Coon M., Nguyen T., Wang L., et al. (2012). A program for annotating and predicting the effects of single nucleotide polymorphisms, SnpEff: SNPs in the genome of Drosophila melanogaster strain w1118; iso-2; iso-3. Fly 6, 80–92. doi: 10.4161/fly.19695
Cunning R., Baker A. C. (2020). Thermotolerant coral symbionts modulate heat stress-responsive genes in their hosts. Mol. Ecol. 29, 2940–2950. doi: 10.1111/mec.15526
Dang Y., Green B. R. (2009). Substitutional editing of Heterocapsa triquetra chloroplast transcripts and a folding model for its divergent chloroplast 16S rRNA. Gene 442, 73–80. doi: 10.1016/j.gene.2009.04.006
Dougan K. E., Bellantuono A. J., Kahlke T., Abbriano R. M., Chen Y., Shah S., et al. (2022a). Whole-genome duplication in an algal symbiont serendipitously confers thermal tolerance to corals. bioRxiv. 2022.04.10.487810. doi: 10.1101/2022.04.10.487810
Dougan K. E., Deng Z. L., Wohlbrand L., Reuse C., Bunk B., Chen Y., et al. (2023). Multi-omics analysis reveals the molecular response to heat stress in a "red tide" dinoflagellate. Genome Biol. 24, 265. doi: 10.1186/s13059-023-03107-4
Dougan K. E., González-Pech R. A., Stephens T. G., Shah S., Chen Y., Ragan M. A., et al. (2022b). Genome-powered classification of microbial eukaryotes: focus on coral algal symbionts. Trends Microbiol. 30, 831–840. doi: 10.1016/j.tim.2022.02.001
Duan Y., Li H., Cai W. (2023). Adaptation of A-to-I RNA editing in bacteria, fungi, and animals. Front. Microbiol. 14. doi: 10.3389/fmicb.2023.1204080
Garrett S., Rosenthal J. J. C. (2012). RNA editing underlies temperature adaptation in K+ channels from polar octopuses. Science 335, 848–851. doi: 10.1126/science.1212795
Gong J., Liu C., Liu W., Xiang Y., Diao L., Guo A.-Y., et al. (2016). LNCediting: a database for functional effects of RNA editing in lncRNAs. Nucleic Acids Res. 45, D79–D84. doi: 10.1093/nar/gkw835
González-Pech R. A., Stephens T. G., Chen Y., Mohamed A. R., Cheng Y., Shah S., et al. (2021). Comparison of 15 dinoflagellate genomes reveals extensive sequence and structural divergence in family Symbiodiniaceae and genus Symbiodinium. BMC Biol. 19, 73. doi: 10.1186/s12915-021-00994-6
Heinrich V., Stange J., Dickhaus T., Imkeller P., Krüger U., Bauer S., et al. (2012). The allele distribution in next-generation sequencing data sets is accurately described as the result of a stochastic branching process. Nucleic Acids Res. 40, 2426–2431. doi: 10.1093/nar/gkr1073
Howe C. J., Nisbet R. E., Barbrook A. C. (2008). The remarkable chloroplast genome of dinoflagellates. J. Exp. Bot. 59, 1035–1045. doi: 10.1093/jxb/erm292
Ishida H., John U., Murray S. A., Bhattacharya D., Chan C. X. (2023). Developing model systems for dinoflagellates in the post-genomic era. J. Phycol. 59, 799–808. doi: 10.1111/jpy.13386
Jain M., Jantsch M. F., Licht K. (2019). The editor’s I on disease development. Trends Genet. 35, 903–913. doi: 10.1016/j.tig.2019.09.004
Khanaychenko A. N., Telesh I. V., Skarlato S. O. (2019). Bloom-forming potentially toxic dinoflagellates Prorocentrum cordatum in marine plankton food webs. Protistology 13, 95–125. doi: 10.21685/1680-0826-2019-13-3-1
Kim D., Paggi J. M., Park C., Bennett C., Salzberg S. L. (2019). Graph-based genome alignment and genotyping with HISAT2 and HISAT-genotype. Nat. Biotechnol. 37, 907–915. doi: 10.1038/s41587-019-0201-4
Kleinman C. L., Adoue V., Majewski J. (2012). RNA editing of protein sequences: a rare event in human transcriptomes. RNA 18, 1586–1596. doi: 10.1261/rna.033233.112
Kulandaisamy A., Zaucha J., Frishman D., Gromiha M. M. (2021). MPTherm-pred: analysis and prediction of thermal stability changes upon mutations in transmembrane proteins. J. Mol. Biol. 433, 166646. doi: 10.1016/j.jmb.2020.09.005
Li H. (2011). A statistical framework for SNP calling, mutation discovery, association mapping and population genetical parameter estimation from sequencing data. Bioinformatics 27, 2987–2993. doi: 10.1093/bioinformatics/btr509
Li H. (2013). Aligning sequence reads, clone sequences and assembly contigs with BWA-MEM. arXiv. 1303.3997v2. doi: 10.48550/arXiv.1303.3997
Li M., Wang I. X., Li Y., Bruzel A., Richards A. L., Toung J. M., et al. (2011). Widespread RNA and DNA sequence differences in the human transcriptome. Science 333, 53–58. doi: 10.1126/science.1207018
Li Q., Gloudemans M. J., Geisinger J. M., Fan B., Aguet F., Sun T., et al. (2022). RNA editing underlies genetic risk of common inflammatory diseases. Nature 608, 569–577. doi: 10.1038/s41586-022-05052-x
Li X., Xiong X., Yi C. (2016). Epitranscriptome sequencing technologies: decoding RNA modifications. Nat. Methods 14, 23–31. doi: 10.1038/nmeth.4110
Liew Y. J., Li Y., Baumgarten S., Voolstra C. R., Aranda M. (2017). Condition-specific RNA editing in the coral symbiont Symbiodinium microadriaticum. PLoS Genet. 13, e1006619. doi: 10.1371/journal.pgen.1006619
Lin S. (2011). Genomic understanding of dinoflagellates. Res. Microbiol. 162, 551–569. doi: 10.1016/j.resmic.2011.04.006
Lin S., Zhang H., Spencer D. F., Norman J. E., Gray M. W. (2002). Widespread and extensive editing of mitochondrial mRNAs in dinoflagellates. J. Mol. Biol. 320, 727–739. doi: 10.1016/S0022-2836(02)00468-0
Liu H., Li Y., Chen D., Qi Z., Wang Q., Wang J., et al. (2017). A-to-I RNA editing is developmentally regulated and generally adaptive for sexual reproduction in Neurospora crassa. Proc. Natl. Acad. Sci. U. S. A 114, E7756–E7765. doi: 10.1073/pnas.1702591114
Love M. I., Huber W., Anders S. (2014). Moderated estimation of fold change and dispersion for RNA-seq data with DESeq2. Genome Biol. 15, 550. doi: 10.1186/s13059-014-0550-8
Megyeri M., Prasad R., Volpert G., Sliwa-Gonzalez A., Haribowo A. G., Aguilera-Romero A., et al. (2019). Yeast ceramide synthases, Lag1 and Lac1, have distinct substrate specificity. J. Cell Sci. 132, jcs228411. doi: 10.1242/jcs.228411
Mungpakdee S., Shinzato C., Takeuchi T., Kawashima T., Koyanagi R., Hisata K., et al. (2014). Massive gene transfer and extensive RNA editing of a symbiotic dinoflagellate plastid genome. Genome Biol. Evol. 6, 1408–1422. doi: 10.1093/gbe/evu109
Nie W., Wang S., He R., Xu Q., Wang P., Wu Y., et al. (2020). A-to-I RNA editing in bacteria increases pathogenicity and tolerance to oxidative stress. PLoS Pathog. 16, e1008740. doi: 10.1371/journal.ppat.1008740
Nishikura K. (2006). Editor meets silencer: crosstalk between RNA editing and RNA interference. Nat. Rev. Mol. Cell Biol. 7, 919–931. doi: 10.1038/nrm2061
Novikova I. V., Hennelly S. P., Sanbonmatsu K. Y. (2012). Structural architecture of the human long non-coding RNA, steroid receptor RNA activator. Nucleic Acids Res. 40, 5034–5051. doi: 10.1093/nar/gks071
Picardi E., Pesole G. (2013). REDItools: high-throughput RNA editing detection made easy. Bioinformatics 29, 1813–1814. doi: 10.1093/bioinformatics/btt287
Piechotta M., Naarmann-de Vries I. S., Wang Q., Altmüller J., Dieterich C. (2022). RNA modification mapping with JACUSA2. Genome Biol. 23, 115. doi: 10.1186/s13059-022-02676-0
Piechotta M., Wyler E., Ohler U., Landthaler M., Dieterich C. (2017). JACUSA: site-specific identification of RNA editing events from replicate sequencing data. BMC Bioinf. 18, 7. doi: 10.1186/s12859-016-1432-8
Ramaswami G., Li J. B. (2016). Identification of human RNA editing sites: a historical perspective. Methods 107, 42–47. doi: 10.1016/j.ymeth.2016.05.011
Ramaswami G., Zhang R., Piskol R., Keegan L. P., Deng P., O'Connell M. A., et al. (2013). Identifying RNA editing sites using RNA sequencing data alone. Nat. Methods 10, 128–132. doi: 10.1038/nmeth.2330
Rieder L. E., Savva Y. A., Reyna M. A., Chang Y.-J., Dorsky J. S., Rezaei A., et al. (2015). Dynamic response of RNA editing to temperature in Drosophila. BMC Biol. 13, 1–16. doi: 10.1186/s12915-014-0111-3
Ruchika, Tsukahara T. (2021). The U-to-C RNA editing affects the mRNA stability of nuclear genes in Arabidopsis thaliana. Biochem. Biophys. Res. Commun. 571, 110–117. doi: 10.1016/j.bbrc.2021.06.098
Schaefer M., Kapoor U., Jantsch M. F. (2017). Understanding RNA modifications: the promises and technological bottlenecks of the 'epitranscriptome'. Open Biol. 7, 170077. doi: 10.1098/rsob.170077
Schrider D. R., Gout J.-F., Hahn M. W. (2011). Very few RNA and DNA sequence differences in the human transcriptome. PLoS One 6, e25842. doi: 10.1371/journal.pone.0025842
Seebens H., Schwartz N., Schupp P. J., Blasius B. (2016). Predicting the spread of marine species introduced by global shipping. Proc. Natl. Acad. Sci. U. S. A 113, 5646–5651. doi: 10.1073/pnas.1524427113
Shah S., Dougan K. E., Chen Y., Bhattacharya D., Chan C. X. (2023). Gene duplication is the primary driver of intraspecific genomic divergence in coral algal symbionts. Open Biol. 13, 230182. doi: 10.1098/rsob.230182
Shah S., Dougan K. E., Chen Y., Lo R., Laird G., Fortuin M. D. A., et al. (2024). Massive genome reduction predates the divergence of Symbiodiniaceae dinoflagellates. ISME J. wrae059. doi: 10.1093/ismejo/wrae059
Shang R., Lee S., Senavirathne G., Lai E. C. (2023). microRNAs in action: biogenesis, function and regulation. Nat. Rev. Genet. 24, 816–833. doi: 10.1038/s41576-023-00611-y
Shoguchi E., Shinzato C., Kawashima T., Gyoja F., Mungpakdee S., Koyanagi R., et al. (2013). Draft assembly of the Symbiodinium minutum nuclear genome reveals dinoflagellate gene structure. Curr. Biol. 23, 1399–1408. doi: 10.1016/j.cub.2013.05.062
Shoguchi E., Yoshioka Y., Shinzato C., Arimoto A., Bhattacharya D., Satoh N. (2020). Correlation between organelle genetic variation and rna editing in dinoflagellates associated with the coral Acropora digitifera. Genome Biol. Evol. 12, 203–209. doi: 10.1093/gbe/evaa042
Sommer B., Köhler M., Sprengel R., Seeburg P. H. (1991). RNA editing in brain controls a determinant of ion flow in glutamate-gated channels. Cell 67, 11–19. doi: 10.1016/0092-8674(91)90568-J
Stephens T. G., Ragan M. A., Bhattacharya D., Chan C. X. (2018). Core genes in diverse dinoflagellate lineages include a wealth of conserved dark genes with unknown functions. Sci. Rep. 8, 17175. doi: 10.1038/s41598-018-35620-z
Vaquerizas J. M., Kummerfeld S. K., Teichmann S. A., Luscombe N. M. (2009). A census of human transcription factors: function, expression and evolution. Nat. Rev. Genet. 10, 252–263. doi: 10.1038/nrg2538
Vogt G., Woell S., Argos P. (1997). Protein thermal stability, hydrogen bonds, and ion pairs. J. Mol. Biol. 269, 631–643. doi: 10.1006/jmbi.1997.1042
Waller R. F., Jackson C. J. (2009). Dinoflagellate mitochondrial genomes: stretching the rules of molecular biology. Bioessays 31, 237–245. doi: 10.1002/bies.200800164
Wang L., Li J., Hou X., Yan H., Zhang L., Liu X., et al. (2020). Genome-wide identification of RNA editing sites affecting intramuscular fat in pigs. Animals 10, 1616. doi: 10.3390/ani10091616
Wang Y., Morse D. (2006). Rampant polyuridylylation of plastid gene transcripts in the dinoflagellate Lingulodinium. Nucleic Acids Res. 34, 613–619. doi: 10.1093/nar/gkj438
Wang I. X., So E., Devlin J. L., Zhao Y., Wu M., Cheung V. G. (2013). ADAR regulates RNA editing, transcript stability, and gene expression. Cell Rep. 5, 849–860. doi: 10.1016/j.celrep.2013.10.002
Wisecaver J. H., Hackett J. D. (2011). Dinoflagellate genome evolution. Annu. Rev. Microbiol. 65, 369–387. doi: 10.1146/annurev-micro-090110-102841
Zaheri B., Morse D. (2021). Assessing nucleic acid binding activity of four dinoflagellate cold shock domain proteins from Symbiodinium kawagutii and Lingulodinium polyedra. BMC Mol. Cell Biol. 22, 27. doi: 10.1186/s12860-021-00368-4
Zauner S., Greilinger D., Laatsch T., Kowallik K. V., Maier U. G. (2004). Substitutional editing of transcripts from genes of cyanobacterial origin in the dinoflagellate Ceratium horridum. FEBS Lett. 577, 535–538. doi: 10.1016/j.febslet.2004.10.060
Zhang H., Bhattacharya D., Maranda L., Lin S. (2008). Mitochondrial cob and cox1 genes and editing of the corresponding mRNAs in Dinophysis acuminata from Narragansett Bay, with special reference to the phylogenetic position of the genus Dinophysis. Appl. Environ. Microbiol. 74, 1546–1554. doi: 10.1128/AEM.02103-07
Keywords: dinoflagellates, protist, RNA editing, genomics, transcriptomics, molecular regulation
Citation: Chen Y, Dougan KE, Bhattacharya D and Chan CX (2024) Nuclear genomes of dinoflagellates reveal evolutionarily conserved pattern of RNA editing relative to stress response. Front. Protistol. 2:1320917. doi: 10.3389/frpro.2024.1320917
Received: 13 October 2023; Accepted: 24 April 2024;
Published: 10 May 2024.
Edited by:
Raúl A. González-Pech, The Pennsylvania State University (PSU), United StatesCopyright © 2024 Chen, Dougan, Bhattacharya and Chan. This is an open-access article distributed under the terms of the Creative Commons Attribution License (CC BY). The use, distribution or reproduction in other forums is permitted, provided the original author(s) and the copyright owner(s) are credited and that the original publication in this journal is cited, in accordance with accepted academic practice. No use, distribution or reproduction is permitted which does not comply with these terms.
*Correspondence: Cheong Xin Chan, Yy5jaGFuMUB1cS5lZHUuYXU=